Customer Stories / Software & Internet / United States
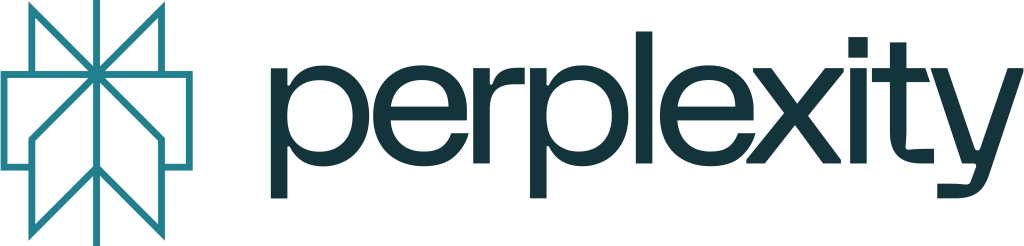
Perplexity Accelerates Foundation Model Training by 40% with Amazon SageMaker HyperPod
Learn how generative AI startup Perplexity performs model training faster and more efficiently using Amazon SageMaker HyperPod.
Up to 40% reduction
in training time
100,000+ queries per hour
supported
Maintains
low latency
Optimizes
user experience
Overview
As a transformative force, generative artificial intelligence (AI) encompasses machine learning (ML) algorithms capable of generating new content, from images to text, by learning from vast amounts of data. Perplexity, a company that is currently building one of the world’s first conversational answer engines, is using the power of generative AI to help users find relevant knowledge.
Faced with the challenge of optimizing its models for accuracy and precision, Perplexity needed a robust solution capable of handling its computational requirements. With a vision to elevate the user experience, Perplexity has turned to Amazon Web Services (AWS). By using advanced ML infrastructure, training libraries, and inference tools from AWS, Perplexity gained the flexibility, performance, and efficiency required to serve a global user base at scale.
Opportunity | Using AWS Services to Optimize User Experience
Unlike traditional search engines, which often boost ads and specific keywords over relevant results, Perplexity’s solution is optimized to connect users with the knowledge that they seek. Approximately 10 million monthly active users rely on Perplexity to learn new concepts, solve challenges, and find answers.
“Using large language models, we can capture human language understanding and reasoning capabilities into one model. That, combined with the facts on the internet, has helped us build our answer engine,” says Aravind Srinivas, CEO and cofounder of Perplexity. “Essentially, we orchestrated a traditional search index (facts engine) and a reasoning engine (large language model) together to build the world’s first conversational answer engine.”
Since its launch in 2022, Perplexity has used core AWS services such as Amazon Elastic Compute Cloud (Amazon EC2)—which provides secure and resizable compute capacity for virtually any workload—to power the backend, front end, and search components of its product. As Perplexity matured and its number of ML models grew, it needed massive compute power to serve users.
Perplexity spoke to AWS experts and learned that Amazon SageMaker HyperPod, a purpose-built infrastructure for distributed training at scale, can meet its needs for large-scale model training. Amazon SageMaker HyperPod is preconfigured with Amazon SageMaker distributed training libraries that are optimized to run highly scalable and cost-effective custom data parallel and model parallel deep learning training jobs at interconnect speeds that exceed 1,600 Gbps. Amazon SageMaker HyperPod also prevents interruptions to foundation model training by periodically saving checkpoints. When a hardware failure occurs during training, the AWS service automatically detects the failure, repairs or replaces the faulty instance, and resumes the training from the last saved checkpoint. This facilitates uninterrupted model training for weeks or months in a distributed setting.

On AWS, the power is in the hands of the customer. There are no requirements regarding which services you need to use.”
Aravind Srinivas
CEO and Cofounder, Perplexity
Solution | Reducing Model Training Time by Up to 40% with Amazon SageMaker HyperPod
AWS offered Perplexity a one-month trial to demonstrate distributed training capabilities, during which the company discovered the advantages of using AWS. For example, Perplexity gained greater flexibility in resource allocation; it uses different Amazon EC2 instance types and GPUs tailored for specific tasks.
