What is Amazon SageMaker Model Training?
Amazon SageMaker Model Training reduces the time and cost to train and tune machine learning (ML) models at scale without the need to manage infrastructure. You can take advantage of the highest-performing ML compute infrastructure currently available, and SageMaker can automatically scale infrastructure up or down, from one to thousands of GPUs. Since you pay only for what you use, you can manage your training costs more effectively. To train deep learning models faster, SageMaker helps you select and refine datasets in real time. SageMaker distributed training libraries can automatically split large models and training datasets across AWS GPU instances, or you can use third-party libraries, such as DeepSpeed, Horovod, or Megatron. Train foundation models (FMs) for weeks and months without disruption by automatically monitoring and repairing training clusters.
How it works
Train and tune ML models at scale with state-of-the art ML tools and the highest performing ML compute infrastructure.
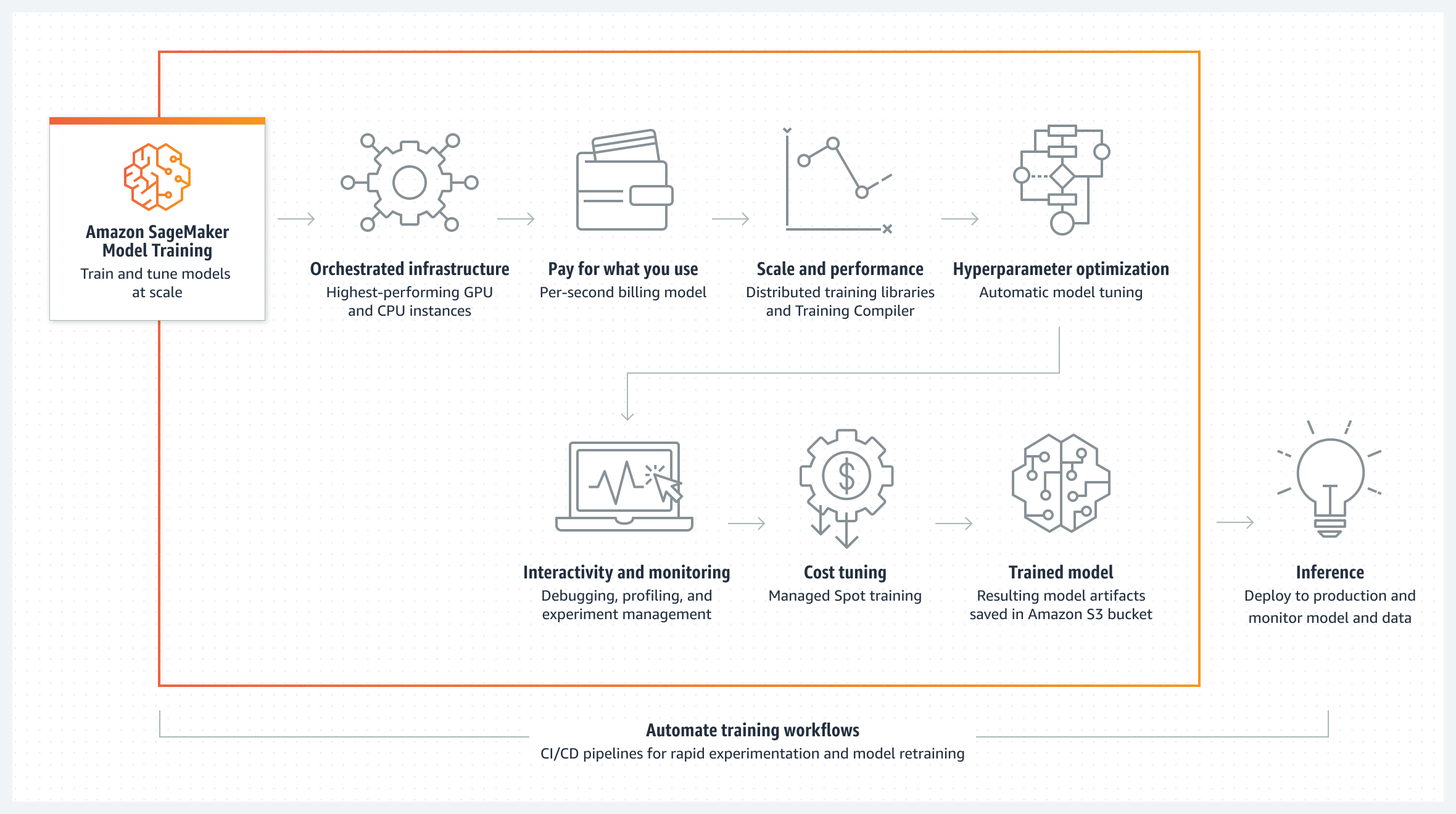
Benefits of cost effective training
Train models at scale
Fully managed training jobs
Amazon SageMaker training jobs offer a fully managed user experience for large distributed FM training, removing the undifferentiated heavy lifting around infrastructure management. SageMaker training jobs automatically spins up a resilient distributed training cluster, monitors the infrastructure, and auto-recovers from faults to ensure a smooth training experience. Once the training is complete, SageMaker spins down the cluster and you are billed for the net training time. In addition, with SageMaker training jobs, you have the flexibility to choose the right instance type to best fits an individual workload (e.g., pre-train an LLM on a P5 cluster or fine tune an open source LLM on p4d instances) to further optimize your training budget. In addition, it offers a consistent user experience across ML teams with varying levels of technical expertise and different workload types.
