Amazon Personalize是 AWS 完全托管的服务。Amazon Personalize 将Amazon.com 二十多年机器学习的应用经验集成到服务当中,并且可以根据用户数据进一步定制化的调整模型。不需要任何ML经验,您就可以开始使用简单的API,通过几次点击就可以构建复杂的个性化推荐功能。
在本文中,将向您展示如何使用Amazon Personalize构建自动训练和推理的推荐服务。文中采用MovieLens电影评分数据作为样本数据并将数据存储在S3中,文中将利用Lambda函数触发数据更新,模型训练,模型更新和模型批量推理。
推荐服务架构
- 应用推送用户数据,电影数据,用户评分数据,推理用户列表数据,推理结果数据到相应的S3桶
- 将全量数据按照定义的格式从S3导入Amazon Personalize中
- Lambda定时触发模型训练任务
- 应用推送增量数据到S3桶中,Lambda函数触发数据更新任务和模型更新任务
- 应用推送推理用户列表数据到S3桶,Lambda函数触发模型推理任务,推理结果文件写入S3桶中
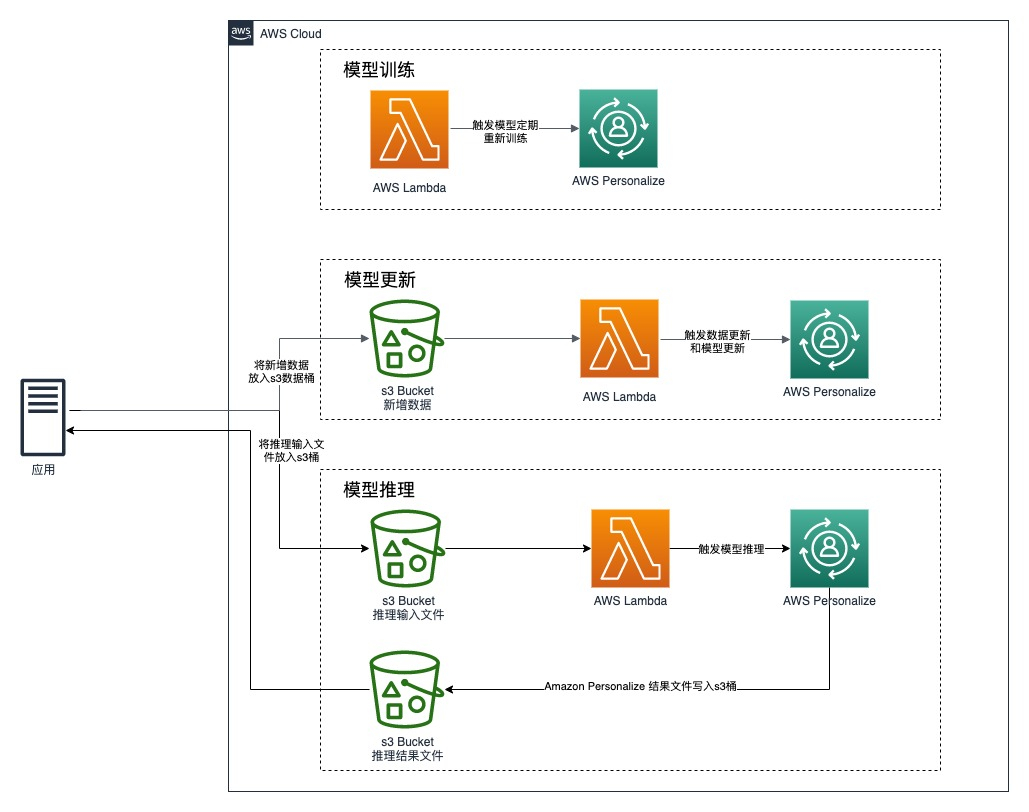
权限设置
在 IAM 中创建 Role 用来 Amazon Personalize 数据导入,数据更新,模型训练,模型更新,模型推理
进入 AWS 控制台中,创建 Personalize 的 service role。将 AmazonPersonalizeFullAccess 权限赋予该 role,取名 PersonalizeRole。我们还需要 PersonalizeRole 能够访问相应的 S3 桶,所以我们要赋予相应的桶访问权限。
为 PersonalizeRole 添加 S3 访问策略:
回到 IAM 首页,点击左侧 Policy。
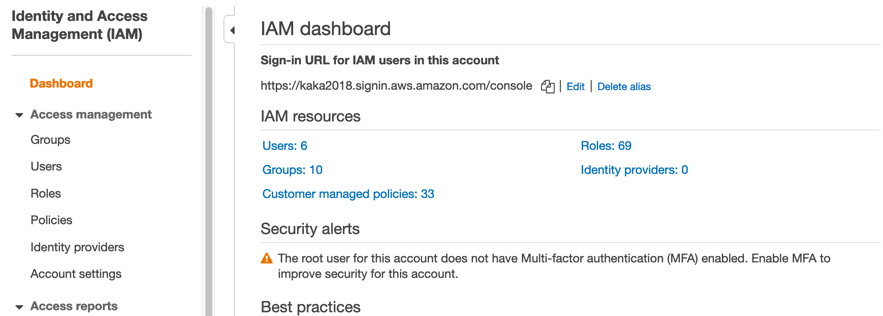
点击Create policy

选择JSON,把下面的json粘贴到输入框中,点击Review policy。
如您在项目中有特定的S3桶,需要在Resource中修改或者添加S3桶名。该blog以global资源为例,如果是用中国区资源需要将相关policy中 arn中 aws 改为 aws-cn
{
"Version": "2012-10-17",
"Id": "PersonalizeS3BucketAccessPolicy",
"Statement": [
{
"Sid": "PersonalizeS3BucketAccessPolicy",
"Effect": "Allow",
"Action": [
"s3:GetObject",
"s3:ListBucket",
"s3:PutObject"
],
"Resource": [
"arn:aws:s3:::user-personalization-demo-batch-input",
"arn:aws:s3:::user-personalization-demo-batch-input/*",
"arn:aws:s3:::user-personalization-demo-batch-output",
"arn:aws:s3:::user-personalization-demo-batch-output/*",
"arn:aws:s3:::user-personalization-demo-fulldata",
"arn:aws:s3:::user-personalization-demo-fulldata/*",
"arn:aws:s3:::user-personalization-demo-datasetupdate",
"arn:aws:s3:::user-personalization-demo- datasetupdate/*",
"arn:aws:s3:::user-personalization-demo",
"arn:aws:s3:::user-personalization-demo/*"
]
}
]
}
添加访问策略名称和描述,点击创建策略
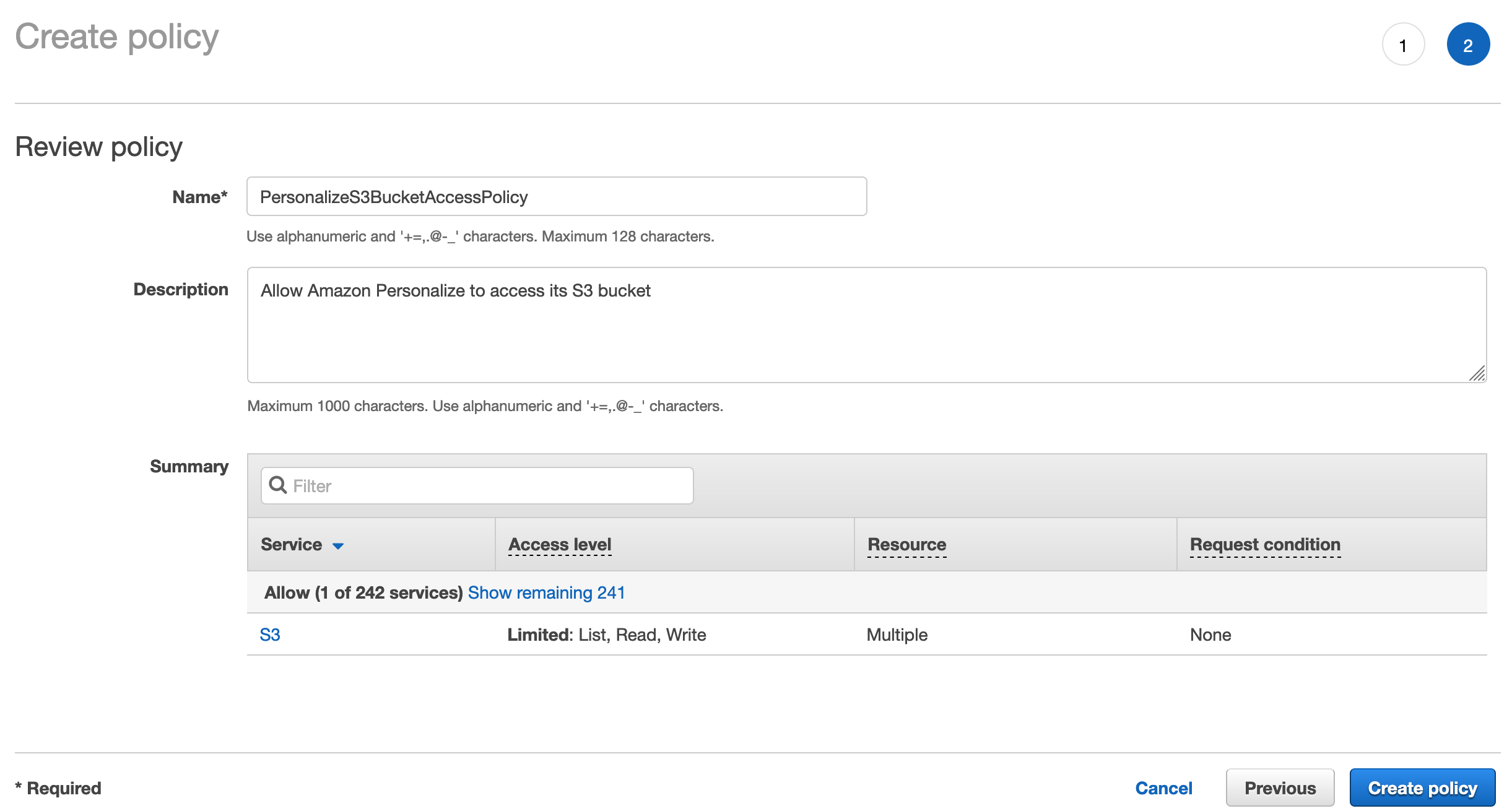
回到角色PersonalizeRole页,添加新创建的PersonalizeS3BucketAccessPolicy
