Why Amazon SageMaker Autopilot?
Amazon SageMaker Autopilot Overview
Benefits of SageMaker Autopilot
Key Features
Data pre-processing and feature engineering
You can use Amazon SageMaker Autopilot even when you have missing data. SageMaker Autopilot automatically fills in the missing data, provides statistical insights about columns in your dataset, and automatically extracts information from non-numeric columns, such as date and time information from timestamps.
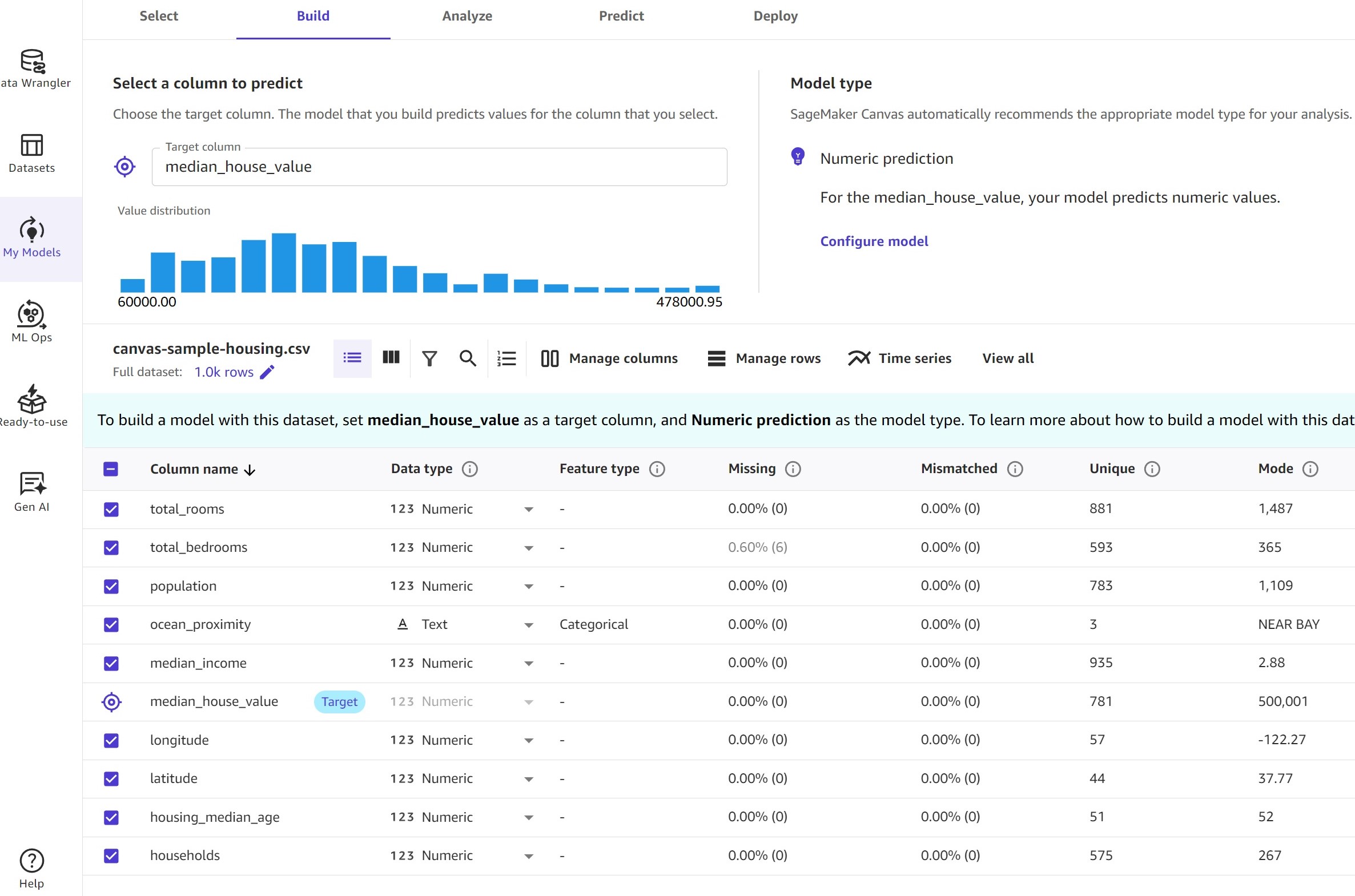
Automatic ML model selection
Amazon SageMaker Autopilot automatically infers the best type of predictions for your data, such as binary classification, multi-class classification, regression, or time series forecasting. It then runs a comprehensive AutoML cycle to evaluate hundreds of model candidates with various hyperparameters and algorithms to identify a highly accurate model that best fits your data.
