Amazon Managed Workflows for Apache Airflow (MWAA)
Secure and highly available managed workflow orchestration for Apache Airflow
Why Amazon MWAA?
Amazon MWAA is accessible in the next generation of Amazon SageMaker
With Amazon MWAA in the next generation of Amazon SageMaker, you can deploy and scale Apache Airflow seamlessly without operational burdens. With automated scaling and built-in fault tolerance, MWAA in Amazon SageMaker ensures your workflows execute reliably—allowing you to focus on innovation, not infrastructure. Learn more.
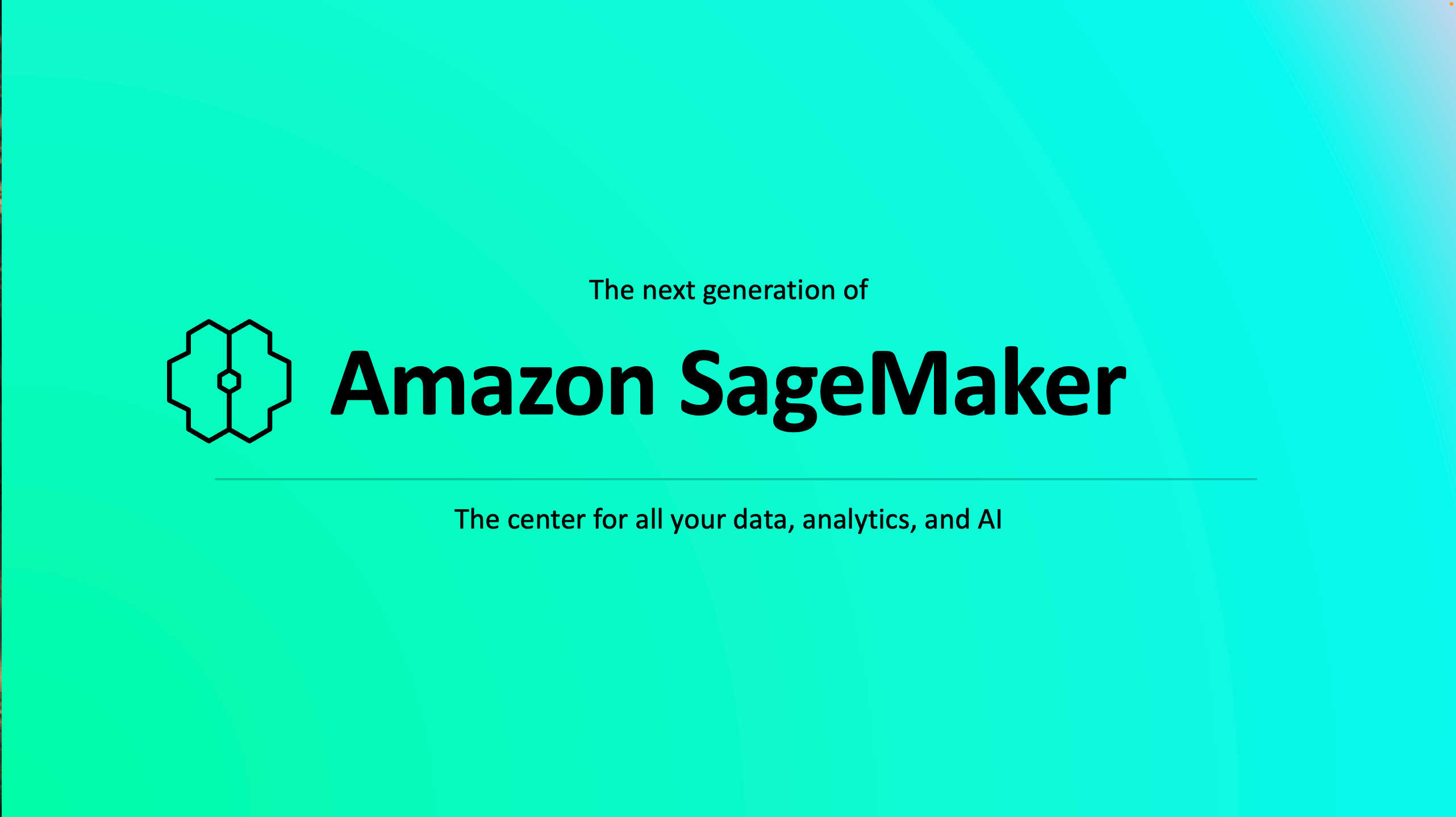