访问策略.
点击Attach policies
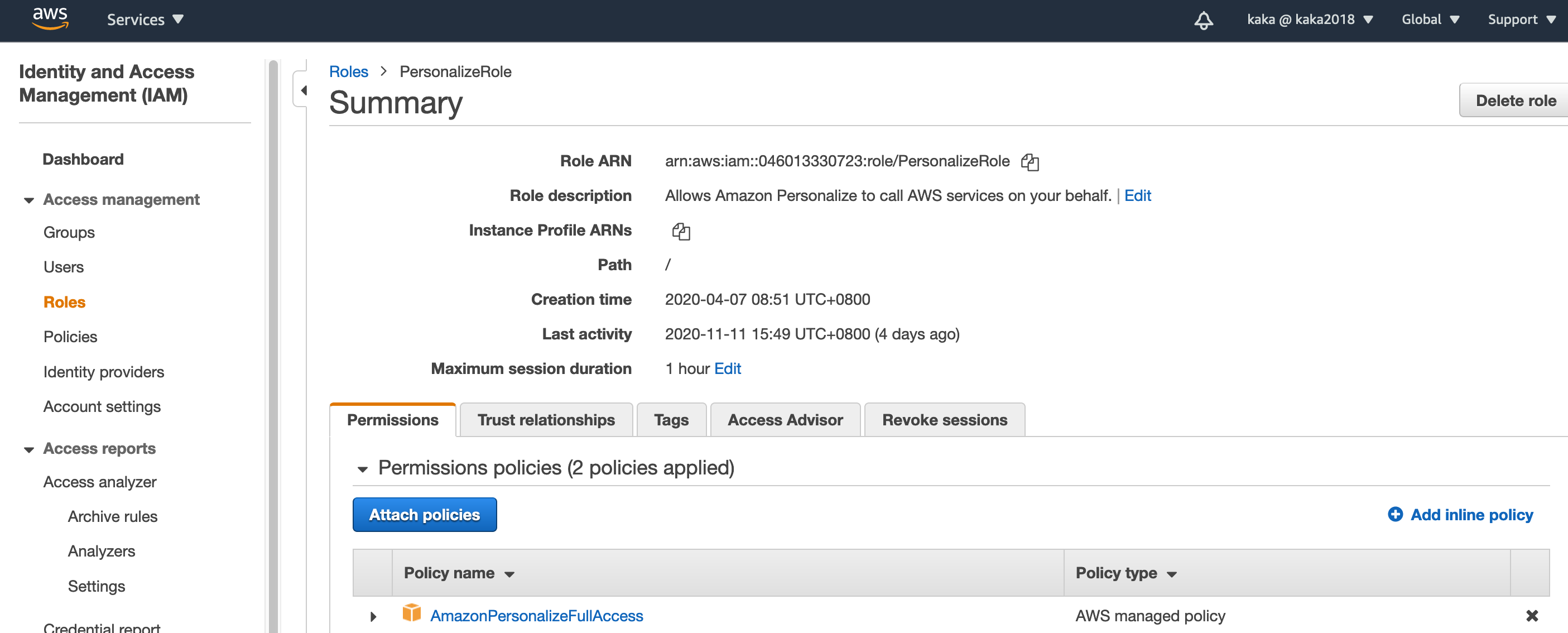
在搜索框中搜索PersonalizeS3BucketAccessPolicy,选中该策略,点击Attach policy
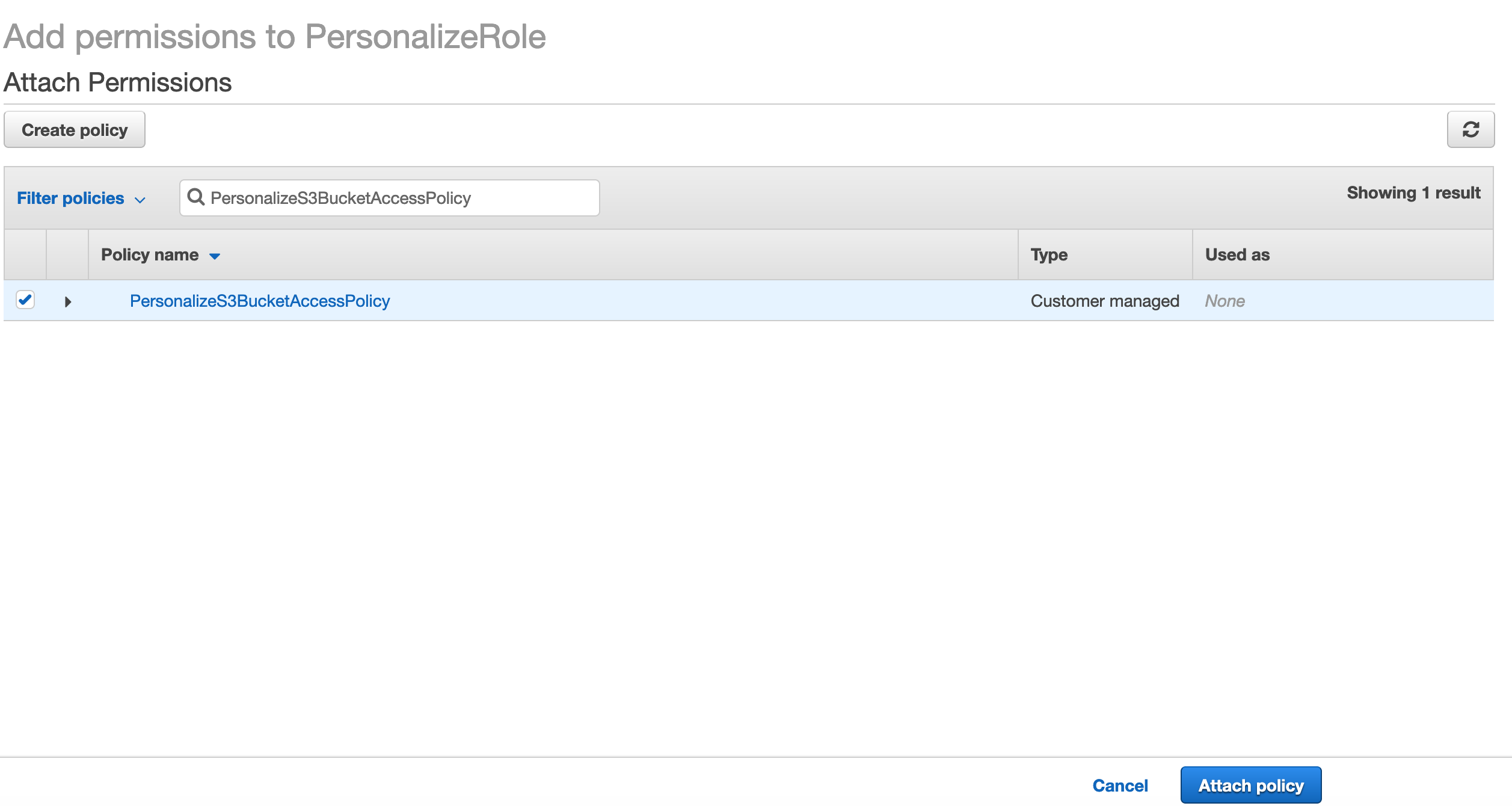
创建S3桶。
下面以创建user-personalization-demo-fulldata为例。其余桶按照同样方法创建
user-personalization-demo-fulldata:存储全量数据(csv格式)
user-personalization-demo-datasetupdate:存储增量数据(csv格式)
user-personalization-demo-batch-input:存储推荐用户列表数据(json格式)
user-personalization-demo-batch-output:存储批量推荐结果(json格式)
AWS 进入S3服务。点击右上角create bucket创建桶

输入S3桶名称,例如user-personalization-demo-fulldata
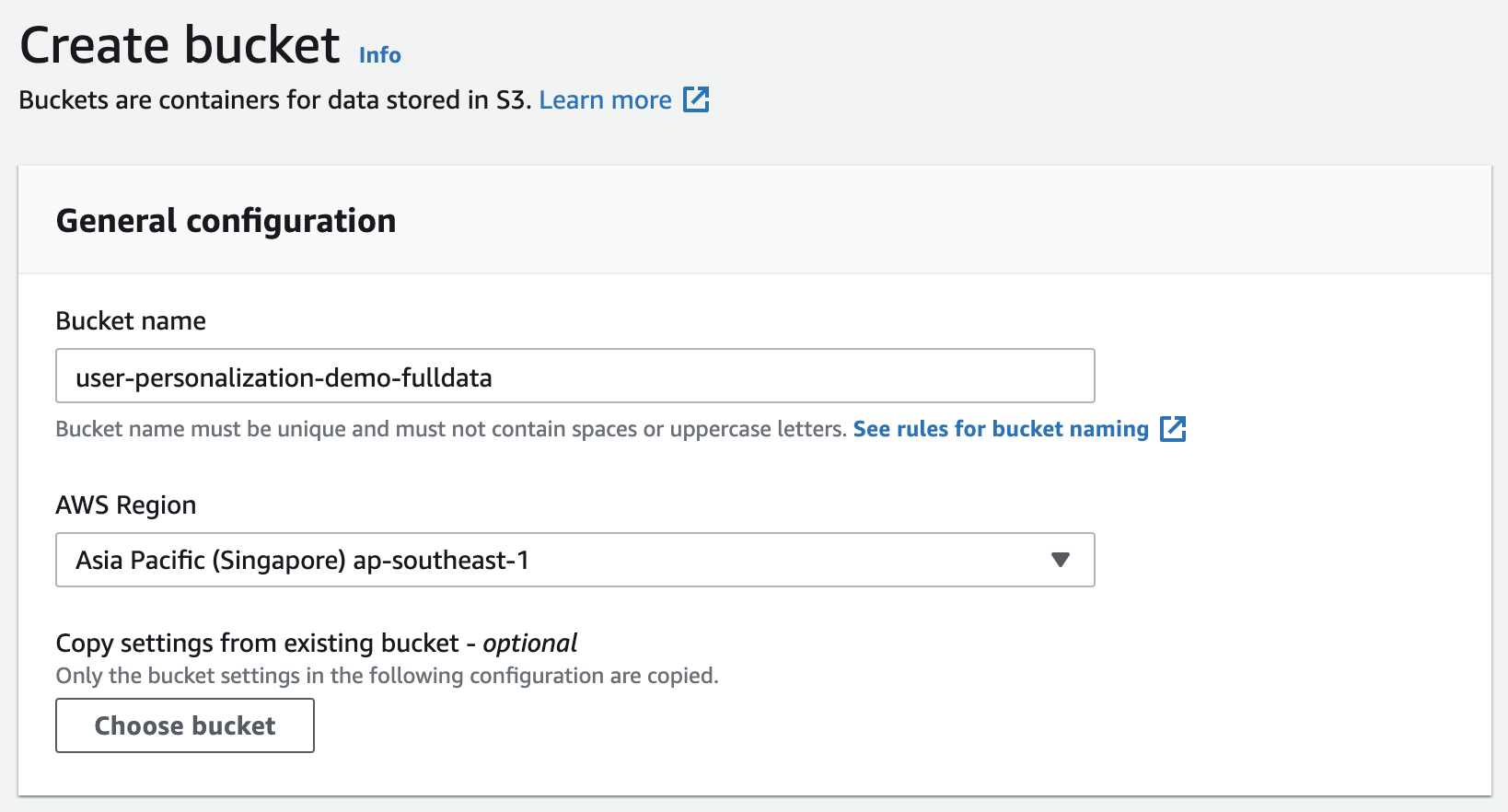
加密部分选择Enable,Amazon S3 key。点击创建桶
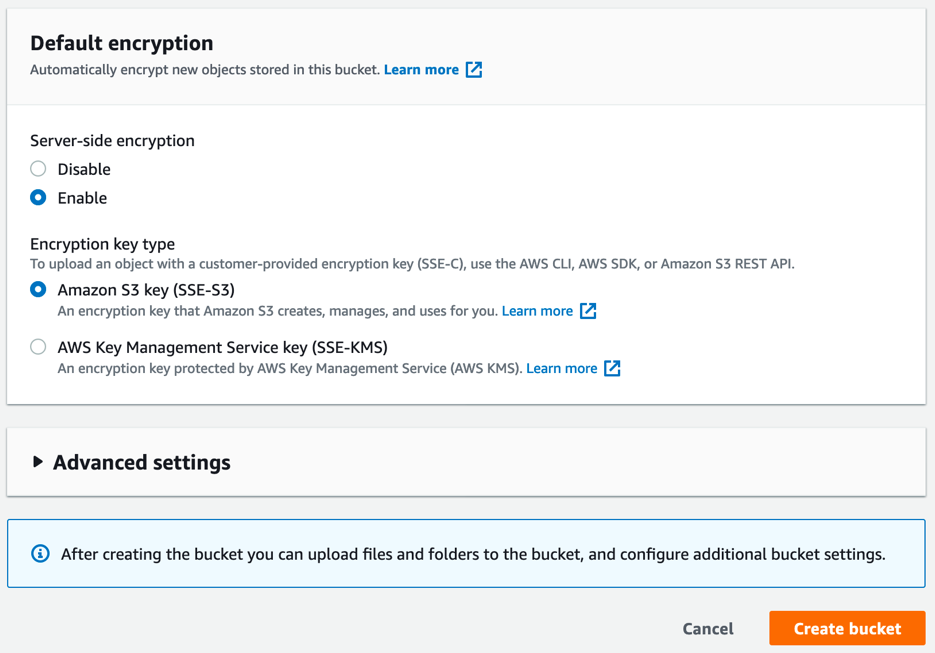
进入S3桶修改桶访问策略,进入Permissions项
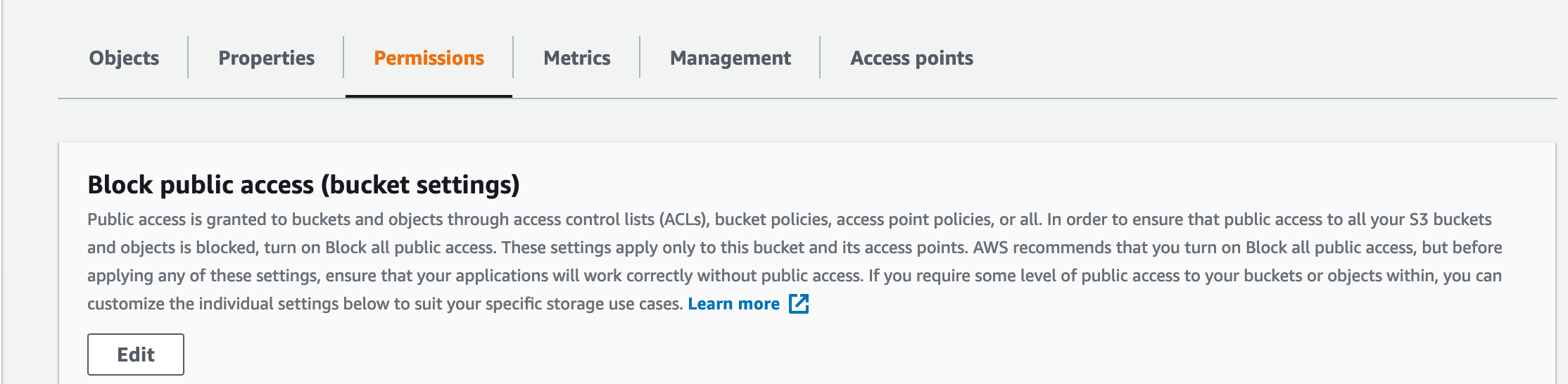
在桶策略部分点击编辑
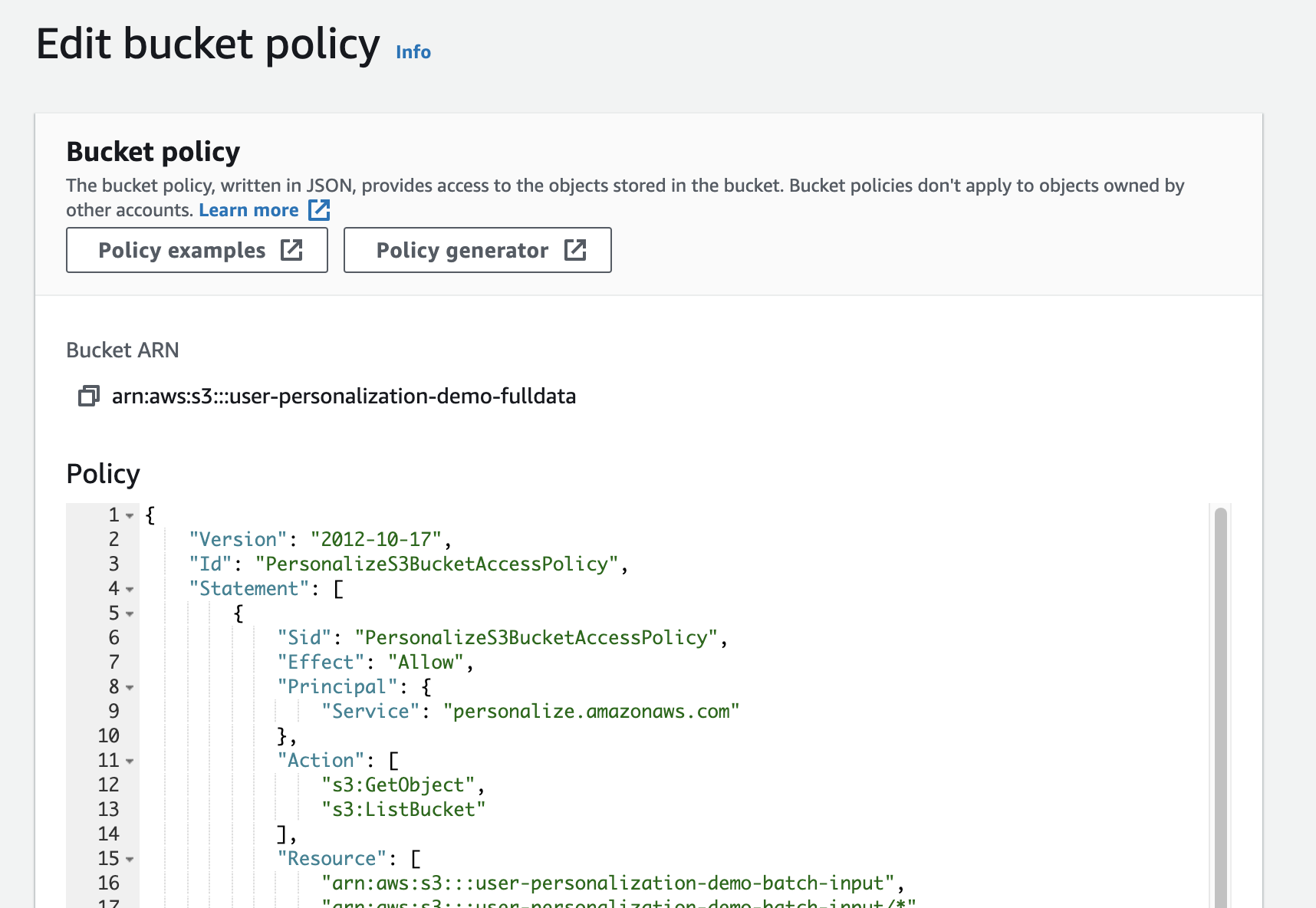
将下面的json拷贝到输入框
如果S3桶名有变化或有添加,需要在Resource中修改或者添加S3桶名。该blog以global资源为例,如果是用中国区资源需要将相关policy中 arn中 aws 改为 aws-cn
{
"Version": "2012-10-17",
"Id": "PersonalizeS3BucketAccessPolicy",
"Statement": [
{
"Sid": "PersonalizeS3BucketAccessPolicy",
"Effect": "Allow",
"Principal": {
"Service": "personalize.amazonaws.com"
},
"Action": [
"s3:GetObject",
"s3:ListBucket"
],
"Resource": [
"arn:aws:s3:::user-personalization-demo-batch-input",
"arn:aws:s3:::user-personalization-demo-batch-input/*",
"arn:aws:s3:::user-personalization-demo-batch-output",
"arn:aws:s3:::user-personalization-demo-batch-output/*",
"arn:aws:s3:::user-personalization-demo-fulldata",
"arn:aws:s3:::user-personalization-demo-fulldata/*",
"arn:aws:s3:::user-personalization-demo-datasetupdate",
"arn:aws:s3:::user-personalization-demo- datasetupdate/*",
"arn:aws:s3:::user-personalization-demo",
"arn:aws:s3:::user-personalization-demo/*"
]
}
]
}
点击保存策略
创建Lambda Service Role,赋予Lambda访问S3,Amazon Personalize的权限
进入IAM,点击Roles,点击Create role
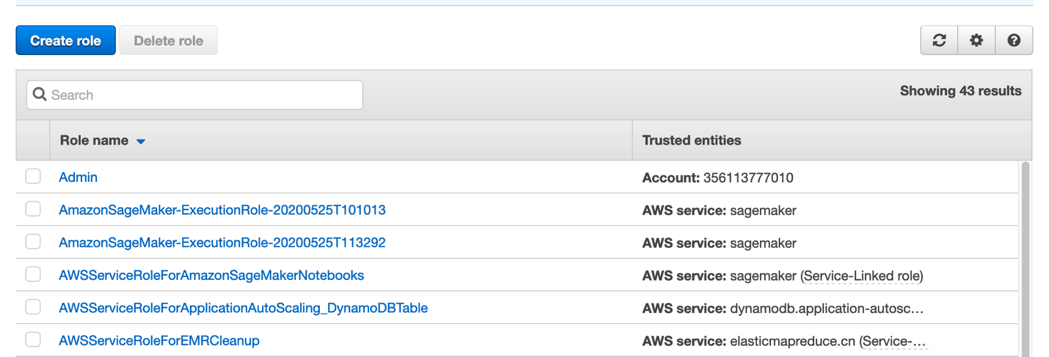
点击Lambda
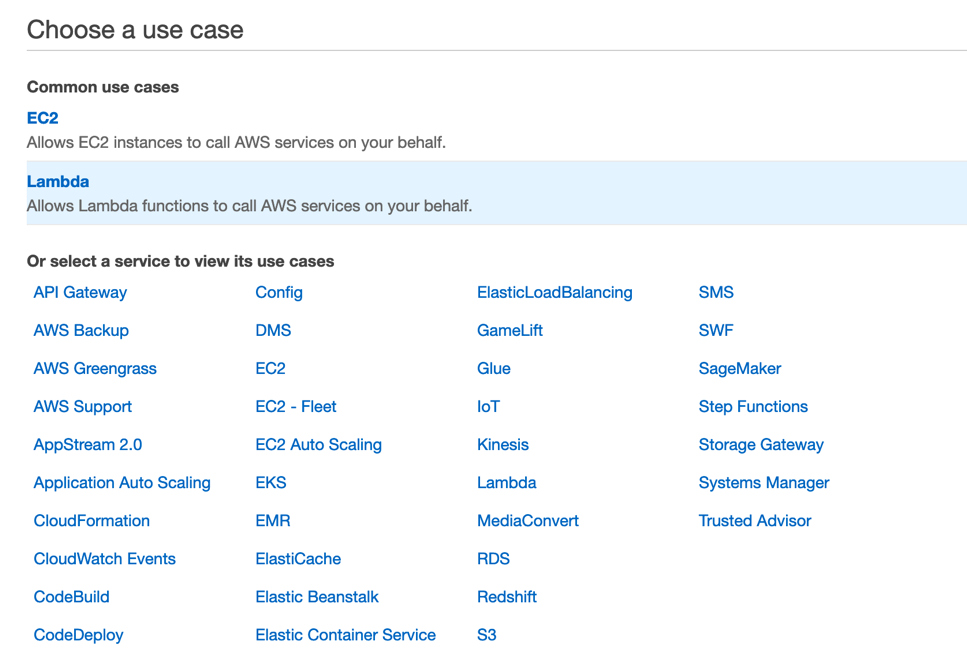
