1.背景
近年来云上的成本优化日益成为热门话题和上云客户的重要任务,EC2 Spot 凭借高达 90% 的折扣价成为成本优化利器,应用在各种成本优化方案中。比如在本系列 blog(一)中,我们讨论的基于 EMR on EKS 的 Spark 集群成本优化方案,就是通过 Karpenter 管理 Spot 和 Graviton。但是 EC2 Spot 作为 EC2 的空闲资源容量是动态变化的,且会因为价格过低、容量不足、或不满足创建 Spot 请求当中的约束条件而中断。因此,很多使用的 Spot 客户经常会遇到以下痛点:
- 无法启动新的 Spot 实例
- 如何选择中断率低的 Spot 实例
- Spot 中断后如何让应用优雅退出
- 是否有方法模拟 Spot 中断验证当前应用程序的可用性
借由本次博客系列 blog(三),我们希望在探讨该方案的可靠性的过程中,以 AWS Well-Architected Framework 的六大支柱之一“可靠性支柱”为出发点,总结出 Spot 容量和中断的最佳实践,帮助客户实现 EC2 Spot 的高可靠管理和部署;并且通过 AWS Fault Injection Simulator 全托管服务模拟 Spot 中断故障,验证该方案下应用的可靠性。
2.Spot 重要知识点
Spot 容量池
不同实例系列、不同实例大小、不同可用区组合成一个容量池。
灵活性是成功使用 Spot 的基本原则,其中使用尽可能多且深的容量池(实例灵活,区域灵活)是我们使用 Spot 时的第一原则。对于每种工作负载,最佳实践是至少使用 10 种实例类型。
Spot 选型工具
Spot 服务发展至今,已经开发了越来越多便利的工具帮助我们更好的实现 Spot 实例选型,比如:
由于目前 Karpenter 还不支持 ABIS 功能,本次方案部署时采用 Spot instance advisor 帮助实例选型。
Spot 中断管理
EC2 Spot 现支持两种中断通知:
- 提前两分钟发出的“EC2 Spot Interruption Notifications”通知(简称 ITN);
- 在 Spot 实例处于中断高风险的情况下发出的“EC2 Instance Rebalance Recommendation”通知(简称 RBR)。该信号可能比该两分钟的 Spot 实例中断通知更早到达,从而让您有机会主动管理 Spot 实例。您可以决定将工作负载再平衡到不处于较高中断风险的新的或现有的 Spot 实例。
很多 AWS 托管服务例如 Amazon ECS、Amazon EKS、AWS Batch 和 AWS Elastic Beanstalk 内置支持管理 EC2 Spot 实例生命周期,这些服务通过与 EC2 Auto Scaling 集成可以响应 Spot 中断。
Spot 理想工作负载
因其中断特性,理想的工作负载需要具备可容错、松散耦合、灵活或者无状态的特点。因为容器的工作负载大多数时无状态的,所以容器与 Spot 结合堪称天作之合。
从 v0.19.0 开始,Karpenter 开始内置了对 Spot 中断的处理 ,不用再额外维护一个 Node Termination Handler 组件,进一步简化运维。Karpenter 使用 SQS 队列和 EventBridge 规则来处理即将发生的非自愿中断事件包括:
- Spot Interruption Warnings
- Scheduled Change Health Events (Maintenance Events)
