AWS goes inside the NFL 2022 Big Data Bowl with the winning team
As strong believers in open science, AWS is a proud sponsor of the Big Data Bowl to spur on innovation from the next generation of engineers, researchers, and data scientists.
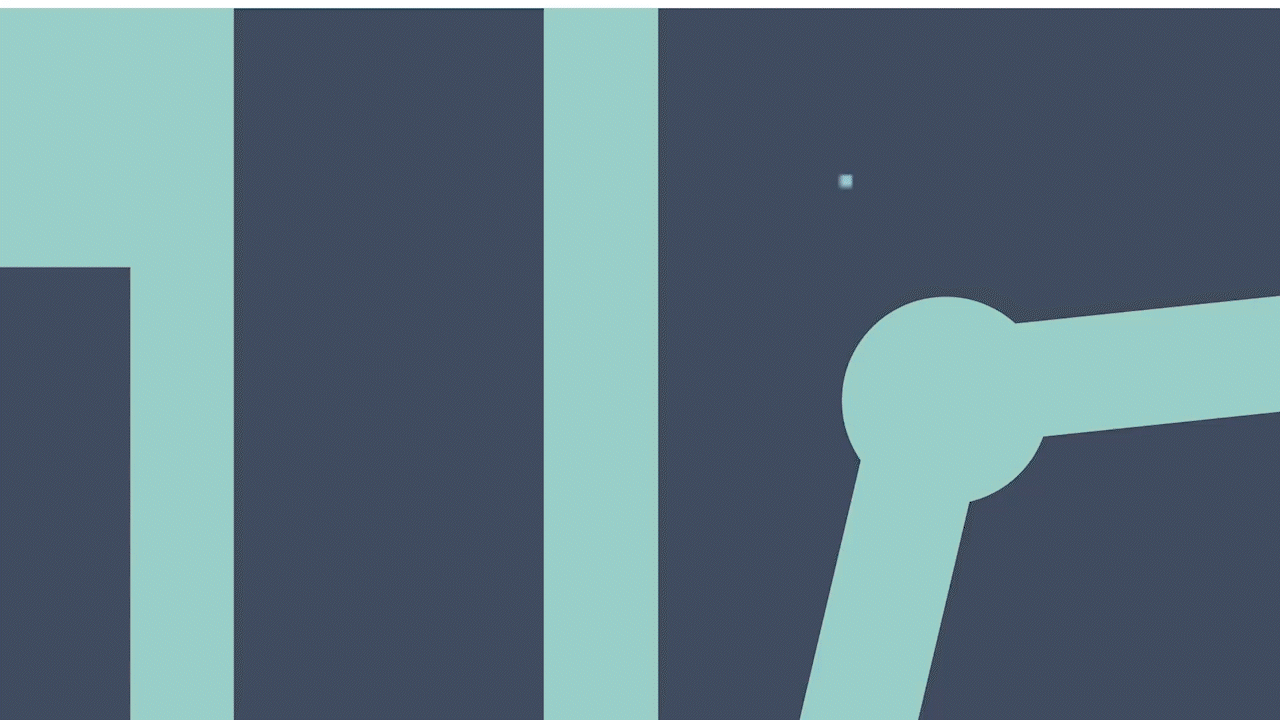
The fourth annual Big Data Bowl champions have been crowned—graduate students Robyn Ritchie, Brendan Kumagai, Ryker Moreau, and Elijah Cavan of Simon Fraser University in Burnaby, British Columbia. This year’s competition marks some exciting firsts. It’s the first time a collegiate team has won the overall competition, and it’s the first time a female has been part of the championship team.
The contest, sponsored by AWS and hosted by NFL Football Operations, challenges members of the analytics community—from college students to professionals—to explore statistical innovations in football. Contestants use traditional football data and Next Gen Stats to create new insights, uncover and learn team and player strategies, and make the game more exciting for fans.
For example, building upon the winning entry of Austrian data scientists Philipp Singer and Dmitry Gordeev in the 2020 Big Data Bowl, the Next Gen Stats Analytics team introduced a set of metrics that use player-tracking data to delve deeper into the ground game, derived from the recently developed ability to calculate Expected Rushing Yards.
This year’s theme centered around devising new approaches in analyzing special teams and identifying what strategies make for a successful punt, field-goal, and extra-point play. Participants were given access to the NFL's Next Gen Stats, including information on the speed, direction, and location of all 22 special teams players on the field from 2018-20. Participants also received data from PFF, allowing entrants to blend tracking and scouting metrics together.
The top idea? A framework to evaluate punt return performance through a mixture of video review, data visualization, and novel metrics.
