感谢大家阅读《生成式 AI 行业解决方案指南》系列博客,全系列分为 4 篇,将为大家系统地介绍生成式 AI 解决方案指南及其在电商、游戏、泛娱乐行业中的典型场景及应用实践。目录如下:
背景介绍
自人类诞生以来,绘画就是学习,交流和创造的重要载体。甚至在语言文字出现之前,人类就已经在用图形的方式来记录对世界的感知,交换彼此的想法。所谓一图胜千言,一幅画能够承载的信息量是非常巨大的。从古老的埃及壁画到如今以数字方式制作,存储和传输的照片,图片等,绘画内容作为信息的载体,它的创造方式都在不停的变革。从 DALL-E 开始,人类创造了一种新兴的绘画方式 – 生成式 AI 绘画,也叫做生成式 AI。 AI 绘画给人们带来了无尽的想象力,但是正如人类科技发展的曲线一般,在开始的几年里,这项新的 AI 技术始终无法被真正使用在大规模的行业生产场景中。游戏行业作为创业设计和美术场景的重度依赖行业,一直在苦苦寻找能够在游戏的生产管线中切实帮助到他们的 AI 绘画工具,以此来提高效率,降低游戏研发的成本。这种情况在去年,也就是 Stable Diffusion,MidJourney 面世之后发生了巨大的改变。随着近几个月围绕着 Stable Diffusion Web UI 所构建的开源社区的飞速发展,游戏的创意者们已经看到了将 AI 技术融入到美术生产管线的巨大潜能。如今我们可以看到,大大小小的游戏公司已经在生成式 AI 这个赛道上投入了大量的精力,并且已经取得了之前无法想象的进展。
游戏行业应用场景
正如前面所提到的,游戏行业重度依赖概念设计和美术资源,同时也是对创意和美术内容质量要求最高的行业之一。对于游戏的美术设计师来说,再精美的二维图片实际上也很难直接用于游戏中的素材生产。因为,除了设计风格之外,场景设计,角色设计都需要考虑大量的细节,比如角色姿势是否自然、细节是否清晰、光照是否合理等等都需要很多的考量。AI 可能可以带来超越人类自身想象力的一些内容,但抽卡式的创作并不能真正的提升美术管线的生产效率,我们需要使用工具来让 AI 更加精准的生成符合预期的图片。
现阶段来看,我们可以通过以下几种方式来控制 AI 绘画的生成结果。首先是文生图,就是使用文字-提示词来控制画面生成的内容。在提示词中,我们可以定义场景,物体,风格,视角等等,但是提示词作为最广泛的控制手段,它的局限性就在于对基础模型的依赖性非常强,相同的提示词在不同的基础模型上的表现可能差异巨大。其次是图生图,使用一张参考图结合提示词来让 AI 对局部进行重绘。本质上和文生图区别不大,可控性依然无法保证。 还有就是通过模型微调的方式来控制生成,常用的 Stable Diffusion 微调模型方式包括了 Text Inversion (Embedding),Hypernetworks,DreamBooth 和 LoRA,其中最流行的是 LoRA。作为一种模型微调的训练方法,LoRA 可以对基础模型的神经网络进行微小的改变,却能够产生惊人的效果。