Amazon SageMaker HyperPod
Amazon SageMake HyperPod is a purpose-built infrastructure to efficiently manage compute clusters to scale foundation model (FM) development. It enables advanced model training techniques, infrastructure control, performance optimization, and enhanced model observability. SageMaker HyperPod is preconfigured with Amazon SageMaker distributed training libraries, allowing you to automatically split models and training datasets across AWS cluster instances to help efficiently utilize the cluster’s compute and network infrastructure. It enables a more resilient environment by automatically detecting, diagnosing, and recovering from hardware faults, allowing you to continually train FMs for months without disruption, reducing training time by up to 40%.
High-performance distributed training
With only a few lines of code, you can add either data parallelism or model parallelism to your training scripts. SageMaker makes it faster to perform distributed training by automatically splitting your models and training datasets across AWS GPU instances.
Built-in tools for the highest accuracy and lowest cost
Automatic model tuning
SageMaker can automatically tune your model by adjusting thousands of algorithm parameter combinations to arrive at the most accurate predictions, saving weeks of effort. It helps you to find the best version of a model by running many training jobs on your dataset.
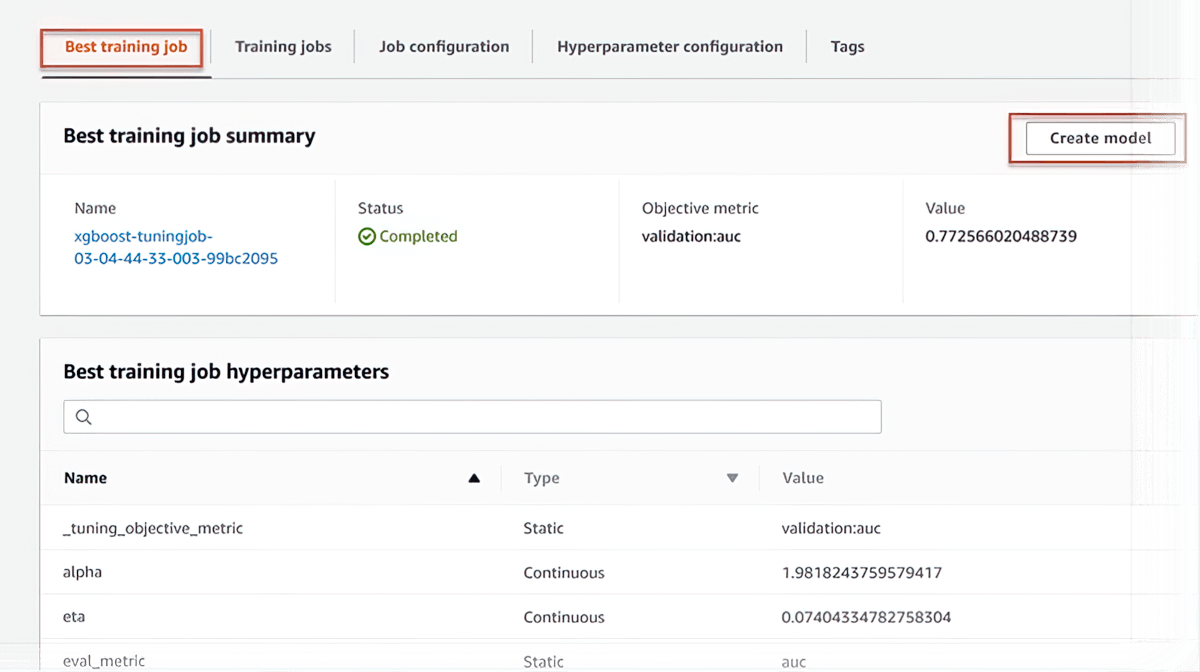
Managed Spot training
SageMaker helps reduce training costs by up to 90 percent by automatically running training jobs when compute capacity becomes available. These training jobs are also resilient to interruptions caused by changes in capacity.