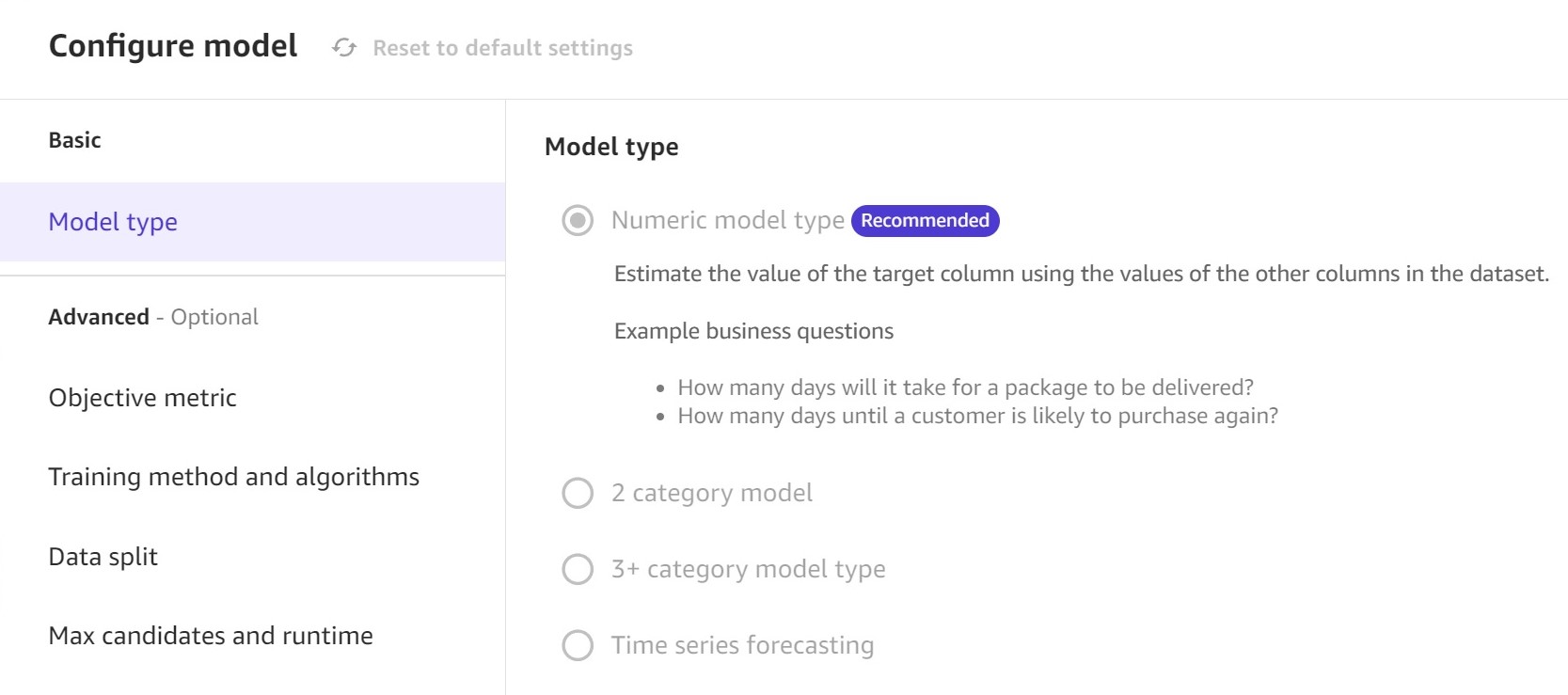
Model leaderboard
Amazon SageMaker Autopilot provides a ranked list of ML models that are automatically generated for your data. You can review key performance metrics, such as accuracy and precision for each model, and deploy the model best suited to your use case.
