AWS Architecture Blog
Field Notes: Deploying Autonomous Driving and ADAS Workloads at Scale with Amazon Managed Workflows for Apache Airflow
Cloud Architects developing autonomous driving and ADAS workflows are challenged by loosely distributed process steps along the tool chain in hybrid environments. This is accelerated by the need to create a holistic view of all running pipelines and jobs. Common challenges include:
- finding and getting access to the data sources specific to your use case, understanding the data, moving and storing it,
- cleaning and transforming it and,
- preparing it for downstream consumption.
Verifying that your data pipeline works correctly and ensuring you provide a certain data quality while your application is being developed adds to the complexity. Furthermore, the fact that the data itself constantly changes poses more challenges. In this blog post, we show you the steps to follow from a workflow run in an Amazon Managed Workflows for Apache Airflow (MWAA) environment. All required infrastructure is created leveraging the AWS Cloud Development Kit (CDK).
We illustrate how image data collected during field operational tests (FOTs) of vehicles can be processed by:
- detecting objects within the video stream
- adding the bounding boxes of all detected objects to the image data
- providing visual and metadata information for data cataloguing as well as potential applications on the bounding boxes.
We define a workflow that processes ROS bag files on Amazon S3, and extracts individual PNGs from a video stream using AWS Fargate on Amazon Elastic Container Service (Amazon ECS). Then we show how to use Amazon Rekognition to detect objects within the exported images and draw bounding boxes for the detected objects within the images. Finally, we demonstrate how users’ interface with MWAA to develop and publish their workflows. We also show methods to keep track of the performance and costs of your workflows.
Overview of the solution
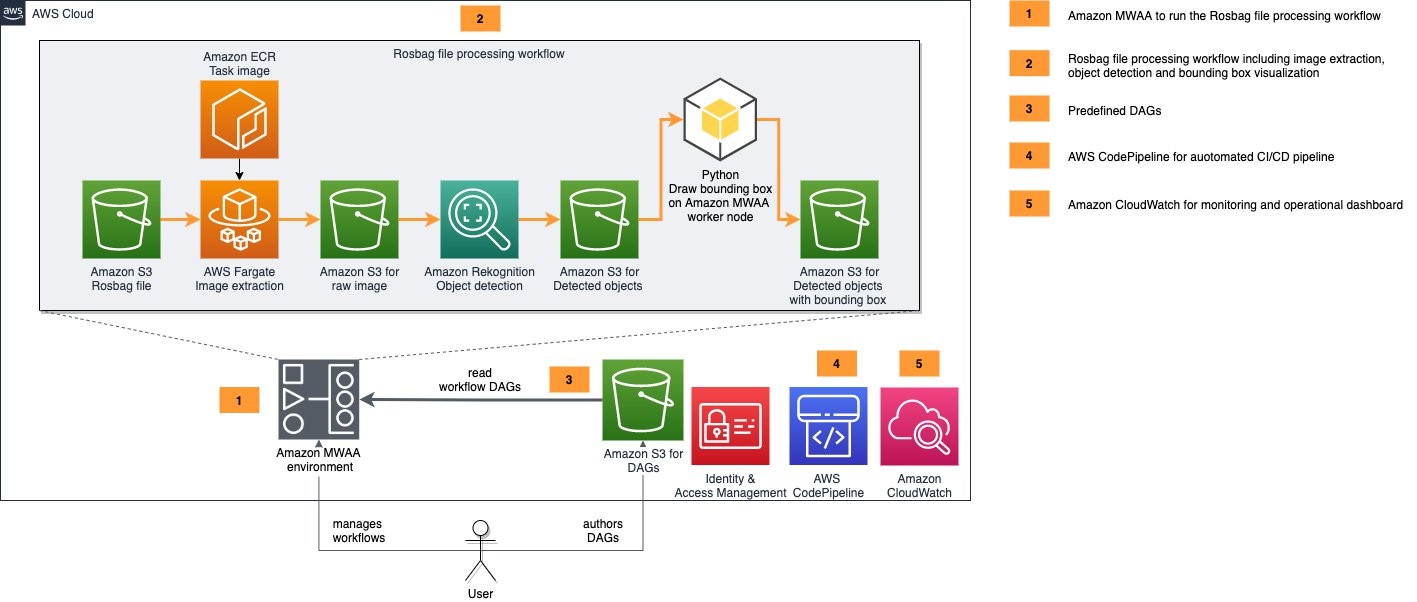
Figure 1 – Architecture for deploying Autonomous Driving & ADAS workloads at scale
The preceding diagram shows our target solution architecture. It includes five components:
- Amazon MWAA environment: we use Amazon MWAA to orchestrate our image processing workflow’s tasks. A workflow, or DAG (directed acyclic graph), is a set of task definitions and dependencies written in Python. We create a MWAA environment using CDK and define our image processing pipeline as a DAG which MWAA can orchestrate.
- ROS bag file processing workflow: this is a workflow run on Managed Workflows for Apache Airflow. This detects and highlights objects within the camera stream of a ROS bag file. The workflow is as follows:
- Monitors the location in Amazon S3 for the new ROS bag files.
- If a new file gets uploaded, we extract video data from the ROS bag file’s topic that holds the video stream, extract individual PNGs from the video stream and store them on S3.
