Amazon EC2 Capacity Blocks for ML
Plan your ML development with confidence by ensuring future available capacity for GPU instances.
Get low-latency, high-throughput network connectivity through colocation in Amazon EC2 UltraClusters for distributed training.
Gain predictable access to GPU instances with the highest performance in Amazon EC2 for machine learning.
With Amazon Elastic Compute Cloud (Amazon EC2) Capacity Blocks for ML, easily reserve Amazon EC2 P5 instances, powered by the latest NVIDIA H100 Tensor Core GPUs, and Amazon EC2 P4d instances, powered by NVIDIA A100 Tensor Core GPUs, for a future start date. EC2 Capacity Blocks are colocated in Amazon EC2 UltraClusters designed for high-performance machine learning (ML) workloads. You can reserve GPU instances for a duration of one to 14 days and in cluster sizes of one to 64 instances (512 GPUs), giving you the flexibility to run a broad range of ML workloads. EC2 Capacity Blocks can be reserved up to eight weeks in advance.
Use cases
Train or fine-tune ML models using GPU instances
Get uninterrupted access to the GPU instances that you reserve to complete ML model training and fine-tuning.
Get GPU instances for the amount of time you need to run your experiments
Run experiments and build prototypes that require GPU instances for short durations.
Plan for future surges in demand for ML applications
Meet your growth needs by reserving the right amount of capacity to serve your customers.
Customers and partners
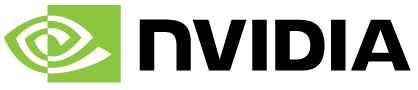
“Demand for accelerated compute is growing exponentially as enterprises around the world embrace generative AI to reshape their business. With AWS’s new EC2 Capacity Blocks for ML, the world’s AI companies can now rent H100 not just one server at a time but at a dedicated scale uniquely available on AWS—enabling them to quickly and cost-efficiently train large language models and run inference in the cloud exactly when they need it.”
Ian Buck, Vice President of Hyperscale and HPC Computing, NVIDIA

"Arcee provides an AI platform that enables the development and advancement of what we coin as SLMs—small, specialized, secure, and scalable language models. Amazon EC2 Capacity Blocks for ML are an important part of our ML compute landscape for training SLMs on AWS because they provide us with reliable access to GPU capacity when we need it. This in turn means both our internal team and our customers get to benefit from flexibility. Knowing we can get a cluster of GPUs within a couple days and without a long-term commitment has been game changing for us."
Mark McQuade, CEO and Co-Founder, Arcee
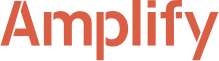
“We have partnered with several founders who leverage deep learning and large language models to bring ground-breaking innovations to market. We believe that predictable and timely access to GPU compute capacity is fundamental to enabling founders to not only quickly bring their ideas to life but also continue to iterate on their vision and deliver increasing value to their customers. Availability of up to 512 NVIDIA H100 GPUs via EC2 Capacity Blocks is a game-changer in the current supply-constrained environment, as we believe it will provide startups with the GPU compute capacity they need, when they need it, without making long-term capital commitments. We are looking forward to supporting founders building on AWS by leveraging GPU capacity blocks and its industry-leading portfolio of machine learning and generative AI services."
Mark LaRosa, Operating Partner, Amplify Partners
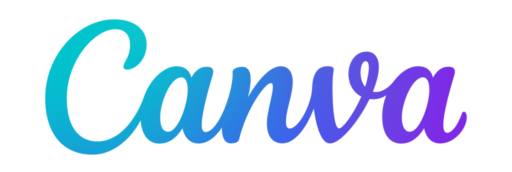
“Today, Canva empowers over 150M monthly active users to create engaging visual assets that can be published anywhere. We’ve been using EC2 P4de instances to train multi-modal models that power new Generative AI tools, allowing our users to experiment with ideas freely and quickly. As we look to train larger models, we need the ability to predictably scale hundreds of GPUs during our training runs. It’s exciting to see AWS launching EC2 Capacity Blocks with support for P5 instances. We can now get predictable access to up to 512 NVIDIA H100 GPUs in low-latency EC2 UltraClusters to train even larger models than before.”