在游戏行业中, 我们发现 LoRA 已经被非常多的用来确定角色设计的风格,视角等。最后,就是我们想结合游戏行业的场景来介绍的 ControlNet。ControlNet 自今年 2 月在开源社区诞生起就是万众瞩目的焦点,因为它让 Stable Diffusion 从游戏头脑风暴阶段的辅助工具,正式进入到美术设计师的工作流中了。可以说它是 AI 绘画的一个重要的里程碑。
首先我们先了解一下 ControlNet 的原理。ControlNet 在现有模型外部叠加一个神经网络结构,通过可训练的 Encoder 副本和在副本中使用零卷积和原始网络相连,来实现在基础模型上了输入更多条件,如边缘映射、分割映射和关键点等图片作为引导,从而达到精准控制输出的内容。
原理图引用来自于 Adding Conditional Control to Text-to-Image Diffusion Models[link]
我们在可以使用插件来选择预处理器和加载 ControlNet 模型。预处理器 Preprocessor(又称 annotator),可以让我们使用现有的图片来生成需要的引导图类型。如图所示,我们可以使用一张角色三视图,然后选定 openpose_full 预处理器来得到一张角色全身多视角的 openpose 引导图,那么在后续生图的时候我们就可以使用这张引导图和 ControlNet 的 OpenPose 模型来进行更可控的创作。
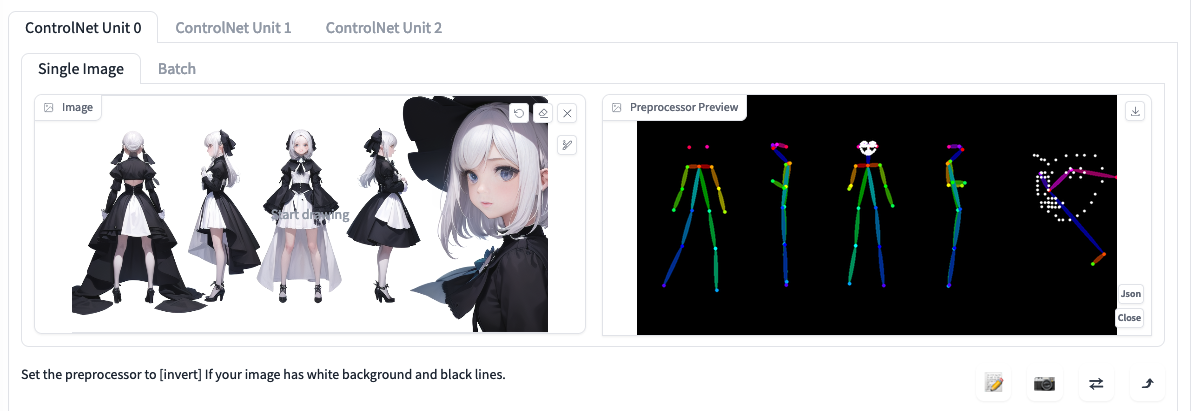
截止到目前 ControlNet 的官方模型已经从 1.0 的 8 种已经增加到了 1.1 的 14 种(11 种生产就绪和 3 种实验模型),预处理器也超过了 30 种。其中包含了多种不同的控制方式,我们可以大致做一下分类:
这里我们将结合 ControlNet 的几种模型来探索一下在游戏行业的细分场景中,如何来实现可控式 AI 生图。
概念创意和场景设计
在游戏制作中,原画师(Concept Artist )和游戏地编(Level Artist)扮演着非常重要的职责,在创作早期需要他们根据游戏策划的需求来编辑地图、地形,制作光效、奠定地图基础风格等等,以呈现更好的游戏视觉效果。在下面的这个例子中,我们使用 ControlNet 的 Segment 模型和引导图来创作游戏场景的概念设计。我们可以在 3D 编辑软件如 Blender 中,创建简单的白模图再按照 ADE20K 的颜色分类标准上色以标识构图,或者利用现有的场景图作为参考,选用 Segment 的预处理来生成 Segment 引导图。这里我们使用一张预先准备的 Segment 引导图,来生成一张概念场景。
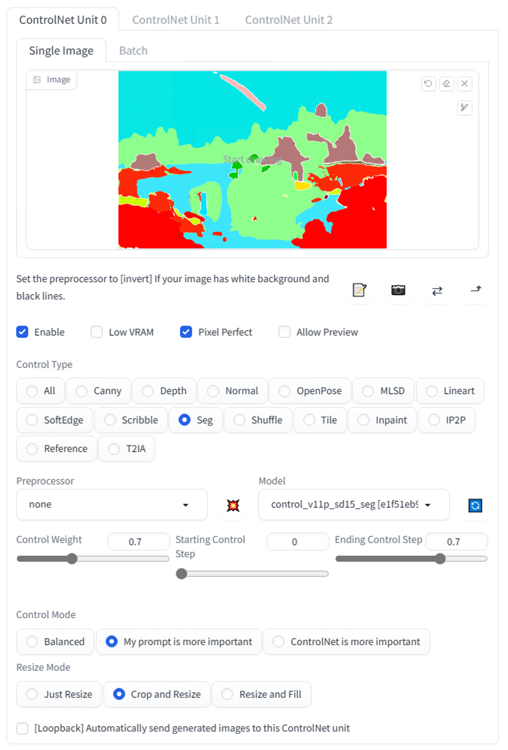
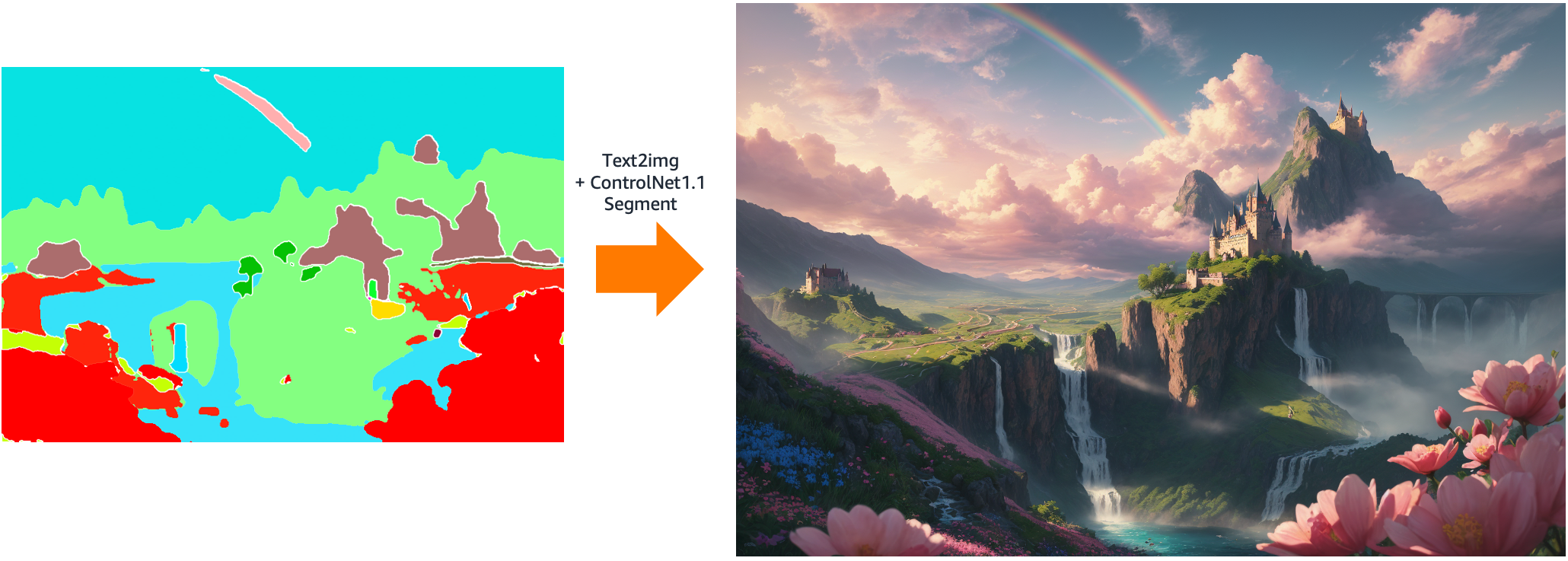