- We use AWS Fargate on Amazon ECS to run a containerized image extraction workload.
- Once the extracted images are stored on S3, they are passed to Amazon Rekognition for object detection. We then provide an image or video to the Amazon Rekognition API, and the service can identify objects, people, text, scenes, and activities. Amazon Rekognition Image can return the bounding box for many object labels.
- We use the information to highlight the detected objects within the image by drawing the bounding box.
- The bounding boxes of the objects Amazon Rekognition detects within the extracted PNGs are rendered directly into the image using Pillow, a fork of the Python Imaging Library (PIL).
- The resulting images are then stored in Amazon S3 and our workflow is complete.
- Predefined DAGs: Our solution comes with a pre-defined ROS bag image processing DAG that will be copied into the Amazon MWAA environment with CDK.
- AWS CodePipeline for automated CI/CD pipeline: CodePipeline automates the build, test, and deploy phases of your release process every time there is a code change, based on the release model you define
- Amazon CloudWatch for monitoring and operational dashboard: With Amazon CloudWatch we can monitor important metrics of the ROS bag image processing workflow, for example. to verify the compute load, number of running workflows or tasks or to monitor and alert on processing errors.
Prerequisites
- Prepare an AWS account to be used for the installation
- AWS CLI installed and configured to point to your AWS account
- Use a named profile to access your AWS account
- Install the AWS Cloud Development Kit
- Our example uses Python version 3.7 or newer
- Docker installed and running
- Download the sample ROS bag file
Deployment
To deploy and run the solution, we follow these steps:
- Configure and create an MWAA environment in AWS using CDK
- Copy the ROS bag file to S3
- Manage the ROS bag processing DAG from the Airflow UI
- Inspect the results
- Monitor the operations dashboard
Each component illustrated in the solution architecture diagram, as well as all required IAM roles and configuration parameters are created from an AWS CDK application. This is included in these example sources.
1. Configure and create an MWAA environment in AWS using CDK
- Download the deployment templates
- Extract the zip file to a directory
- Open a shell and go to the extracted directory
- Start the deployment by typing the following code:
- Replace
<named-profile>
with the named profile which you configured for your AWS CLI to point to your target AWS account. - Replace
<region>
with the Region that is associated with your named profile, for example,us-east-1
. - As an example: if your named profile for the AWS CLI is called
rosbag-processing
and your associated Region is us-east-1 the resulting command would be:
After confirming the deployment, the CDK will take several minutes to synthesize the CloudFormation templates, build the Docker image and deploy the solution to the target AWS account.
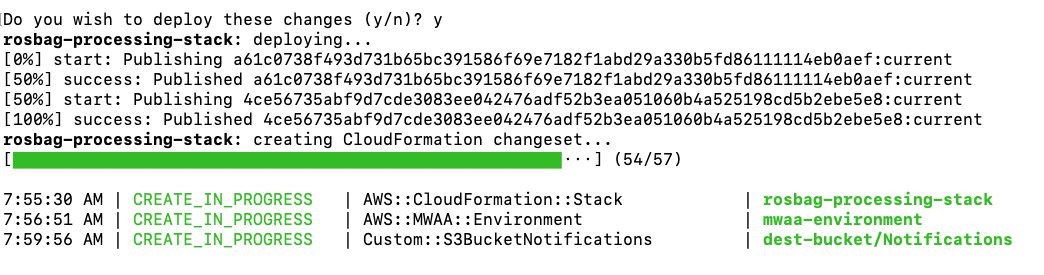
Figure 2 – Screenshot of Rosbag processing stack
Inspect the CloudFormation stacks being created in the AWS console. Sign into your AWS console and select the service CloudFormation in the AWS Region that you chose for your named profile (for exmaple, us-east-After the installation script has run, the CloudFormation stack overview in the console should show the following stacks:
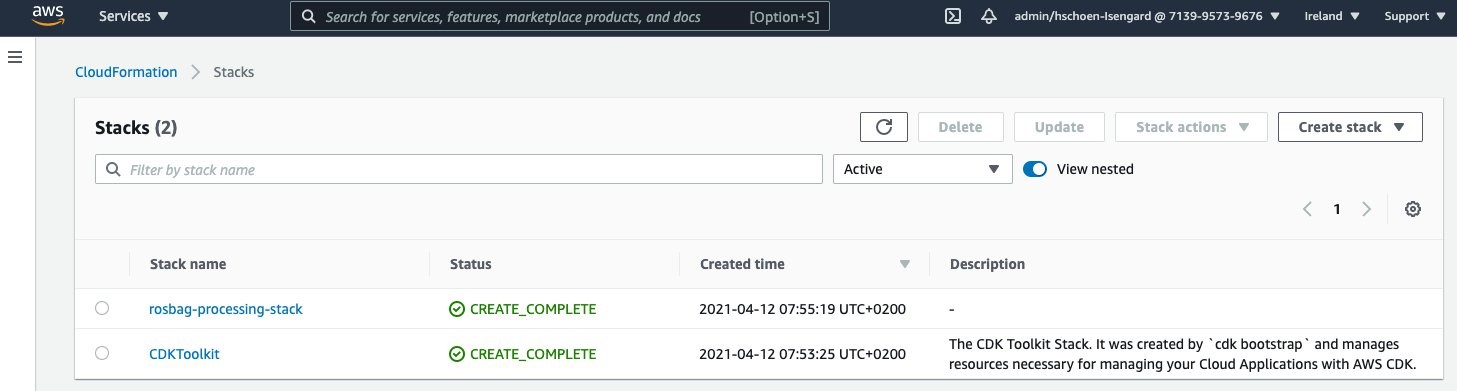
Figure 3 – Screenshot of CloudFormation stack overview
2. Copy the ROS bag file to S3
- You can download the ROS bag file here. After downloading you need to copy the file to the input bucket that was created during the CDK deployment in the previous step.