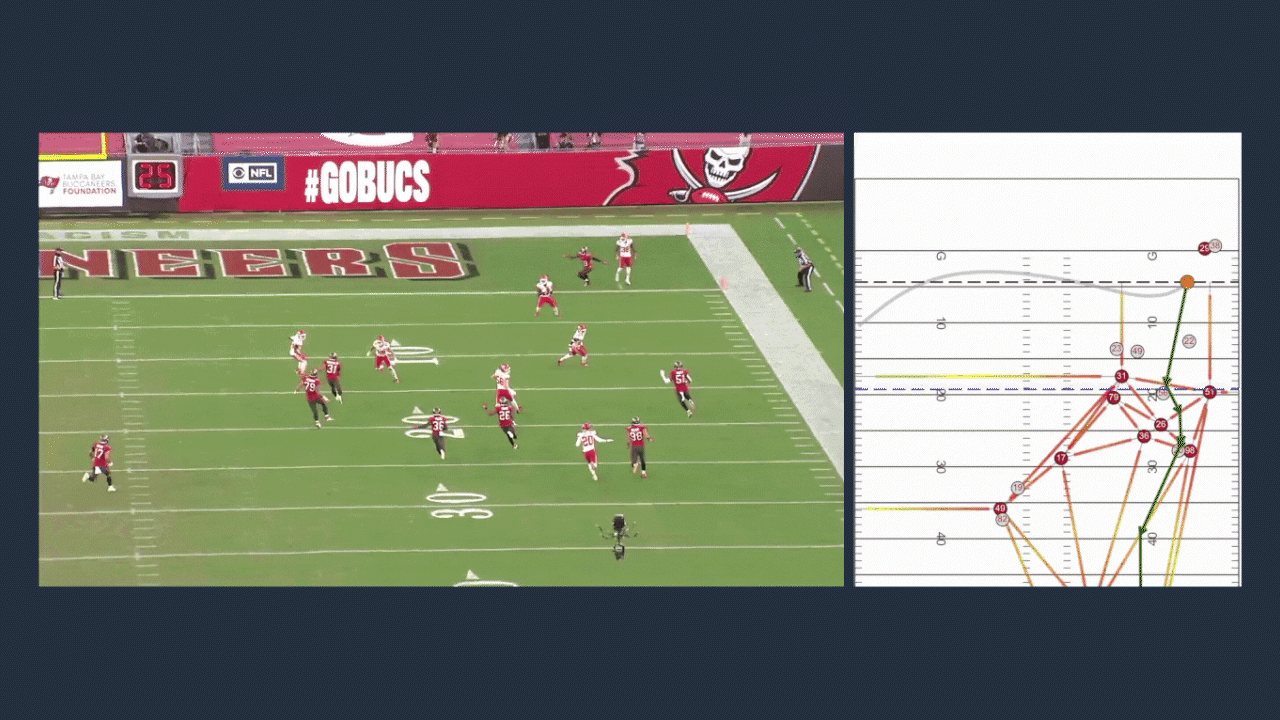
To begin, the optimal route to the endzone is determined by identifying gaps the returner can exploit through quantifying field pressure. An algorithm then connects the dots between the actionable gaps and finds the path that leads to as many yards as possible. Once this path is determined, the model quantifies the path deviation between the optimal and observed paths. This is used to evaluate the punt returner’s decision-making and as a novel metric in predicting the expected yards remaining on a play. Finally, the distribution from the model is used to evaluate returns through a metric called Return Yards Above Expected (RYAE). All together, these methods quantify how successful the return was and the reason behind the success.
We huddled up with the champs to learn more about their experience and innovative approach.
You can read their technical submission on Kaggle here.
SFU: Robyn Ritchie, Brendan Kumagai, Ryker Moreau, and Elijah Cavan
Q1: What drew you to entering the Big Data Bowl?
Brendan: All of us came here to do sports analytics in some capacity. There’s a bit of a history with previous Big Data Bowl winners here and a lot of alumni are now in the sports analytics industry. I think our first week here, the Big Data Bowl was announced. So, I thought, hey, they won it here before a few years ago, so we should give it a shot.
Q2: What made this year’s Big Data Bowl unique or appealing to you?
Robyn: It’s definitely becoming more common to see these types of competitions in sports. They all release minimal data. The NFL is really unique in how much data they give you and the quality of the data is unreal. You don’t see that from a lot of contests like this.
Brendan: Looking at the NFL data, you can see just how clean the tracking data is to work with. You look at the trajectories, they're very smooth compared to some of the other data that I’ve worked with like experimental broadcast-feed player tracking data, which is choppier and tougher to manage.
Ryker: All the previous Big Data Bowls were for instance offensive based, but you had to focus on running backs; defense, but you had to focus on pass coverage. This year, they gave us special teams and they were like, go nuts. Do whatever you want. You can pick any facet of special teams and run with it that way. So that's what kind of made it unique.
Q3: How did you come up with your idea? Why punt returns?