- Instance Terminating Events
- Instance Stopping Events
当 Karpenter 检测到其中一个事件将发生在您的节点上时,它会自动在中断事件之前连接、耗尽和终止节点,以便在中断之前提供最大工作负载清理时间。
Karpenter 要求预置 SQS 队列,并添加 EventBridge 规则和目标,将中断事件从 AWS 服务转发到 SQS 队列。系列blog(一)中安装 Karpenter 时使用的入门指南的 CloudFormation 模板中已经提供了配置此基础设施的详细信息。
3.混沌工程测试工具 – AWS Fault Injection Simulator 全托管服务
AWS Fault Injection Simulator(FIS)是一项完全托管式服务,用于运行故障注入实验,以便改进应用程序的性能、可观测性和恢复能力。FIS 简化了跨一系列 AWS 服务来设置和运行受控故障注入实验的过程。
AWS FIS 实验注入的 Spot 实例中断的行为方式与其在 Amazon EC2 回收时相同(包括实例终止通知、重新平衡通知和您指定的中断行为),因此可以准确地再现真实状况。我们只需运行 AWS FIS 实验就可以模拟当 Amazon EC2 回收 Spot 实例时会发生的情况,让我们轻松可以观察应用程序是如何响应的,从而提高其性能和弹性。
在理解 Spot 重要特性和混沌工程工具 FIS 之后,我们可以开始进行可靠性测试 — FIS 模拟 Spot 中断观察计算结果。
4. 可靠性测试
第一步:配置 Fault Toleration Simulator 故障注入实验 – Spot 中断
首先创建用于 FIS 实验的 IAM Role:
cat <<EOF >fis-role-trust-policy.json
{
"Version": "2012-10-17",
"Statement": [
{
"Effect": "Allow",
"Principal": {
"Service": [
"fis.amazonaws.com"
]
},
"Action": "sts:AssumeRole"
}
]
}
EOF
aws iam create-role --role-name fis-role --assume-role-policy-document file://fis-role-trust-policy.json
aws iam attach-role-policy --role-name fis-role --policy-arn arn:aws:iam::aws:policy/service-role/AWSFaultInjectionSimulatorEC2Access
aws iam attach-role-policy --role-name fis-role --policy-arn arn:aws:iam::aws:policy/CloudWatchLogsFullAccess
创建 FIS 实验:
- 通过
resourceType
,resourceTags
和 filters
限定只选择本实验的 EKS 集群中 Running 状态的 Spot 实例作为 Spot 中断实验的对象。
- 配置将 FIS 日志传送到对应的 cloudwatch 日志组“fis-experiment-logging”中。
- 设置“durationBeforeInterruption”:“PT2M”模拟在中断 Spot 前 2 分钟发出 Spot 实例中断通知。