- Open the AWS management console and navigate to the service S3. You should find a list of your buckets including the buckets for input data, output data and DAG definitions that were created during the CDK deployment.
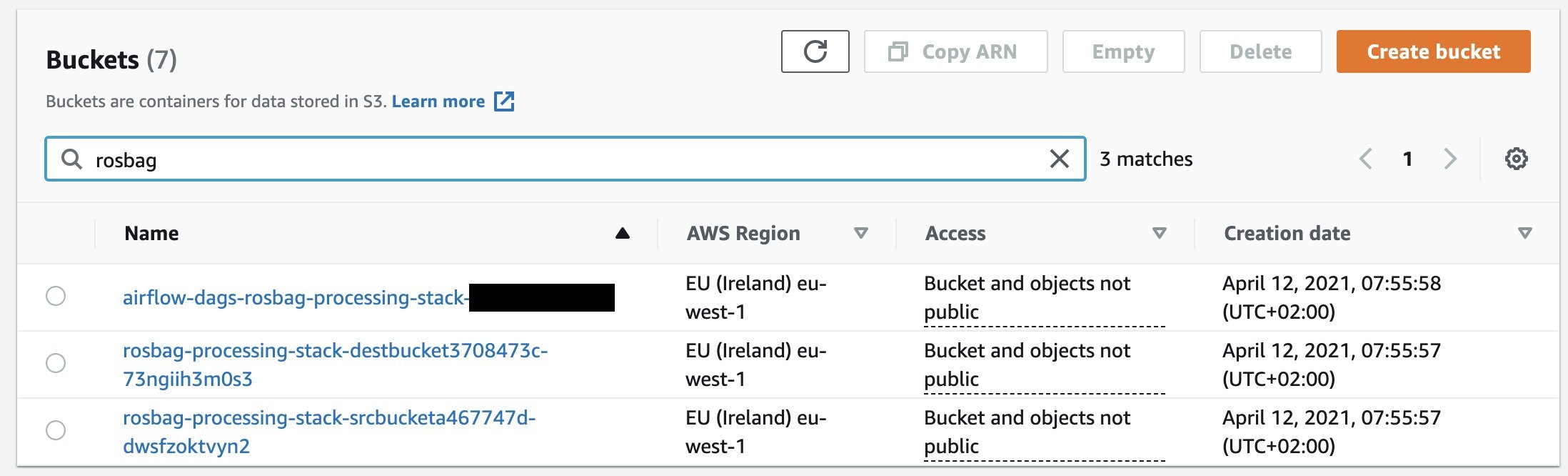
Figure 4 – Screenshot showing S3 buckets created
- Select the bucket with prefix
rosbag-processing-stack-srcbucket
and copy the ROS bag file into the bucket. - Now that the ROS bag file is in S3, we need to enable the image processing workflow in MWAA.
3. Manage the ROS bag processing DAG from the Airflow UI
- In order to access the Airflow web server, open the AWS management console and navigate to the MWAA service.
- From the overview, select the MWAA environment that was created and select Open Airflow UI as shown in the following screenshot:
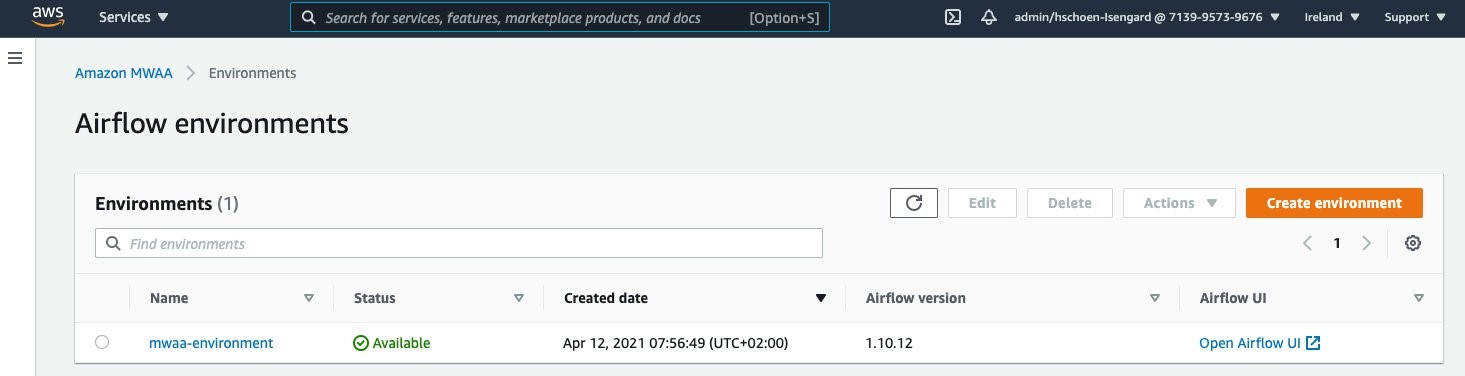
Figure 5 – Screenshot showing Airflow Environments
The Airflow UI and the ROS bag image processing DAG should appear as in the following screenshot:
- We can now enable the DAG by flipping the switch (1) from “Off” to “On” position. Amazon MWAA will now schedule the launch for the workflow.