我们使用的提示词如下:
正向提示词:
(masterpiece:1.2), (best quality:1.2), (highres), ultra detailed, photorealistic, a concept painting for gaming, scenery, view from distance, no humans, cloud, waterfall, outdoors, flower, sky, mountain, water, day, pink flower, architecture, petals, castle, cloudy sky, blue sky, tree, landscape, building, (rainbow:0.9)
反向提示词:
dim, dark, abstract, unclear,repetitive, ugly, monotonous,paintings, sketches, (worst quality:1), (low quality1), (normal quality:1), lowres, normal quality, ((monochrome)), ((grayscale)), skin spots, acnes, skin blemishes, age spot, glan,nsfw, lowres, bad anatomy, text, error, extra digit, fewer digits, cropped, worst quality, low quality, normal quality, jpeg artifacts, signature, watermark, username, {{bad_construction}}, {bad_structure}, bad_wail, {bad_windows}, {blurry}, cloned_window, cropped, {deformed}, {disfigured}, error, {extra_windows}, {extra_chimney}, {extra_door}, extra_structure,extra_frame, {fewer_digits}, {fused_structure}, gross_proportions, jpeg_artifacts, {{long_roof}}, low_quality, {structure_limbs}, missing_windows, {missing_doors}, missing_roofs, mutated_structure, {mutation}, normal_quality, out_of_frame, owres, poorly_drawn_structure, poorly_drawn_house, signature, text, too_many_windows, {ugly} username,uta,watermark,worst_quality
