PyTorch on AWS
A highly performant, scalable, and enterprise-ready PyTorch experience on AWS
Accelerate time to train with Amazon EC2 instances, Amazon SageMaker, and PyTorch libraries.
Speed up research prototyping to production scale deployments using PyTorch libraries.
Build your ML model using fully managed or self-managed AWS machine learning (ML) services.
How it works
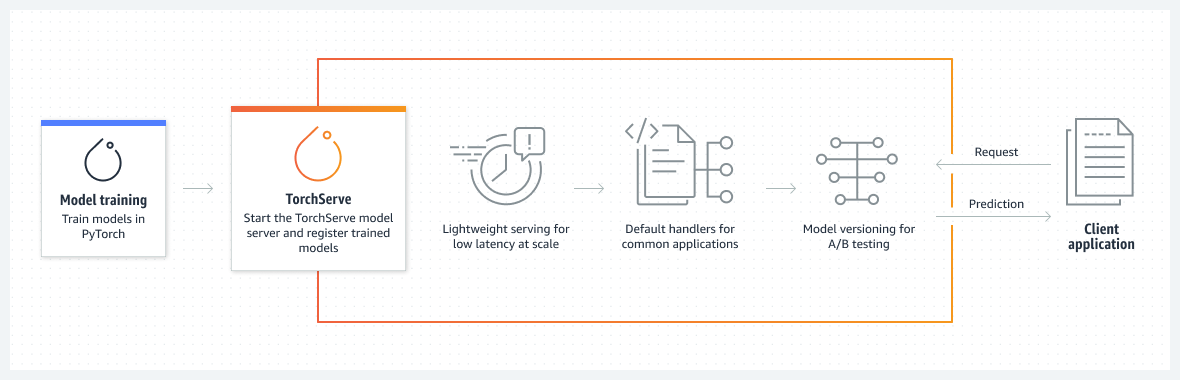
Use cases
Distributed training for large language models
Use PyTorch Distributed Data Parallel (DDP) systems to train large language models with billions of parameters.
Learn more »
Inference at scale
Scale inference using SageMaker and Amazon EC2 Inf1 instances to meet your latency, throughput, and cost requirements.
Learn more »
Multimodal ML models
Use PyTorch multimodal libraries to build custom models for use cases such as real-time handwriting recognition.
Learn more »
How to get started
Learn ML with Amazon SageMaker Studio Lab
Learn and experiment with ML using a no-setup, free development environment
Get started with PyTorch on AWS
Find everything you need to get started with PyTorch on AWS.
Explore more about PyTorch on AWS
Check out key features and capabilities to begin working with PyTorch.
Build with Amazon SageMaker JumpStart
Discover prebuilt ML solutions that you can deploy with a few clicks.