To train ML models, Perplexity requires large amounts of memory so that it can run massive amounts of data and store different gradients. It chose Amazon EC2 P4de Instances—which provide the highest performance for ML training and high-performance computing applications—to run training jobs, achieving memory and bandwidth requirements. By using Amazon SageMaker HyperPod, Perplexity transfers data among different GPUs much faster, which has reduced ML model training time by up to 40 percent.
“Amazon SageMaker HyperPod’s built-in data and model parallel libraries helped us optimize training time on GPUs and double the training throughput,” says Srinivas. “As a result, our training experiments can now run twice as fast, which means our developers can iterate more quickly, accelerating the development of new generative AI experiences for our customers. Because Amazon SageMaker HyperPod automatically monitors cluster health and remediates GPU failures, our developers are able to focus on model building instead of spending time on managing and optimizing the underlying infrastructure.”
Perplexity aims to provide rapid and accurate responses to user queries, which requires near-real-time inference capabilities. Using Amazon EC2 P5 Instances—which deliver the highest performance GPU-based instances for deep learning applications—Perplexity can generate answers at a much higher throughput than before. In fact, the company can handle spike periods with 10,000 concurrent users and over 100,000 queries per hour without compromising latency or affecting the user experience. Perplexity also hosts the publicly available Llama 2 model on Amazon EC2 P5 Instances and uses Amazon SageMaker HyperPod to fine-tune the open-source model using its own data. Fine-tuning models helps enhance the accuracy and relevancy of responses, tailoring the model to the needs of Perplexity’s answer engine.
Outcome | Advancing Generative AI Using AWS Infrastructure and AI/ML Services
Building on its successes, Perplexity is poised to break new ground in generative AI. As part of its forward-looking strategy, the company will experiment with AWS Trainium, a high-performance ML training accelerator, to further improve training throughput. Perplexity also launched an API to provide users access to its large language models, which runs entirely on AWS and has been optimized by Amazon SageMaker HyperPod.
To expand its knowledge base and provide more accurate answers for its users, Perplexity has also adopted Amazon Bedrock, a fully managed service that offers a choice of high-performing foundation models from leading AI companies with a single API. For example, Perplexity has begun to use Claude 2 through Amazon Bedrock to incorporate advanced capabilities for coding, math, and reasoning into its service.
“On AWS, the power is in the hands of the customer,” says Srinivas. “There are no requirements regarding which services you need to use. The AWS team always tells us, ‘Do what’s best for your customers. Do what’s best for your business.’ That customer alignment is what we really love about AWS.”
About Perplexity
Perplexity is building a functional and conversational answer engine optimized to help users find knowledge rather than boost ads and keywords.
AWS Services Used
Amazon SageMaker HyperPod
AmazonSageMaker HyperPod removes the undifferentiated heavy lifting involved in building and optimizing machine learning (ML) infrastructure for training foundation models (FMs), reducing training time by up to 40%.
Amazon EC2 P5 Instances
Amazon Elastic Compute Cloud (Amazon EC2) P5 instances, powered by the latest NVIDIA H100 Tensor Core GPUs, deliver the highest performance in Amazon EC2 for deep learning (DL) and high performance computing (HPC) applications.
Amazon EC2 P4de Instances
P4de instances are powered by 8 NVIDIA A100 GPUs with 80GB high-performance HBM2e GPU memory, 2X higher than the GPUs in our current P4d instances.
Amazon Bedrock
Amazon Bedrock is a fully managed service that offers a choice of high-performing foundation models (FMs) from leading AI companies like AI21 Labs, Anthropic, Cohere, Meta, Stability AI, and Amazon via a single API, along with a broad set of capabilities you need to build generative AI applications with security, privacy, and responsible AI.
More Software and Internet Customer Stories
Get Started
Organizations of all sizes across all industries are transforming their businesses and delivering on their missions every day using AWS. Contact our experts and start your own AWS journey today.