aws logs create-log-group --log-group-name=fis-experiment-logging
cat <<EOF > fis_experiment.json
{
"description": "interrupt random spot instance in EKS cluster $CLUSTER_NAME",
"targets": {
"SparkExecutor": {
"resourceType": "aws:ec2:spot-instance",
"resourceTags": {
"aws:eks:cluster-name": "$CLUSTER_NAME"
},
"filters": [
{
"path": "State.Name",
"values": [
"running"
]
}
],
"selectionMode": "COUNT(1)"
}
},
"actions": {
"interrupt": {
"actionId": "aws:ec2:send-spot-instance-interruptions",
"description": "interrupt Spot instances in EKS cluster $CLUSTER_NAME",
"parameters": {
"durationBeforeInterruption": "PT2M"
},
"targets": {
"SpotInstances": "SparkExecutor"
}
}
},
"stopConditions": [
{
"source": "none"
}
],
"roleArn": "arn:aws:iam::$AWS_ACCOUNT_ID:role/fis-role",
"logConfiguration": {
"cloudWatchLogsConfiguration": {
"logGroupArn": "arn:aws:logs:us-east-1:$AWS_ACCOUNT_ID:log-group:fis-experiment-logging:*"
},
"logSchemaVersion": 1
},
"tags": {
"Name": "Spot_Interruption"
}
}
EOF
aws fis create-experiment-template --cli-input-json file://fis_experiment.json
第二步:创建测试 2 的 EMR Job Json 文件
- 更改 entryPointArguments 和 logStreamNamePrefix 中的 Demo1 为 Demo2,替换 EMR_on_EKS_ClusterID 还有 EMR_execution_role_ARN。
- 创建该测试环境时 EMR on EKS 最新版本是 emr-6.8.0-latest,支持 Spark 3.3.0-amzn-0。自 EMR 6.3.0 版本开始,其支持的 Spark 版本更新到 3.1.1,因此支持 Spark Node Decommission 功能。启用该功能 Spark 可以在 Spot 中断时复制 shuffle data 和缓存数据到其他 executor,使得 Spark 在使用 EC2 Spot 进行部署时更具弹性和性能。通过以下参数配置可启用该功能:
spark.decommission.enabled
spark.storage.decommission.enabled