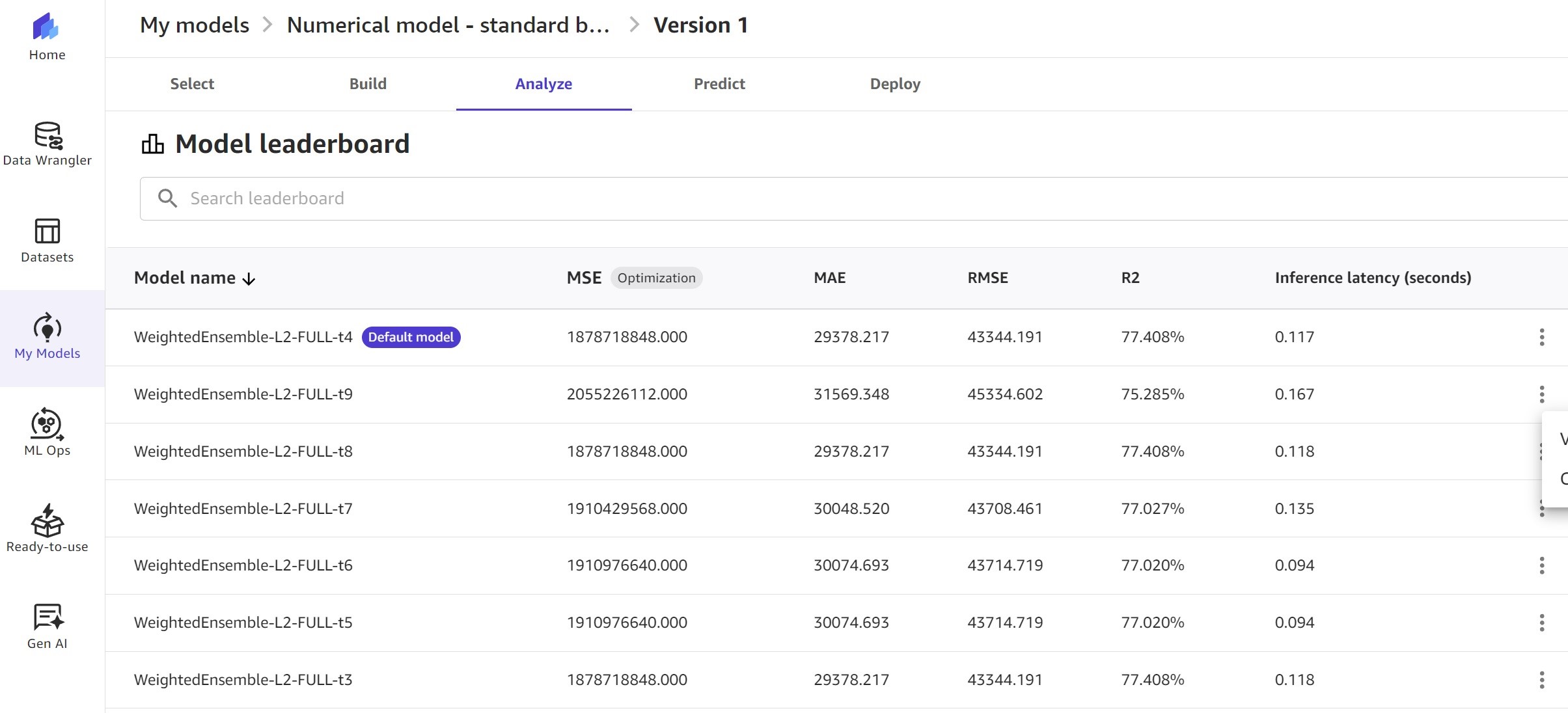
Customizable autoML journey
With Amazon SageMaker Autopilot, you can customize steps in your autoML journey to help create high quality ML models. You can apply your own data preprocessing and feature engineering transformations with 300+ pre-configured data transformations. You can iterate with custom data splits and training options, such as ensemble or hyperparameter optimization, for high-quality results.