添加AmazonS3FullAccess, CloudWatchFullAccess , AWSLambdaFullAccess , AmazonPersonalizeFullAccess
输入Role name: lambda-s3-personalize。点击 Create role 完成 role 创建。
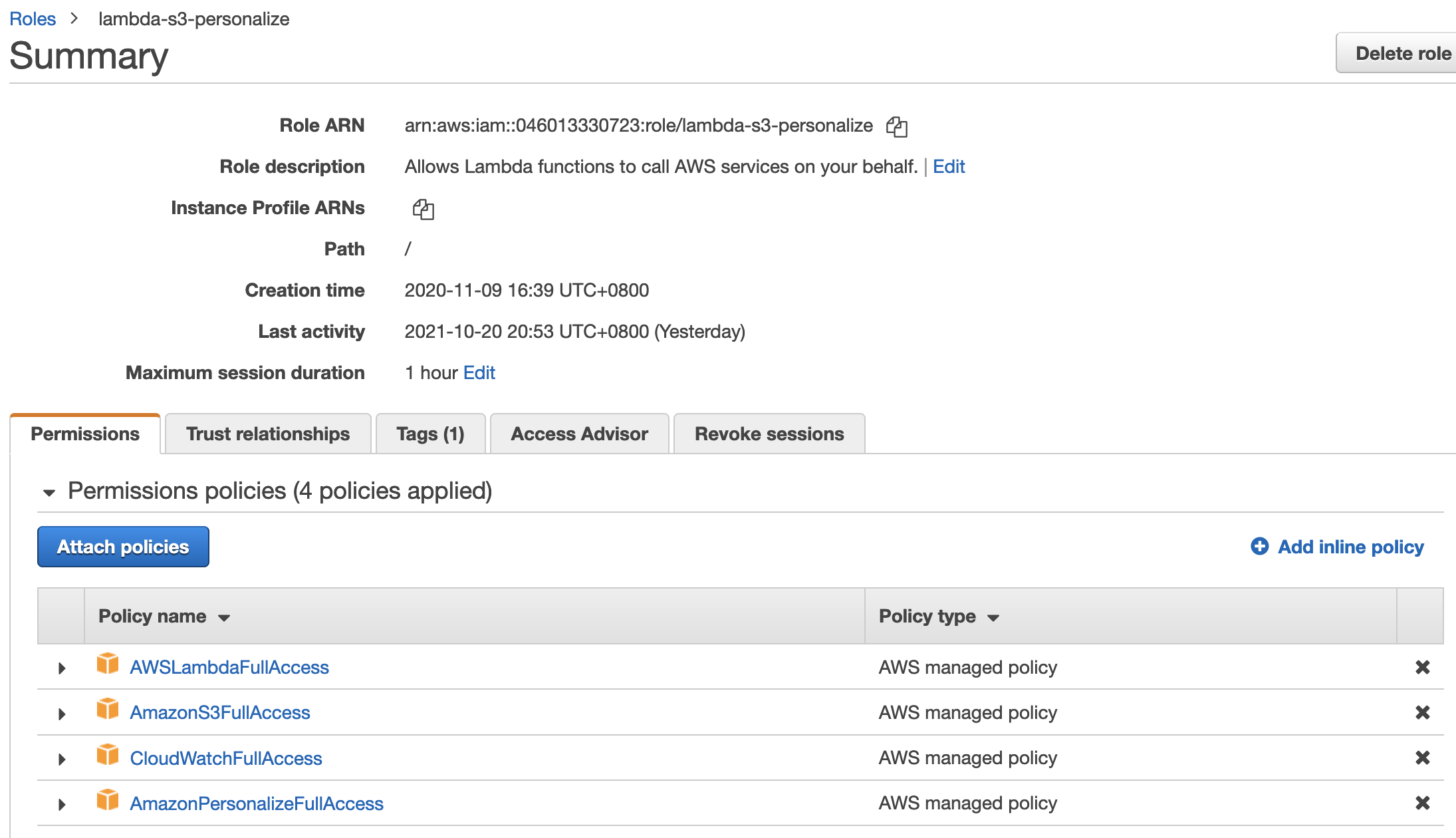
数据处理
MovieLens 数据需要进行处理来满足 Amazon Personalize的数据要求。下面的代码会对评分数据修改列名,生成用户数据,对电影数据修改列名。并结果保存成csv格式
- ‘users.csv’ 用户数据
- ‘items.csv’ 电影数据
- ‘interacts.csv’ 用户评分数据
import pandas as pd
# 读取评分数据,修改列名
dfRatings = pd.read_csv('./ml-latest-small/ratings.csv')
dfRatings.rename(columns={'userId':'USER_ID','movieId':'ITEM_ID','rating':'EVENT_VALUE','timestamp':'TIMESTAMP'},
inplace=True)
dfRatings['EVENT_TYPE'] = 'RATE'
dfRatings.to_csv('interacts.csv',index=False)
# 生成用户数据,生成评分次数列
dfUsers = dfRatings.USER_ID.value_counts().reset_index()
dfUsers.rename(columns={'index':'USER_ID','USER_ID':'RATE_F'},
inplace=True)
dfUsers.to_csv('users.csv',index=False)
# 读取电影数据,修改列名
dfMovies = pd.read_csv('./ml-latest-small/movies.csv')
dfMovies.rename(columns={'movieId':'ITEM_ID','genres':'GENRES'},
inplace=True)
dfMovies=dfMovies[['ITEM_ID','GENRES']]
dfMovies.to_csv('items.csv',index=False)
数据导入
本文中,需要用户数据集,电影数据集和交互数据集创建一个数据集组。有关创建数据集组的说明,请参阅Getting started (console)。
{
"type": "record",
"name": "Items",
"namespace": "com.amazonaws.personalize.schema",
"fields": [
{
"name": "ITEM_ID",
"type": "string"
},
{
"name": "GENRES",
"type": [
"null",
"string"
],
"categorical": true
}
],
"version": "1.0"
}
{
"type": "record",
"name": "Users",
"namespace": "com.amazonaws.personalize.schema",
"fields": [
{
"name": "USER_ID",
"type": "string"
},
{
"name": "RATE_F",
"type": [
"float",
"null"
]
}
],
"version": "1.0"
}
{
"type": "record",
"name": "Interactions",
"namespace": "com.amazonaws.personalize.schema",
"fields": [
{
"name": "USER_ID",
"type": "string"
},
{
"name": "ITEM_ID",
"type": "string"
},
{
"name": "RATING",
"type": [
"null",
"float"
]
},
{
"name": "TIMESTAMP",