spark.storage.decommission.rddBlocks.enabled
spark.storage.decommission.shuffleBlocks.enabled
执行以下命令创建测试 2 的 EMR Job Json 文件:
cat << EOF > start-job-run-request_wordcount_demo2.json
{
"name": "spark-demo",
"virtualClusterId": "<EMR_on_EKS_ClusterID>",
"executionRoleArn": "<EMR_execution_role_ARN>",
"releaseLabel": "emr-6.8.0-latest",
"jobDriver": {
"sparkSubmitJobDriver": {
"entryPoint": "$s3DemoBucket/wordcount.py",
"entryPointArguments": ["$s3DemoBucket/output/wordcount_output_demo2"],
"sparkSubmitParameters": "--class org.apache.spark.examples.SparkPi --conf spark.executor.instances=3 --conf spark.executor.memory=2G --conf spark.executor.cores=1 --conf spark.driver.cores=1 --conf spark.driver.memory=2G"
}
},
"configurationOverrides": {
"applicationConfiguration": [
{
"classification": "spark-defaults",
"properties": {
"spark.dynamicAllocation.enabled":"true",
"spark.dynamicAllocation.shuffleTracking.enabled":"true",
"spark.dynamicAllocation.shuffleTracking.timeout":"120s",
"spark.dynamicAllocation.minExecutors":"3",
"spark.dynamicAllocation.maxExecutors":"10",
"spark.dynamicAllocation.initialExecutors":"3",
"spark.kubernetes.allocation.batch.size":"1",
"spark.dynamicAllocation.executorAllocationRatio":"1",
"spark.dynamicAllocation.schedulerBacklogTimeout": "1s",
"spark.dynamicAllocation.executorIdleTimeout": "120s",
"spark.kubernetes.executor.deleteOnTermination": "true",
"spark.decommission.enabled": "true",
"spark.storage.decommission.enabled": "true",
"spark.storage.decommission.rddBlocks.enabled": "true",
