Customer Stories / Life Sciences / USA
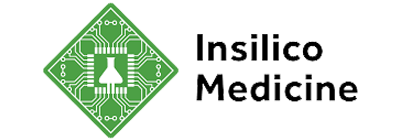
Insilico Medicine Accelerates Drug Discovery Using Amazon SageMaker
Learn how Insilico Medicine in life sciences increased the velocity of its ML model training pipeline by over 16 times using Amazon SageMaker.
83% reduction
in time to deploy model updates
>16x accelerated
model iteration and deployment time
Overview
Insilico Medicine (Insilico) is accelerating drug discovery by using its complete machine learning (ML) platform, Pharma.AI. Since 2014, the company has been using generative artificial intelligence (AI) for drug discovery, which was years before the technology gained widespread adoption. Insilico uses ML-based techniques to identify novel therapeutic targets, design new molecules, and select ideal candidates for further testing. Insilico is one of the first AI-driven drug discovery companies to nominate preclinical candidates in less than 18 months and get to human clinical trials in 30 months.
To accelerate its drug discovery process, Insilico required flexible access to computing resources to rapidly train new ML models, so it turned to Amazon Web Services (AWS). Insilico migrated its model training to Amazon SageMaker—a solution to build, train, and deploy ML models with fully managed infrastructure, tools, and workflows. Using Amazon SageMaker, the company reduced the time required to implement new models from 50 days to 3 days, significantly accelerating the discovery of novel drug candidates and enhancing the operational efficiency of its rapid prototyping team.
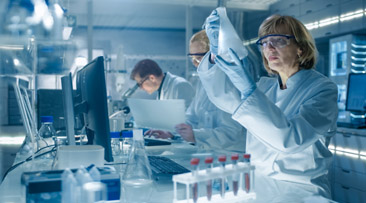
Opportunity | Accelerating Drug Discovery with Flexible Model Training on Amazon SageMaker for Insilico
Founded in 2014, Insilico harnesses AI and deep learning techniques to identify promising therapeutic targets and design therapeutics with optimized properties. In traditional drug discovery, researchers must perform numerous iterations of testing and synthesizing molecules to achieve successful results. Insilico streamlines the initial stages by employing ML to design strong candidate molecules for further evaluation in wet labs. “Our technology solutions can consider far more parameters than a human could when designing a molecule manually,” says Daniil Polykovskiy, senior director of technology at Insilico. “For each step, we have automated processes or ML models that assess the feasibility of a candidate.” Currently, the company has thirty programs in development, including five in phase 1 clinical trials and one in phase 2.
Behind the scenes, Insilico’s rapid prototyping team develops and trains a diverse range of new models to continuously enhance its ML-based solutions. This team consistently explores new frontiers and collaborates with other Insilico teams to provide models that are tailored to specific research needs. To facilitate this process, the team requires access to GPU resources, which it previously obtained through a different cloud provider. However, the previous architecture relied on static virtual machines and Kubernetes clusters that necessitated manual scaling, leading to inefficiencies—sometimes hardware was underused, whereas, at other times, teams had to wait for resources to become available.
“We needed a streamlined approach to build models without focusing on hardware or implementation details,” says Polykovskiy. “We also required a centralized environment where teams could access sufficient resources, collaborate, train and evaluate models, and share results seamlessly.” To achieve this, Insilico began migrating to Amazon SageMaker in 2024.

The whole training pipeline is more efficient on Amazon SageMaker. We can rapidly scale our experiments and select the backend and hardware that we need for each model."
Daniil Polykovskiy
Senior Director of Technology, Insilico Medicine
Solution | Using Amazon SageMaker to Train New Models in 3 Days
On Amazon SageMaker, Insilico reduced the time required to iterate and deploy a new ML model from 50 to 3 days, thus accelerating the process by over 16 times. The company’s previous ML pipeline, which was developed in-house, involved sequentially trying various models, training them with different parameters, and selecting the ideal one for deployment. Using Amazon SageMaker, Insilico can perform these steps in parallel, automatically scale up resources for model testing, and access cutting-edge GPUs and CPUs as needed. “The whole training pipeline is more efficient on Amazon SageMaker,” says Polykovskiy. “We can rapidly scale our experiments and select the backend and hardware that we need for each model.” The rapid prototyping team deploys updated models every 2 weeks rather than every few months, a roughly 83 percent time reduction. By accelerating the model-release cycle, Insilico can advance scientific discoveries at a rapid pace.
Insilico has also reduced the time and effort that are required of its DevOps team to maintain and scale infrastructure. Previously, requesting and deploying new resources through the DevOps team took around 1 week, but using Amazon SageMaker, teams can independently deploy and scale resources as needed. “The teams know their resource budget, so they know what they can train and when, and they don’t need to wait for resources to be allocated by another team,” says Polykovskiy.
The rapid prototyping team at Insilico uses the scalable resources in Amazon SageMaker to train new foundation models and test models alongside other Insilico teams. “Using Amazon SageMaker, we have very flexible computing resources and can collaborate with multiple teams to run tests in parallel,” says Polykovskiy. In one example, the team received a hypothesis to test and delivered results—a new model that was trained on a specific dataset—within 1 day. Insilico has migrated half of its technology teams to Amazon SageMaker as of July 2024 and continues to migrate the rest. “The teams see a lot of value in Amazon SageMaker,” says Polykovskiy. “Everyone feels the need to move forward with more flexible access to compute.”
Outcome | Extending Healthy Longevity Using ML on AWS
Using Amazon SageMaker, Insilico has improved its model training capabilities, enhancing its solutions for accelerating drug discovery. Insilico now has two systems that benefit from the advanced models that were trained on Amazon SageMaker: PandaOmics, a solution that empowers researchers to identify potential therapeutic targets, and Chemistry42, a comprehensive small-molecule drug discovery platform.
Using AI and ML on AWS, Insilico will continue its groundbreaking work in discovering new targets and developing innovative molecules in pursuit of life-changing drugs to combat diseases. “Our mission is to extend healthy, productive longevity for everyone,” says Petrina Kamya, global head of AI platforms for Insilico. “That is what we set our minds to every day when we come into work.”
About Insilico Medicine
Insilico Medicine develops technologies to help accelerate drug discovery and development, in addition to developing therapeutics. The company's mission is to extend healthy, productive longevity for everyone.
AWS Services Used
Amazon SageMaker
Amazon SageMaker is a fully managed service that brings together a broad set of tools to enable high-performance, low-cost machine learning (ML) for any use case.
More Life Sciences Customer Stories
Total results: 216
no items found
-
United States
Greenway Health Fuels EHR Innovation Using AWS HealthLake
Greenway Health uses AWS HealthLake to offer a fully managed certified FHIR electronic health record solution for providers, helping them serve patients more efficiently while creating a foundation for innovation. -
United States
Accelerating the Design of Candidate Drugs Using Amazon SageMaker with Nimbus Therapeutics
Learn how computational chemists at Nimbus Therapeutics built an agile and effective ML pipeline using Amazon SageMaker. -
India
Healthify Innovates Using AWS Generative AI Solutions and Achieves Operational Excellence with AWS Enterprise On-Ramp
This case study demonstrates how Healthify, a social determinants of health technology company, leverages AWS to provide a reliable and scalable platform for its customer support services. -
Switzerland
Modernizing a Legacy Application on AWS Serverless with Alcon
Alcon has increased the efficiency of its sales representatives and enhanced the customer experience by modernizing Custom Pak Designer using a serverless approach on AWS.
Get Started
Organizations of all sizes across all industries are transforming their businesses and delivering on their missions every day using AWS. Contact our experts and start your own AWS journey today.