¿Qué le pareció este contenido?
La naturaleza al frente del camino: cómo Sakana AI está transformando la creación de modelos
El auge de la inteligencia artificial (IA) generativa ha hecho que los cambios se produzcan a un ritmo frenético. Ahora las empresas están totalmente centradas en crear modelos de mayor rendimiento, y cada día surgen un gran número de modelos de lenguaje de gran tamaño (LLM) nuevos y mejorados. El modelo de transformador, de eficacia probada, ha estado en el centro del auge de la IA generativa, ya que ha permitido a los fundadores escalar y lanzar con rapidez nuevos LLM.
Sin embargo, estas nuevas versiones a menudo conllevan un costo superior, ya que demandan más potencia de procesamiento y recursos. Al mismo tiempo, las versiones anteriores de LLM pueden quedar eclipsadas con rapidez por modelos de mayor tamaño y que consumen más recursos de computación. En un contexto de escasez mundial de GPU, que ponía el límite en la escalabilidad práctica del entrenamiento del modelo, los cofundadores David Ha y Llion Jones sentían curiosidad por encontrar una forma más eficiente de ampliar las fronteras de la IA. Juntos emprendieron un viaje de investigación para explorar técnicas creativas para el desarrollo de modelos fundacionales (FM) inspirados en una fuente de energía diferente: el poder de la naturaleza.
Sakana AI, su startup con sede en Tokio, lidera en la actualidad una nueva tendencia en el entrenamiento del modelo de IA, que se basa en la creación de LLM de vanguardia diseñados a partir de otros ya existentes. Desde que fundaron la empresa en 2023, su investigación ya está abriendo nuevos caminos al sacar partido de recursos que a menudo se pasan por alto. Con base en ideas ancestrales como la evolución y la selección natural, la empresa está avanzando hacia un futuro en el que los FM hereden de manera automática los rasgos más destacados de versiones anteriores. De este modo, tienen como objetivo un método de entrenamiento en el que los modelos evolucionan de manera constante y se adaptan a entornos cambiantes.
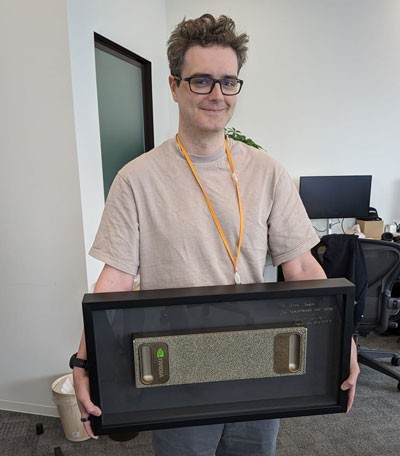
Adoptar las nuevas generaciones de IA
Gracias a su auténtico espíritu empresarial, la startup no solo espera la llegada del próximo cambio en la IA generativa, sino que está adentrándose en lo desconocido para descubrir qué vendrá después. Jones, Chief Technology Officer de Sakana AI, explica por qué dejaron sus puestos en las principales empresas de tecnología para fundar Sakana AI: “David y yo no estábamos llevando a cabo la investigación especulativa a largo plazo que queríamos hacer, así que sabíamos que teníamos que empezar por nuestra cuenta”. Al observar la existencia de patrones históricos en el desarrollo tecnológico, los cofundadores vieron la oportunidad de realizar descubrimientos significativos.
Jones agrega: “pienso que la investigación de la IA pasa por fases de exploración y explotación. La gente prueba diferentes enfoques hasta que encuentran algo que funciona bien; luego, todo el mundo se centra en explotar esa tecnología. Pero, aunque haya tanta expectación sobre la forma en que el modelo de transformador entrena a la IA generativa, significa que no estamos explorando más allá de eso”.
El modelo de transformador supuso un gran avance en la arquitectura de aprendizaje profundo en 2017 y desde entonces ha conquistado el mundo. A diferencia de los modelos anteriores, el de transformador puede entrenarse con conjuntos de datos mucho más grandes, usarse para una variedad de tareas y comprender mejor los textos que lee y escribe. Sin embargo, una escalabilidad mucho mayor conlleva la necesidad de una mayor capacidad de cómputo, tanta que los fabricantes de hardware no han podido crear chips de inteligencia artificial con la rapidez suficiente para satisfacer la demanda.
Sakana AI está explorando métodos de entrenamiento del modelo alternativos y más sostenibles. Takuya Akiba, Research Scientist de Sakana AI, explica: “todo el mundo converge hacia objetivos similares cuando entrenamos modelos. Por este motivo, no vemos mucha diferencia en los resultados. En Sakana AI estamos creando un nuevo paradigma inspirado en la naturaleza. Esto nos permite encontrar nuevas aplicaciones que no serían posibles solamente escalando”.