"spark.storage.decommission.shuffleBlocks.enabled": "true",
"spark.kubernetes.driver.podTemplateFile":"$s3DemoBucket/pod_templates/spark_driver_pod_template.yaml",
"spark.kubernetes.executor.podTemplateFile":"$s3DemoBucket/pod_templates/spark_executor_pod_template.yaml"
}
}
],
"monitoringConfiguration": {
"cloudWatchMonitoringConfiguration": {
"logGroupName": "/emr-on-eks/spot-graviton-karpenter-demo",
"logStreamNamePrefix": "wordcount-demo2"
},
"s3MonitoringConfiguration": {
"logUri": "$s3DemoBucket/job_monitoring_logging/"
}
}
}
}
EOF
第三步:提交任务
使用 start-job-run
命令和存储在本地的 start-job-run-request_wordcount_demo2.json
文件路径。
aws emr-containers start-job-run --cli-input-json file://./start-job-run-request_wordcount_demo2.json
第四步:等到 Spark 命名空间下开始有 executor pod 在 running 状态,开始 FIS 故障注入实验模拟 Spot 中断
watch kubectl get pods -n spark
替换 fis_experiment_id 为之前的 FIS 实验 ID。
aws fis start-experiment --experiment-template-id <fis_experiment_id>
第五步:实验开始通过以下命令观察到 Spot 节点被中断
因为 Karpenter v0.23.0 版本内置 spot interruption 处理,所以对于被中断的 Spot 节点进行了cordon 和 drain,其上运行的两个executor pods也被终止。
watch kubectl get pods -n spark
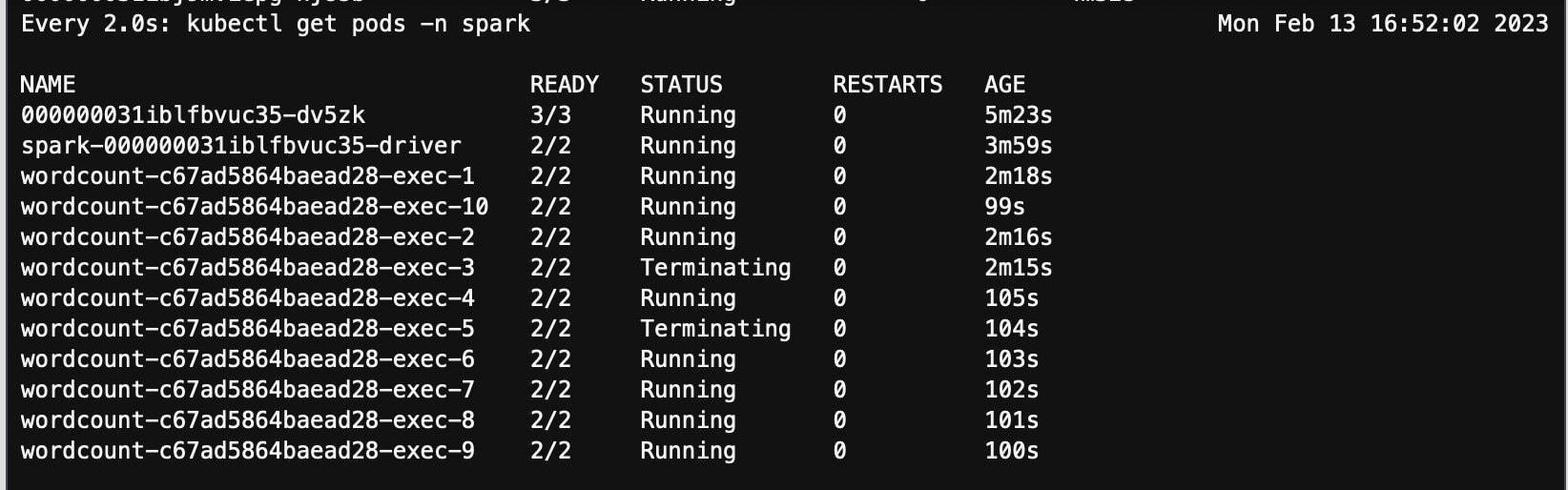
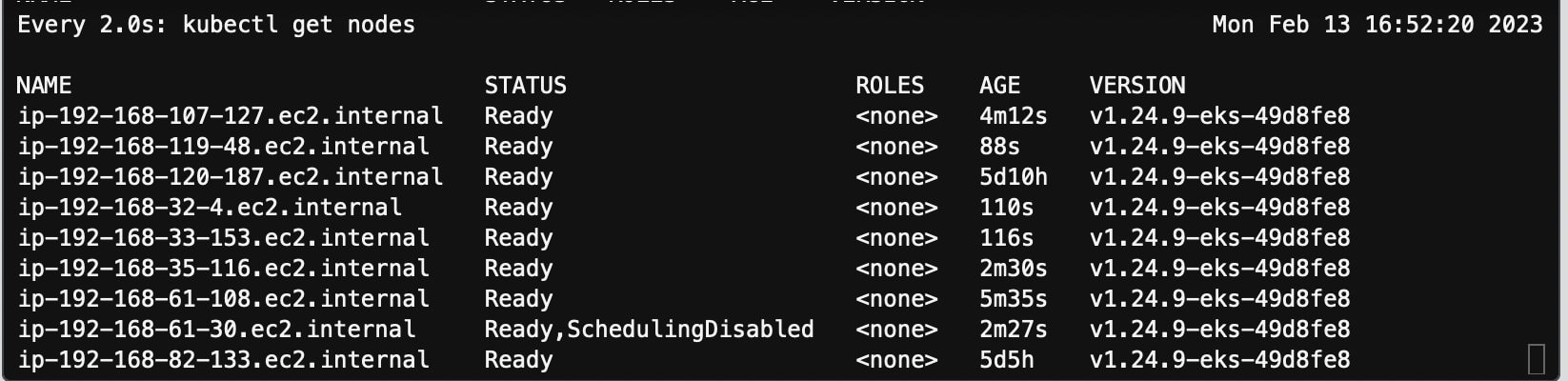
第六步:观察到新的 pod 和节点启动
随后 Spark Driver 启动了两个新的 pod executor-11 和 executor-12 继续完成任务,两个新 pod 处在 pending 状态。
watch kubectl get pods -n spark
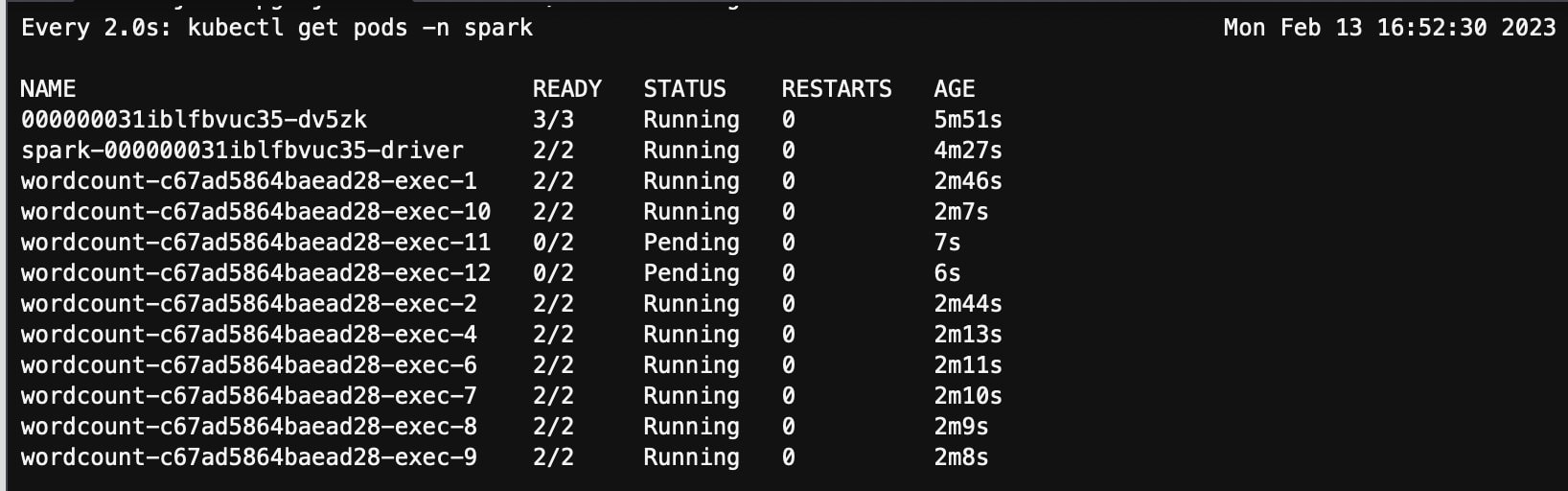
从 karpenter controller 日志看到 karpenter 在观察到整个过程,pending pod 出现之后启动了新的 Spot 实例 192.168.118.170,机型 r6g.large。
kubectl logs -f --tail=100 <karpenter-pod-name> -c controller -n karpenter