"type": "long"
}
],
"version": "1.0"
创建模型训练Lambda函数
使用lambda-s3-personalize role和下面的代码创建模型训练Lambda函数,训练需指定训练recipe,数据组
import json
import urllib.parse
import boto3
import logging
import os
import re
import datetime
from botocore.exceptions import ClientError
import time
logger = logging.getLogger()
logger.setLevel(logging.INFO)
def lambda_handler(event, context):
# 获取用户ID,aws区域
record = event['Records'][0]
client = boto3.client("sts")
account_id = client.get_caller_identity().get('Account')
awsRegion = record['awsRegion']
create_solution_response = None
solution_name = 'user-personalization-demo'
recipe_arn = "arn:aws:personalize:::recipe/aws-user-personalization" # 训练的recipe
dataset_group_arn = 'arn:aws:personalize:%s:%s:dataset-group/user-personalization-demo' % (awsRegion,account_id)
personalize = boto3.Session().client('personalize')
# 创建一个新的solution
try:
create_solution_response = personalize.create_solution(name=solution_name,
recipeArn= recipe_arn,
datasetGroupArn = dataset_group_arn,
performHPO = True,
solutionConfig={
'hpoConfig': {
'hpoResourceConfig': {
'maxNumberOfTrainingJobs': '30',
'maxParallelTrainingJobs': '10'}}})
solution_arn = create_solution_response['solutionArn']
print('solution_arn: ', solution_arn)
except personalize.exceptions.ClientError as e:
if 'EVENT_INTERACTIONS' not in str(e):
print(json.dumps(create_solution_response, indent=2))
print(e)
time.sleep(120)
# 首先创建一个新的solution version。此过程为模型训练,时间较长,所以不需要等待其训练结果。执行完成后直接结束lambda函数即可。
try:
solution_arn='arn:aws:personalize:%s:%s:solution/user-personalization-demo' % (awsRegion,account_id)
create_solution_version_response = personalize.create_solution_version(solutionArn = solution_arn)
solution_version_arn = create_solution_version_response['solutionVersionArn']
print('solution_version_arn:', solution_version_arn)
except Exception as e:
print(e)
raise e