- By selecting the DAG’s name (
rosbag_processing
) and selecting the Graph View, you can review the workflow’s individual tasks:

Figure 6 – The Airflow UI and the ROS bag image processing DAG
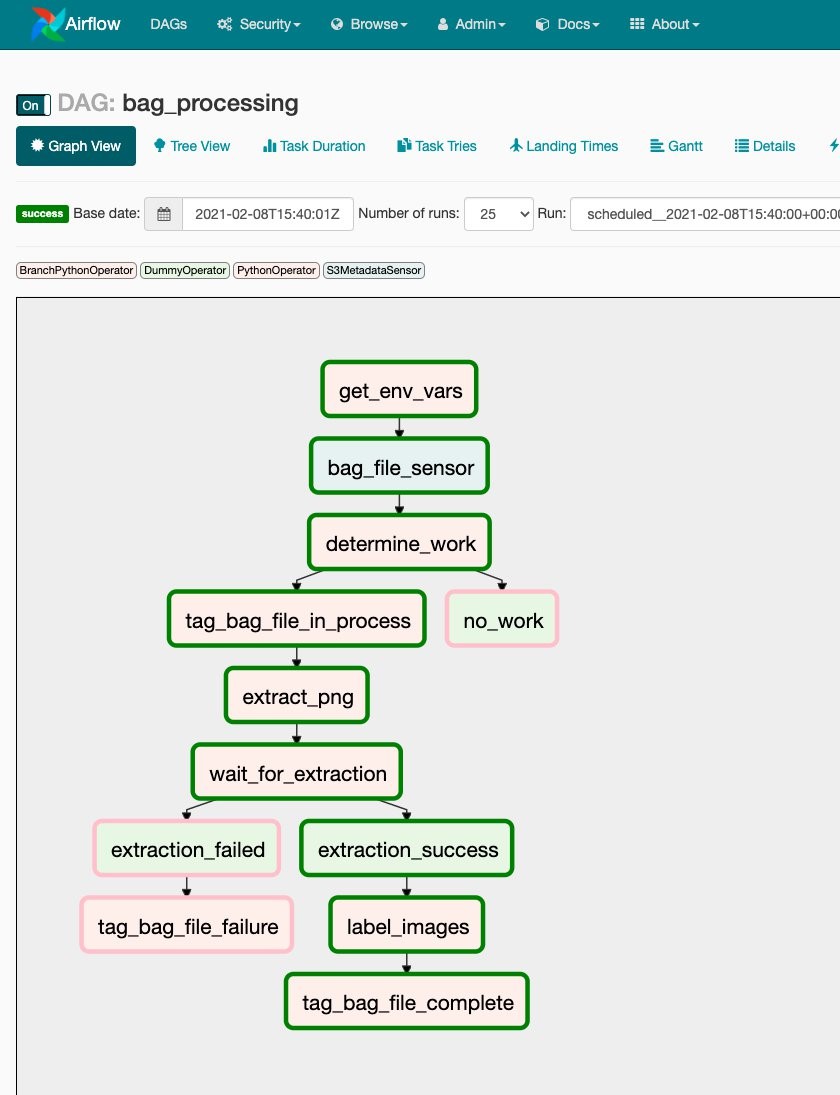
Figure 7 – Dag Workflow
After enabling the image processing DAG by flipping the switch to “on” in the previous step, Airflow will detect the newly uploaded ROS bag file in S3 and start processing it. You can monitor the progress from the DAG’s tree view (1), as shown in the following screenshot:
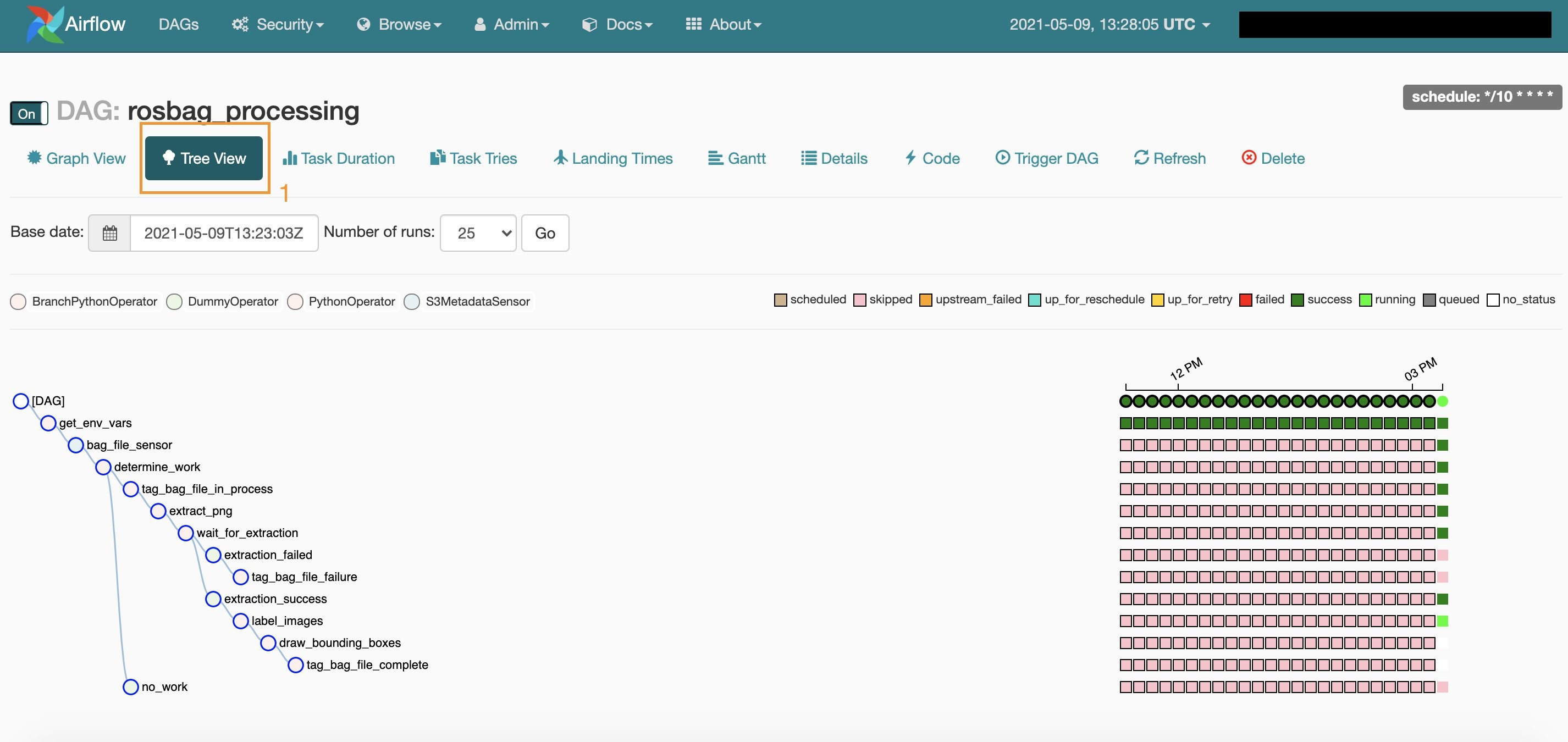
Figure 8 – Monitor progress in the DAG’s tree view
Re-running the image processing pipeline
Our solution uses S3 metadata to keep track of the processing status of each ROS bag file in the input bucket in S3. If you want to re-process a ROS bag file:
- open the AWS management console,