对于游戏场景中的建筑环境,我们也可以使用 Canny 模型来产生不同的风格背景的同时保证主要物体的一致性。
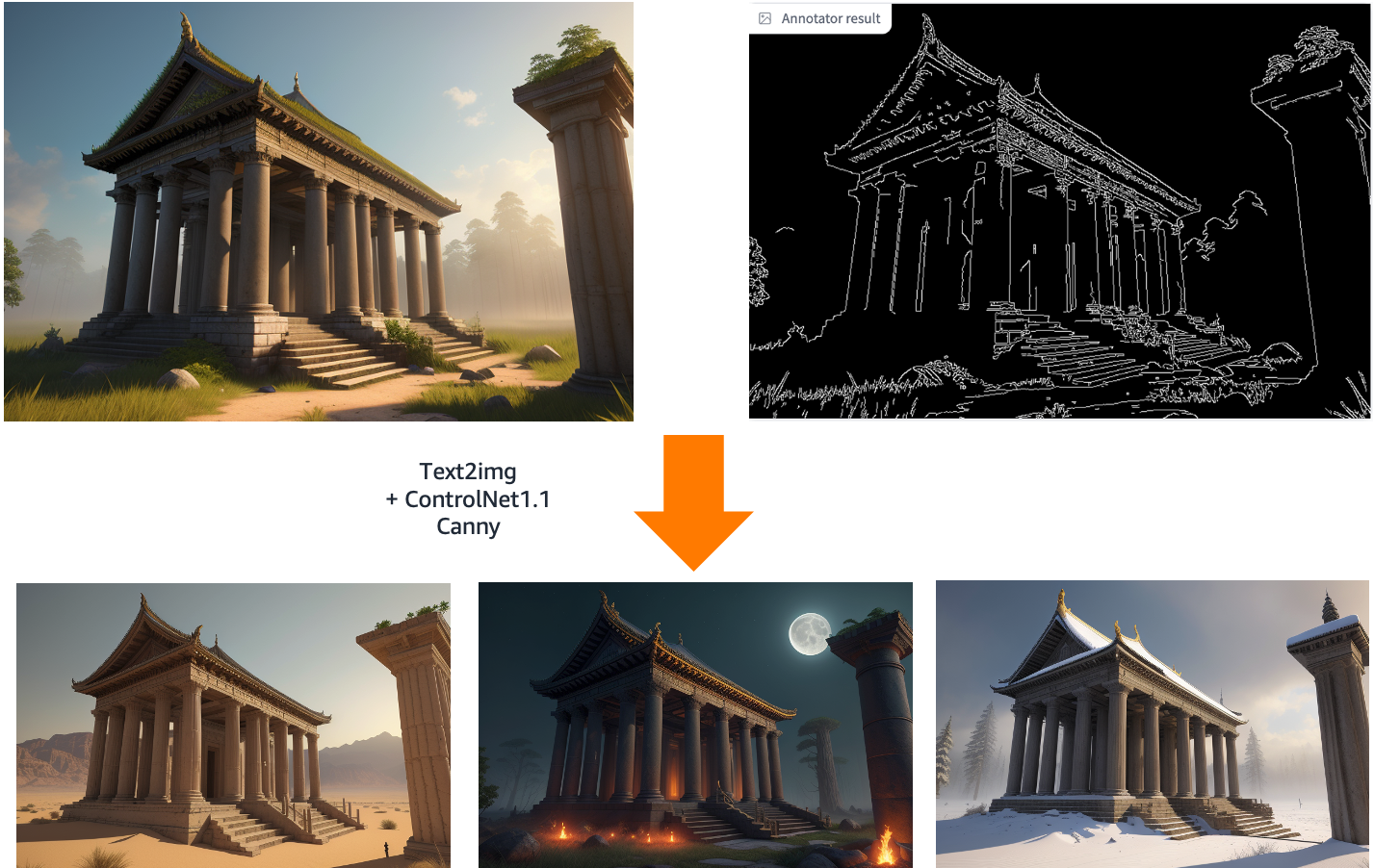
我们先使用文生图,并选定模型来生成原始概念图片。
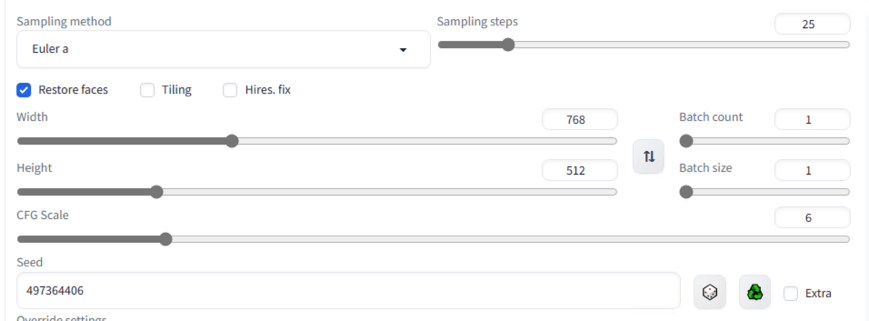
正向提示词:
(masterpiece:1.4), (best quality), (highres),<br />temple in ruines, forest, stairs, columns, cinematic, detailed, atmospheric, epic, concept art, Matte painting, mist, photo-realistic, concept art, volumetric light, cinematic epic + rule of thirds octane render, corona render, movie concept art, octane render, cinematic, trending on artstation, movie concept art, cinematic composition, ultra-detailed, realistic, hyper-realistic, volumetric lighting
反向提示词:
(EasyNegative:1.4), (lowres), (low quality), (normal quality), watermark, car, cars on the street, human
将符合概念设计的图片放入 ControlNet 并选择 canny 预处理器来生成线稿,之后就可以通过修改提示词来变换不同的场景风格而不改变图片主体。
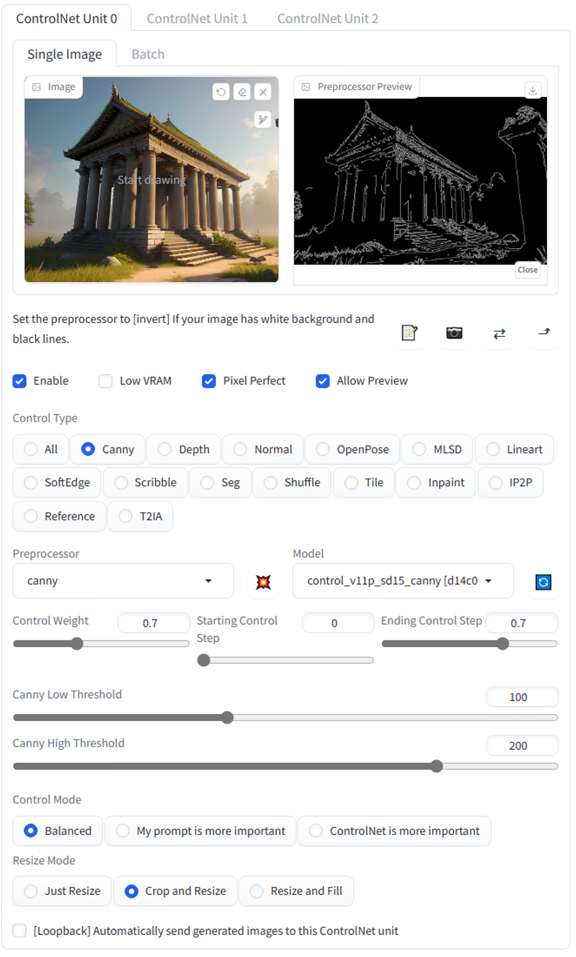
沙漠效果
正向提示词:
(masterpiece:1.4), (best quality), (highres), temple in ruines, desert, stairs, columns, cinematic, detailed, atmospheric, epic, concept art, Matte painting, mist, photo-realistic, concept art, volumetric light, cinematic epic + rule of thirds octane render, corona render, movie concept art, octane render, cinematic, trending on artstation, movie concept art, cinematic composition, ultra-detailed, realistic, hyper-realistic,
