Customer Stories / Software & Internet / Colombia
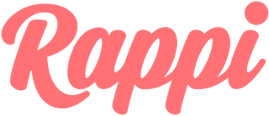
Building a Powerful Retail Personalization Engine Using Amazon Personalize with Rappi
Learn how on-demand delivery company Rappi built an advanced retail personalization engine using Amazon Personalize.
147% increase in revenue
from personalized recommendations
102% increase
in clickthrough rate
Improves
customer engagement
Decreased
cold products
Generates insights
quickly
Overview
Last-mile delivery company Rappi lets customers browse products and request delivery for almost anything, from food and clothing to insurance and financial services. Yet, this diversity posed a unique challenge—many products garnered significant attention, but this attention did not lead to purchases. To keep customers engaged and increase revenue, Rappi wanted to add a new feature to its vast digital landscape: an advanced personalization engine.
Turning to Amazon Web Services (AWS), Rappi adopted Amazon Personalize, a service that lets developers quickly build and deploy curated recommendations and intelligent user segmentation at scale using machine learning (ML). Since integrating personalized recommendations with its retail app, Rappi has seen significant improvements in both customer engagement and revenue.
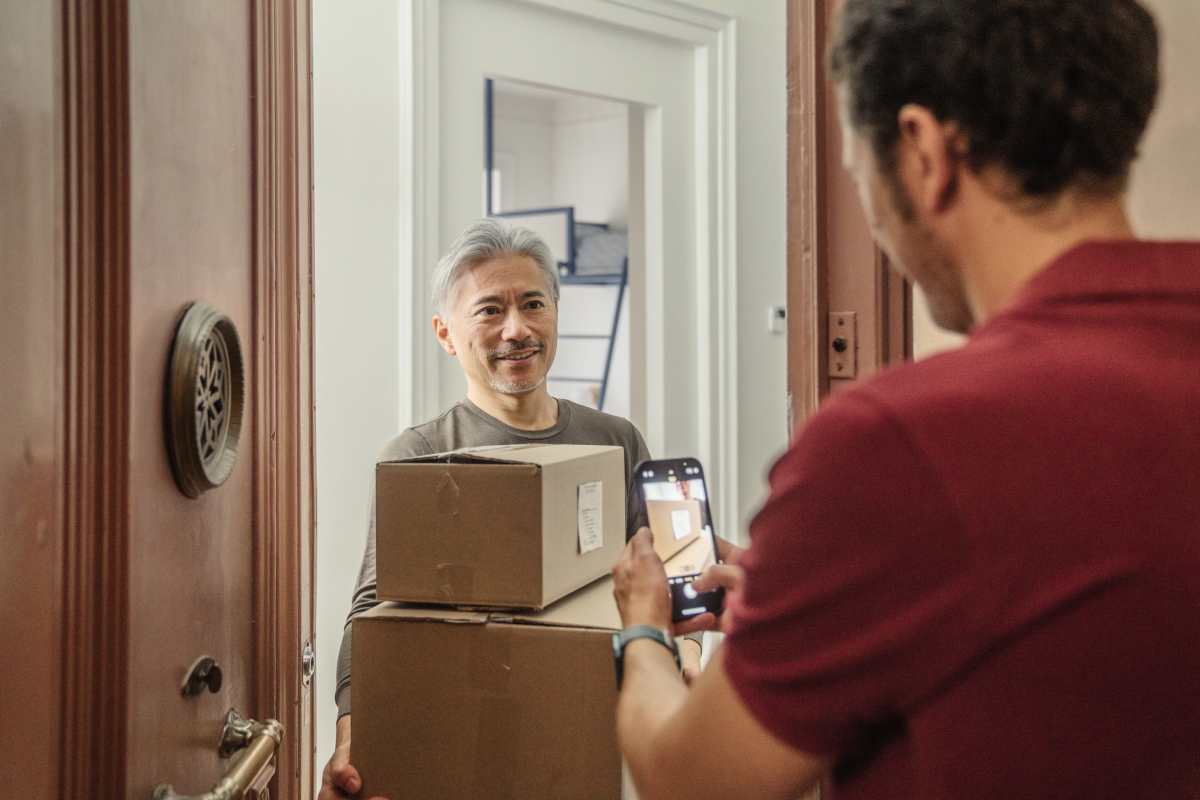
Opportunity | Using Amazon Personalize to Drive Customer Engagement for Rappi
Founded in 2015, Rappi offers on-demand, last-mile delivery services in nine Latin American countries. Its app lets customers order a wide range of items from local stores and supermarkets, including groceries, household items, personal care products, and even electronics.
The Rappi app displays different items in horizontal scroller corridors, helping users select different products by browsing through a set of cards or tiles. While these sections made browsing simple, they were not initially optimized for each user. Rappi wanted to show the right product recommendations at the right place, at the right time, for the right user—and it needed a new personalization service to accomplish this.
“Some product corridors had high views, but didn’t have high sales,” says Jose Rodrigo Hernandez, senior data scientist at Rappi. “To improve content quality, we wanted to create a new corridor that shows content, but in a personalized way. We chose Amazon Personalize as a tool to address that problem.”
In terms of project realization, Rappi’s primary focus was on rapidly developing a minimum viable product to confirm its hypothesis. This was especially crucial considering that their internal teams had minimal proficiency in data science and development.
Rappi has been all in on AWS since 2015 and is familiar with the services and support that AWS provides. Because Amazon Personalize is a fully managed service where all of the infrastructure is maintained by AWS, Rappi realized that it could focus on maximizing the value of its recommendation systems without having to manage system components. At the same time, Rappi could connect with AWS experts to identify the right Amazon Personalize features and components to meet its business needs.
