แค็ตตาล็อก Amazon SageMaker
ค้นพบ กำกับดูแล และประสานการทำงานด้านข้อมูลและ AI อย่างปลอดภัย
ภาพรวม
Amazon SageMaker รุ่นใหม่ช่วยลดความยุ่งยากในการสำรวจ การกำกับดูแล และการทำงานร่วมกันสำหรับข้อมูลและ AI ใน Lakehouse โมเดล AI และแอปพลิเคชันของคุณ ด้วย Amazon SageMaker Catalog ซึ่งสร้างขึ้นจาก Amazon DataZone ผู้ใช้สามารถค้นพบและเข้าถึงข้อมูลและโมเดลที่ได้รับการอนุมัติได้อย่างปลอดภัยโดยใช้การค้นหาเชิงความหมายด้วยข้อมูลเมตาที่สร้างขึ้นโดย AI ช่วยสร้าง หรือคุณสามารถขอให้ Amazon Q Developer ด้วยภาษาธรรมชาติที่ค้นหาข้อมูลของคุณ ผู้ใช้สามารถกำหนดและบังคับใช้นโยบายการเข้าถึงได้อย่างสม่ำเสมอโดยใช้โมเดลการอนุญาตเพียงหนึ่งเดียว พร้อมการควบคุมการเข้าถึงแบบละเอียดใน สตูดิโอแบบครบวงจรของ Amazon SageMaker แชร์และทำงานร่วมกันกับข้อมูลและแอสเซท AI ได้อย่างราบรื่นผ่านเวิร์กโฟลว์การเผยแพร่และสมัครรับข้อมูลที่ง่ายดาย ด้วย SageMaker คุณสามารถปกป้องและคุ้มครองโมเดล AI ของคุณโดยใช้กฎควบคุมระบบ Amazon Bedrock และนำนโยบาย AI ที่มีความรับผิดชอบมาใช้ สร้างความไว้วางใจในองค์กรของคุณด้วยการตรวจสอบคุณภาพข้อมูลและระบบอัตโนมัติ การตรวจจับข้อมูลที่ละเอียดอ่อน ตลอดจนการติดตามที่มาของข้อมูลและแมชชีนเลิร์นนิง (ML)
ดูแค็ตตาล็อก Amazon SageMaker ในขณะกำลังใช้งาน
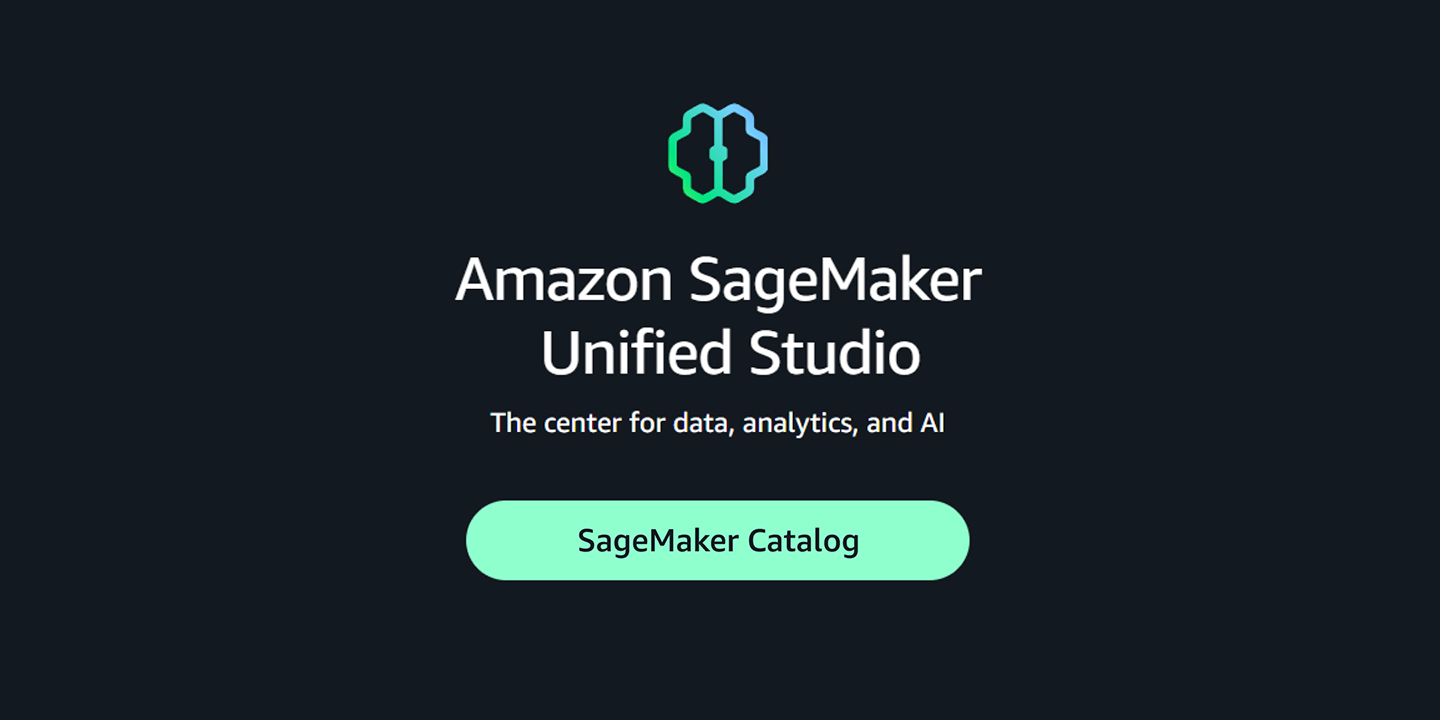
ประโยชน์
คุณสมบัติ
ข้อมูลที่จัดสรรไว้สำหรับบริบทและความสามารถในการค้นหา
แคตตาล็อกจะนำบริบททางธุรกิจเข้าสู่ข้อมูลเมตาทางเทคนิคของคุณและช่วยให้คุณสามารถเสริมสร้างบริบททางธุรกิจได้ คุณสามารถทำให้ข้อมูลปรากฏพร้อมบริบททางธุรกิจเพื่อให้ผู้ใช้ทุกคนค้นหา ทำความเข้าใจ และเชื่อถือข้อมูลได้อย่างรวดเร็วและไม่ซับซ้อน