- navigate to S3, click on the ROS bag file you want to re-process,
- remove the file’s metadata tag
processing.status
from theproperties
tab:

Figure 9 – Metadata tag
With the metadata tag processing.status
removed, Amazon MWAA picks up the file the next time the image processing workflow is launched. You can manually start the workflow by choosing Trigger DAG (1) from the Airflow web server UI.
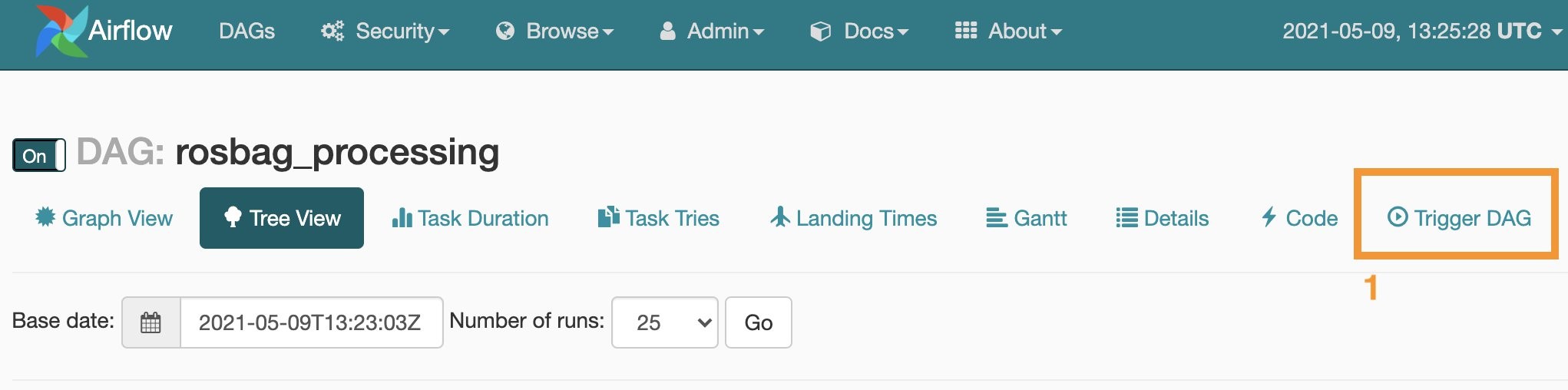
Figure 10 – Manually start the workflow by choosing Trigger DAG
4. Inspect the results
In the AWS management console navigate to S3, and select the output bucket with prefix rosbag-processing-stack-destbucket
. The bucket holds both the extracted images from the ROS bag’s topic containing the video data (1) and the images with highlighted bounding boxes for the objects detected by Amazon Rekognition (2).