Greg Roodt, Head of Data Platforms, Canva
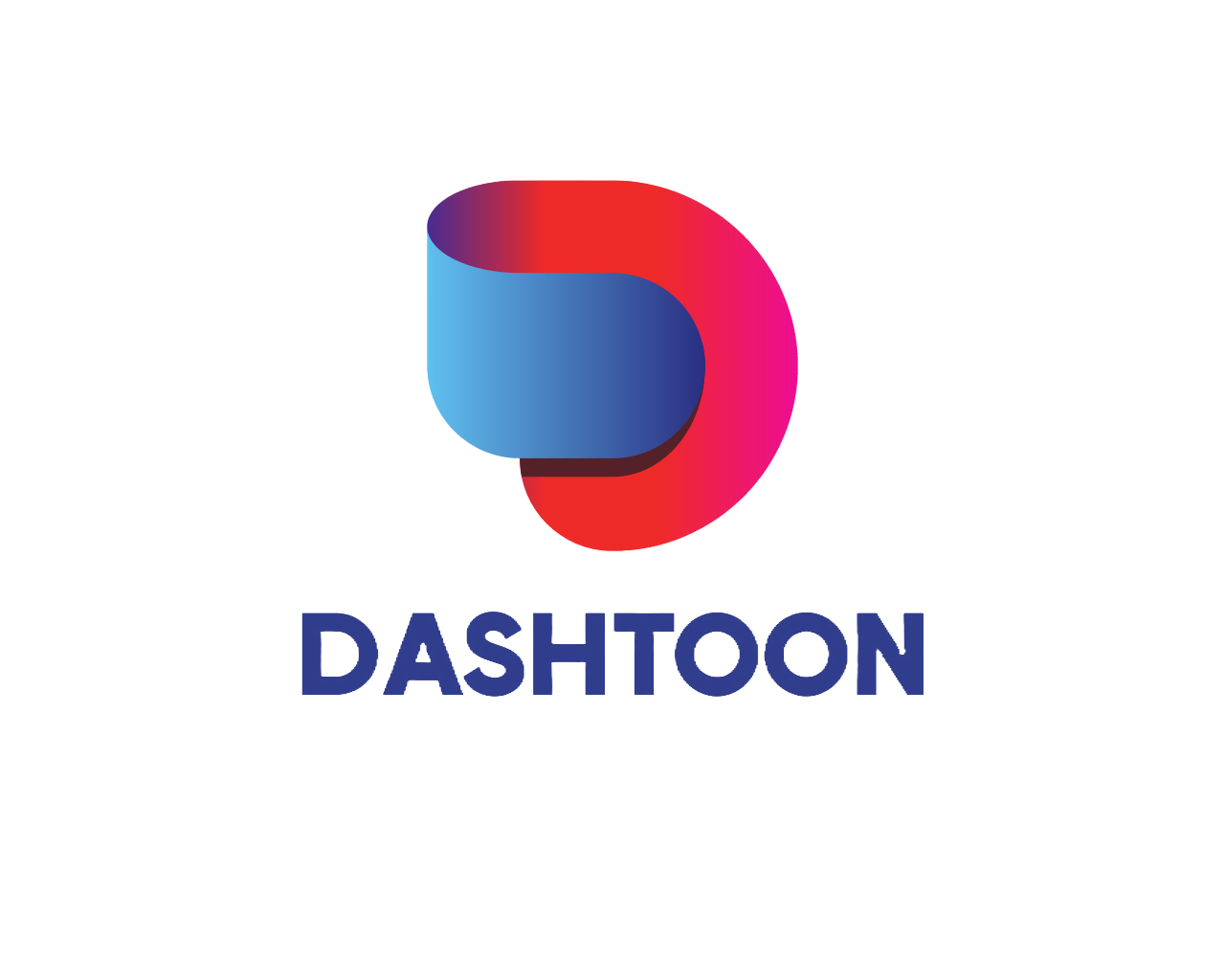
“Dashtoon blends cutting-edge AI with creativity to turn storytellers into artists who can create digital comics regardless of their artistic skills or technical knowledge, breaking traditional barriers in illustrated content creation. We have more than 80K monthly active users (MAUs) using our app to consume comics, while our creators are generating 100K+ images per day on Dashtoon Studio. We have been using AWS since inception, and we use Amazon EC2 P5 instances to train and fine-tune multi-modal models including Stable Diffusion XL, GroundingDINO, and Segment Anything. We have seen performance improve by 3x while using P5 instances, powered by NVIDIA H100 GPUs, compared to using equivalent P4d instances, powered by NVIDIA A100 GPUs. Our training data sets vary in size, and as we look to scale our model training, Amazon EC2 Capacity Blocks for ML allows us to be elastic with our GPU needs with predictable, low lead times (as soon as next-day), helping us to reduce time to release new capabilities for our users. We’re excited to continue leveraging EC2 Capacity Blocks to accelerate our innovation.”
