Amazon EMR Partners
Drive innovation and unlock greater business value with AWS Specialization Partners that have deep technical knowledge and proven customer success
Amazon EMR Delivery Partners are AWS Partners who help you build and deploy big data analytics applications to easily run big data frameworks such as Apache Hadoop, Spark, Presto, Hive, and HBase on massively scalable clusters. They build solutions that handle big data use cases including log analysis, web indexing, data transformations (ETL), machine learning, financial analysis, scientific simulation, and bioinformatics according to best practices.
The AWS Service Delivery Program enables AWS customers to identify AWS Partners with experience and a deep understanding of specific AWS services. These Amazon EMR Delivery Partners have passed a rigorous technical validation where AWS makes sure they are following best practices with EMR. They also provide case studies demonstrating successful customer service delivery.

Connect with Amazon EMR Partners
Drive innovation, meet business objectives, and get the most out of your AWS services by partnering with technically validated AWS Partners.
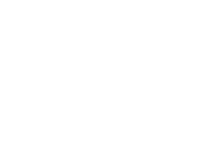
Next Steps
Find an AWS Partner »
Connect with AWS Specialization Partners with global expertise in Partner Solutions Finder.