2023-02-13T16:51:51.899Z DEBUG controller.interruption removing offering from offerings {"commit": "5a7faa0-dirty", "queue": "spot-graviton-karpenter-demo", "messageKind": "SpotInterruptionKind", "node": "ip-192-168-61-30.ec2.internal", "action": "CordonAndDrain", "unavailable-reason": "SpotInterruptionKind", "instance-type": "c6g.xlarge", "zone": "us-east-1a", "capacity-type": "spot", "unavailable-offerings-ttl": "3m0s"}
2023-02-13T16:51:51.933Z INFO controller.interruption deleted node from interruption message {"commit": "5a7faa0-dirty", "queue": "spot-graviton-karpenter-demo", "messageKind": "SpotInterruptionKind", "node": "ip-192-168-61-30.ec2.internal", "action": "CordonAndDrain"}
2023-02-13T16:51:51.992Z INFO controller.termination cordoned node {"commit": "5a7faa0-dirty", "node": "ip-192-168-61-30.ec2.internal"}
2023-02-13T16:52:24.304Z INFO controller.termination deleted node {"commit": "5a7faa0-dirty", "node": "ip-192-168-61-30.ec2.internal"}
2023-02-13T16:52:29.144Z INFO controller.provisioner found provisionable pod(s) {"commit": "5a7faa0-dirty", "pods": 2}
2023-02-13T16:52:29.144Z INFO controller.provisioner computed new node(s) to fit pod(s) {"commit": "5a7faa0-dirty", "newNodes": 1, "pods": 1}
2023-02-13T16:52:29.144Z INFO controller.provisioner computed 1 unready node(s) will fit 1 pod(s) {"commit": "5a7faa0-dirty"}
2023-02-13T16:52:29.145Z INFO controller.provisioner launching node with 1 pods requesting {"cpu":"1155m","memory":"3187Mi","pods":"5"} from types m6g.large, r6g.xlarge, m6g.xlarge, c7g.xlarge, c6g.xlarge and 1 other(s) {"commit": "5a7faa0-dirty", "provisioner": "mixed"}