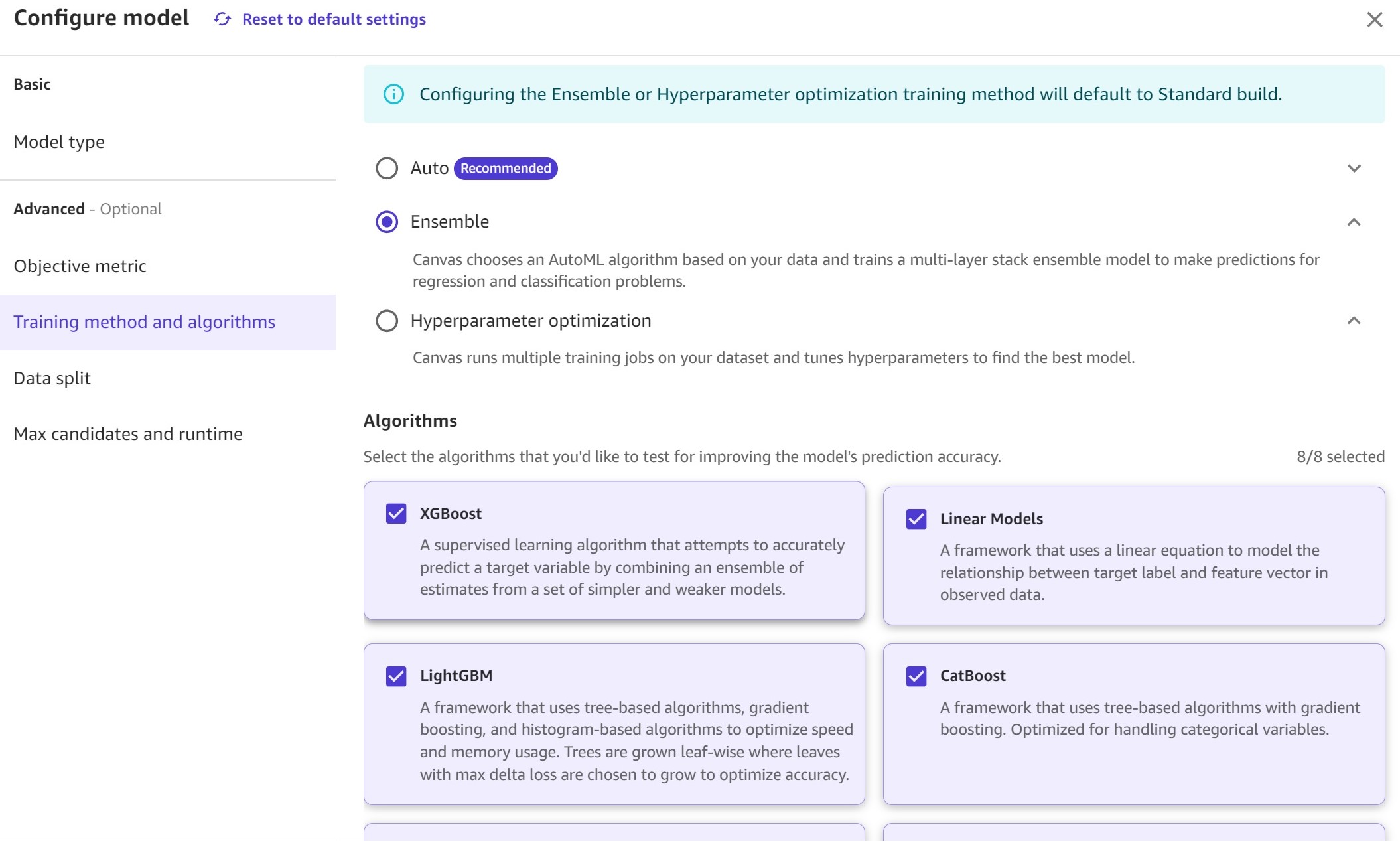
Automatic notebook creation
You can automatically generate a Amazon SageMaker Studio Notebook for any model Amazon SageMaker Autopilot creates and dive into the details of how it was created, refine it as desired, and recreate it from the notebook at any point in the future.
