Amazon SageMaker Ground Truth features
Use human generated data to customize FMs on specific tasks or with company and industry data
Supervised Fine Tuning
Through supervised learning, models are provided concrete examples of desired outputs. These examples are called demonstration data, and they allow a model to learn how to respond and answer to future, unseen user requests. With SageMaker Ground Truth Plus, an AWS team of expert annotators can generate new high quality demonstration data based on your specific instructions. Some examples of demonstration data include captions for images and videos, text summarizes, answers to questions, and more. Demonstration data can be used to either customize an existing FM for your use case or to fine-tune a model you build from scratch.
- Question and answer: With question and answer pairs, you can prepare demonstration datasets to train your large language model on how to answer questions.
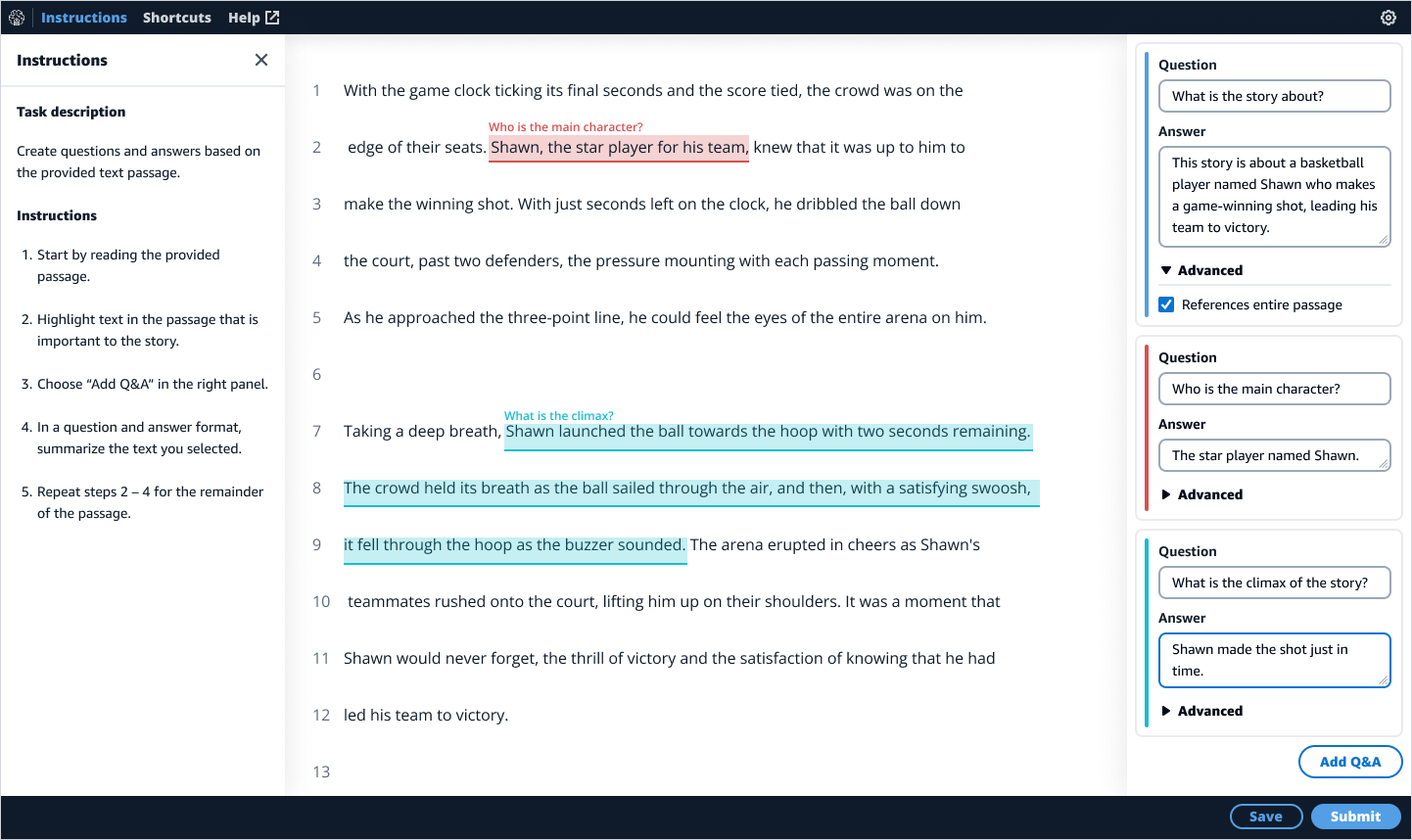
- Image captioning: With image captioning, you can prepare datasets that describe the scene and objects in an image in rich detail in order to train text-to-image models so they create accurate and creative images aligned with your intent. It can also be used to train image-to-text models so they output accurate descriptions of the image scene.
