Kinesis Data Analytics for SQL [Legacy]
AWS is no longer offering Amazon Kinesis Data Analytics for SQL applications. After careful consideration, we have made the decision to end support for Amazon Kinesis Data Analytics for SQL applications effective January 27, 2026. We have found that customers prefer Amazon Managed Service for Apache Flink offerings for real-time data stream processing workloads. Amazon Managed Service for Apache Flink is a serverless, low latency, highly scalable and available real-time stream processing service using Apache Flink, an open source engine for processing data streams. Amazon Managed Service for Apache Flink offers functionality such as native scaling, exactly-once processing semantics, multi-language support (including SQL), over 40 source and destination connectors, durable application state, and more. These features help customers build end to end streaming pipelines and ensure the accuracy and timeliness of data.
We recommend customers upgrade their existing Kinesis Data Analytics for SQL applications to either Amazon Managed Service for Apache Flink Studio or Amazon Managed Service for Apache Flink. In Amazon Managed Service for Apache Flink Studio customers create queries using SQL, Python, or Scala using interactive notebooks. For long running applications in Kinesis Data Analytics for SQL, we recommend Amazon Managed Apache Flink, where customers can create applications using Java, Python, Scala, and embedded SQL using all of Apache Flink’s APIs, connectors, and more.
Q: How do customers upgrade from Amazon Kinesis Data Analytics for SQL applications to an Amazon Managed Service for Apache Flink offering?
To upgrade to Amazon Managed Service for Apache Flink or Amazon Managed Service for Apache Flink Studio, customers will need to re-create their application. To help, we have provided a library of common SQL queries and how to re-write them in Amazon Managed Service for Apache Flink Studio. We have also provided common pattern architectures customers can follow if they are building long running applications or using machine learning in Amazon Managed Service for Apache Flink.
Customers can find migration guides in our Kinesis Data Analytics for SQL Applications documentation.
Configuring input for SQL applications
SQL applications in Kinesis Data Analytics support two types of inputs: streaming data sources and reference data sources. A streaming data source is continuously generated data that is read into your application for processing. A reference data source is static data your application uses to enrich data coming in from streaming sources. Each application can have no more than one streaming data source and no more than one reference data source. An application continuously reads and processes new data from streaming data sources, including Amazon Kinesis Data Streams or Amazon Kinesis Data Firehose. An application reads a reference data source, including Amazon S3, in its entirety for use in enriching the streaming data source through SQL JOINs.
Q: What is a reference data source?
A reference data source is static data that your application uses to enrich data coming in from streaming sources. You store reference data as an object in your S3 bucket. When the SQL application starts, Kinesis Data Analytics reads the S3 object and creates an in-application SQL table to store the reference data. Your application code can then join it with an in- application stream. You can update the data in the SQL table by calling the UpdateApplication API.
Q: How do I set up a streaming data source in my SQL application?
A streaming data source can be an Amazon Kinesis data stream or an Amazon Kinesis Data Firehose delivery stream. Your Kinesis Data Analytics SQL application continuously reads new data from streaming data sources as it arrives in real time. The data is made accessible in your SQL code through an in-application stream. An in-application stream acts like a SQL table because you can create, insert, and select from it. However, the difference is that an in- application stream is continuously updated with new data from the streaming data source.
You can use the AWS Management Console to add a streaming data source. You can learn more about sources in the Configuring Application Input section of the Kinesis Data Analytics for SQL Developer Guide.
Q: How do I set up a reference data source in my SQL application?
A reference data source can be an Amazon S3 object. Your Kinesis Data Analytics SQL application reads the S3 object in its entirety when it starts running. The data is made accessible in your SQL code through a table. The most common use case for using a reference data source is to enrich the data coming from the streaming data source using a SQL JOIN.
Using the AWS CLI, you can add a reference data source by specifying the S3 bucket, object, IAM role, and associated schema. Kinesis Data Analytics loads this data when you start the application, and reloads it each time you make any update API call.
Q: What data formats are supported for SQL applications?
SQL applications in Kinesis Data Analytics can detect the schema and automatically parses UTF-8 encoded JSON and CSV records using the DiscoverInputSchema API. This schema is applied to the data read from the stream as part of the insertion into an in-application stream.
For other UTF-8 encoded data that does not use a delimiter, uses a different delimiter than CSV, or in cases were the discovery API did not fully discover the schema, you can define a schema using the interactive schema editor or use string manipulation functions to structure your data. For more information, see Using the Schema Discovery Feature and Related Editing in the Amazon Kinesis Data Analytics for SQL Developer Guide.
Q: How is my input stream exposed to my SQL code?
Kinesis Data Analytics for SQL applies your specified schema and inserts your data into one or more in-application streams for streaming sources, and a single SQL table for reference sources. The default number of in-application streams is the one that meets the needs of most of your use cases. You should increase this number if you find that your application is not keeping up with the latest data in your source stream as defined by CloudWatch metric MillisBehindLatest. The number of in-application streams required is impacted by both the amount of throughput in your source stream and your query complexity. The parameter for specifying the number of in-application streams that are mapped to your source stream is called input parallelism.
Authoring application code for SQL applications
Q: What does my SQL application code look like?
Application code is a series of SQL statements that process input and produce output. These SQL statements operate on in-application streams and reference tables. An in-application stream is like a continuously updating table on which you can perform the SELECT and INSERT SQL operations. Your configured sources and destinations are exposed to your SQL code through in-application streams. You can also create additional in-application streams to store intermediate query results.