在创建完Lambda函数之后,可以为训练函数添加定时训练触发。例如我们可以用 EventBridge 定义每月训练一次cron(0 2 1 * ? *)。
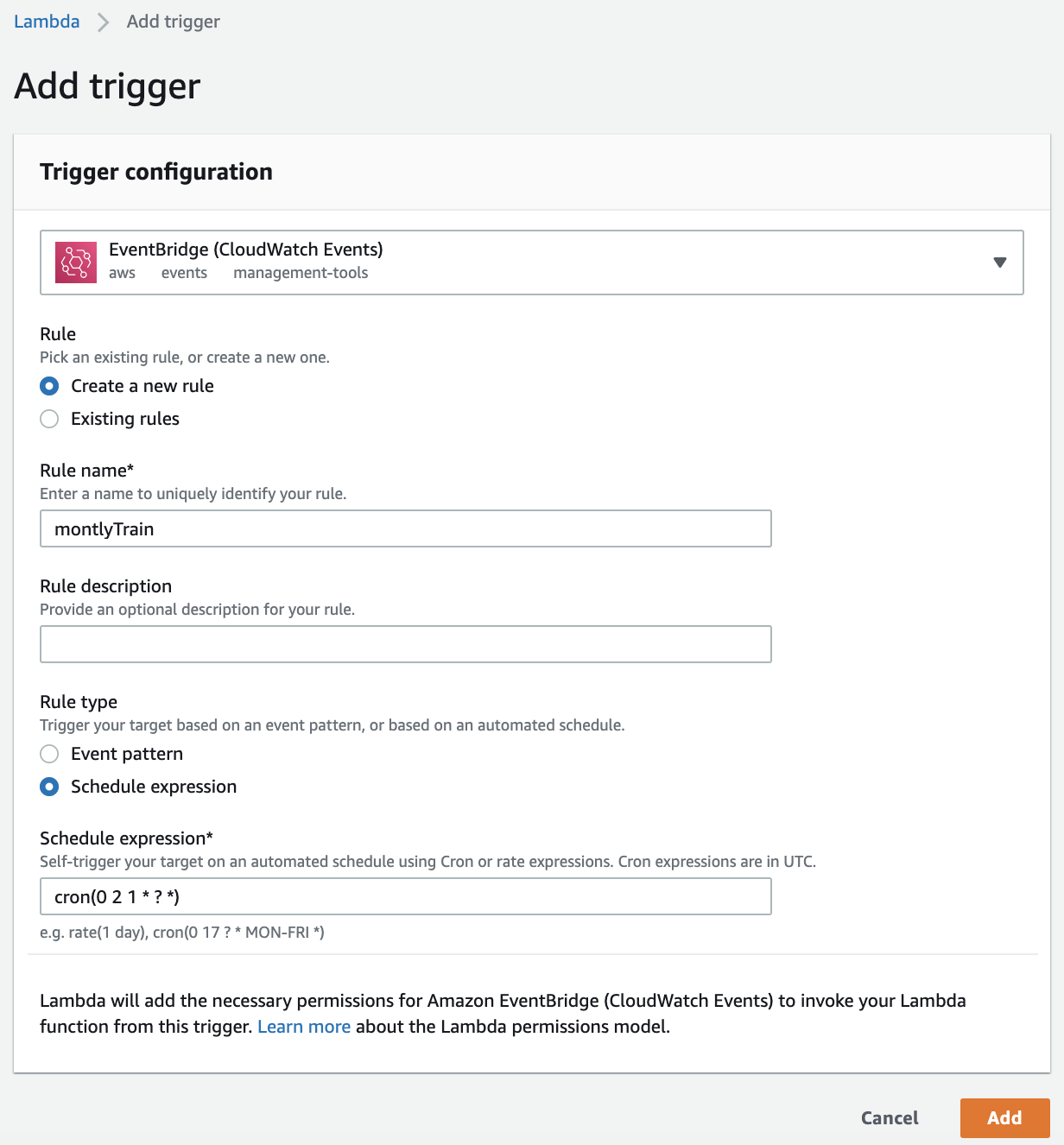
创建数据更新,模型更新Lambda函数
使用lambda-s3-personalize role和下面的代码创建Lambda函数,代码会对新增的数据csv文件进行解析,并更新Amazon Personalize中相应的数据,最后对模型进行更新。模型更新是为了在未来的推荐中有新用户或者是新电影
如果您的数据中有必须字段之外的字段,需在代码中添加相应字段以完成数据导入。
import json
import urllib.parse
import boto3
import logging
import os
import re
import datetime
import csv
from botocore.exceptions import ClientError
import time
logger = logging.getLogger()
logger.setLevel(logging.INFO)
print('Loading function')
s3_client = boto3.client('s3')
def lambda_handler(event, context):
# 获取s3文件触发相关信息(s3路径)
record = event['Records'][0]
downloadBucket = record['s3']['bucket']['name']
key = urllib.parse.unquote(record['s3']['object']['key'])
# 获取用户ID,aws区域
client = boto3.client("sts")
account_id = client.get_caller_identity().get('Account')
awsRegion = record['awsRegion']
print(key)
print(event)
print(account_id)
print(awsRegion)
logger.info(key)
prefix = 'user-personalization-'
personalize = boto3.Session().client('personalize')
personalize_runtime = boto3.Session().client('personalize-runtime')
personalize_events = boto3.Session().client('personalize-events')
role_arn = 'arn:aws:iam::%s:role/PersonalizeRole' % account_id
# 下载文件到lambda本地目录进行处理
download_path = '/tmp/{}'.format(key)
s3_client.download_file(downloadBucket, key, download_path)
try:
# 用户数据增量更新
if 'users' in key:
datasetType = 'USERS'
with open(download_path, 'r') as this_csv_file:
# 读取csv文件
data = csv.reader(this_csv_file, delimiter=",")
colList = []
userlist = []
for line in data:
if len(colList) == 0:
colList = line
else:
newTmp = {
'userId': line[colList.index( 'USER_ID' )],
'properties':"{\"RATE_F\":%s}" %(line[colList.index( 'RATE_F' )])
}
userlist = userlist + [newTmp]