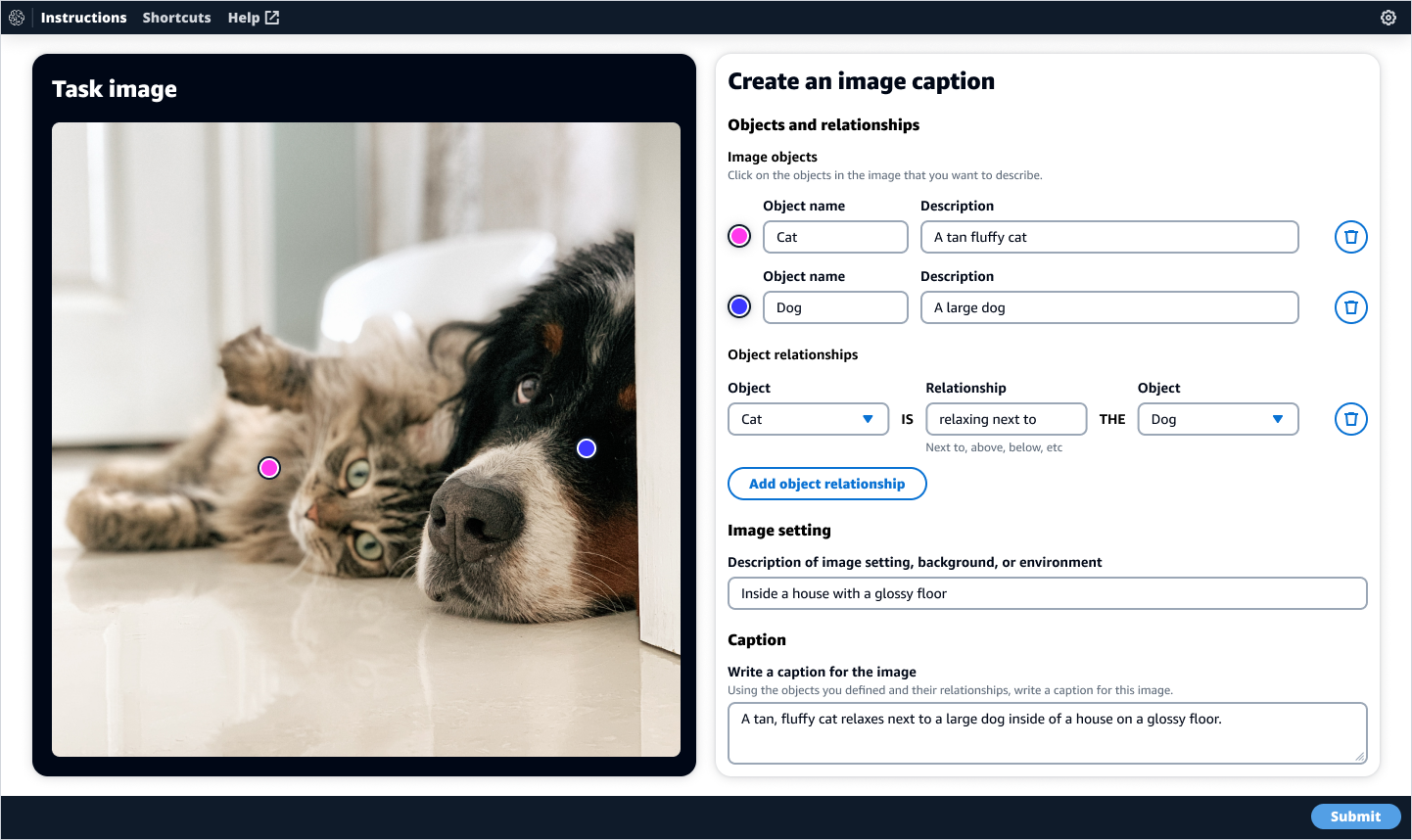
- Video captioning: With video captioning, you can prepare datasets that describe actions and the scene of a video in rich detail in order to train text-to-video models. High quality video captioning training data results in more accurate and creative videos aligned with your intent. It can also be used to train video-to-text models so they give an accurate description of the video.
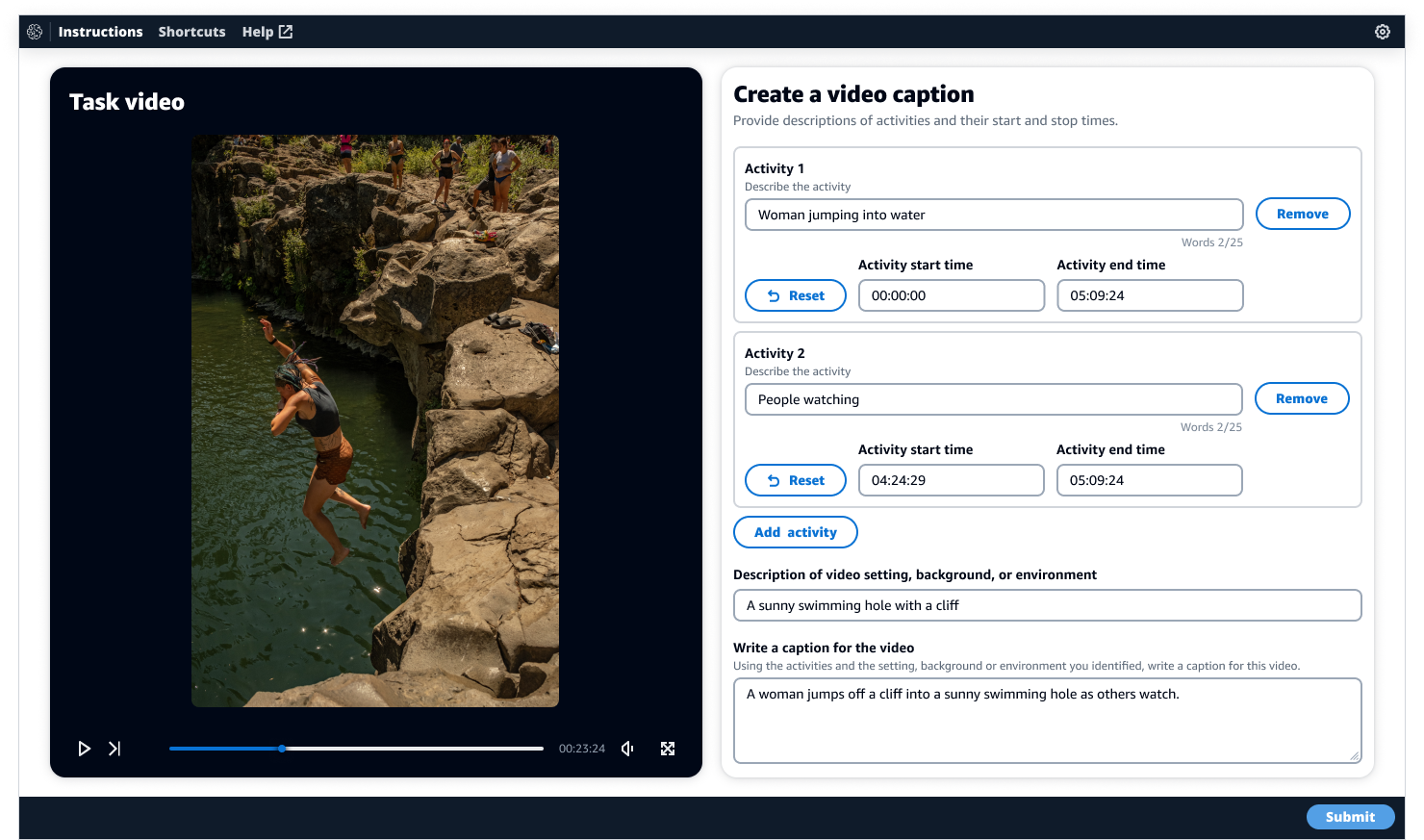
Reinforcement learning from human feedback (RLHF)
In reinforcement learning from human feedback (RLHF), a data annotator can give direct feedback and guidance on output that a model has generated by ranking and/or classifying its responses. The data, which is referred to as comparison and ranking data, is then used to train the model. An example comparison and ranking data includes ranking text responses from best to worst based on criteria like accuracy, relevancy, or clarity. Comparison and ranking data can be used to either customize an existing FM for your use case or to fine-tune a model you build from scratch.