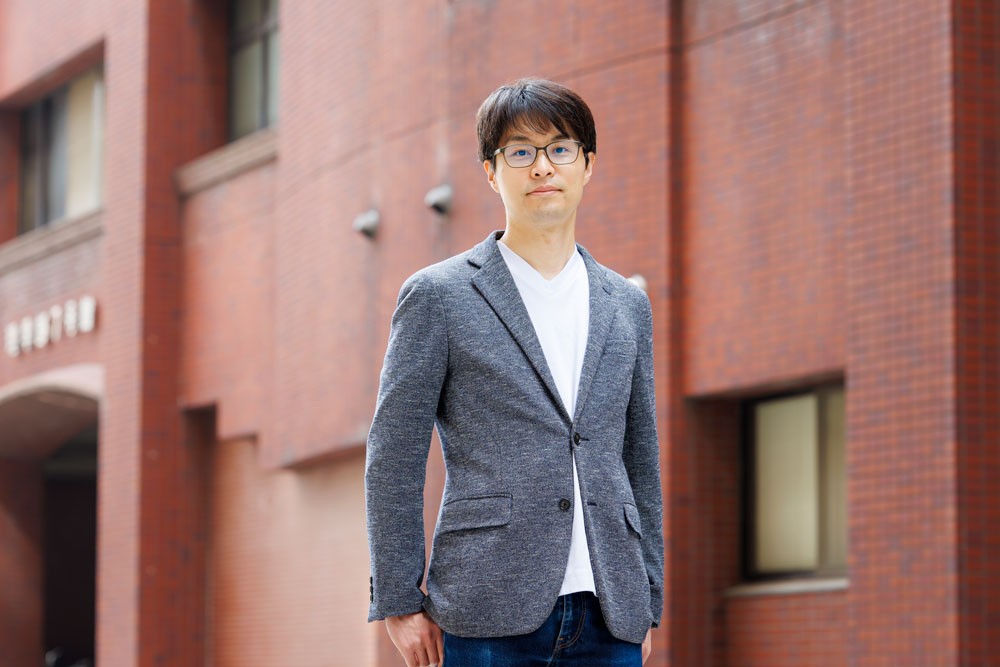
Aprovechar la sabiduría de la naturaleza
“Sakana”, que significa “pez” en japonés, alude a sus técnicas inspiradas en la naturaleza y la influencia evolutiva. El logotipo representa con fidelidad sus métodos pioneros: muestra un banco de peces nadando en una dirección, mientras que un pez rojo nada de manera desafiante en sentido contrario. El gráfico también captura la idea de inteligencia colectiva que inspira su pensamiento, es decir, la idea de que los modelos más pequeños pueden interactuar de manera más eficiente con menos información y recursos que los modelos grandes y densos que distribuyen gran cantidad de información.
Ahora que la tecnología está alcanzando un punto de inflexión, Sakana AI está poniendo a prueba la idea de la computación evolutiva en los FM. La técnica consolidada de entrenamiento y optimización de modelos se conoce como gradiente descendente, pero, al igual que el modelo de transformador, tiene un costo computacional elevado. Sin embargo, sería un error asumir que la eficiencia de los recursos es simplemente una necesidad imperativa para avanzar en el camino de una startup. El equipo de IA de Sakana lo ve como una ventaja estratégica que les permite pensar de manera innovadora, maximizar los recursos disponibles y fomentar la innovación. Como dice Jones: “Creo que esa limitación significa que podemos encontrar cosas más interesantes”.
“Nuestra filosofía es ‘aprender siempre suma’. Y para aprender cosas, no basta con utilizar el algoritmo más popular. Debes usar diferentes técnicas, como la computación evolutiva, para buscar en esos espacios”, agrega. Desde entonces, gracias al soporte técnico estratégico de AWS, Sakana AI ha incorporado ideas de la naturaleza en el ámbito tecnológico, y ya está viendo los frutos de su trabajo.
La revolución de la combinación de modelos basada en la evolución
Un avance clave hasta la fecha es el novedoso enfoque de Sakana AI para la combinación de modelos. El equipo observó que los modelos actuales pueden aportar un enorme valor agregado, pero cientos de miles de ellos no se utilizan o se descartan cuando son reemplazados por nuevas versiones. “Ya existe un océano enorme de LLM únicos y de código abierto”, afirma Akiba.
Al combinar diferentes modelos, en lugar de entrenarlos desde cero, pueden tomar las mejores cualidades de cada uno para crear un modelo nuevo y más potente. La combinación de modelos no es una idea nueva en sí misma (la gente ha experimentado con el arte de “hackear” modelos para crear LLM especializados), pero lo que sí es novedoso es cómo Sakana AI aplica un algoritmo inspirado en la naturaleza para automatizar el proceso.
