AWS Executive Insights / Innovation / ...

Accelerating Enterprise-Wide AI/ML Innovation
A conversation with Karley Yoder, VP and GM of AI, GE Healthcare and Mike Haley, VP of Research, Autodesk
Hear from leaders who have experience in establishing enterprise-wide strategies for accelerating AI/ML innovation and structuring organizations to execute on that strategy. They cover the challenges they've encountered, how they've structured teams, and the communication strategies they've used.
This interview is also available in an audio format. Listen to the podcast by clicking your favorite player icon below, and subscribe to AWS Conversations with Leaders podcast to never miss an episode.

Shaown Nandi (00:06):
Welcome to our panel on accelerating enterprise artificial intelligence and machine learning innovation. I am excited to have two guests from two organizations that have been leading in this space for quite a while. I'm Shaown Nandi, I work for Amazon Web Services where I run solution architects for our strategic accounts. And the guests with me here today are first Mike Haley, VP of Research at Autodesk, and Karley Yoder, our GM of artificial intelligence at GE Healthcare. Mike, can you tell us a little bit about your role?
Mike Haley (00:37):
I head up the research group at Autodesk. Part of my role is creating various centers of excellence in technology to keep Autodesk on the forefront of leveraging technology for designing and creating things in the world. But part of my role over the last five or six years has also been establishing a true machine learning practice at Autodesk. That's a very large focus for research right now. But it's also been a dedicated focus to get the company prepared and leveraging machine learning in all of our products and solutions.
Shaown Nandi (01:15):
Awesome. And Karley, please tell us about yourself in your role.
Karley Yoder (01:19):
My role is the General Manager of Artificial Intelligence across the business we do here at GE Healthcare. I get the joy of leading our engineering, data science and product teams, as we work to understand how we can apply AI to make devices and solutions smarter. And then also working with a startup ecosystem to help folks who are really brilliant and driving innovation in this space, bring their innovations into the healthcare workflows. It's a job where I get to get up every morning and think about how we can bring the best of AI to help providers all over the world provide the best outcomes and solutions to their patients.
How is AI/ML affecting designers and customers?

Shaown Nandi (02:10):
How has AI/ML started to change the role of the designers and impacting your customers in general, what's it doing for their experiences?
Mike Haley (02:18):
So if you look at the average designer, whether you're talking manufacturing, construction, media or entertainment, the average designer has really two fundamental things that they're dealing with. One of them is that the process of design is actually about bringing an enormous number of variables together. I mean, imagine designing a building. You have to think of the people in the building, the function of the building, the materials are building, the heating of the building, how the building is created this, there are literally 10s of 1000s of variables that can go into creating a building and most other products as well. No designer, no human designer, at least, can actually take all of those 10s of 1000s of variables, and truly give them each the attention they deserve. While you're designing it just our brains can't handle that capacity. So machine learning is beginning to provide the ability for designers to start taking all of those requirements and objectives and constraints and all of that, and interpreting them and helping guide them through the design process to better designs at the end of the day that almost a little bit beyond what a human by themselves, might be able to come up with. So that's the first thing.
The other thing that that designers struggle with terribly today and you know, is largely the creation of all of us in the software business is there's a lot of tedium in design, you know, not everything is creative in the design process, you have to translate files, or you have to do lots of small drawing of details. You have to figure out how to how to get something moved to a process to the right person and the next stage of a construction project. So for example, none of those are creative things. So machine learning is beginning to provide automated mechanisms to start doing that. And that's wonderful, because then that gives more time for the creative people to spend time doing precisely that: creating.
Shaown Nandi (04:24):
That makes total sense. You know, it's interesting, I got to work in the media industry for a long time. And it was very painful to see designers have to flip from that tedious work until they get into the fun stuff. So it's exciting to hear you're changing that.
So Karley, you know, for almost everyone right now healthcare is top of mind, how is AI/ML affecting your customers? How are they sort of leveraging the change experiences in your field?
Karley Yoder (04:48):
I love how Mike focused in on the time that we can give back to the folks who use his solutions. It's very similar here in health care. I mean, this year has been, you know, a far extreme. But even before the global pandemic, health care systems across the world were pressed on costs razor thin margins pressed on time with more folks who needed care than they could give care to, pressed on outcomes, pressed on burnout. You know, a recent studies show that around, you know, 27% of a clinician's time actually gets to be with patients. No one went into health care to spend 73% of their time doing administration or documentation or paperwork. So when we think about what AI can do in healthcare, we think about how it can put the patient back in the center of health care, it can take tasks that providers were already doing, and do them faster, do them more efficiently, let the provider focus their precious time on those high level tasks that only they can tackle, or explaining and connecting with the care team surrounding the patient, or actually interacting with the patient.
So a lot of what we do have done with AI across the healthcare spectrum, is just tried to do those high power pattern recognition tasks, do them extremely efficiently, extremely quickly, and then deliver those insights back to our providers.
To build on it a little bit more than one of the things that we have found and becomes really important when you think about AI in healthcare is, it's not good enough to have a brilliant AI solution, it's not good enough to have good sensitivity and specificity for your deep learning model. If you build beautiful data science, if you build this amazing data science, but deliver it into the workflow in a way that actually makes a provider's life harder, makes them go to a new screen, makes them open a new application, makes them go to a different room, it won't be adopted. And even if you have that amazing insight, identifying a critical condition, taking time out in a documentation process, it won't be adopted and used. So we have found that, just as important as developing the AI and harnessing the power that this new technology has brought to healthcare, is how you think through the process of delivering that AI to clinicians in a way that feels invisible.
AI vs ML vs deep learning