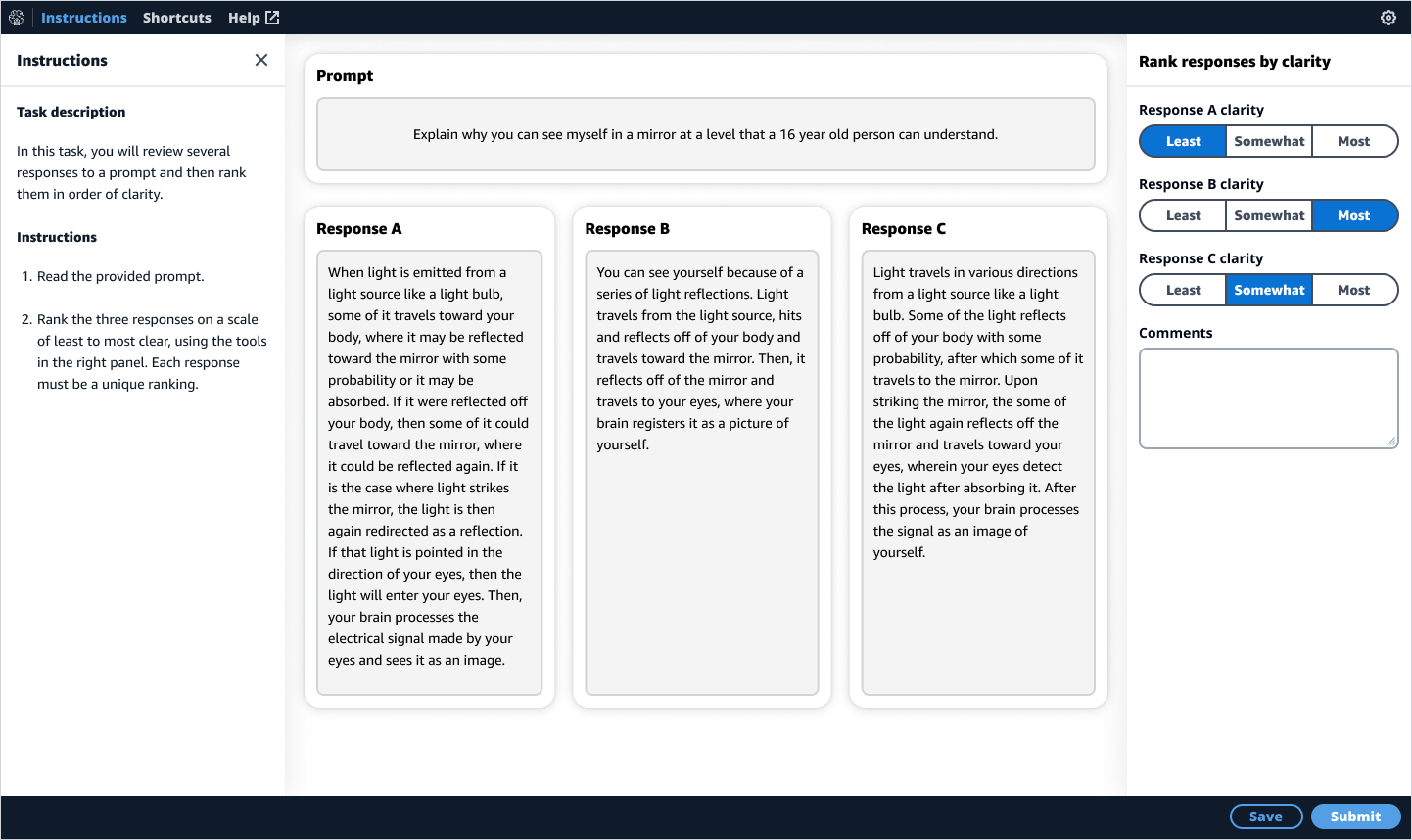
Select the model that is best suited for your use case through human evaluation
Model Evaluation
Leverage human feedback to evaluate and compare the output of models against a customizable list of criteria that are most important to you (such as accuracy, relevancy, toxicity, bias, brand voice, and style) and select the model that is best suited for your use case. AWS provides you with a variety of ways to quickly get started with model evaluation. You can leverage an AWS-managed team to evaluate, compare, and select models through SageMaker Ground Truth. You can now also access model evaluation capabilities through SageMaker Studio, SageMaker Jumpstart, and Amazon Bedrock, and empower your in-house teams to get started evaluating models in just a few clicks.