Basta con considerar el proceso de selección natural. Las especies han evolucionado con el tiempo para transmitir genes que les ayudan a adaptarse y prosperar en su entorno. Mientras tanto, los rasgos que amenazaban la supervivencia de las especies acababan desapareciendo. Del mismo modo, los algoritmos evolutivos de Sakana AI pueden encontrar las combinaciones óptimas de diferentes partes de FM para producir nuevos FM basados en la selección natural que funcionen bien para una aplicación en particular. El nuevo modelo hereda las características ganadoras de los modelos anteriores en función de lo que el usuario haya especificado. Está muy lejos de un enfoque al estilo Frankenstein basado en combinar diferentes elementos del modelo.
Las técnicas de combinación de modelos anteriores se basaban en la experiencia humana, el conocimiento del dominio y la intuición, todos los cuales tienen límites. “Al desarrollar diferentes formas de combinar los algoritmos, conseguimos un modelo combinado mejor que el que un humano podría diseñar a mano”, explica Llion. “Siempre que consigas que una computadora busque en un espacio de soluciones para ti, ganas. Eso es mejor que un humano que intente hacerlo de forma manual, porque la computadora puede hacerlo más rápido y probar más cosas y, además, tiene más paciencia”.
Solo los FM más aptos sobreviven
La diversidad de modelos abiertos y tareas que puede realizar la IA generativa sigue en aumento, lo que significa que el enfoque mucho más sistemático de Sakana AI para la fusión de modelos será cada vez más importante. Como afirma Akiba: “hay un número casi infinito de formas de combinar diferentes modelos, por lo que necesitamos estos modelos de optimización heurística”. En sus experimentos, Sakana AI permitió que el proceso de evolución funcionara durante unos cientos de generaciones, cuando los modelos con mejor puntuación sobreviven para repoblar la siguiente generación.
Ya se ha demostrado que el enfoque de combinación de modelos evolutivos hace evolucionar los FM de formas, a menudo poco intuitivas, pero muy eficaces. Por ejemplo, si bien hay una gran cantidad de modelos de código abierto en Japón, ninguno de ellos podía manejar las matemáticas anteriormente porque no existe un conjunto de datos matemáticos japoneses. En lugar de empezar desde cero y entrenar un nuevo modelo, Sakana AI fusionó un modelo que hablaba japonés con fluidez con un modelo en inglés que es bueno en matemáticas, pero que no habla japonés.
El resultado fue un LLM de última generación con un razonamiento japonés mejorado y sólidas capacidades matemáticas, y que brinda un rendimiento excepcionalmente bueno en comparación con los puntos de referencia en ambas áreas. La combinación manual de estos modelos habría sido increíblemente difícil, especialmente cuando se manejaban dominios tan distintos. Al automatizar el proceso, la startup puede transformar rápidamente los FM existentes y llevar sus cualidades únicas a diferentes culturas.
Sakana AI ha descubierto que los algoritmos evolutivos no solo admiten los LLM de texto, sino que también han fusionado con éxito los LLM con modelos de lenguaje visual japoneses. De hecho, el modelo resultante mejoró la precisión de las preguntas relacionadas con imágenes e incluso permitió aprender matices y conocimientos culturales específicos sobre Japón. El equipo también ha obtenido resultados prometedores al aplicar el mismo método a diferentes modelos de generación y difusión de imágenes.
El poder de adaptarse y aprender
Abrir nuevos caminos en la IA generativa requiere experiencia y conocimientos especializados además de una base técnica sólida compuesta por soluciones flexibles y rentables. AWS proporciona esas soluciones a Sakana AI, además de orientación estratégica y créditos a través del programa AWS Activate. El acceso a la financiación les ha permitido experimentar con su enfoque inspirado en la naturaleza en la nube de AWS sin el obstáculo de los costos iniciales. El soporte técnico personalizado del equipo de startups de AWS también les ha permitido progresar y publicar los resultados rápidamente.
Elegir las instancias de Amazon EC2 adecuadas es solo una de las formas mediante las que impulsan su investigación: el alquiler de instancias bajo demanda o bloques de capacidad les permite seguir siendo ágiles y seleccionar las mejores opciones en cualquier momento. Este enfoque en la potencia de computación también ha contribuido a reducir los costos y a ocupar mucha menos memoria de lo que se habría necesitado con los métodos de descenso gradual. Akiba explica: “AWS comprende perfectamente nuestra carga de trabajo y lo que estamos intentando lograr. Nos ha ayudado a superar con rapidez desafíos, como los problemas de capacidad”.