Soumyadeep Mukherjee, Co-Founder and Chief Technology Officer, Dashtoon
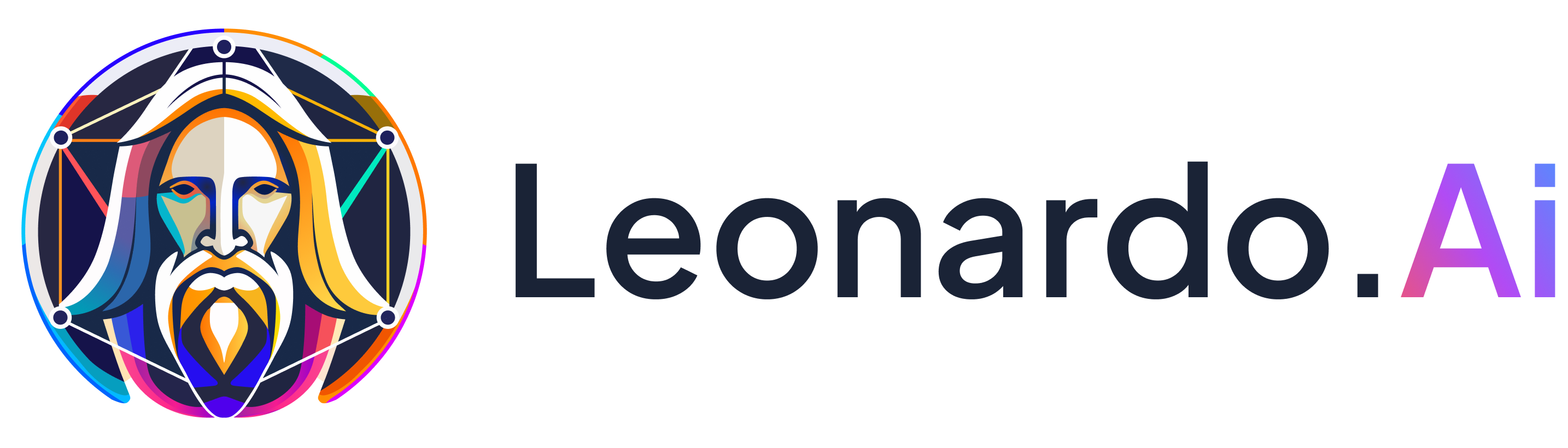
"Our team at Leonardo leverages generative AI to enable creative professionals and enthusiasts to produce visual assets with unmatched quality, speed, and style consistency. Our foundation rests upon a suite of fine-tuned AI models and powerful tooling, offering granular control both before and after hitting generate. We leverage a wide range of AWS services to not only build and train our models, but also to host them to support usage from millions of monthly active customers. We are delighted with the launch of EC2 Capacity Blocks for ML. It enables us to elastically access GPU capacity for training and experimenting while preserving the option for us to switch to different EC2 instances that might better meet our compute requirements.”
Peter Runham, CTO, Leonardo.Ai
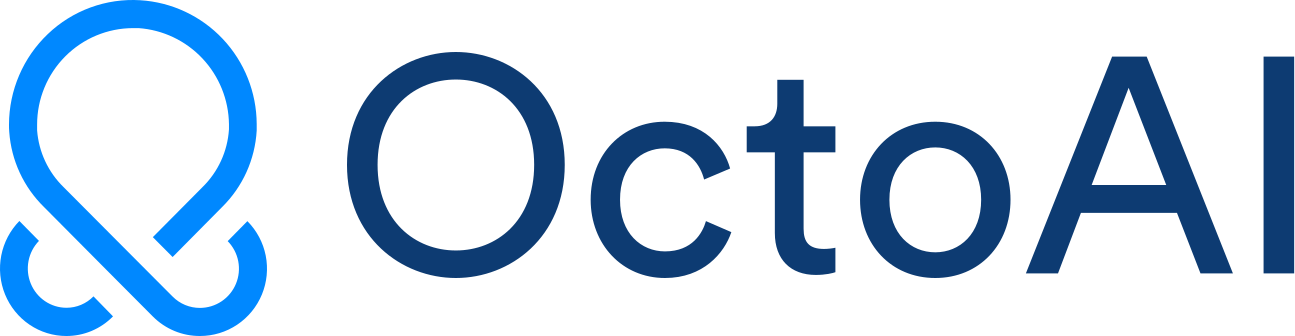
“At OctoAI, we empower application builders to easily run, tune, and scale generative AI, optimizing model execution and using automation to scale their services and reduce engineering burden. Our ability to scale up on GPU capacity for short durations is critical, especially as we work with customers seeking to quickly scale their ML applications from zero to millions of users as part of their product launches. EC2 Capacity blocks for ML enables us to predictably spin up different sizes of GPU clusters that match our customers’ planned scale-ups, while offering potential cost savings as compared to long-term capacity commits or deploying on-prem.”