Red Teaming
Deliberately attempt to elicit harmful responses from a model and systematically review its outputs to discover vulnerabilities, improving overall safety, robustness, and reliability.
Create high quality labeled datasets for model training
Pre-built labeling templates
With SageMaker Ground Truth you can use 30+ purpose-built labeling workflows for multiple annotation use cases in images data, videos, text, and 3D point clouds.
- Image classification: Image Classification workflow allows you to categorize images against a pre-defined set of labels. Image classification is useful for scene detection models that need to consider the full context of the image. For example, we can build an image classification model
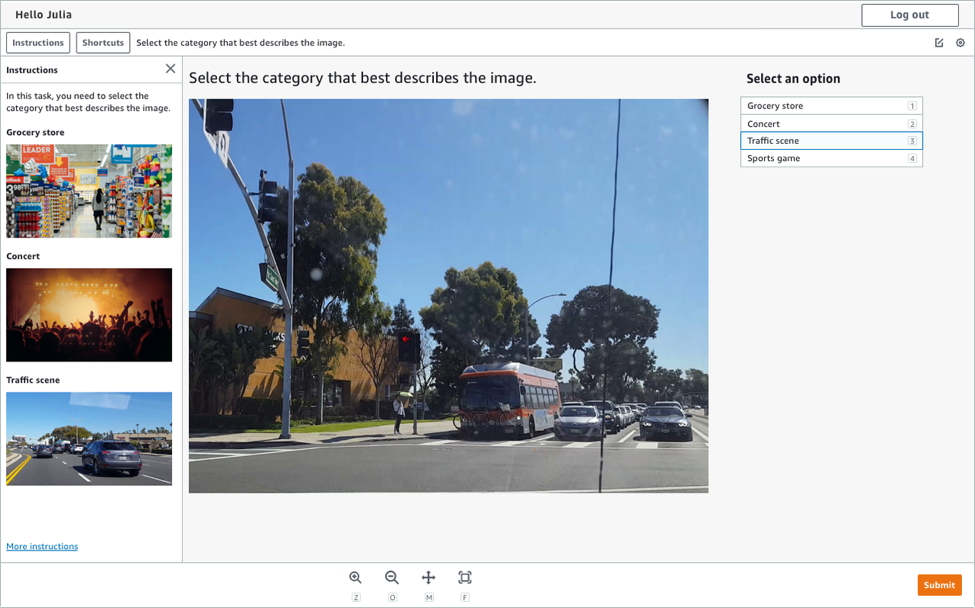
- Image Object detection: You can use the object detection workflow to identify and label objects of interest (e.g., vehicles, pedestrians, dogs, cats) in images. The labeling task involves drawing a bounding box, a two-dimensional (2D) box, around the objects of interest within an image. Computer vision models trained from images with labeled bounding boxes learn that the pixels within the box correspond to the specified object.
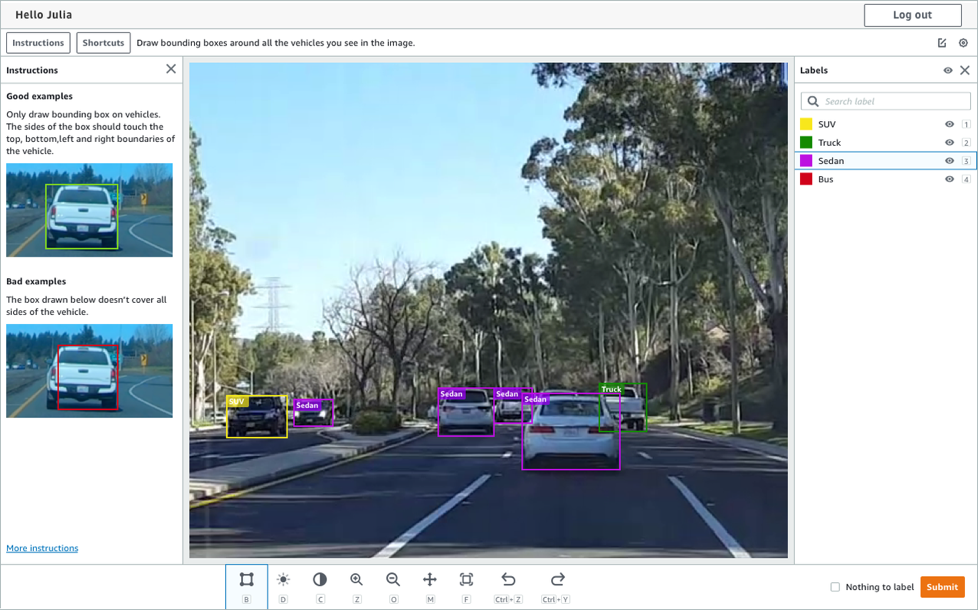
- Image Semantic segmentation: You can use the semantic segmentation workflow to label the exact parts of an image that correspond to the labels your model needs to learn. It provides high precision training data because the individual pixels are labeled. For example, the irregular shape of a car in an image could be captured exactly with semantic segmentation.