“We had good support from AWS to understand the technology,” says Maximiliano Illbele, engineer manager at Rappi. “We had a multiday training session to understand how to put the first iteration into production and make a proof of concept. That was a big advantage, and one of the major reasons why we chose Amazon Personalize.”

On AWS, we can quickly deploy recommendation models and start testing and getting important insights about our products and users much faster.”
Moshe
Machine Learning Engineer, Rappi
Solution | Boosting Clickthrough Rate by 102% with Accurate Recommendations
In January 2022, Rappi began to design the first iteration of Just For You (JFY), a personalization engine that analyzes user behavior and preferences to provide product recommendations. These results are displayed in a dedicated corridor of the Rappi app.
JFY was initially launched in June 2022, and the team began running A/B tests to analyze the engine’s performance compared to a popularity-based corridor. “We launched JFY in two parts. First, we implemented an automation process that updates the ML training every month,” says Moshe Ojeda Dejo, ML engineer at Rappi. “Then, we established an API endpoint dedicated to product recommendations. In August 2022, we realized that we were outperforming the older corridor and launched a full rollout of JFY.”
After the initial deployment, Rappi saw a 20 percent increase in clickthrough rate and a 13 percent increase in customer engagement. JFY also increased revenue by 23 percent compared to the popularity-based corridor. Although the personalization provided by the service led to significant improvements, its reach was limited.
In November 2022, Rappi began to build a new solution on top of JFY: a Retail Personalization Engine (RPE). It focused on establishing a dedicated API, stronger automation, and more flexible A/B testing. By February 2022, the second iteration of JFY was launched with a fully integrated RPE, two new automation pipelines, and a dedicated, personalized API that retrieves results from both ML models.
“There are many customization options on Amazon Personalize, and we explored many of those after we had already integrated the first version of JFY into our backend,” says Moshe. “We had the chance to test and tweak different configurations to improve our results, and we got what we wanted. The metrics that we were checking to monitor the performance have improved with every iteration of the process.”
Since launching multiple iterations of JFY integrated with RPE, Rappi has seen a 102 percent increase in clickthrough rate. Engagement is 69 percent higher compared to the initial deployment version, and revenue has increased by 147 percent.
Architecture Diagram
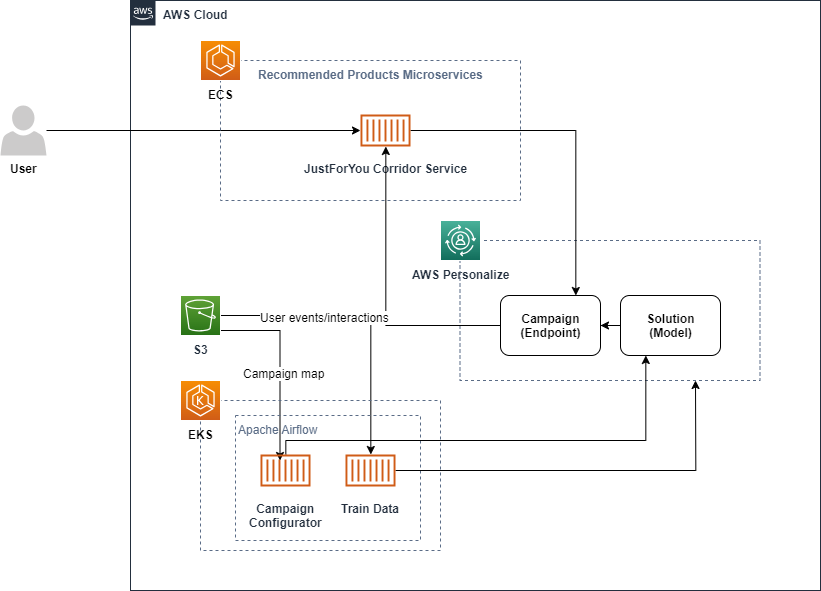
Outcome | Expanding ML Personalization Capabilities to Other Use Cases at Rappi
With the JFY corridor, Rappi customers are exploring more of its content and its catalog. The app can display new products with high accuracy, resulting in a remarkable decrease in cold products without sales or rotation. Users can see and browse more corridors, generating revenue and boosting engagement.
“Now, users can see novel content based on their activities,” says Hernandez. “Let’s say that the RPE finds out that I like sodas. Now, I can explore all branches of soda and not just the most popular brands. The solution has improved the exploration of products, helping customers find novel content that they couldn’t see before.”
The journey isn’t over yet. Rappi continues to optimize and strengthen JFY and RPE by exploring new technologies and use cases. It plans to expand these personalization capabilities to other areas of its business and help different teams achieve deeper customer engagement with tailored recommendations.
AWS makes it possible for Rappi to achieve these goals quickly and at scale. “On AWS, we can quickly deploy recommendation models and start testing and getting important insights about our products and users much faster than if we were to build an ML model from scratch,” says Moshe. “This flexibility and speed are really powerful for us, and it’s all been made possible by Amazon Personalize.”
About Rappi
Founded in 2015, Rappi offers on-demand, last-mile delivery for almost anything—from food and clothing to insurance and financial services—in nine Latin American countries.
AWS Services Used
Amazon Personalize
Amazon Personalize allows developers to quickly build and deploy curated recommendations and intelligent user segmentation at scale using machine learning (ML).
More Software & Internet Customer Stories
Get Started
Organizations of all sizes across all industries are transforming their businesses and delivering on their missions every day using AWS. Contact our experts and start your own AWS journey today.