Production Optimization
- Artificial lift optimization
Increase productivity and avoid unnecessary downtime across all types of artificial lifts - electric submersible pumps (ESPs), plunger lift, rod lift, progressing cavity pumps (PCPs), gas lift, and hydraulic lift.
- Full-field optimization of networked resources
Production is increased while accounting for the dependencies between wells in the same field connected by porous media.
Improving maintenance and field operations could add up to $250 billion of value by 2030
Upstream Use Case
1
Drilling optimization and automation
2
Production optimization
3
Enhanced field operations
4
Digitally enabled logistics
5
Smart maintenance
Incremental value creation by 2030
$80-110B
$25-50B
$25-37B
$30B
$20B
Cost reduction for barrel of oil equivalent (BOE)
3-7%
2-5%
2-3%
2-5%
2-3%
*Source: McKinsey, How tapping connectivity in oil and gas can fuel higher performance (November 2020)
Customer References
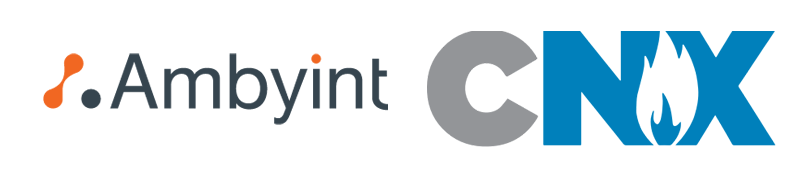
Challenge:
CNX Resources had under-optimized wells with increased liquid loading frequency which was resulting in increased GHG emissions, decreased gas production, and overall reduced workforce efficiency.
Solution:
Ambyint’s InfinityPL allowed CNX to optimize well productivity with improved analytics, develop autonomous management of plunger triggers/setpoints, improve production efficiency, with only data integration with no additional hardware.
Impact:
- Reduced GHG emissions by 48% through reduced liquid loading and well venting events
- Increased natural gas production by 4% with predictive maintenance and autonomous management of control system setpoints
- Scaled up solution to additional wells after successful pilot results
- Enhanced workforce efficiency by reducing number of screens, simplifying workflows, and automating manual tasks
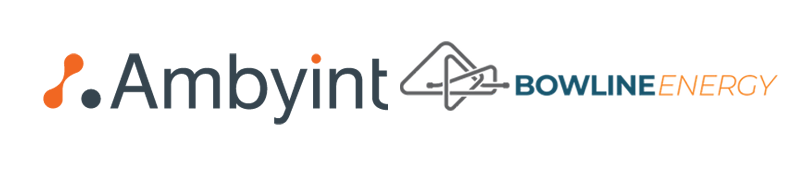
Challenge:
Bowline Energy needed to optimize and upgrade rod lift wells that had high failure rates resulting in significant equipment downtime and deferred revenue.
Solution:
Ambyint’s InfinityRL allowed for scaled production workflows without additional headcount and delivered autonomous operations for setpoint management and rod lift optimization.
Impact:
- Improved Mean Time Between Failures (MTBF) by 28%, reducing workover costs, well downtime, and deferred production
- Expansion to all wells resulted in 10x ROI through production gains, OpEx reduction, workforce efficiency, and GHG reduction
- Reduced GHG emissions by 10% through reduced electricity consumption
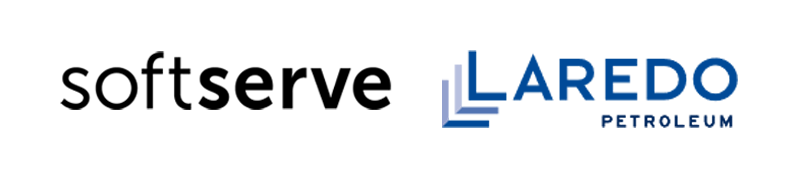
Challenge:
Laredo Petroleum had wells that run on ESP which needed constant monitoring and tuning of operating parameters to achieve efficiency and production targets. Finding the optimal combination of operating parameters was also a difficult and time-consuming process.
Solution:
Softserve developed an a custom application to optimize Laredo’s ESP performance with a combination of ML-based and physics-based models. The models were trained in several months from 50 ESPs. The application included interactive visualization of simulation scenarios and recommendation of control parameters.
Impact:
- Real-time dashboards provide summary statistics, forecasts, and recommendations for better decision-making
- Pilot objective was estimated to see additional 400 BPD. Now they have achieved improved production by >800 BPD across 29 pilot wells
- Reduced downtime, better maintenance practices, reduced OpEx
- The solution scaled to 100+ wells in production in several months.
- The solution is highly flexible and could be adapted to a vast variety of ESP system.
“Implementing an AI-based solution within a large oil and gas company is a challenge... it was SoftServe’s expertise that helped us successfully move to AI-driven ESP optimization built on AWS, which led to increased oil and gas production.”
David Benham, Principal Data Scientist & Manager of Innovation and Data Analytics, Laredo Petroleum
“Our leadership team understands the role that technology plays in improving production outcomes. After a thorough vetting process, we chose Ambyint to give us scaled production optimization across our asset base. Their technology provides our operations team a wider span of control significantly reducing failures, lowering LOE, and increasing production.”
Tony Hale, Vice President of Operations, Bowline Energy
Reducing GHG emissions, increasing production for plunger lift in the Marcellus shale
Boost oil production by optimizing operating parameters of ESP with AI | SoftServe
How to get started
Step 1: Deployment Readiness Assessment
Activities
- Business case definition
- Feasibility analysis for analytics/ML
- Data strategy
- Security Strategy
Outcomes
- Readiness and Maturity Assessment
- Infrastructure inputs into Planning
- Engagement Scope
Step 2: Deployment planning
Activities
- Evaluate analytics/ML strategies
- Data lake environment review
- Enrich data lake with additional sources
- Architecture design
Outcomes
- Reference architecture
- Analytics/ML strategy
- End-to-end engagement plan
Step 3: Deployment execution
Activities
- Security (implement firewalls and data security) implement analytics workflow
- Build data visualization/UI
- End-to-end testing of workflow
Outcomes
- Implemented analytics/ML workflow
- Implemented data visualizations/UI
- Deployed use cases
Visit the AWS Solutions Library so you can learn how to get started with Production Optimization and other solutions for the energy industry.