2023-02-13T16:52:31.291Z INFO controller.provisioner.cloudprovider launched new instance {"commit": "5a7faa0-dirty", "provisioner": "mixed", "id": "i-070ea910ba58afd09", "hostname": "ip-192-168-118-170.ec2.internal", "instance-type": "r6g.large", "zone": "us-east-1a", "capacity-type": "spot"}
同样从 EMR console 选中任务点击 view logs,从 Spark history server 同样可以看到 Spot 节点中断造成的 executor pod 的替换。
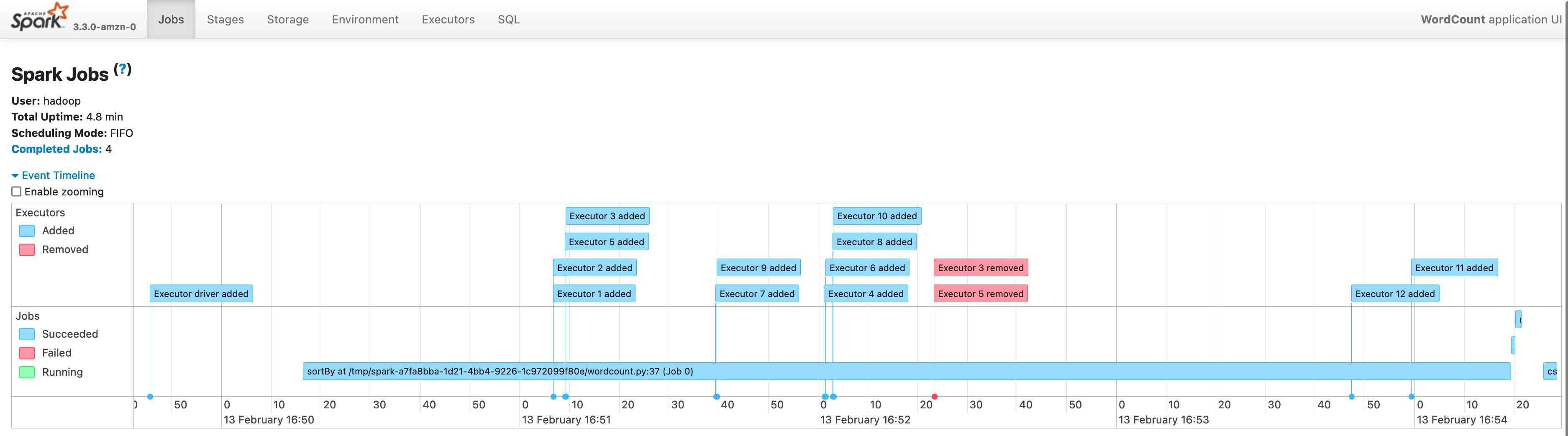
比较 S3 桶中实验一和二的结果,可以发现结果一致。
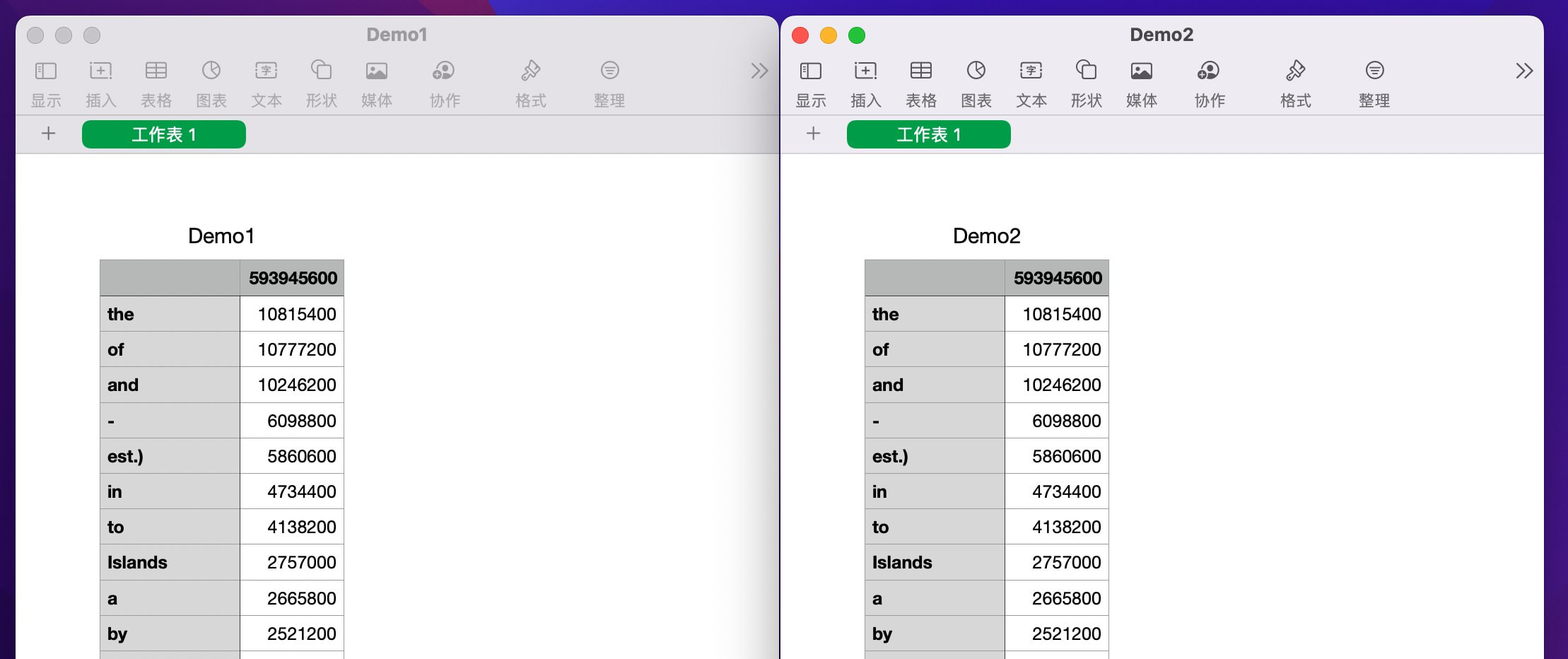
小结:
至此,我们可以证明 Spot 中断发生后,karpenter 内置的 Spot 中断的处理逻辑可以启动新实例运行新启动的 pending executor pod。启动新实例的过程仍是按照 karpenter mixed provisioner 设置优先启动 arm 架构 Spot 实例。并且通过对 Spark 应用功能的调优,保证了中断重算的效率和结果一致性。
5. 总结
在本次系列blog(三) 中,我们通过分析 Spot 容量和中断的特性,从 AWS Well-Architected Framework 的六大支柱之一“可靠性支柱”出发,在方案中应用了 Spot 容量和中断最佳实践;同时通过对 Spark 功能的调优(启用 Spark Node Decommission 降低重算率)、Kubernetes 节点弹性伸缩开源组件 Karpenter 内置的对 Spot 处理机制,降低了 Spot 中断对业务的影响;并且通过 AWS Fault Injection Simulator 全托管服务模拟 Spot 中断故障,验证该方案下应用的可靠性。
针对这一具体场景,我们也总结了一些 Spot 使用的最佳实践:
(1)通过 Spot 选型工具(比如 Spot Instance Advisor,Spot Placement Score 等)选择中断率低的实例
(2)使用 Price capacity optimized 或 Capacity Optimized 的 Spot 分配策略优先选择容量多的实例
(3) 启用 Karpenter 内置 interruption handler 优雅处理 Spot 中断
(4)利用 Karpenter 同时管理 Spot 和 On-demand,实现 Spot 容量不足时自动启动 On-demand,降低运维成本
(4) 启用 Spark Node Decommissioning 功能降低任务重算率
(6)多通过 FIS 服务进行 Spot 中断演练,测试业务稳定性
最后建议您先采用真实业务数据测试,在各项参数调优后性能指标比如任务处理时长等达到您的预期后,再在生产环节中实际应用参考本方案。
本篇作者