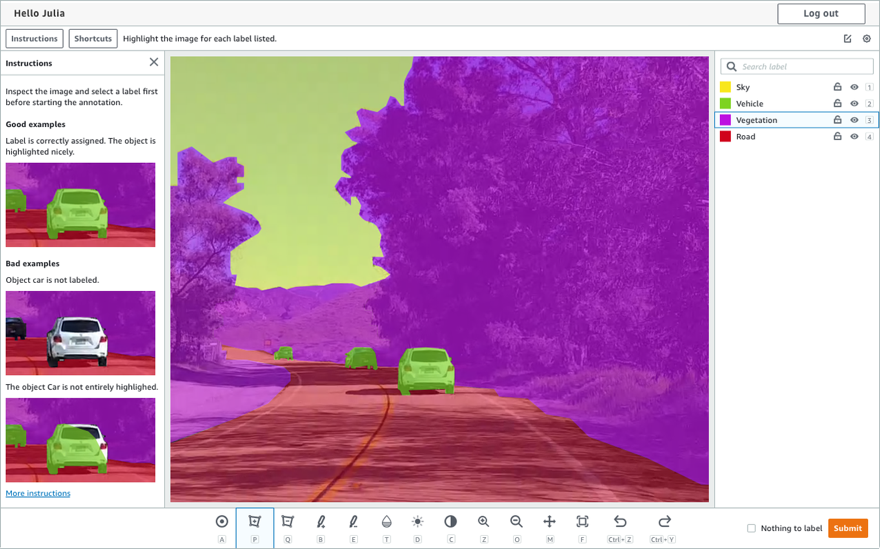
- Video object detection: The video object detection workflow allows you to identify objects of interest within a sequence of video frames. For example, in building a perception system for an autonomous vehicle, you can detect other vehicles in the scene around the vehicle.
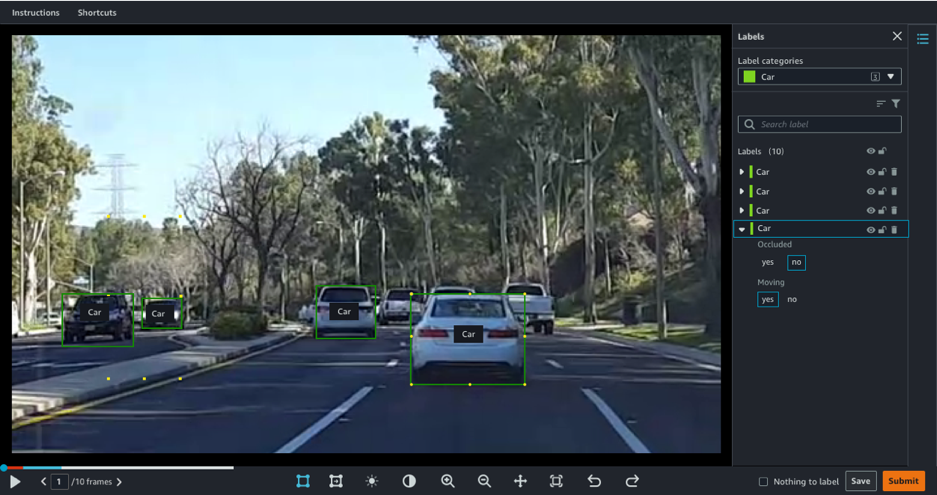
- Video object tracking: With the video object tracking workflow, you can track objects of interest across a sequence of video frames. For example, in a sports game use case, you can accurately label players across the duration of a play.
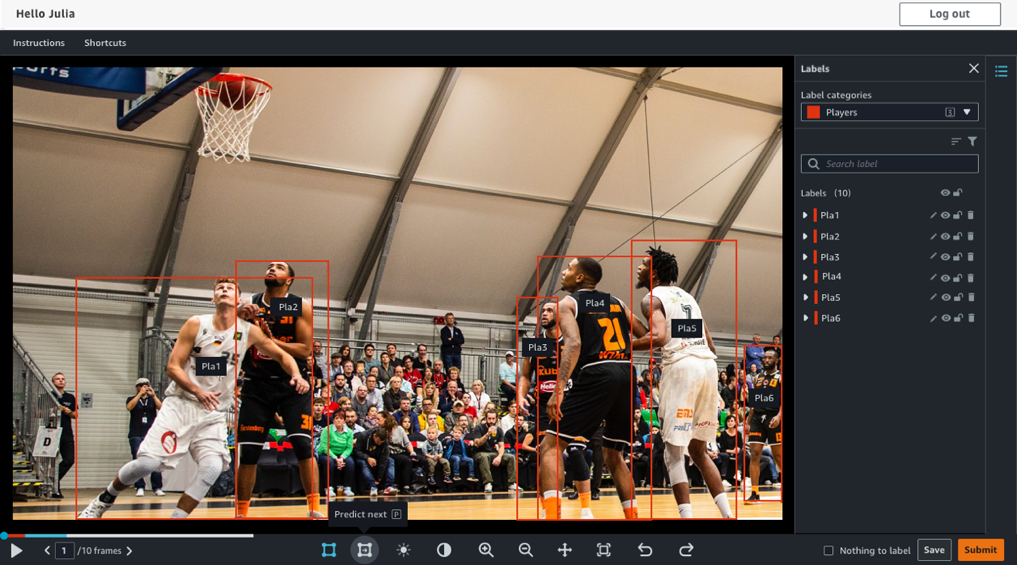
- Video clip classification: With the video clip classification workflow, you can classify a video file into a pre-specified category. For example, you can select pre-specified categories that best describe the video such as a sports play or traffic congestion at a busy intersection.
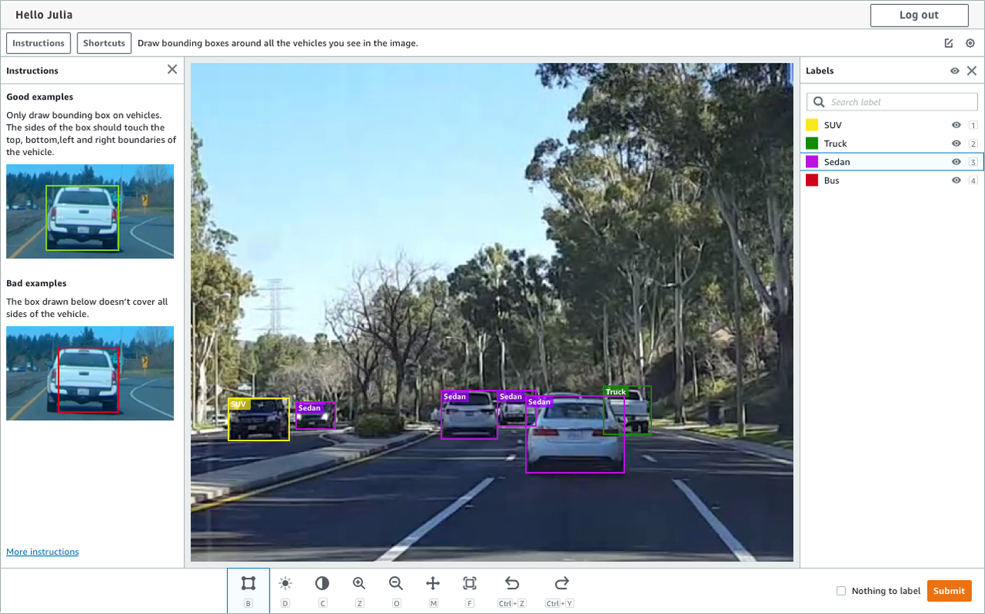
- Text classification: Text classification involves categorizing text strings against a pre-defined set of labels. It is often used for natural language processing (NLP) models that identify things like topics (e.g., product descriptions, movie reviews) or sentiment.