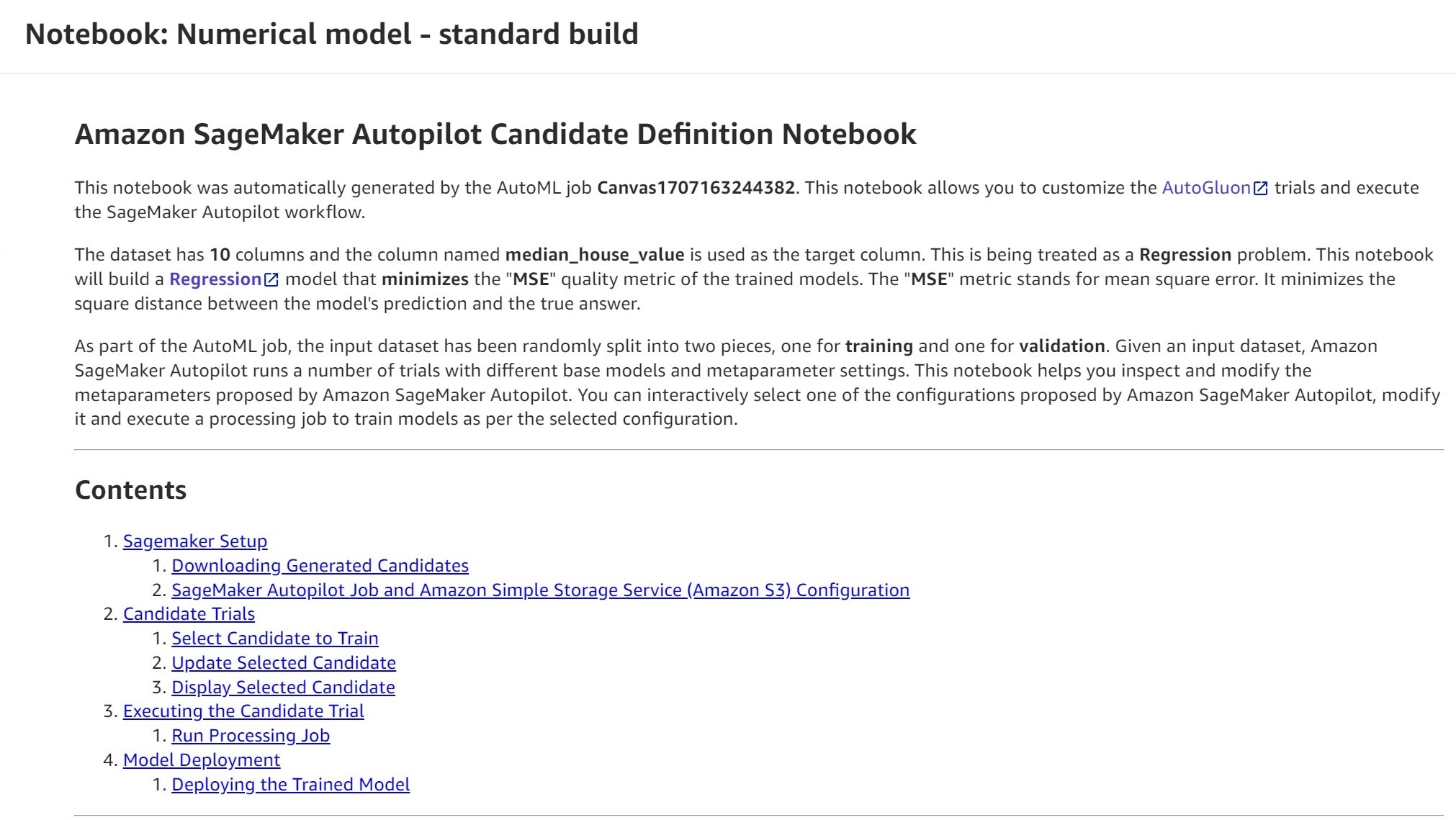
Use cases
-
Deloitte
Deloitte is a leading global provider of audit and assurance, consulting, financial advisory, risk advisory, tax and related services.
