When building a new application, it is important to plan out your data model before diving into implementation. This approach ensures that you have a solid foundation on which you can build your application. In this module, you learn about the main entities in your application, plan the data model, and prepare your database.
A common way to plan your data model is to create an entity-relationship diagram (ERD). An ERD shows the different entities in your application and their properties. It also shows how the entities relate to each other.
In your simple application, you have two entities: Users and Items. A User represents a human user in your game and has properties like Username, Height, and Weight.
An Item represents an ownable item in the game. This could be a weapon, armor, clothing, potions, or various other types of objects. An Item has Type and Weight properties, as well as type-specific properties such as Attack points for a weapon or Defense points for armor.
You can represent your two entities in an ERD as shown below.
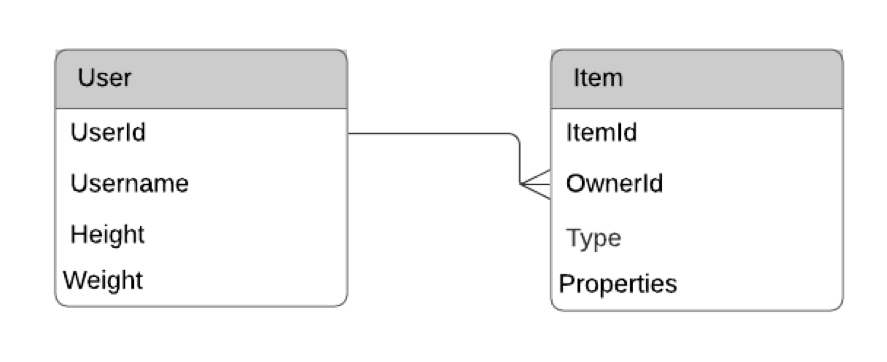
You can see you have both the User entity and the Item entity represented with the various properties. Additionally, the two entities are connected by a line. This line represents a relationship between the two entities. Each Item is owned by one and only one User, and a User can own many Items. Therefore, there is a one-to-many relationship between Users and Items.
In the following steps, you translate your ERD into database code. First, you create the tables and relationships to match your ERD. Then, you load some sample data into your database. Finally, you run some queries on your database to handle some of your use cases.
Time to Complete Module: 20 Minutes