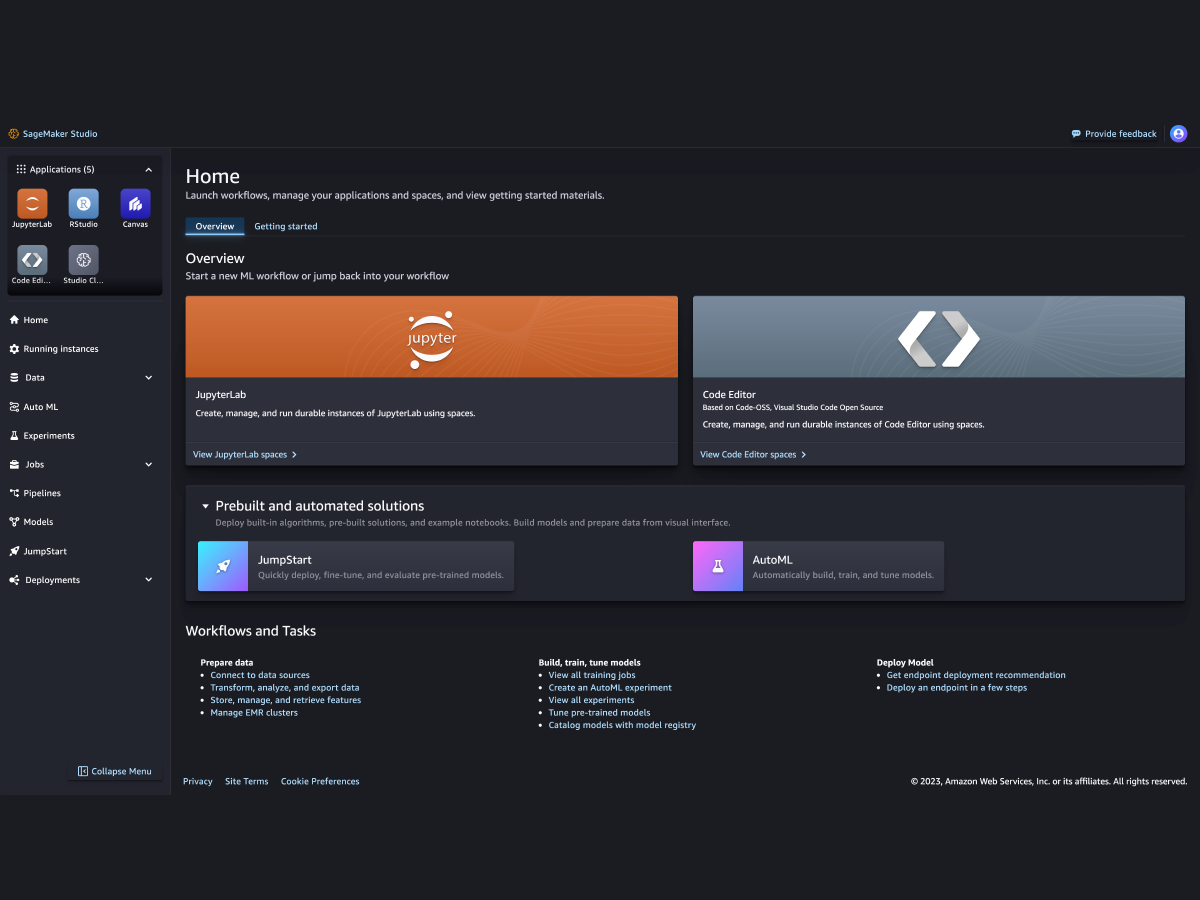
Why SageMaker Studio?
Amazon SageMaker Studio offers a wide choice of purpose-built tools to perform all machine learning (ML) development steps, from preparing data to building, training, deploying, and managing your ML models. You can quickly upload data and build models using your preferred IDE. Streamline ML team collaboration, code efficiently using the AI-powered coding companion, tune and debug models, deploy and manage models in production, and automate workflows—all within a single, unified web-based interface.
How it works
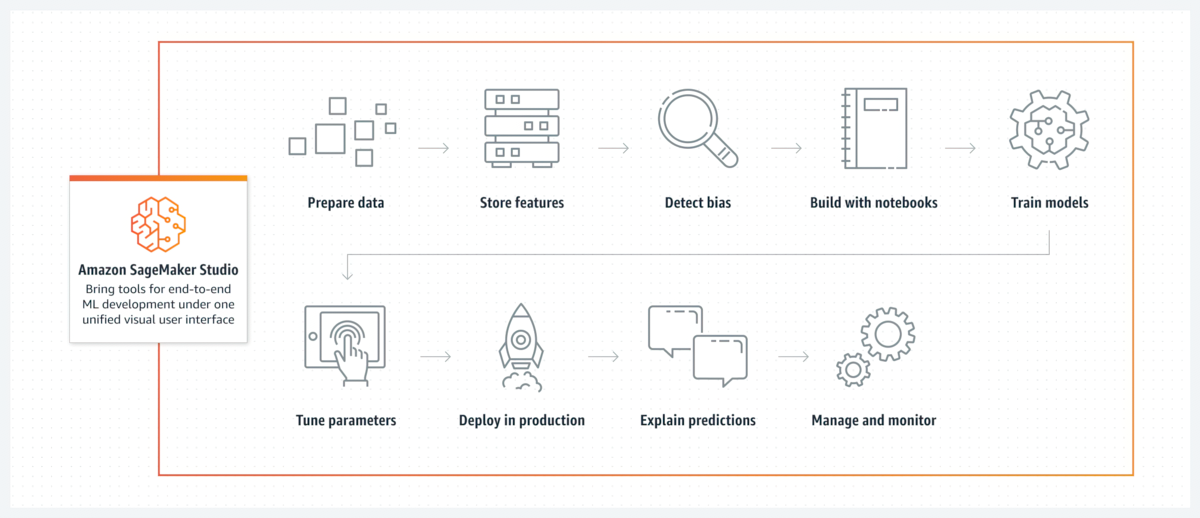