You can use the following pattern to work with in-application streams:
- Always use a SELECT statement in the context of an INSERT statement. When you select rows, you insert results into another in-application stream.
- Use an INSERT statement in the context of a pump.
- You use a pump to make an INSERT statement continuous, and write to an in-application stream.
CREATE OR REPLACE STREAM "DESTINATION_
For more information about application code, see Application Code in the Amazon Kinesis Data Analytics for SQL Developer Guide.
Q: How does Kinesis Data Analytics help me with writing SQL code?
Kinesis Data Analytics includes a library of analytics templates for common use cases including streaming filters, tumbling time windows, and anomaly detection. You can access these templates from the SQL editor in the AWS Management Console. After you create an application and navigate to the SQL editor, the templates are available in the upper-left corner of the console.
Q: How can I perform real-time anomaly detection in Kinesis Data Analytics?
Kinesis Data Analytics includes pre-built SQL functions for several advanced analytics including one for anomaly detection. You can simply make a call to this function from your SQL code for detecting anomalies in real-time. Kinesis Data Analytics uses the Random Cut Forest algorithm to implement anomaly detection. For more information on Random Cut Forests, see the Streaming Data Anomaly Detection whitepaper.
Configuring destinations in SQL applications
Kinesis Data Analytics for SQL supports up to three destinations per application. You can persist SQL results to Amazon S3, Amazon Redshift, and Amazon OpenSearch Service (through Amazon Kinesis Data Firehose), and Amazon Kinesis Data Streams. You can write to a destination not directly supported by Kinesis Data Analytics by sending SQL results to Amazon Kinesis Data Streams, and leveraging its integration with AWS Lambda to send to a destination of your choice.
Q: How do I set up a destination?
In your application code, you write the output of SQL statements to one or more in- application streams. Optionally, you can add an output configuration to your application to persist everything written to specific in-application streams to up to four external destinations. These external destinations can be an Amazon S3 bucket, Amazon Redshift table, Amazon OpenSearch Service domain (through Amazon Kinesis Data Firehose), and an Amazon Kinesis data stream. Each application supports up to four destinations, which can be any combination of the above. For more information, see Configuring Output Streams in the Amazon Kinesis Data Analytics for SQL Developer Guide.
Q: My preferred destination is not directly supported. How can I send SQL results to this destination?
You can use AWS Lambda to write to a destination that is not directly supported using Kinesis Data Analytics for SQL. We recommend that you write results to an Amazon Kinesis data stream, and then use AWS Lambda to read the processed results and send it to the destination of your choice. For more information, see the Example: AWS Lambda Integration in the Amazon Kinesis Data Analytics for SQL Developer Guide. Alternatively, you can use a Kinesis Data Firehose delivery stream to load the data into Amazon S3, and then trigger an AWS Lambda function to read that data and send it to the destination of your choice. For more information, see Using AWS Lambda with Amazon S3 in the AWS Lambda Developer Guide.
Q: What delivery model does Kinesis Data Analytics provide?
SQL applications in Kinesis Data Analytics uses an "at least once" delivery model for application output to the configured destinations. Kinesis Data Analytics applications take internal checkpoints, which are points in time when output records were delivered to the destinations and there was no data loss. The service uses the checkpoints as needed to ensure that your application output is delivered at least once to the configured destinations. For more information about the delivery model, see Configuring Application Output in the Amazon Kinesis Data Analytics for SQL Developer Guide.
Comparison to other stream processing solutions
The Amazon Kinesis Client Library (KCL) is a pre-built library that helps you build consumer applications for reading and processing data from an Amazon Kinesis data stream. The KCL handles complex issues such as adapting to changes in data stream volume, load balancing streaming data, coordinating distributed services, and processing data with fault-tolerance. The KCL enables you to focus on business logic while building applications.
With Kinesis Data Analytics, you can process and query real-time, streaming data. You use standard SQL to process your data streams, so you don’t have to learn any new programming languages. You just point Kinesis Data Analytics to an incoming data stream, write your SQL queries, and then specify where you want the results loaded. Kinesis Data Analytics uses the KCL to read data from streaming data sources as one part of your underlying application. The service abstracts this from you, as well as many of the more complex concepts associated with using the KCL, such as checkpointing.
If you want a fully managed solution and you want to use SQL to process the data from your data stream, you should use Kinesis Data Analytics. Use the KCL if you need to build a custom processing solution whose requirements are not met by Kinesis Data Analytics, and you are able to manage the resulting consumer application.
Service Level Agreement
Q: What does the Amazon Kinesis Data Analytics SLA guarantee?
Our Amazon Kinesis Data Analytics SLA guarantees a Monthly Uptime Percentage of at least 99.9% for Amazon Kinesis Data Analytics.
Q: How do I know if I qualify for an SLA Service Credit?
You are eligible for an SLA credit for Amazon Kinesis Data Analytics under the Amazon Kinesis Data Analytics SLA if more than one Availability Zone in which you are running a task, within the same region has a Monthly Uptime Percentage of less than 99.9% during any monthly billing cycle. For full details on all of the terms and conditions of the SLA, as well as details on how to submit a claim, please see the Amazon Kinesis SLA details page.
Get started with Amazon Kinesis Data Analytics
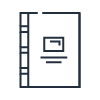
Learn how to use Amazon Kinesis Data Analytics in the step-by-step guide for SQL or Apache Flink.
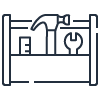
Build your first streaming application from the Amazon Kinesis Data Analytics console.