คำแนะนำสำหรับข้อมูลเมตาแบบอัตโนมัติ
เพิ่มคำอธิบายและชื่อธุรกิจลงในข้อมูลโดยอัตโนมัติ ซึ่งจะช่วยให้คุณสามารถเข้าใจบริบทได้อย่างง่ายดาย และช่วยให้คุณไม่ต้องจัดการกับชื่อทางเทคนิคที่เป็นความลับ ซึ่งระบบอัตโนมัตินี้ขับเคลื่อนโดยโมเดลภาษาขนาดใหญ่ (LLM) เพื่อช่วยเพิ่มความแม่นยำและความสม่ำเสมอ
สร้างระดับความปลอดภัยของ AI ที่สม่ำเสมอในทุกแอปพลิเคชันของคุณ
Amazon Bedrock Guardrails จะช่วยประเมินอินพุตของผู้ใช้และการตอบสนอง FM ตามนโยบายเฉพาะกรณีใช้งาน และให้ชั้นการป้องกันเพิ่มเติมโดยไม่คำนึงถึงโมเดลพื้นฐาน
ตรวจสอบและติดตามโมเดลได้อย่างรวดเร็ว
ตรวจสอบและแก้ไขปัญหาประสิทธิภาพอย่างรวดเร็วสำหรับโมเดล ตำแหน่งข้อมูล และงานเฝ้าสังเกตโมเดลทั้งหมดผ่านมุมมองแบบรวม ติดตามการเบี่ยงเบนจากพฤติกรรมของโมเดลที่คาดไว้ รวมถึงงานการเฝ้าสังเกตที่ขาดหายไปหรือไม่ได้ใช้งานด้วยการแจ้งเตือนอัตโนมัติ
คุณภาพข้อมูล
ผู้บริโภคข้อมูลสามารถใช้สถิติคุณภาพข้อมูลเพื่อดูเมตริกคุณภาพข้อมูลจากการตรวจคุณภาพข้อมูลของ AWS หรือระบบจากภายนอกได้ ผู้บริโภคข้อมูลสามารถไว้วางใจแหล่งที่มาของข้อมูลที่ใช้ในการตัดสินใจ และมีบริบทคุณภาพของข้อมูลขณะค้นหาสินทรัพย์ นอกจากนี้ ผู้ผลิตข้อมูลและทีมไอทีสามารถใช้ API เพื่อรวมสถิติคุณภาพข้อมูลจากระบบจากภายนอกเข้ากับพอร์ทัลที่ไม่ใช่คอนโซลแบบรวมศูนย์
ข้อมูลและเส้นทางขั้นตอนของข้อมูล ML