反向提示词:
(EasyNegative:1.4), (lowres), (low quality), (normal quality), watermark, car, cars on the street, human, forest, cloud,
暗夜效果
正向提示词:
(masterpiece:1.4), (best quality), (highres), temple in ruines,(midnight bliss), (moon:1.2), (star \(sky\)), (dark at night), torch, forest, stairs, columns, cinematic, detailed, atmospheric, epic, concept art, Matte painting, mist, photo-realistic, concept art, volumetric light, cinematic epic + rule of thirds octane render, corona render, movie concept art, octane render, cinematic, trending on artstation, movie concept art, cinematic composition, ultra-detailed, realistic, hyper-realistic,
反向提示词:
(EasyNegative:1.4), (lowres), (low quality), (normal quality), watermark, car, cars on the street, human, sunlight,
雪地效果
正向提示词:
(masterpiece:1.4), (best quality), (highres), temple in ruines, forest, winter, snow, stairs, columns, cinematic, detailed, atmospheric, epic, concept art, Matte painting, mist, photo-realistic, concept art, volumetric light, cinematic epic + rule of thirds octane render, corona render, movie concept art, octane render, cinematic, trending on artstation, movie concept art, cinematic composition, ultra-detailed, realistic, hyper-realistic
反向提示词:
(EasyNegative:1.4), (lowres), (low quality), (normal quality), watermark, car, cars on the street, human, sunlight
游戏皮肤道具和资产
在游戏的制作当中,游戏内数量庞大的物品设计是非常耗时且费力的部分,装备、皮肤、道具、药剂等物品可能数以千计,美术团队从概念设计到最终放到游戏里的资源,可能耗费很长时间和大量的预算。我们在这里尝试使用 lineart_anime 来提取动漫人物线稿来创建不同的人物套装。
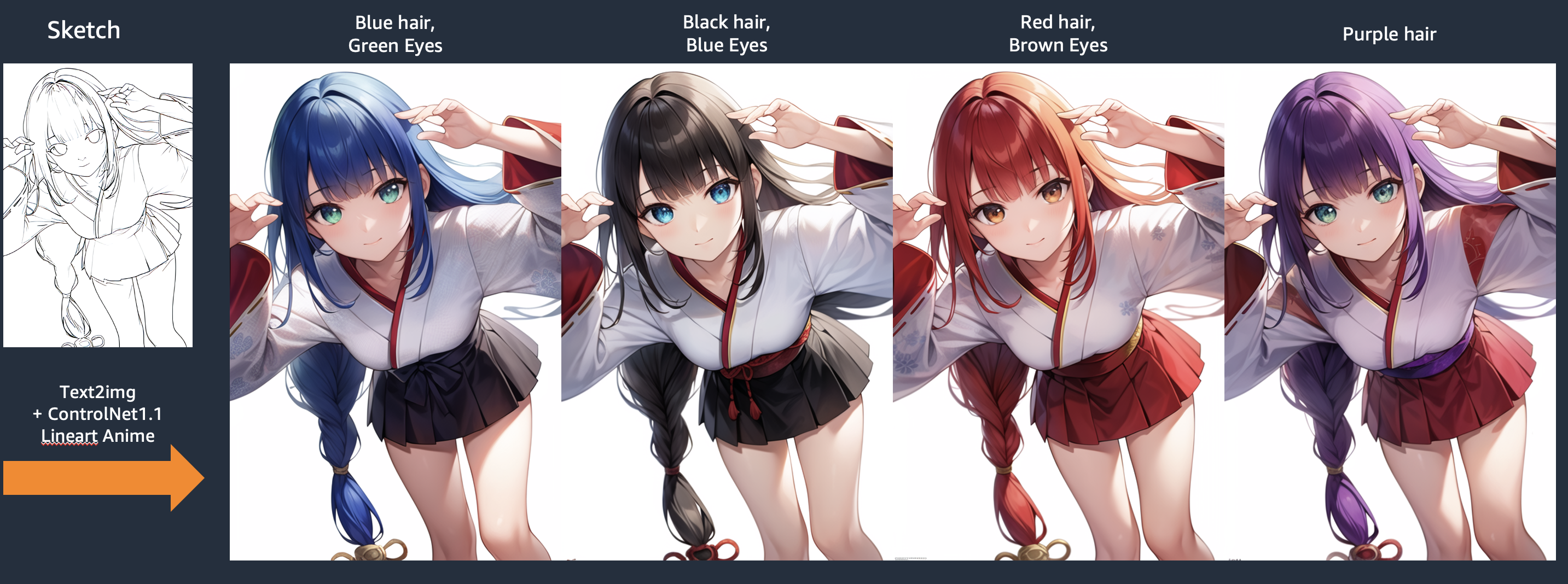
我们还是先选定自己的基础模型,通过提示词来生成原始概念图片。
正向提示词:
(masterpiece),(best quality:1.0), (ultra highres:1.0), (bent over), detailed clothes, blunt bangs, braid, wide-sleeved kimono, hair ornament, white japanese clothes, (red obi:1.4), (purple hair:1.4), very long hair, straight hair, detailed face, cool face, (smooth chin:0.85), closed mouth, looking at viewer, beautiful eyes, detailed eyes, (ulzzang-6500:0.7), skirt, (from below:1.1), photon mapping, physically-based rendering, RAW photo, clear background, (white background:1.4), (photo realistic:1.35), high res, perspective
反向提示词:
(sexy:1.4), 3d, sepia, painting, cartoons, sketch, (worst quality:2), (low quality:2), (normal quality:2), lowres, bad anatomy, bad hands, normal quality, ((monochrome)), ((grayscale)), futanari, full-package_futanari, newhalf, nipplepierces, collapsed eyeshadow, multiple eyeblows, pink hair, (nsfw:1.4)