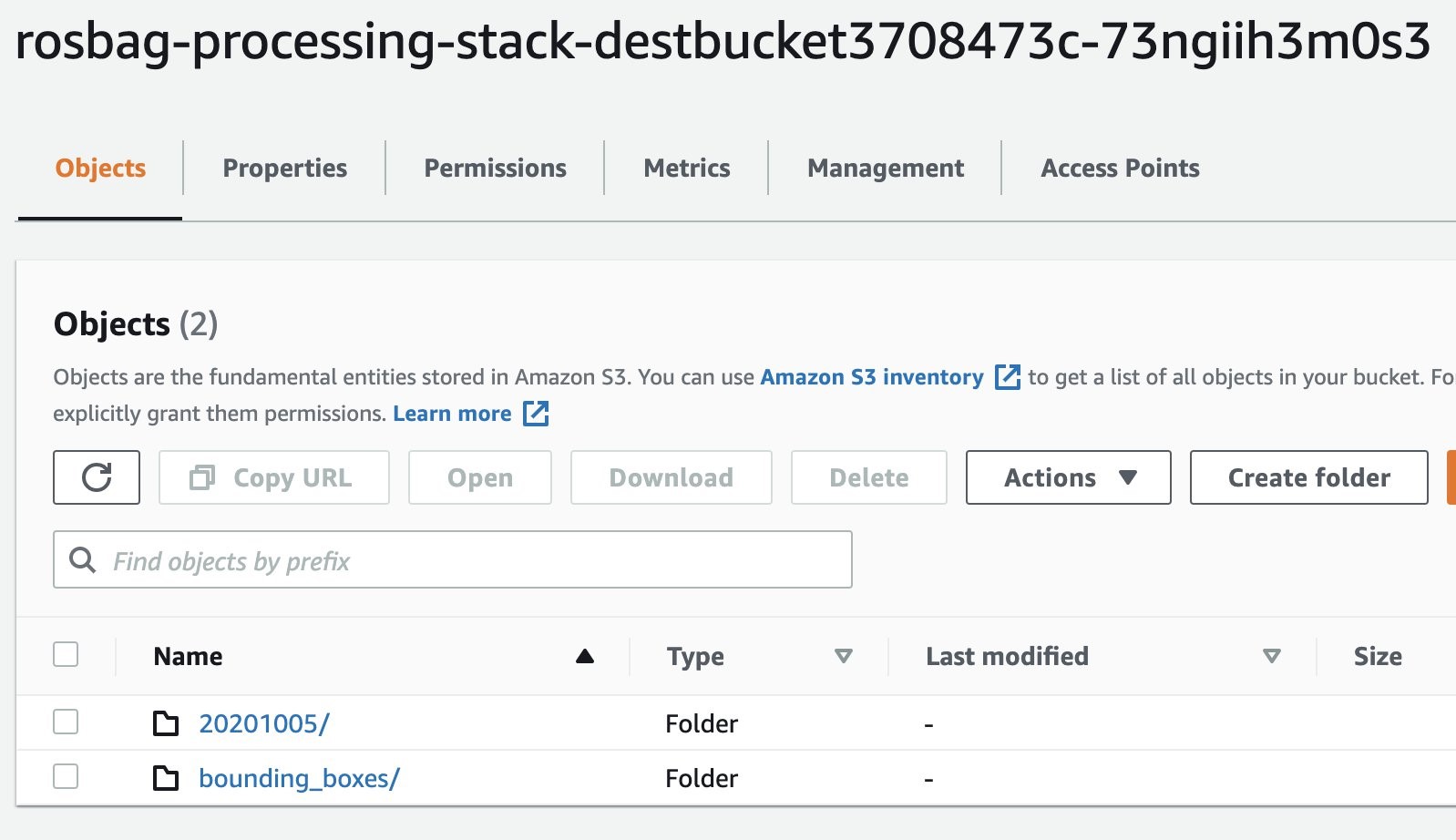
Figure 11 – Output S3 buckets
- Navigate to the folder
20201005
and select an image, - Navigate to the folder
bounding_boxes
- Select the corresponding image. You should receive an output similar to the following two examples:
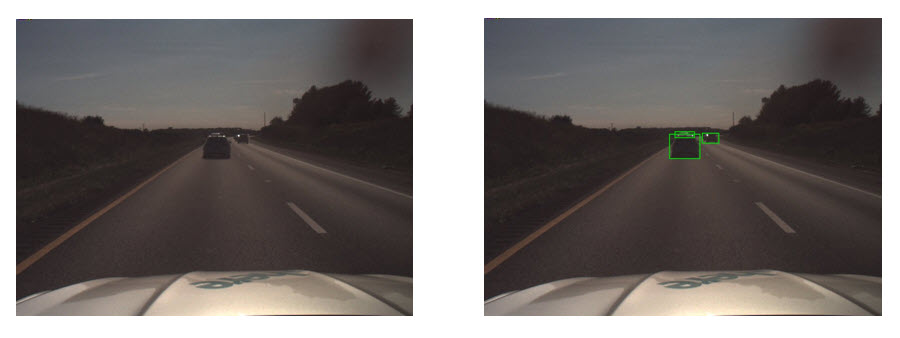
Figure 12 – Left: Image extracted from ROS bag camera feed topic. Right: Same image with highlighted objects that Amazon Rekognition detected.