ทำความเข้าใจการย้ายข้อมูลเมื่อเวลาผ่านไป เส้นทางขั้นตอนของข้อมูลสามารถเพิ่มความเชื่อมั่นและการรู้เท่าทัน AI ขององค์กรได้โดยช่วยให้ผู้บริโภคข้อมูลเข้าใจว่าข้อมูลมาจากไหน มีการเปลี่ยนแปลง และการบริโภคอย่างไร คุณสามารถลดเวลาที่ใช้ในการแมปข้อมูลและแอสเซท AI พร้อมด้วยความสัมพันธ์ การแก้ไขปัญหาและการพัฒนาไปป์ไลน์ รวมถึงการยืนยันแนวทางปฏิบัติในการกำกับดูแลข้อมูลและ AI
ลูกค้า
CISCO
“คุณต้องการค้นหา แชร์ และควบคุมดูแลข้อมูลของคุณ ข้อมูลมีอยู่ในทีมต่าง ๆ ในหลาย Silo และคุณต้องการวิธีการรวบรวมข้อมูลเข้าด้วยกัน ไม่ว่าคุณจะเรียกว่าตาข่ายข้อมูลหรือผืนผ้าข้อมูลก็ตาม แคตตาล็อก Amazon SageMaker จะเชื่อมต่อผู้ผลิตข้อมูลและผู้บริโภคเข้าด้วยกัน ทำให้ผู้ผลิตสามารถแชร์ข้อมูลโดยใช้การควบคุมในตัวและสัญญาข้อมูลได้ในขณะที่อนุญาตให้ผู้บริโภคสามารถเข้าถึงข้อมูลโดยใช้เครื่องมือที่ต้องการได้”
Shaja Arul Selvamani ผู้อำนวยการอาวุโสฝ่าย AI/ML ของ Cisco
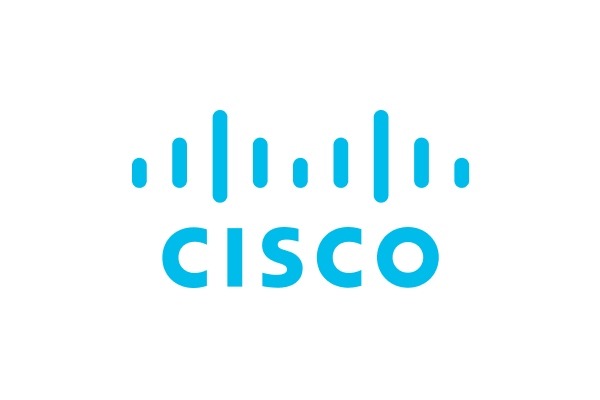
Natera, Inc
“องค์กรของเราได้ใช้ประโยชน์จาก Amazon DataZone, Amazon SageMaker AI, Amazon Athena และ Amazon Redshift เพื่อจัดการและวิเคราะห์ข้อมูลทางคลินิกและจีโนมของเรา เรารู้สึกตื่นเต้นที่ได้มีการกำกับดูแลแบบครบวงจรของ Amazon SageMaker Catalog ซึ่งจะช่วยเพิ่มประสิทธิภาพในการค้นพบและการเข้าถึงข้อมูลของเรา โดยเปิดโอกาสให้ทีมของเราสามารถวิเคราะห์ข้อมูลที่เกี่ยวข้องในโดเมนทั้งหมดของเราได้อย่างรวดเร็ว การผสานการทำงานนี้จะช่วยให้เราสามารถสร้างชุดข้อมูลที่ปรับแต่งได้ ซึ่งอาจช่วยลดเวลาในการรับข้อมูลเชิงลึก และผลักดันให้ผู้ป่วยมีอาการดีขึ้นในที่สุดเมื่อเรากำลังก้าวไปสู่เป้าหมายของเราในการทำให้การทดสอบทางพันธุกรรมที่ปรับให้เหมาะกับแต่ละบุคคลเป็นส่วนมาตรฐานของการดูแลรักษา”
Mirko Buholzer รองประธานฝ่ายวิศวกรรมซอฟต์แวร์ของ Natera, Inc.