personalize_events.put_users(datasetArn='arn:aws:personalize:%s:%s:dataset/user-personalization-demo/%s'% (awsRegion,account_id,datasetType),
users=userlist
)
print('updated users')
# 商品数据增量更新
if 'items' in key:
datasetType = 'ITEMS'
with open(download_path, 'r') as this_csv_file:
# 读取csv文件
data = csv.reader(this_csv_file, delimiter=",")
colList = []
itemlist = []
for line in data:
if len(colList) == 0:
colList = line
else:
newTmp = {
'itemId': str(line[colList.index( 'ITEM_ID' )]),
'properties':'''{\"creationTimestamp\":%s,\"GENRES\":\"%s\"}''' %(int(time.time()),line[colList.index( 'GENRES' )])
}
itemlist = itemlist + [newTmp]
personalize_events.put_items(datasetArn='arn:aws:personalize:%s:%s:dataset/user-personalization-demo/%s'% (awsRegion,account_id,datasetType),
items=itemlist)
print('updated items')
# 交互数据增量更新
if 'interacts' in key:
datasetType = 'INTERACTIONS'
event_tracker_name = 'user-personalization-demo'
dataset_group_arn = 'arn:aws:personalize:%s:%s:dataset-group/user-personalization-demo'% (awsRegion,account_id)
# 创建 eventTracker
even_tracker_response = personalize.create_event_tracker(name=event_tracker_name,
datasetGroupArn=dataset_group_arn)
event_tracker_arn = even_tracker_response['eventTrackerArn']
event_tracking_id = even_tracker_response['trackingId']
print(even_tracker_response)
print(event_tracking_id)
time.sleep(180)
# 逐行导入交易数据
with open(download_path, 'r') as this_csv_file:
# 读取csv文件
data = csv.reader(this_csv_file, delimiter=",")
colList = []
for line in data:
if len(colList) == 0:
colList = line
else:
personalize_events.put_events(
trackingId = event_tracking_id,
userId= line[colList.index( 'USER_ID' )],
sessionId = '1',
eventList = [{
'sentAt': int(time.time()),
'eventType' : str(line[colList.index( 'EVENT_TYPE' )]),
'itemId' : line[colList.index( 'ITEM_ID' )],
'properties': '''{\"EVENT_VALUE\":%s} ''' % (line[colList.index( 'EVENT_VALUE' )])
}]
)
print('updated interacts')