Debugging
Amazon SageMaker Debugger captures metrics and profiles training jobs in real time, so you can quickly correct performance issues before deploying the model to production. You can also remotely connect to the model training environment in Amazon SageMaker for debugging with access to the underlying training container.
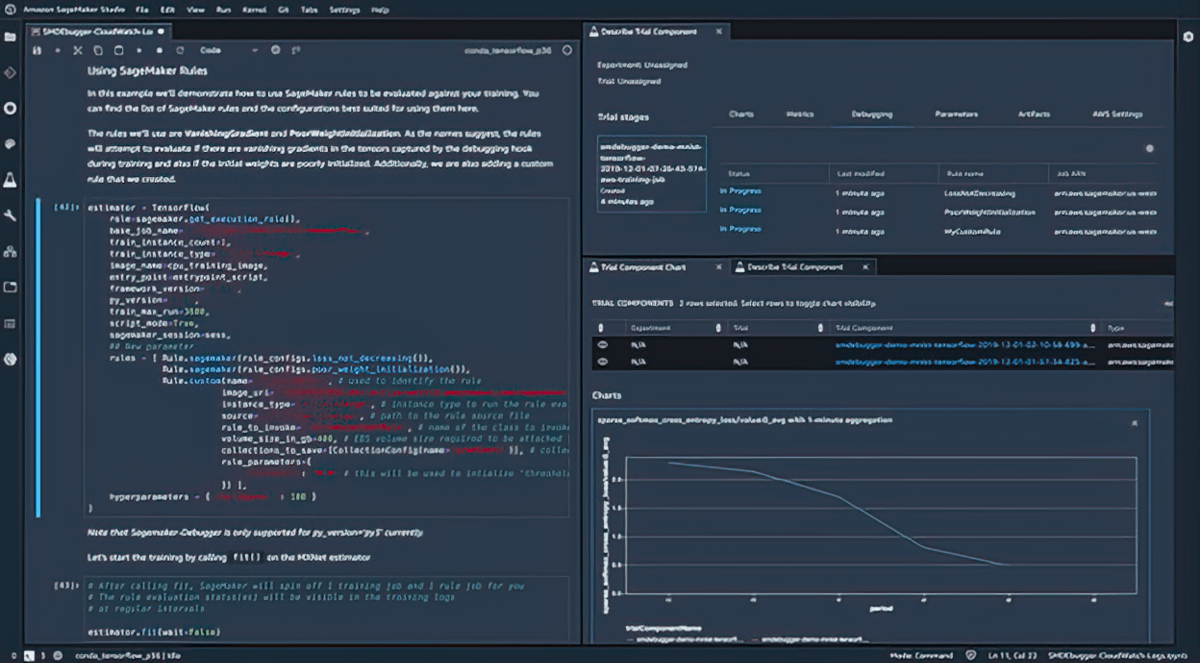
Profiler
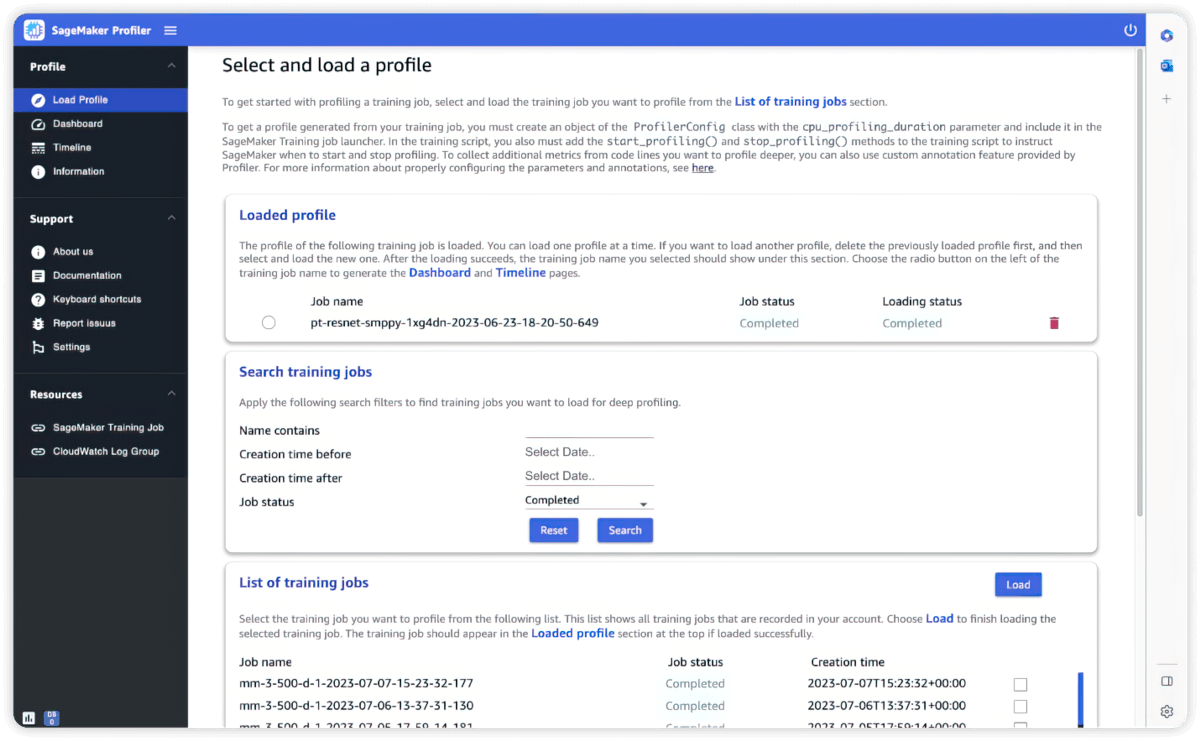
Built-in tools for interactivity and monitoring
Amazon SageMaker with MLflow