Brendan: From the beginning we were thinking punt returns because we felt you could leverage the tracking data more and create cooler things than other areas of special teams. Additionally, punt returns also offer an interesting advantage over your typical run play. If you were looking at a running play on offense, the running back starts behind a large cluster of offensive and defensive linemen. It would be very difficult to find seams in this cluster that he can exploit to gain yards as all the linemen packed so tightly together. Whereas in our case, we had the punt returner catching the ball well downfield with all the defenders spread out as they run towards the returner in an attempt to stop him.
Robyn: I personally enjoy looking at the decision making in different sports and observing players. It's really hard to quantify something like this. You want a longer play where there's more points where you can make a decision. Punt returns tend to be somewhat of the longer situations where they'll be multiple decision points throughout the play, and we can try to evaluate as many of them as possible to give that play even just one qualifying measure to be able to compare them all.
Q4: What was the process of building your idea out?
Robyn: It really originally started with the convex hull that quantifies the space the team is taking up. Where are they around the punt returner? And that's where we really started and that took us the whole first month. Eventually, I asked, can we find our way through the convex hull? And then it just kind of snowballed. We had so many different ideas to bring in, like blocker leverages, the convex hull, the modeling aspects, and the optimal path. Brendan and I focused on getting the optimal path correct and working properly. And then Riker and Eli took a whole lot of the modeling aspects and building various metrics that we wanted to put into our model.
Q5: Was there a pivotal moment along the way?
Elijah: We were trying to develop a model that complemented what Robyn and Brendan were doing with finding the optimal punt return path. Is it possible to approximate how many yards per play we get when the returner catches the ball? Ryker came to me, showed me a paper and he said, hey, these guys are doing that kind of setup. Instead of just one estimate, like the player is going to get seven yards, they were using this kind of conditional density to give you a distribution that says, okay, when x probability is going to get this amount, y probability is going to get you this much. You're introducing that and then working together to implement that model, which is more complex than what we were starting out with. That was a lightbulb moment for us.
Q6: What was the biggest challenge you faced?
Ryker: Prepping of the data was the hardest part. We had all these different measures we created like blocker leverage, defender leverage, determining if a player was blocked or not, stuff like that. So, there was a lot of manipulation in that sense. With a row of data for every 10th of a second of footage over three years, it became a computationally heavy aspect of the work. A lot of time we were like, oh, we have a new metric, let's add it. So, you have to redo the whole process over and over again. The optimal path stuff was its own beast. But the modeling side and the data prep was computationally heavy.
Q7: How do you see your work applying to coaches and players in the NFL?
Ryker: The main things that we presented in our final presentation was being able to make post-game analysis and evaluate player decision-making. There's a human element in football that’s never going away and analytics is never going to be the end all, but I think this is another tool that can be used by teams in their review process. This is a way to make minor improvements. However, I also think it's going to be a big player evaluation thing, where this allows teams to identify free agents or players in their own organization they may want to test out as a returner.
Q8: What has winning this meant to you? Any advice for other students?
Brendan: I didn't really expect to get this far, to actually win the grand championship, including the open division. When we first started this out, we were just hoping to make it to the finals. it was cool to look at the other schools and professionals we were going up against—there was a professor, past champions, and students from a lot of other great schools like MIT, Duke, and Wharton. To come from this little university up in Canada and to be able to compete with them was pretty cool.
Ryker: It's inspiring for people to register and form a team, even undergrad or graduate students, and use the data to put together a cool project. I didn't really expect to be a finalist until we got positive feedback online. A lot of people said, wow, you should have went for the open division. And we were kind of shocked by it, coming from a little school up in Canada. I think this bodes so well for the NFL internationally getting more people working with their data and into sports analytics.
Elijah: Yeah, I’ll just add on that with companies like AWS, Kaggle, Google—the way that they're putting up tutorials makes it so that undergrads and high school students can access this material and can get the kind of knowledge industry experts have. So, it wasn't too surprising for me to see some really, really good college submissions along with the open submissions because I think we’re at the point now where it's hard to tell the difference between the two.
Robyn: I would say to any student who's interested, it's great—just even to start looking at the data, maybe reading a couple papers on other sports that you might like, and then try to adapt it to work in a football environment. A lot of ideas started from other sports like soccer, curling, and hockey. Being able to adapt and manipulate it to the specific football situation is great practice. Even if you're a student and you're not sure about entering, just play around with the data. It's available. You can learn from it and then try again next year when you have a team and more skill development.
Q9: Robyn, what are your thoughts on being the first female champion?
Robyn: I think it's definitely exciting. I'm very honored to be the first female and it's nice to see that community come together. There’s a good amount of women in sports analytics that support each other and are trying to push each other and push the issue that there needs to be more women on those teams and that we have great perspectives. It's great to see the support and it’s definitely something that's building, like the CFL introducing the Women in Football Program which is meant to encourage inclusivity, diversity and equity. It's great to see stuff like that; small initiatives that will get the ball rolling.
Congrats to the NFL 2022 Big Data Bowl champions!