Amazon CloudWatch for monitoring and creating operational dashboard
Amazon MWAA comes with CloudWatch metrics to monitor your MWAA environment. You can create dashboards for quick and intuitive operational insights. To do so:
- Open the AWS management console and navigate to the service CloudWatch.
- Select the menu “Dashboards” from the navigation pane and click on “Create dashboard”.
- After providing a name for your dashboard and selecting a widget type, you can choose from a variety of metrics that MWAA provides. This is shown in the following picture.
MWAA comes with a range of pre-defined metrics that allow you to monitor failed tasks, the health of your managed Airflow environment and helps you right-size your environment.
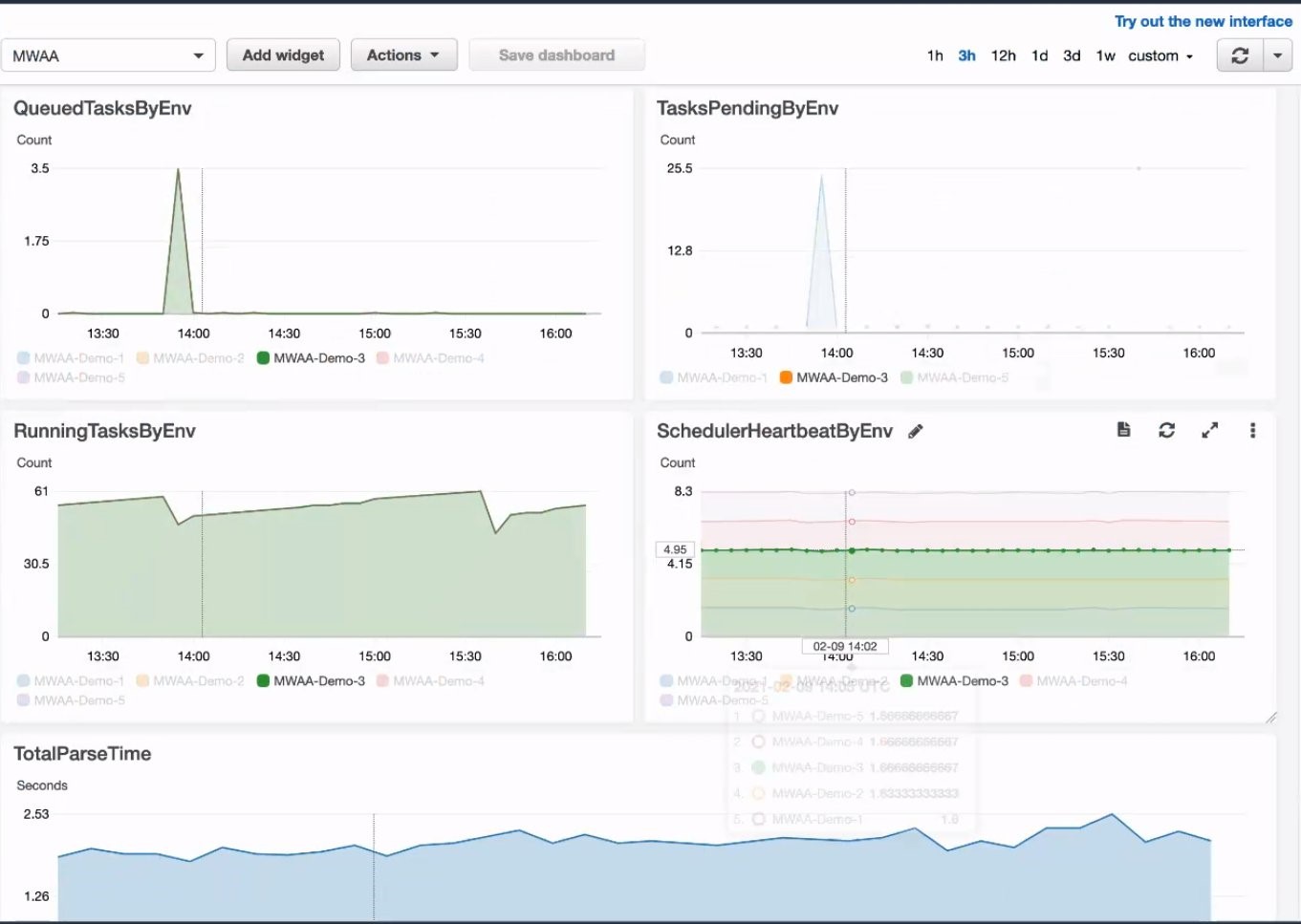
Figure 13 – CloudWatch metrics in a dashboard help to monitor your MWAA environment.
Clean Up
In order to delete the deployed solution from your AWS account, you must:
1. Delete all data in your S3 buckets
- In the management console navigate to the service S3. You should see three buckets containing rosbag-processing-stack that were created during the deployment.
- Select each bucket and delete its contents. Note: Do not delete the bucket itself.
2. Delete the solution’s CloudFormation stack
You can delete the deployed solution from your AWS account either from the management console or the command line interface.
Using the management console:
- Open the management console and navigate to the CloudFormation service. Make sure to select the same region you deployed your solution to (for exmaple,. us-east-1).