# 删除 eventTracker
response = personalize.delete_event_tracker(eventTrackerArn=event_tracker_arn)
# 在更新完交易数据后进行user-personalization模型更新
solution_arn = 'arn:aws:personalize:%s:%s:solution/user-personalization-demo'% (awsRegion,account_id)
create_solution_version_response = personalize.create_solution_version(solutionArn = solution_arn, trainingMode = "UPDATE")
solution_version_after_update = create_solution_version_response['solutionVersionArn']
print('updated solution')
except Exception as e:
print(e)
print('Error getting object {} from bucket {}. Make sure they exist and your bucket is in the same region as this function.'.format(key, downloadBucket))
raise e
由于是数据更新,所以我们在 Lambda 函数的触发设定为 S3 桶事件触发。在 S3 新增数据桶中有任何的数据导入,都会触发该函数。当user-personalization-demo-datasetupdate 桶中有数据更新时,会触发数据更新和模型更新。
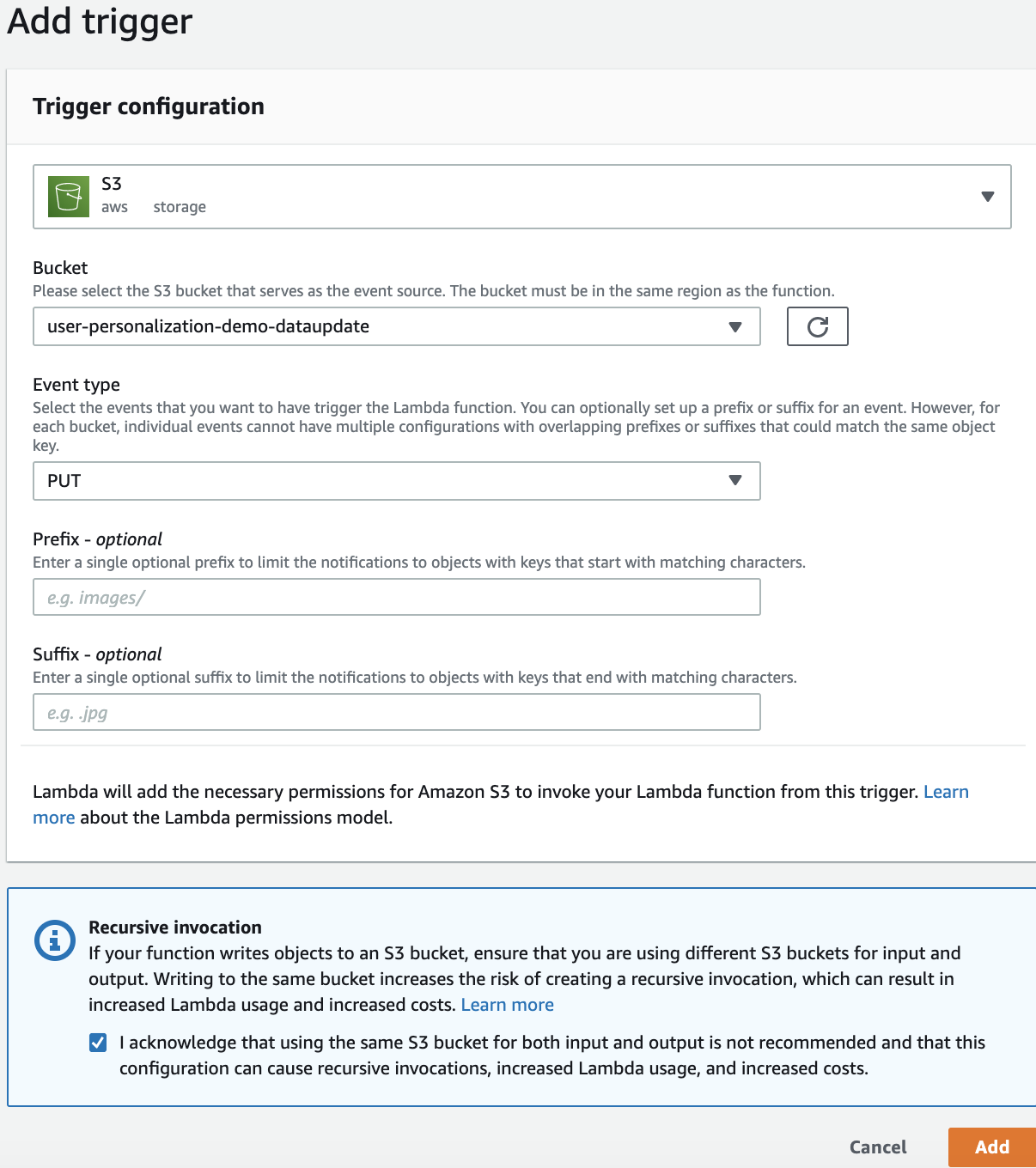
创建模型批量推理Lambda函数
用下面的代码创建Lambda函数,代码会根据user-personalization-demo-batch-input中的用户数据列表,Amazon Personalize会为这些用户做出系统推荐,并且将模型推荐结果写入user-personalization-demo-batch-output
import json
import urllib.parse
import boto3
import logging
import os
import re
import datetime
from botocore.exceptions import ClientError
import time
logger = logging.getLogger()
logger.setLevel(logging.INFO)
def lambda_handler(event, context):
# 获取用户ID,aws区域
record = event['Records'][0]
client = boto3.client("sts")
account_id = client.get_caller_identity().get('Account')
awsRegion = record['awsRegion']
# batch的job名字和用户权限名称
current_time = int(time.time())
batchJobName = 'user-personalization-demo-batchPredict-%s'%current_time
role_arn = 'arn:aws:iam::%s:role/PersonalizeRole' % account_id
personalize = boto3.Session().client('personalize')
# 获取最新的模型地址
solution_versions_response = personalize.list_solution_versions(
solutionArn='arn:aws:personalize:%s:%s:solution/user-personalization-demo' % (awsRegion,account_id),
maxResults=100