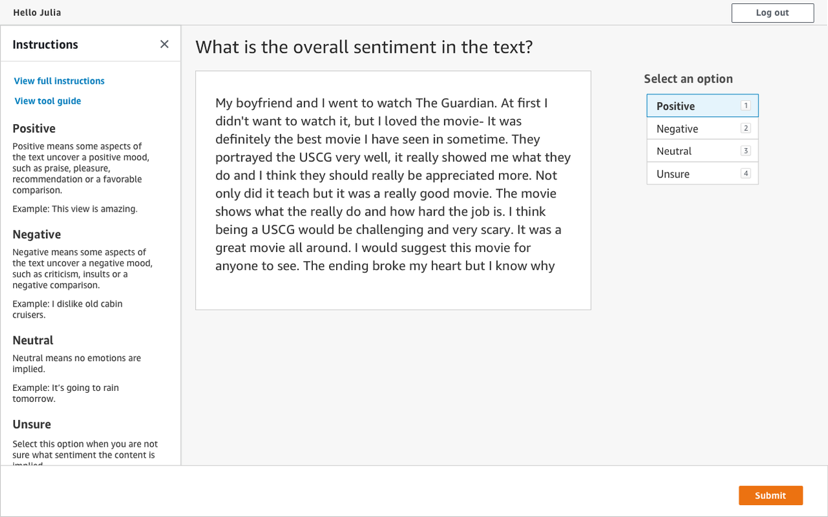
- Named Entity Recognition: Named Entity (NER) involves sifting through text data to locate phrases called named entities, and categorizing each with a label, such as “person,” “organization,” or “brand.”
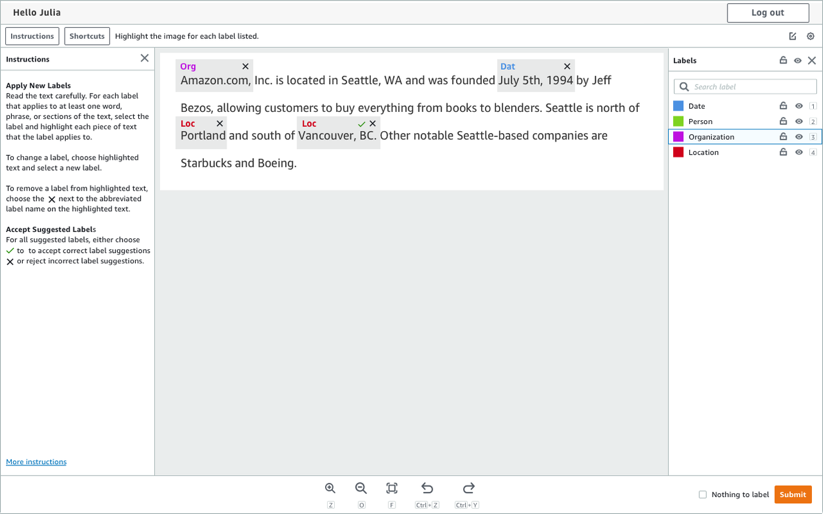
- 3D Point Cloud Object detection: With the object detection workflow, you can identify and label objects of interest within a 3D point cloud. For example, in an autonomous vehicle use case, you can accurately label vehicles, lanes, and pedestrians.
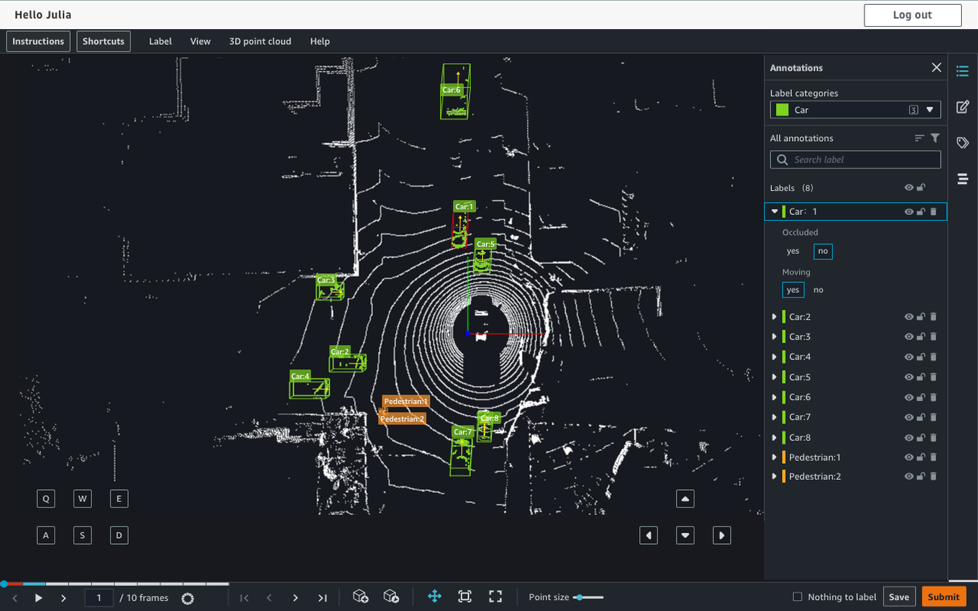
- 3D Point Cloud Object tracking: With the object tracking workflow, you can track the trajectory of objects of interest. For example, an autonomous vehicle needs to track the movement of other vehicles, lanes, and pedestrians.