Leverage MLflow with SageMaker training to capture input parameters, configurations, and results, enabling you to quickly identify the best-performing models for your use case. The MLflow UI allows you to analyze model training attempts and effortlessly register candidate models for production with a single click.
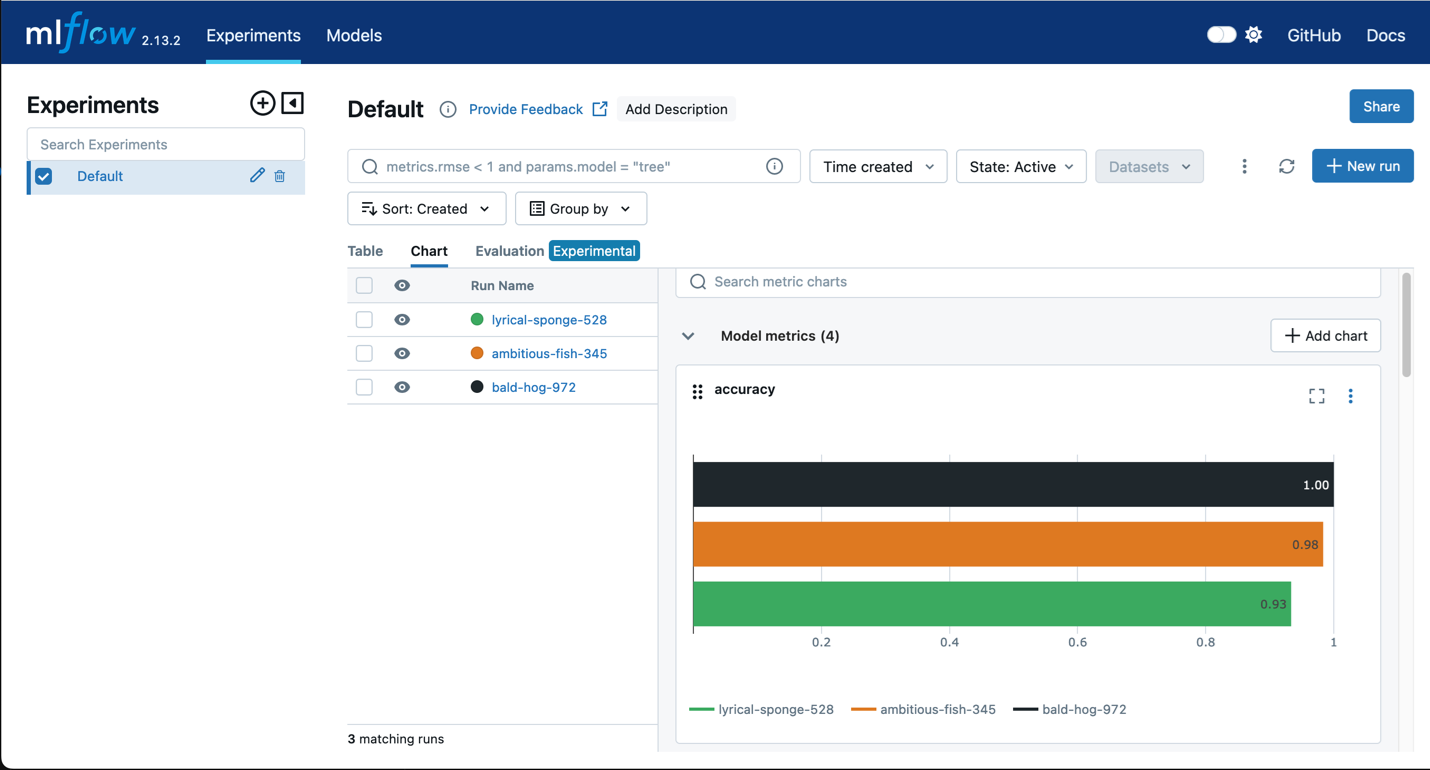
Amazon SageMaker with TensorBoard
Amazon SageMaker with TensorBoard helps you to save development time by visualizing the model architecture to identify and remediate