Amazon EMR
Easily run and scale Apache Spark, Hive, Presto, and other big data workloads
Run big data applications and petabyte-scale data analytics faster, and at less than half the cost of on-premises solutions.
Build applications using the latest open-source frameworks, with options to run on customized Amazon EC2 clusters, Amazon EKS, AWS Outposts, or Amazon EMR Serverless.
Get up to 2X faster time-to-insights with performance-optimized and open-source API-compatible versions of Spark, Hive, and Presto.
Easily develop, visualize, and debug your applications using EMR Notebooks and familiar open-source tools in EMR Studio.
How it works
Amazon EMR is the industry-leading cloud big data solution for petabyte-scale data processing, interactive analytics, and machine learning using open-source frameworks such as Apache Spark, Apache Hive, and Presto.
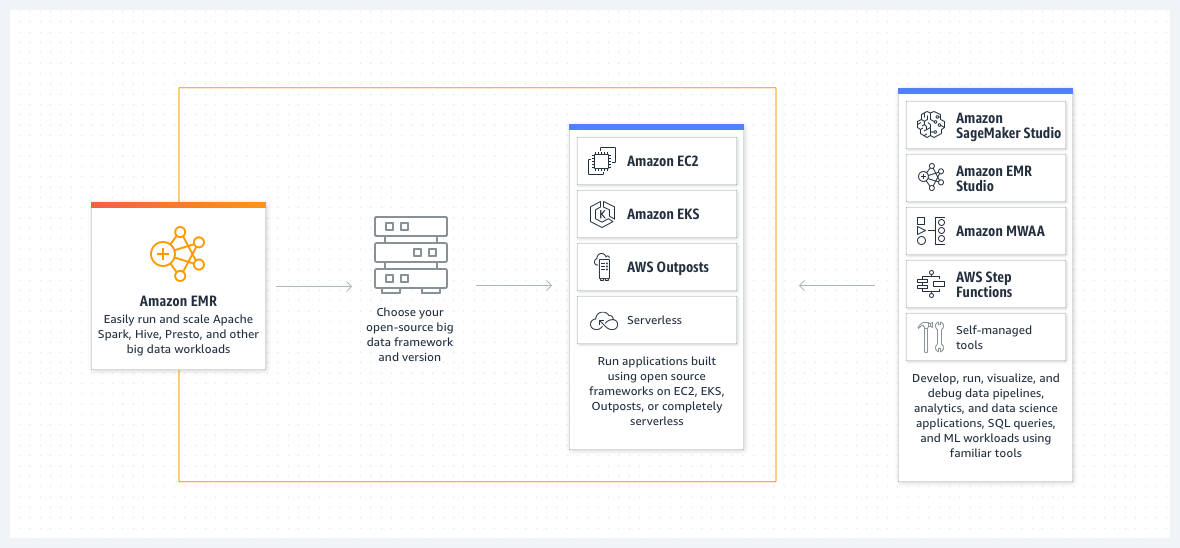
Use cases
Perform big data analytics
Run large-scale data processing and what-if analysis using statistical algorithms and predictive models to uncover hidden patterns, correlations, market trends, and customer preferences.
Build scalable data pipelines
Extract data from a variety of sources, process it at scale, and make it available for applications and users.
Process real-time data streams
Analyze events from streaming data sources in real-time to create long-running, highly available, and fault-tolerant streaming data pipelines.
Accelerate data science and ML adoption
Analyze data using open-source ML frameworks such as Apache Spark MLlib, TensorFlow, and Apache MXNet. Connect to Amazon SageMaker Studio for large-scale model training, analysis, and reporting.
How to get started
Find out how Amazon EMR works
Learn more about provisioning clusters, scaling resources, configuring high availability, and more.
Explore Amazon EMR pricing
Pay per second with options to run EMR clusters on Amazon EC2, Amazon EKS, AWS Outposts, or Amazon EMR Serverless.
Get started with Amazon EMR
Learn about real-time stream processing, large-scale machine learning, and more using EMR.