Inspirados por la ambición e inteligencia de Sakana AI, AWS ha ayudado a la empresa desde el primer día. Como afirma Yoshitaka Haribara, Solutions Architect de AWS: “Es un placer trabajar con un equipo con tanto talento que está en la cima de su carrera. Estamos encantados de ver los interesantes resultados de sus investigaciones y esperamos que AWS pueda seguir respaldando sus esfuerzos ofreciéndoles recursos, experiencia y pensamiento creativo”.
Akiba destacó que la asociación con AWS y sus servicios permitieron a la empresa empezar de inmediato: “somos un equipo bastante pequeño, por lo que no teníamos un ingeniero de plataformas para configurar un clúster. Es muy fácil usar los servicios de AWS, lo que ha facilitado la exploración de nuestra investigación”.
Exploración de nuevas fronteras de la IA
Si bien el espacio de la IA generativa es muy competitivo y evoluciona a buen ritmo, la investigación de Sakana AI promete acelerar aún más el progreso. “En este momento, existe una competencia entre los modelos propietarios y los modelos de código abierto, y muchos piensan que los modelos propietarios van por delante. Sin embargo, creo que nuestra investigación puede cambiar las reglas del juego para acelerar la creación de modelos de código abierto y el desarrollo de nuevas habilidades en la comunidad”, afirma Akiba.
Sakana AI continúa investigando a fondo de qué manera las nuevas técnicas pueden crear ciclos de innovación más rápidos. Sin embargo, como señala Jones, no buscan recompensas rápidas: “nuestro enfoque de exploración a largo plazo hace que sea mucho más difícil ver lo que nos depara el futuro. Pero me siento muy cómodo con ese riesgo porque es sumamente emocionante explorar temas tan fascinantes”.
A medida que Sakana AI gana impulso en varios proyectos, están estudiando cómo otros servicios de AWS pueden respaldar las pruebas de concepto, como el uso de Amazon Bedrock para ampliar el uso de modelos básicos como Claude de Anthropic. Más allá de las técnicas de combinación de modelos, la empresa también está investigando cómo hacer evolucionar los sistemas inteligentes basados en agentes, y AWS respalda su visión en este emocionante espacio.
Jones tiene grandes esperanzas puestas en el ritmo actual de avance de la tecnología: “dado que la cantidad de computación utilizada para entrenar modelos sigue duplicándose cada seis meses, podríamos alcanzar una inteligencia a nivel humano si seguimos mejorando los algoritmos de entrenamiento y optimizando la forma en que los colocamos en un agente. Si luego pudiéramos reclutar 10 000 agentes de inteligencia artificial para resolver un problema, sería posible que una semana equivaliese a dos de años de investigación científica”. Desde automatizar el descubrimiento de fármacos hasta mejorar operaciones básicas de computación, la investigación podría resolver algunos de mayores desafíos del mundo.
Trabajar en equipo con socios como AWS ha sido crucial para el viaje de Sakana AI, y es solo el comienzo del valor a largo plazo que aún tienen que alcanzar. ¿Su consejo para otras startups que buscan expandir el potencial de la IA generativa? A Jones le encantaría ver a otros fundadores sacar partido de su libertad profundizando en la tecnología: “sé ambicioso con tus ideas. No te apresures por la fiebre del oro ni lances la primera versión de una aplicación por ser el primero: tómate tu tiempo para explorar”.
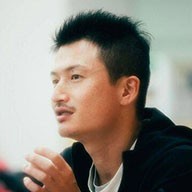
Kan Kato
Kan Kato es gerente de desarrollo empresarial de startups en AWS y ha demostrado su compromiso de fomentar el éxito y la innovación de las startups mediante asociaciones estratégicas con empresas de capital riesgo, aceleradoras e iniciativas de desarrollo empresarial. Además de trabajar para startups, también le gusta jugar al fútbol y entrenar para triatlones.
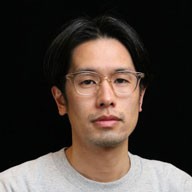
Arata Yanase
Arata Yanase es gerente de cuentas de startups en AWS Japón. Tras haber trabajado anteriormente como responsable de ventas y desarrollo empresarial en una startup de tecnología financiera, dirigió las medidas para el desarrollo comercial y el crecimiento de los servicios con el fin de captar usuarios.
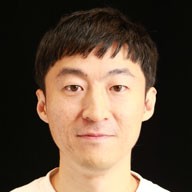
Yoshitaka Haribara
Yoshitaka Haribara es arquitecto sénior de soluciones de ML para startups en AWS Japón. En este puesto, Yoshitaka ayuda a las startups a innovar en IA generativa en AWS. En su tiempo libre, a Yoshitaka le gusta tocar la batería.
¿Qué le pareció este contenido?