- Select
stacks
from the side menu. The solution’s CloudFormation stack is shown in the following screenshot:
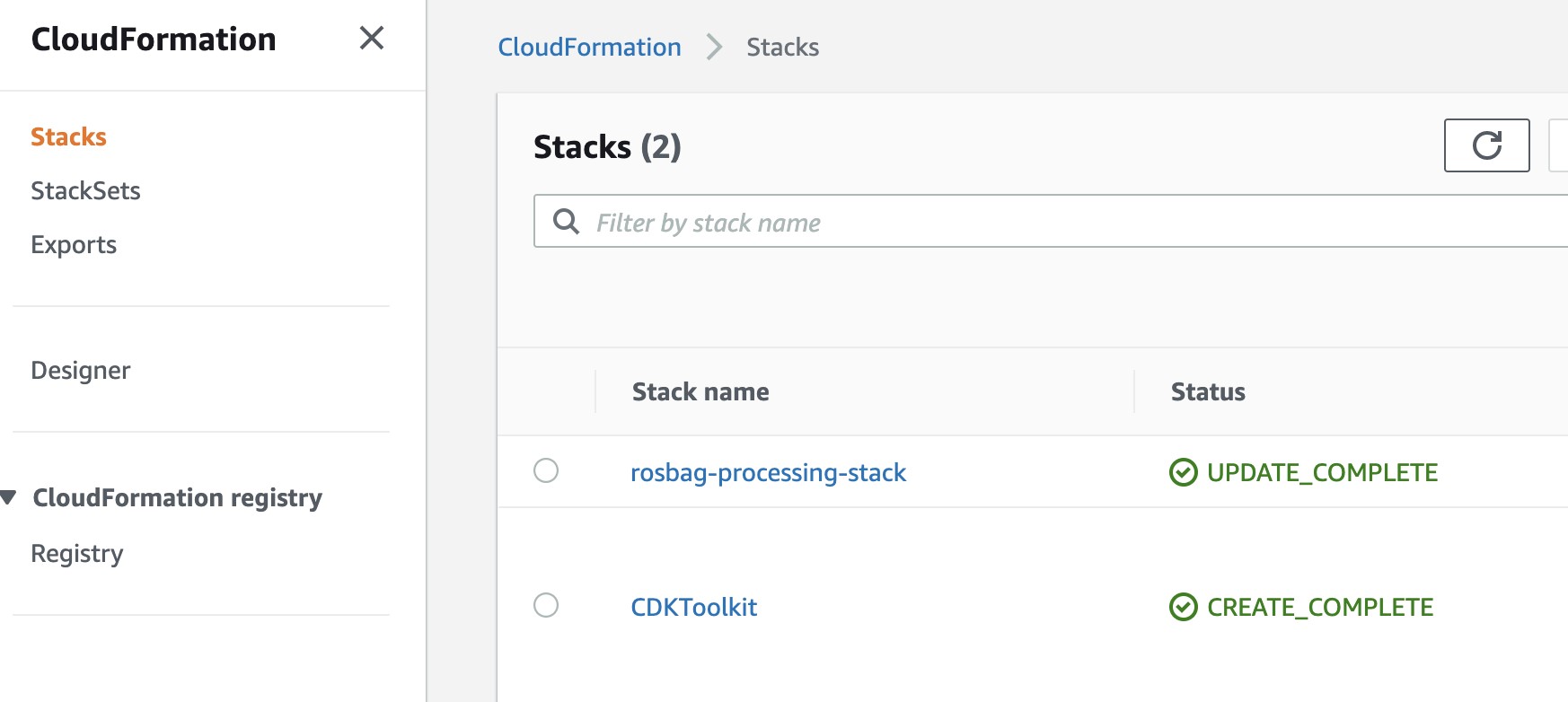
Figure 14 – Cleaning up the CloudFormation Stack
Select the rosbag-processing-stack
and select Delete. CloudFormation will now destroy all resources related to the ROS bag image processing pipeline.
Using the Command Line Interface (CLI):
Open a shell and navigate to the directory from which you started the deployment, then type the following:
Replace <named-profile>
with the named profile which you configured for your AWS CLI to point to your target AWS account.
As an example: if your named profile for the AWS CLI is called rosbag-processing
and your associated Region is us-east-1
the resulting command would be:
After confirming that you want to destroy the CloudFormation stack, the solution’s resources will be deleted from your AWS account.
Conclusion
This blog post illustrated how to deploy and leverage workflows for running Autonomous Driving & ADAS workloads at scale. This solution was designed using fully managed AWS services. The solution was deployed on AWS using AWS CDK templates. Using MWAA we were able to set up a centralized, transparent, intuitive workflow orchestration across multiple systems. Even though the pipeline involved several steps in multiple AWS services, MWAA helped us understand and visualize the workflow, its individual tasks and dependencies.
Usually, distributed systems are hard to debug in case of errors or unexpected outcomes. However, building the ROS bag processing pipelines on MWAA allowed us to inspect the log files involved centrally from the Airflow UI, for debugging or auditing. Furthermore, with MWAA the ROS bag processing workflow is part of the code base and can be tested, refactored, versioned just like any other software artifact. By providing the CDK templates, we were able to architect a fully automated solution which you can adapt to your use case.
We hope you found this interesting and helpful and invite your comments on the solution!