Amazon Redshift
Power data driven decisions with the best price-performance cloud data warehouseWhy Amazon Redshift?
Tens of thousands of customers use Amazon Redshift every day to modernize their data analytics workloads and deliver insights for their businesses. With a fully managed, AI powered, massively parallel processing (MPP) architecture, Amazon Redshift drives business decision making quickly and cost effectively. AWS’s zero-ETL approach unifies all your data for powerful analytics, near real-time use cases and AI/ML applications. Share and collaborate on data easily and securely within and across organizations, AWS regions and even 3rd party data providers, supported with leading security capabilities and fine-grained governance.
Benefits
How it works
Amazon Redshift uses SQL to analyze structured and semi-structured data across data warehouses, operational databases, and data lakes, using AWS-designed hardware and machine learning to deliver the best price performance at any scale.
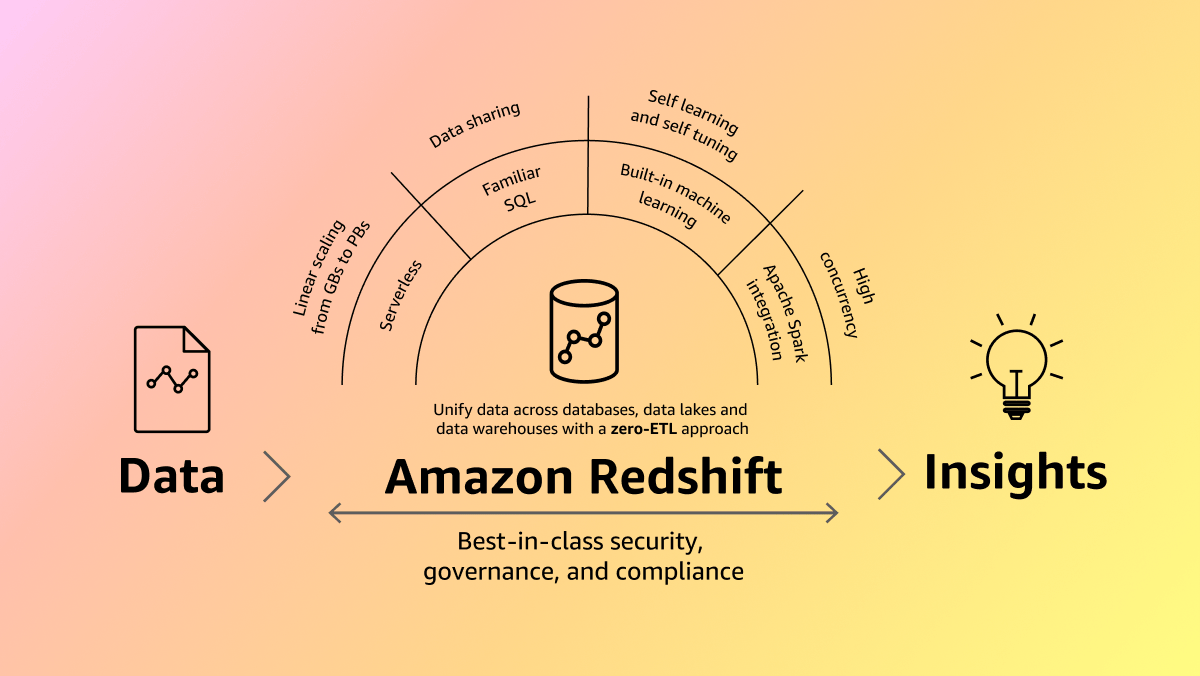
Use cases
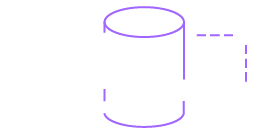
Amazon Redshift Serverless
Easily run and scale analytics in seconds without provisioning and managing a data warehouse