Through AWS’s no-code ML services such as SageMaker Canvas and SageMaker Data Wrangler, we at Deloitte Consulting have unlocked new efficiencies, enhancing the speed of development and deployment productivity by 30–40% across our client-facing and internal projects.
Vishveshwara Vasa, Cloud Chief Technology Officer, Deloitte -
SuccessKPI
SageMaker Canvas has empowered SuccessKPI to fine-tune Foundation Models using historical question-answer pairs, enabling more accurate assessment of agent-customer conversations without extensive (time consuming, laborious, costly) manual review. The fine-tuning capabilities leveraging AutoML allow SuccessKPI to optimize models and provide access to crucial performance metrics. By leveraging Canvas, SuccessKPI has significantly enhanced operational efficiency, automating conversation scoring, and improving the overall quality of contact center interactions.
Piyush Patel, Chief Technology Officer – SuccessKPI -
Thomson Reuters
Thomson Reuters is a leading source of information, including one of the world’s most trusted news organizations.
One of the challenges that our engineers face is managing customer call resources during peak seasons to ensure the optimal number of customer service personnel are hired to handle the influx of inquiries, historical analysis of call center data containing call volume, wait time, date, and other relevant metrics is time consuming. Our teams are leveraging the new data preparation and customization capabilities in SageMaker Canvas to train models on company data to identify patterns and trends that impact call volume during peak hours. It was extremely easy for us to build ML models using our own data, and we look forward to increasing the use of foundational models—without writing any code—through Canvas.
Maria Apazoglou, vice president of Artificial Intelligence, Business Intelligence and Data Platforms at Thomson Reuters. -
Bain & Company
Bain & Company’s Founder’s Studio is a part of Engine 2 Ventures, focused on ideating and building disruptive B2B SaaS ventures. Aura, one of its ventures, redefines workforce analysis by harnessing the power of 950 million professional profiles and 400 million job postings.
One of the biggest challenge for Aura is to extract meaningful insights from a vast pool of unstructured professional data, by employing Large Language Models via Amazon SageMaker Canvas, we've automated the data extraction process, transforming how companies assess workforce competencies and organizational structures. This approach not only helped us scale data analysis, but also bypassed the limitations of traditional data analysis methods like keyword matching. Using SageMaker Canvas' new data preparation and LLM capabilities, Aura is able to quantitatively score and benchmark companies on the effectiveness of their organization structure, skills of the workforce, and performance in terms of financial results.
Purna Doddapaneni, CTO of Founder’s Studio and Partner at Bain & Co. -
Samsung Electronics
Based in South Korea, Samsung Electronics is a global company offering people around the world access to technology, such as mobile phones, computers, and smart devices. The Samsung Device Solutions division of the company focuses on the inner workings of electronic devices to provide maximum performance, reliability, and longevity.