Luis Ceze, CEO, OctoAI
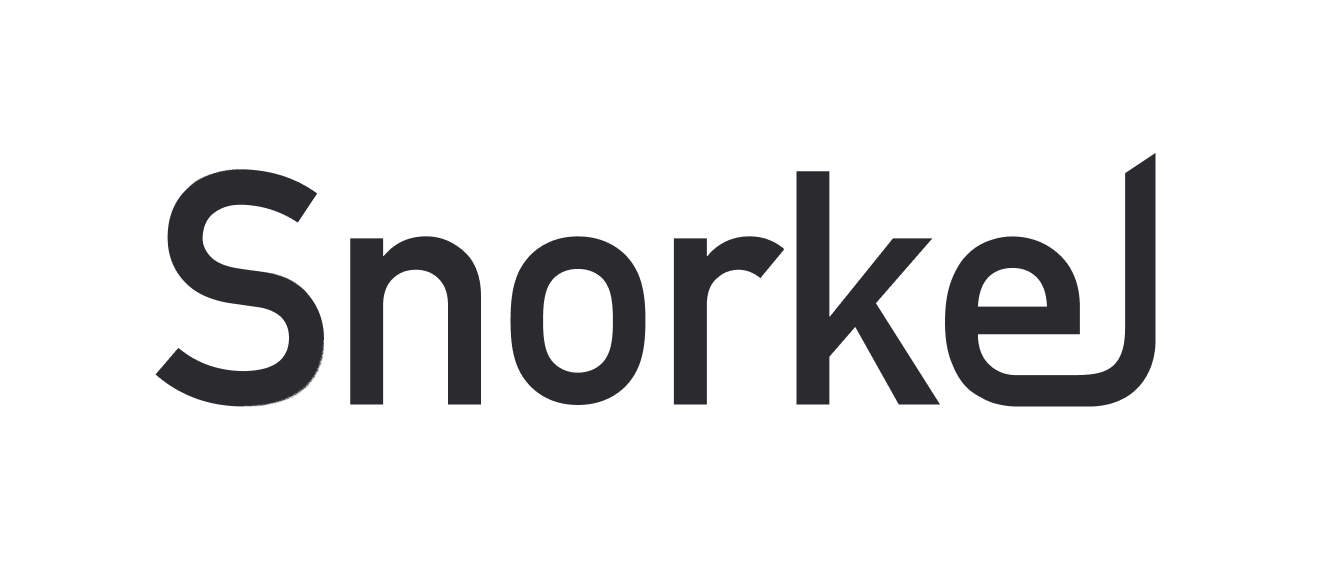
"Snorkel’s AI data development platform helps enterprises quickly create and use AI. Increasingly, that includes distilling information from compute-intensive LLMs into smaller specialist models, requiring short-term bursts of compute during development. EC2 Capacity Blocks for ML have the potential to deliver a major improvement over existing options to acquire GPU capacity. Guaranteed access to short-term GPU capacity and the high networking performance of EC2 UltraClusters are critical enablers for the AI development workflows enterprises need to support today and in the coming years."
Braden Hancock, Co-Founder and Head of Technology, Snorkel
How to get started
Discover features
Learn how to use EC2 Capacity Blocks with Amazon EC2 Auto Scaling.
Learn about pricing
EC2 Capacity Blocks prices are dynamic and change based on available supply and demand.
Explore a walk-through
See how to reserve an EC2 Capacity Block that matches your workload needs.