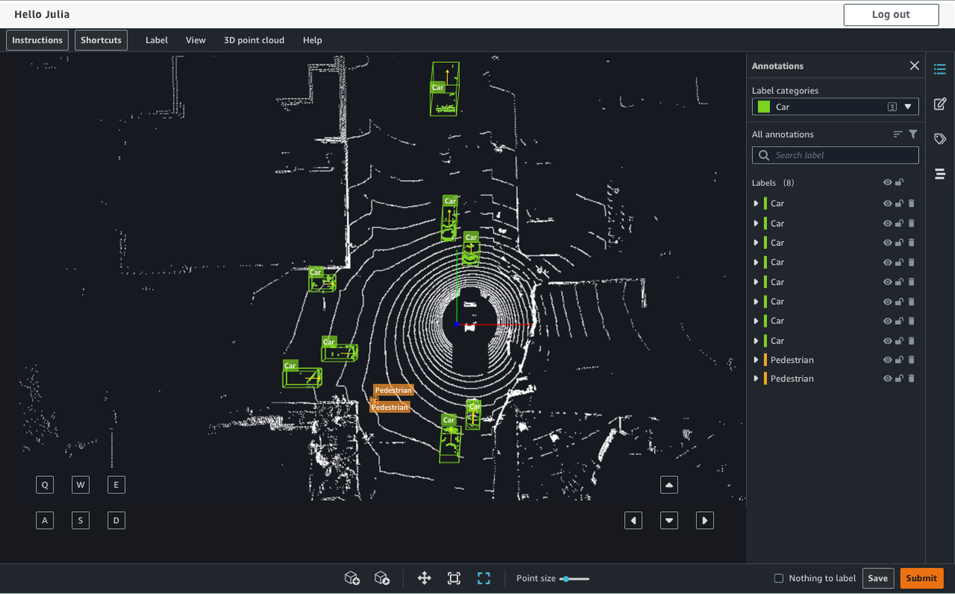
- 3D Point Cloud Semantic segmentation: With the semantic segmentation workflow, you can segment the points of a 3D point cloud into pre-specified categories. For example, for autonomous vehicles, Ground Truth could categorize the presence of streets, foliage, and structures.
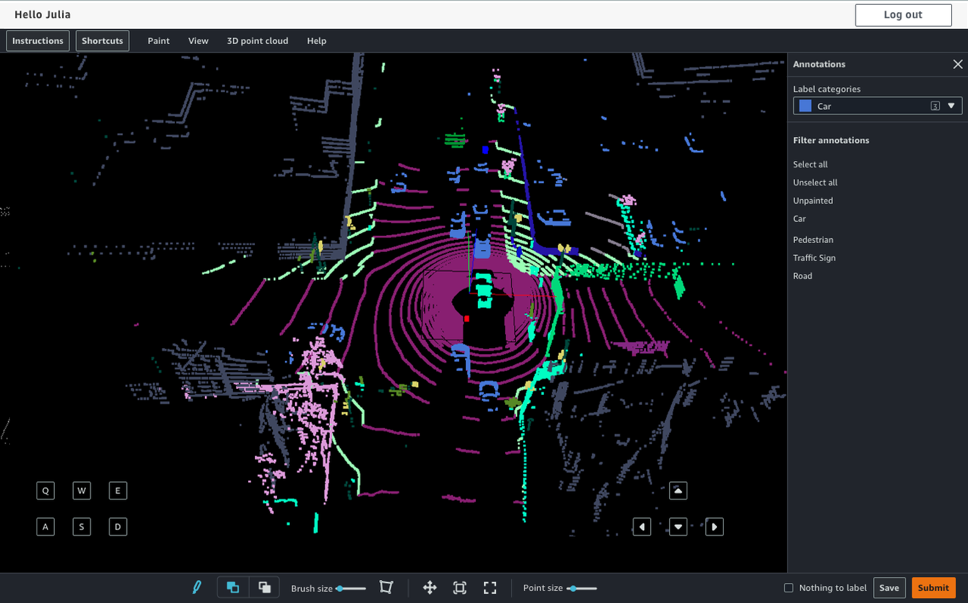
Custom workflows
SageMaker Ground Truth allows you to create your own custom labeling workflows. A workflow consists of: (1) A UI template that provides human labelers with instructions and tools to complete the labeling task. A large selection of UI templates is available or you can upload your own Javascript/HTML template. (2) Any pre-processing logic encapsulated in an AWS Lambda function. The Lambda function can serve the data to be labeled with any additional context for the labeler, and (3) Any post-processing logic encapsulated in an AWS Lambda function, to be used to add an accuracy improvement algorithm. The algorithm can assess the quality of the annotations made by the humans or can find consensus on what is “right” when the same data is provided to multiple human labelers.

Quality Assurance and Consensus
SageMaker Ground Truth allows you to validate the quality of annotation tasks by implementing quality assurance steps like setting up approval workflows, reviewing and changing annotations, routing tasks, leveraging machine validation, and tracking quality metrics. You can also create consensus witin your workflow to agree on the level of data accuracy by using algorithms for routing task reviews to multiple individuals.