Using Amazon SageMaker Canvas is simple, and the interface is user friendly. Even a business analyst like me can analyze data and get insights using machine learning.
Dooyong Lee, Manager of Marketing Intelligence, Samsung Electronics -
Clarium
Clarium is a healthcare supply chain platform that uses machine learning and data tools to empower healthcare providers by optimizing inventory levels, reducing costs, and improving operational efficiency.
Our clients are often hindered by the quality and integration of their own data—a challenge that is ubiquitous among hospitals across the US. In addition, accumulated decades of inconsistent quality assurance practices and fragmented data processes have resulted in the arduous task of cleansing, validating, and integrating billions of rows of erroneous data. To help them tackle this challenge, our analytics teams use Amazon SageMaker Canvas to derive, cleanse, and standardize data coming from an unlimited number of sources from healthcare providers in the US, allowing them to easily build custom text classification models—all without writing a single line of code. With SageMaker Canvas, we offer our clients validated and standardized product descriptions and classifications for ERP data, verified clinical classifications for procedural utilization data, and data-driven recommendations designed to optimize the quality of patient care, all while reducing costs and saving time. By equipping our clients with trustworthy data and cutting-edge analytics, we empower them to look ahead and build a brighter future for healthcare, instead of constantly playing catch-up with the present.
Justin Jacobson, Head of Analytics, Clarium Health -
Siemens Energy
Siemens Energy is energizing society. They are transforming in key focus areas of environmental, social, and governance (ESG), and their innovation is making the future of tomorrow different today, for both their partners and their people.
The core of our data science strategy at Siemens Energy is to bring the power of machine learning to all business users by enabling them to experiment with different data sources and machine learning frameworks without requiring a data science expert. This enables us to increase the speed of innovation and digitalization of our energy solutions such as Dispatch Optimizer and Diagnostic services. We found Amazon SageMaker Canvas a great addition to the Siemens Energy machine learning toolkit, because it allows business users to perform experiments while also sharing and collaborating with data science teams. The collaboration is important because it helps us productionalize more ML models and ensure all models adhere to our quality standards and policies.
Davood Naderi, Data Science Team Lead at Industrial Applications, Siemens Energy -
INVISTA
A subsidiary of Koch Industries since 2004, INVISTA brings to market the proprietary ingredients for nylon 6,6 and recognized brands including CORDURA and ANTRON.
Our business analysts are data savvy, and we needed the ability to let them create predictive models. Equally important, however, was to ensure that our data science team had visibility into the models built so that they can support and productionize as needed. We foresee Amazon SageMaker Canvas empowering our business users and process engineers to start working on data science problems that were previously owned by the data science team. The intuitive user interface and easy-to-navigate options of Amazon SageMaker Canvas allow business users to import a variety of data, minimize the need to manually clean up data, and apply a variety of algorithms to find the model that best fits the data with a few clicks. The code and data can easily be sent to the data science team through Amazon SageMaker Studio, allowing them to integrate models into their model management system and see a full picture of models enterprise wide.
Caleb Wilkinson, Lead Data Scientist, INVISTA -
BMW Group
The BMW Group, headquartered in Munich, Germany, is a global manufacturer of premium automobiles and motorcycles, covering the brands BMW, BMW Motorrad, MINI, and Rolls-Royce. It also provides premium financial and mobility services.
The use of Artificial Intelligence as a key technology is an integral element in the process of digital transformation at the BMW Group. The company already employs AI throughout the value chain, enabling it to generate added value for customers, products, employees, and processes. In the past few years, we have industrialized many top BMW Group use cases measured by business value impact. We believe Amazon SageMaker Canvas can add a boost to our AI/ML, scaling across the BMW Group. With SageMaker Canvas, our business users can easily explore and build ML models to make accurate predictions without writing any code. SageMaker also allows our central data science team to collaborate and evaluate the models created by business users before publishing them to production.