)
solution_version_arn = solution_versions_response['solutionVersions'][0]['solutionVersionArn'] # 选取最新模型
try:
# 批量推荐
personalize.create_batch_inference_job (
solutionVersionArn = solution_version_arn,
jobName = batchJobName,
roleArn = role_arn,
batchInferenceJobConfig = {
# optional USER_PERSONALIZATION recipe hyperparameters,模型探索比例,新电影时间定义(样例中为20天)
"itemExplorationConfig": {
"explorationWeight": "0.3,
"explorationItemAgeCutOff": "20"
}
},
# 输入数据的s3桶地址
jobInput =
{"s3DataSource": {"path": "s3://user-personalization-demo-batch-input/"}},
# 输出结果的s3桶地址
jobOutput =
{"s3DataDestination": {"path": "s3://user-personalization-demo-batch-output/"}}
)
except Exception as e:
print(e)
raise e
Lambda函数的触发设定为S3桶事件触发。在S3模型预测用户列表数据桶中有任何的数据导入,都会触发该函数
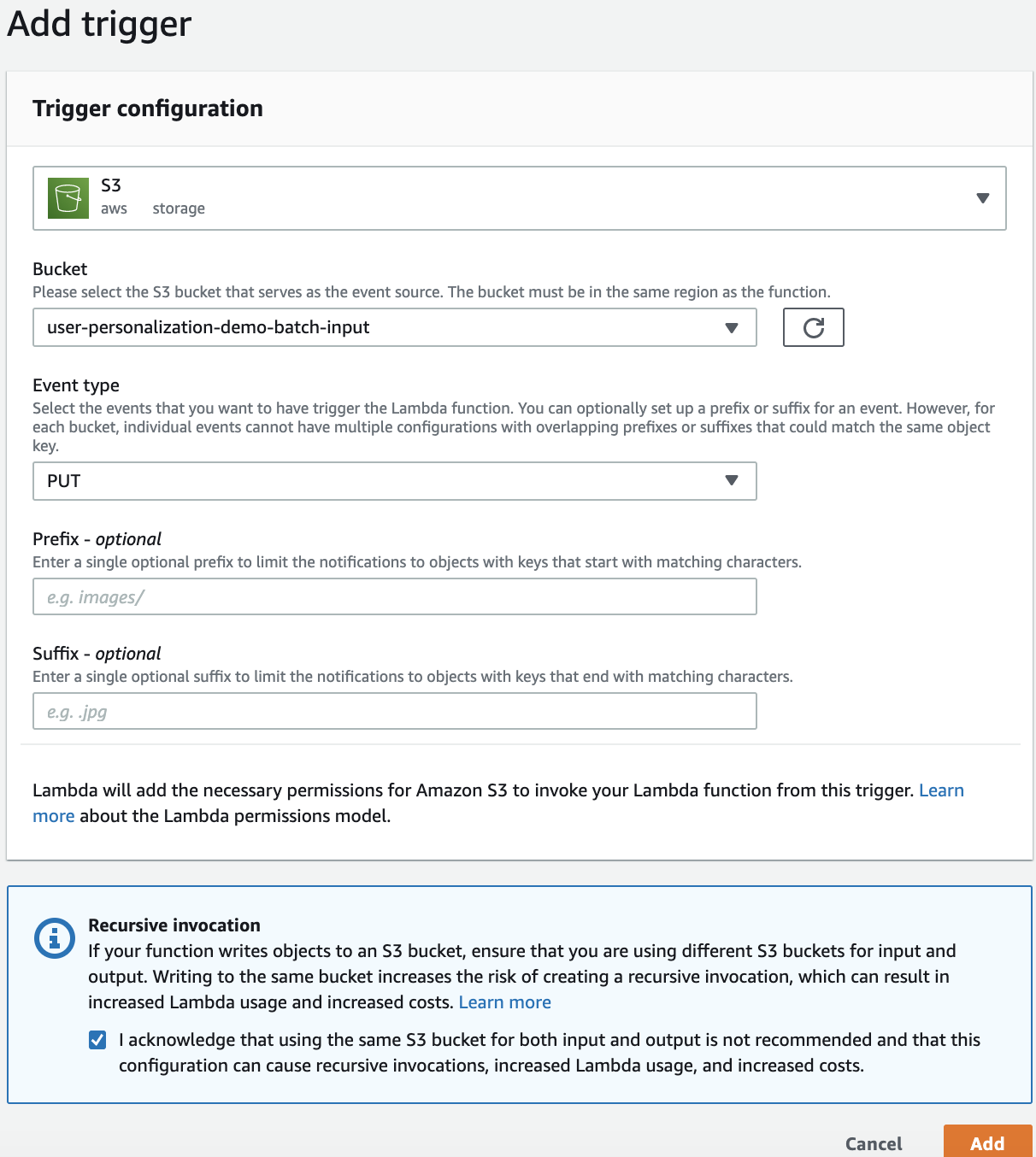
推理用户列表数据要求为json格式
{"userId" : "XXXX1"}
{"userId" : "XXXX2"}
{"userId" : "XXXX3"}
推荐结果存在user-personalization-demo-batch-output s3桶中,格式如下:
{"input":{"userId":"1"},"output":{"recommendedItems":["1485","2012","1391","2770","2539"],"scores":[0.0070954,0.0070838,0.0056013,0.0054147,0.0052189]},"error":null}
{"input":{"userId":"2"},"output":{"recommendedItems":["32587","4878","5679","91658","7438"],"scores":[0.0088202,0.0087002,0.0073412,0.0067746,0.006359]},"error":null}
{"input":{"userId":"3"},"output":{"recommendedItems":["48","367","485","673","2694"],"scores":[0.0041751,0.0039367,0.0039029,0.0037938,0.0036826]},"error":null}
{"input":{"userId":"4"},"output":{"recommendedItems":["5299","1060","2539","2144","1777"],"scores":[0.0063484,0.005426,0.0050096,0.0043322,0.0041946]},"error":null}
总结
到此我们已经利用Amazon Personalize 构建了一个推荐服务。现在 Amazon Personalize 将每个月定期重新训练模型。当我们的应用往新增数据桶中导入数据时,Amazon Personalize 也将为数据和模型进行更新。当应用往‘user-personalization-demo-batch-input’S3 桶中导入新的用户数据列表时,Amazon Personalize 将为这些用户进行批量推荐,并将推荐结果写到‘user-personalization-demo-batch-input’S3 桶中。
本篇作者