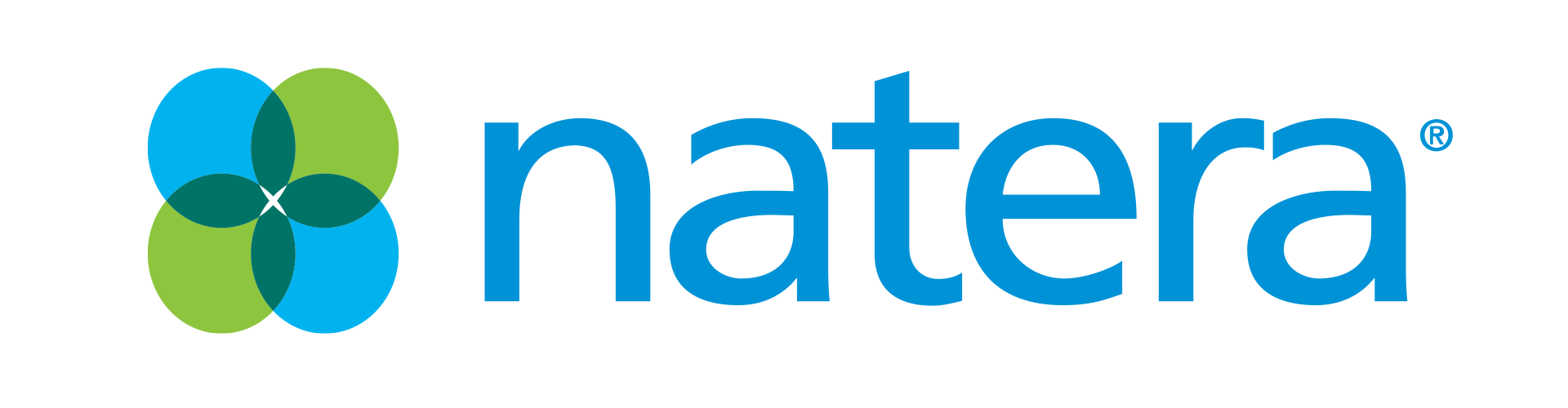
NatWest
“ทีมวิศวกรรมแพลตฟอร์มข้อมูลของเราได้นำเครื่องมือสำหรับผู้ใช้ปลายทางหลายอย่างมาใช้ในงานด้านวิศวกรรมข้อมูล ML, SQL และ AI ช่วยสร้าง ขณะที่เราต้องการลดความซับซ้อนของกระบวนการทั่วทั้งธนาคาร เราก็ได้มองหากาเพิ่มประสิทธิภาพในการยืนยันตัวตนผู้ใช้และการอนุญาตการเข้าถึงข้อมูล Amazon SageMaker มอบประสบการณ์ผู้ใช้สำเร็จรูปเพื่อช่วยให้เราใช้งานในสภาพแวดล้อมเดียวภายในองค์กร ซึ่งช่วยลดเวลาที่ผู้ใช้ข้อมูลของเราใช้ในการเข้าถึงเครื่องมือใหม่ ๆ ได้ประมาณ 50%”
Zachery Anderson ประธานเจ้าหน้าที่ฝ่ายวิเคราะห์ข้อมูลของ NatWest Group
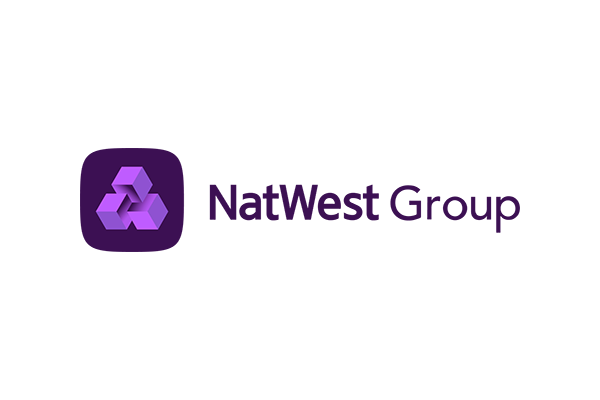
เริ่มต้นใช้งานแค็ตตาล็อก Amazon SageMaker
วันนี้คุณพบสิ่งที่กำลังมองหาแล้วหรือยัง
การแจ้งให้เราทราบจะช่วยให้เราปรับปรุงคุณภาพของเนื้อหาในหน้าเว็บได้