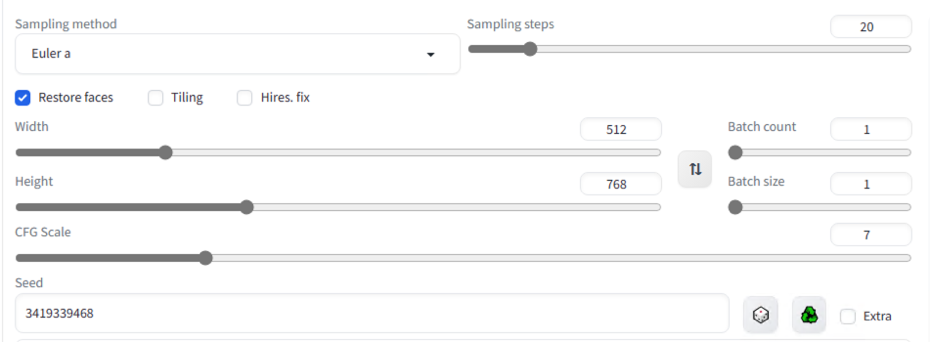
然后使用 lineart_anime 的预处理加上 lineart_anime 的模型,我们就可以调整提示词中和人物特征相关的部分来生成示例中的不同套装。
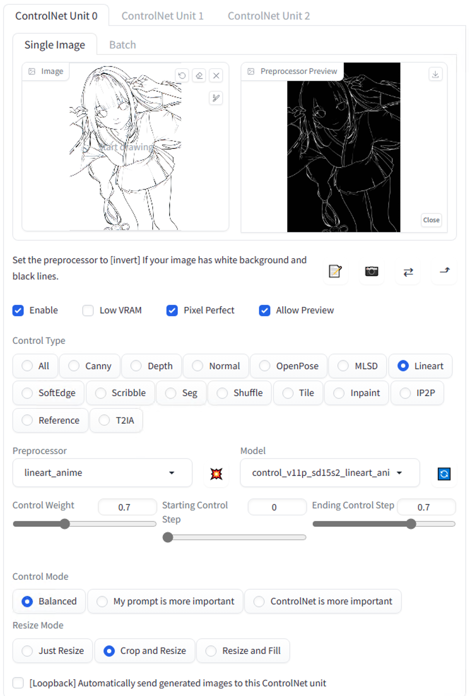
角色设计三视图
游戏原画具体到一个角色的设计 ,一般会以三视图的方式来交给建模师。因为最终角色会以三维形式来表现细节。三视图包含的正面图,背面图,侧面图展示让建模师能够快速理解原画师的设计意图。通过 OpenPose 编辑器插件或者其他的图片编辑工具,我们可以绘制 3-4 张人物造型引导图,需要注意的是长宽和最终出图的像素要保持比例一致,然后通过 ControlNet 的 OpenPose 模型再结合提示词以及特定的模型,就可以生成效果还不错的人物角色三视图。
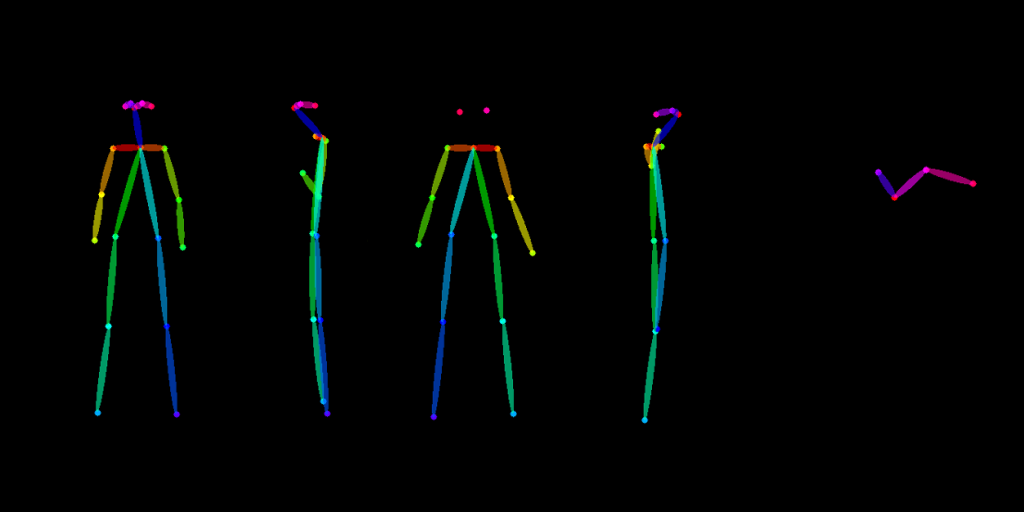
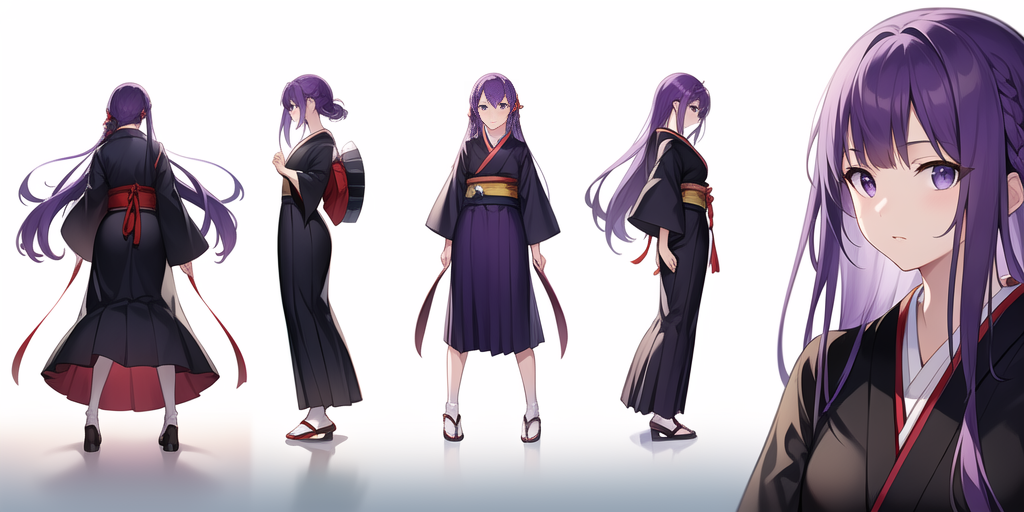
正向提示词:
(masterpiece),(best quality:1.0), (ultra highres:1.0), (bent over), full body, detailed clothes, blunt bangs, braid, wide-sleeved kimono, hair ornament, white japanese clothes, (red obi:1.4), (purple hair:1.4), very long hair, straight hair, detailed face, cool face, (smooth chin:0.85), closed mouth, looking at viewer, beautiful eyes, detailed eyes, (ulzzang-6500:0.7), (long skirt:1.4), (from below:1.1), photon mapping, physically-based rendering, RAW photo, clear background, (white background:1.4),(photo realistic:1.35),high res,perspective,(((full body))), multiple views, <lora:charturnerbetaLora_charturnbetalora:0.1>
反向提示词:
(sexy:1.4), 3d, sepia, painting, cartoons, sketch, (worst quality:2), (low quality:2), (normal quality:2), lowres, bad anatomy, bad hands, normal quality, ((monochrome)), ((grayscale)), futanari, full-package_futanari, newhalf, collapsed eyeshadow, multiple eyeblows, pink hair, (nsfw:1.4)
架构与工作原理
本篇以生成式 AI 行业解决方案指南为基础,其解决方案的工作原理如下图:
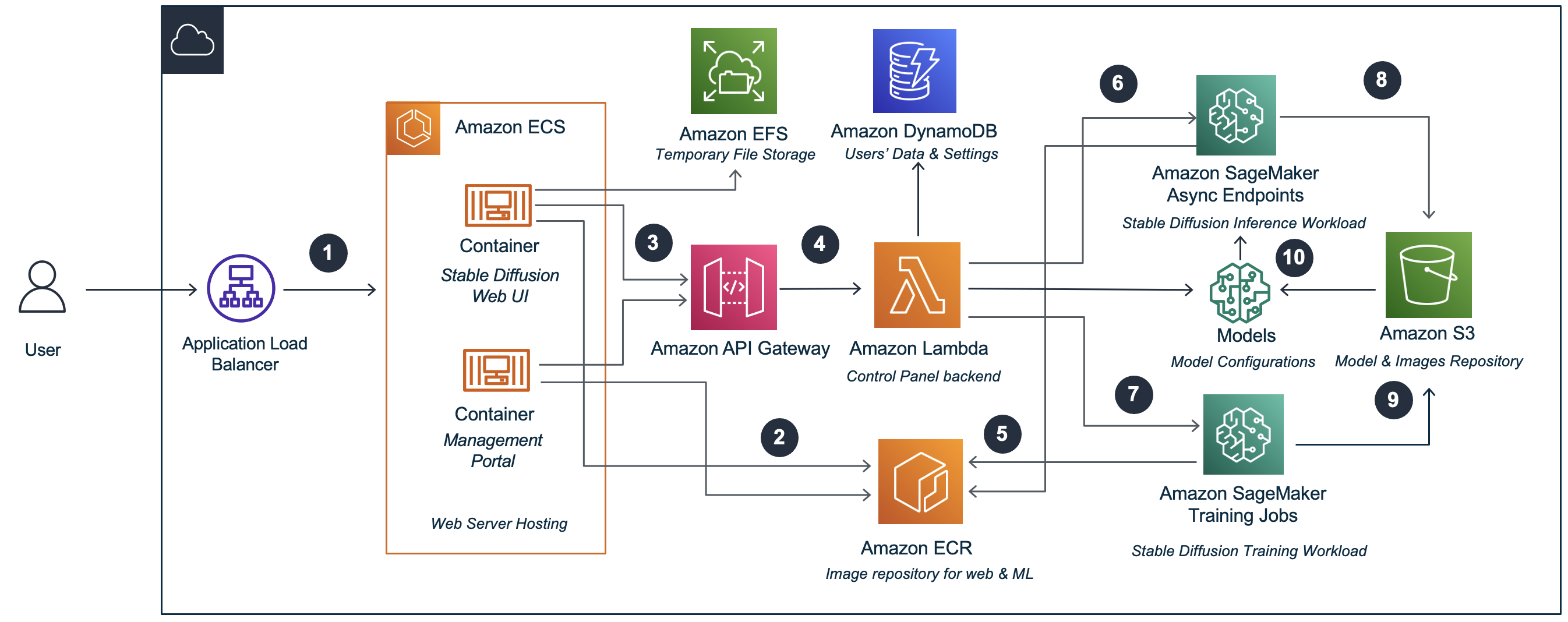
生成式 AI 行业解决方案指南架构图
生成式 AI 行业解决方案指南,将前端 Stable Diffusion WebUI 部署在容器服务 Amazon ECS 上,后端使用无服务器服务 Amazon Lambda 进行处理,前后端通过 Amazon API Gateway 调用进行通信。模型训练及部署均通过 Amazon SageMaker 进行。同时使用 Amazon S3、Amazon EFS、Amazon DynamoDB 分别进行模型数据、临时文件、使用数据的存储。详情请见系列博客的第一篇《生成式 AI 行业解决方案指南与部署指南》。
快速部署流程
本行业解决方案指南可使用 CloudFormation 一键部署。如需使用生成式 AI 行业解决方案指南,可参考系列博客的第一篇《生成式 AI 行业解决方案指南与部署指南》,本篇不做重点介绍。
小结
在本文中,我们大致介绍了在游戏行业中几个场景中,如何使用亚马逊云科技的生成式 AI 行业解决方案指南以及 ControlNet 来高效率的生成可控性高的图片素材。希望大家使用亚马逊云科技的生成式 AI 行业解决方案指南多多练习 AI 绘画的技巧,并让它成为您创意辅助和提升效率的利器。
参考资料
1. 生成式 AI 行业解决方案指南 Workshop:https://catalog.us-east-1.prod.workshops.aws/workshops/bae25a1f-1a1d-4f3e-996e-6402a9ab8faa
2. Stable-diffusion-webui:https://github.com/AUTOMATIC1111/stable-diffusion-webui
3. Hugging Face:https://huggingface.co/
4. https://arxiv.org/abs/2302.05543
5. https://github.com/lllyasviel/ControlNet
6. https://github.com/Mikubill/sd-webui-controlnet
7. https://huggingface.co/blog/train-your-controlnet
本篇作者