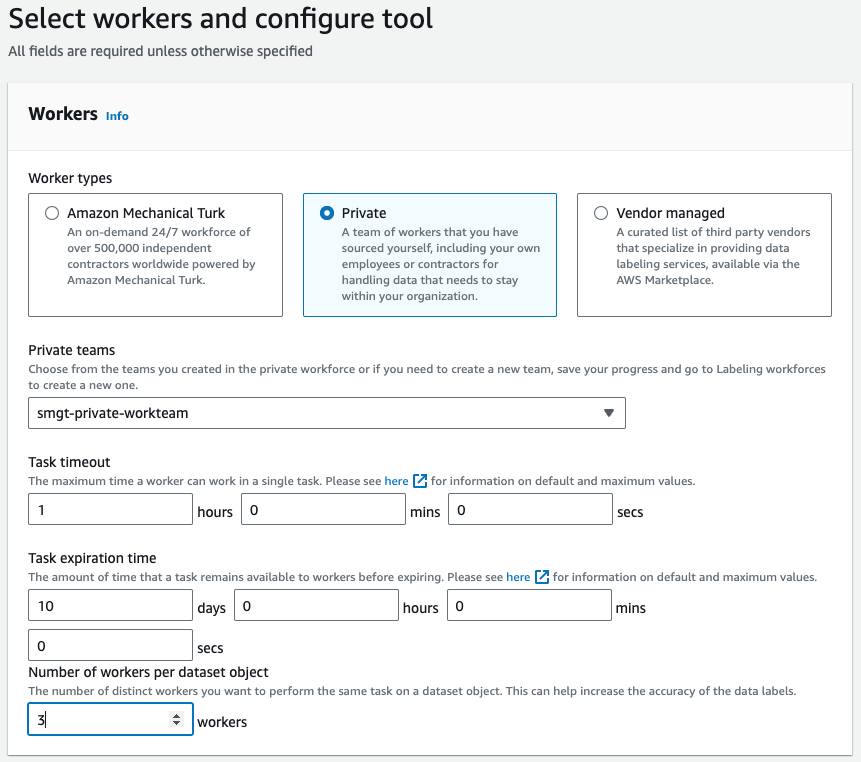
Select the workforce option that works for you
Whether you want AWS to manage a workforce on your behalf or leverage an existing internal workforce, SageMaker Ground Truth offers options and flexibility.
AWS managed workforce
SageMaker Ground Truth Plus can hire and manage a scalable, domain-expert workforce on your behalf. For example, you may require a team experienced in labeling audio files, or with specific language proficiency. For more advanced use cases, you may require a work team that can generating written content for demonstration data. AWS can recruit, hire, train and manage teams of any size for projects of varied duration, across the globe. An AWS-managed workforce can meet your security, privacy, and compliance requirements.
In-house private workforce
If you have an existing data operations team in-house, they can leverage SageMaker Ground Truth tooling and workflows to annotate data across a wide variety of use cases. This is an option if you prefer your own team’s expertise or have certain data confidentiality requirements.
Your preferred vendor
You can select a preferred annotation vendor from the AWS Marketplace to complete your tasks in SageMaker Ground Truth. This helps reduce the manual work of finding individual workers and building a team.
Crowd
Crowdsourcing your annotation work through Amazon Mechanical Turk can be a cost-effective and scalable approach to both small and large projects. You can access a large number of geographically diverse workers, quickly design and iterate on tasks, and adapt the workflow to your specific requirements.
Accelerate and automate human-in-the-loop tasks, while reducing costs
Built-in assistive tooling
Use SageMaker Ground Truth’s built-in assistive tooling to reduce the effort required to apply labels and help workers efficiently move through human-in-the-loop tasks, saving time and costs.
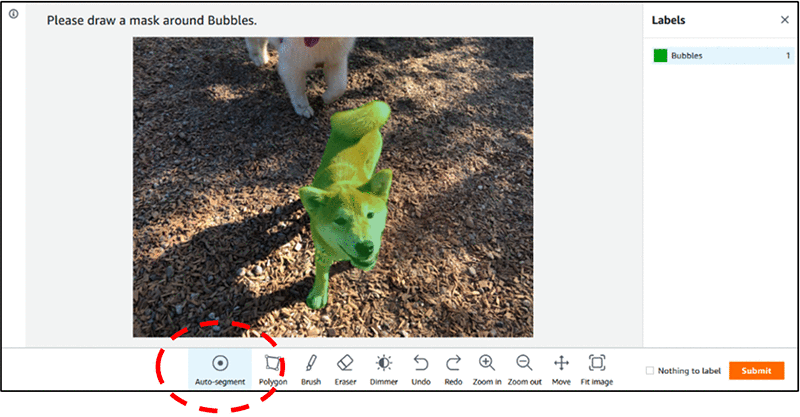
Interactive dashboards
SageMaker Ground Truth Plus provides interactive dashboards and user interfaces, so you can monitor progress of training datasets across multiple projects, track project metrics such as daily throughput, inspect labels for quality, and provide feedback on the labeled data.
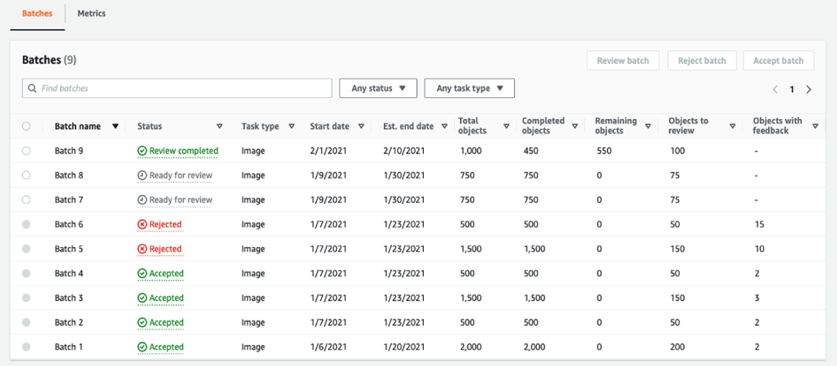