convergence issues, such as validation loss not converging or vanishing gradients.
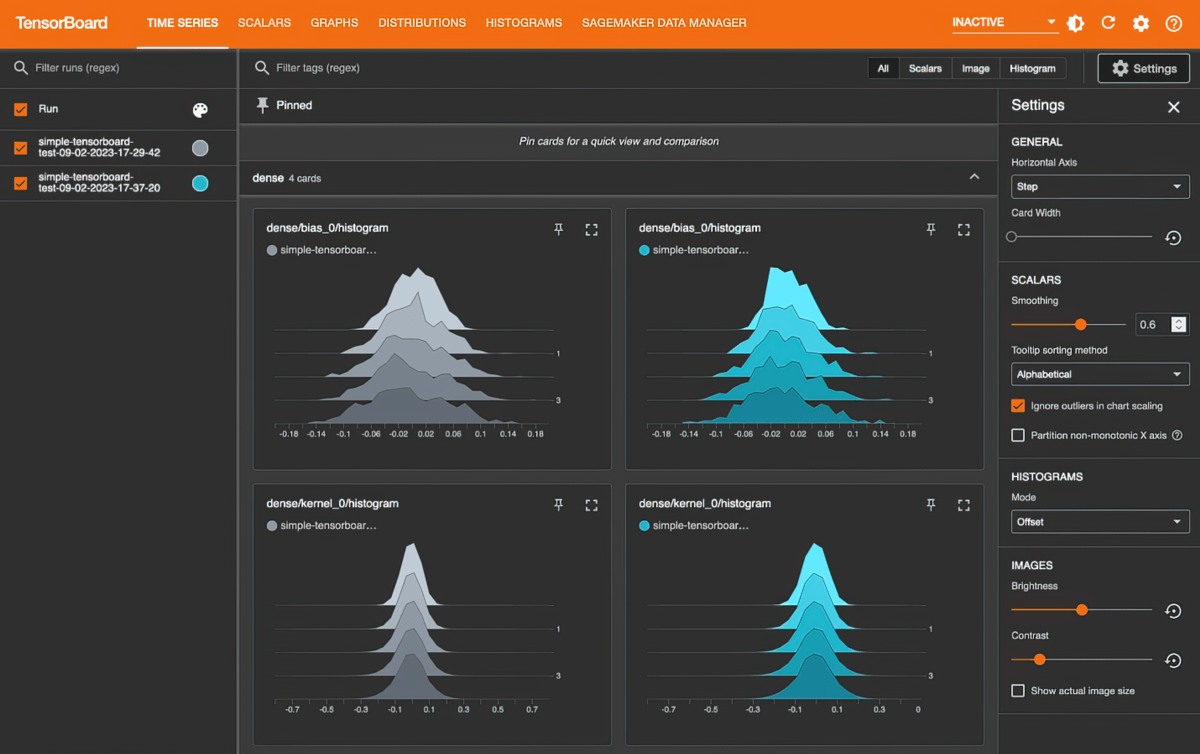
Flexible and faster training
Full customization
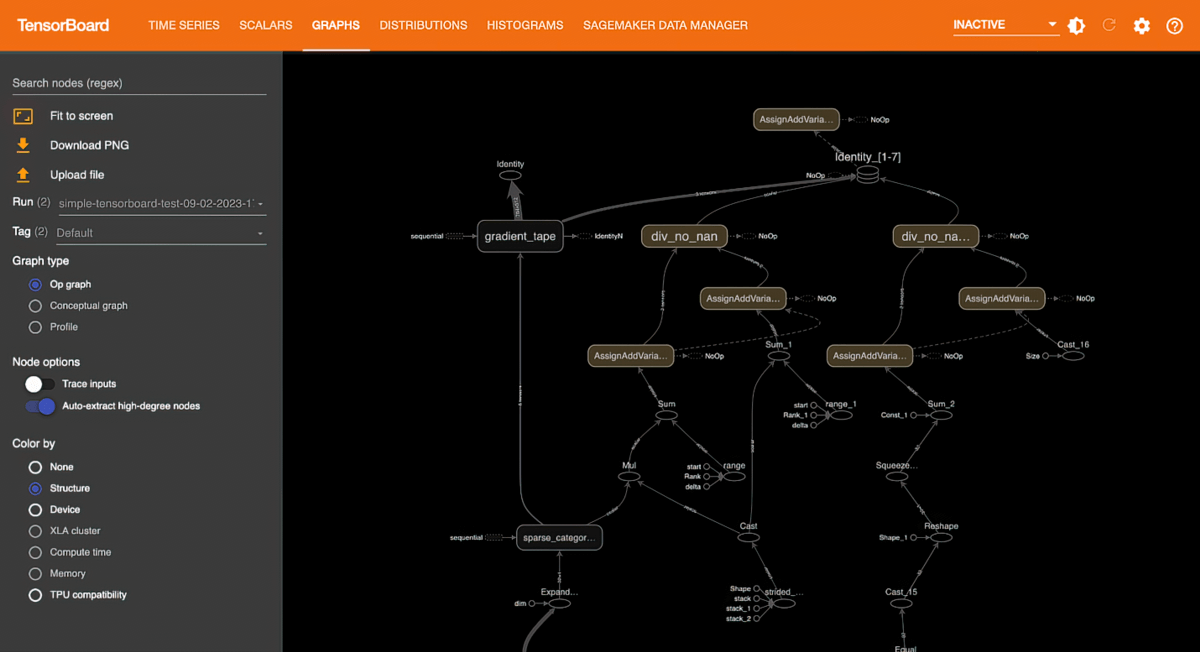
Local code conversion
Amazon SageMaker Python SDK helps you execute ML code authored in your preferred IDE and local notebooks along with the associated runtime dependencies as large-scale ML model training jobs with minimal code changes. You only need to add a line of code (Python decorator) to your local ML code. SageMaker Python SDK takes the code along with the datasets and workspace environment setup and runs it as a SageMaker Training job.
Automated ML training workflows
Automating training workflows using Amazon SageMaker Pipelines helps you create a repeatable process to orchestrate model development steps for rapid experimentation and model retraining. You can automatically run steps at regular intervals or when certain events are initiated, or you can run them manually as needed.