Shaown Nandi (07:32):
Both of you actually mentioned artificial intelligence, machine learning, deep learning. You know, not every organization has hit the same level of maturity that Autodesk and GE Healthcare have had. Can you tell us a little bit about how you explain AI versus ML versus deep learning? What does that what does that mean to these organizations?
Karley Yoder (07:55):
It's really a hierarchy. Artificial intelligence is is a science practice that's been around since the 1950s. It encompasses a whole lot. Machine learning is a sub segment of artificial intelligence. And deep learning is a sub segment underneath machine learning that specifically recognises and looks at large, large amounts of data to almost write code itself. So it mimics the way the human brain works. And if you see enough patterns, if you see enough examples of that data, it's actually able to turn all those data inputs into essentially code or pathways to the right answer on its own.
Shaown Nandi (08:38):
Can you tell us a little bit about the the maturity of AI/ML, considering the science of it, and that the practice of it hasn't always been as mature as some of the ideas, how do you see that evolution? And how are we starting to see early value?
Mike Haley (08:54):
Yeah, you can barely find a university on the planet today that has got a computer science department that's not looking at some aspect of deep learning, some area of machine learning. So there's this rapid uptake of a new technology, which is super exciting. But what what's also concerning a little bit with that is there's a maturation cycle you have to go with through technology before it reaches a level where it's truly a practice. Generally if I looked across industries right now, I would say the academic advances in the technology have been astonishing. The actual practices of truly scaling it within a company, really delivering it through products is still really at its very, very infancy. So there's an interesting tension point between those two.
I'm incredibly excited around what we can do with deep learning. We are doing work in an area at Autodesk called generative design, where we use the computational power to actually examine these incredibly complicated design spaces. It doesn't even use data. But it is based on optimization algorithms, which is very similar to machine learning. But now we're beginning to couple machine learning with these high computation optimization problems and taking them to new levels. So as we go in, we are discovering new uses for the machine learning, new places we can put it in our products, new problems we can solve for our customers. What we're seeing right now is the challenge: how do you stay on one hand, right at the bleeding edge of this machine learning, you know, tsunami, that we're in the middle of that's going to continue for a good long time, while at the same time operationalizing that technology, figuring out where you're going to put it into the product? How are you going to use it, and you don't you don't get to check it out on the user interface. And in fact, the nature of user interfaces themselves are fundamentally changing as a result of machine learning where we're talking to our devices as we are gesturing to them. There's tons of potential. But we're also in the middle of the sort of slightly operational crisis, I would say, of figuring out how to really make this truly land and be accessible to everyone.
Communicating the importance of AI to non-technical teams

Shaown Nandi (11:35):
You can see that future vision where we don't talk about artificial intelligence or machine learning or deep learning, because we're just embedded concepts. But how do you deal with that today? How do you explain the importance of investing in AI and ML to non technical leaders? You alluded to the HR teams and the finance teams, and maybe in some businesses, sales teams or engineering teams, what method you use to address that?
Karley Yoder (11:59):
I usually point myself to non-healthcare industries and say, when you type something into Google and it pre-populates exactly what you were going to say, that's AI.You don't call it AI, you just find yourself more effective and efficient in your day, right? When you finish Stranger Things on Netflix and they immediately suggest the next show that you've been dying to watch, you don't know that's AI, you just know that they have built a product that responds to your needs. And so when I think about encouraging, whether it's a marketing team, a sales team, a finance team, to think about how they could use artificial intelligence in the work that they do, don't think about the technology. First, think about the problems you're trying to solve. Think about really being able to define what it is that takes more of your day, more of your money, more of your team's energy than it should. Understand that problem well, and then also think about partnerships. Not everyone has to build the data science model themselves, that would be a crazy way to approach artificial intelligence. Even at GE Healthcare, we rely deeply on partnerships. So when we are defining a problem to solve, we live in the hospital, we live with our clinical partners walking in their shoes, understanding their problem, before we ever start doing the data science aspect of it.
Shaown Nandi (13:27):
How are you enabling your teams to realize this value? I've heard that you've been working on a center of excellence to drive faster innovation?
Mike Haley (13:35):
About five years ago, we created a center that we call our AI Lab. And it's, as you said, it's our center of excellence in AI. This is a group of researchers and engineers who are solely focused on cutting edge AI, and working with our product teams to see what problems can we really focus that on. So on one hand, it's connecting with academics, it's connecting with the research community and the very bleeding edge of machine learning technology. While on the other side, they are connecting with the product teams' problems, the real problems we're dealing with, and then trying to find ways that we can bring these together. What we found is that creating a centralised team brings velocity, it brings a tension within the company. Indeed, one of the hardest things to do right now is bringing new talent onto your team. There are a lot of companies that are waking up to machine learning and are trying to acquire talent and the salaries are going through the ceiling. The competition is getting harder. So once we once we created this team, we found as a result, we were able to attract good talent, build the team to a decent capacity, and then begin to work even more significantly with our product teams.
Shaown Nandi (15:20):
Mike talks about vision and how that can get people excited and attract talent. Karley, can you tell us a little about the vision that you've gotten to work with around patient care? Right, you talked about that earlier in the first and last mile? And how you're sort of reacting to that in this space?
Karley Yoder (15:39):
So our mission is really a precision health mission, which is being able to do the right thing at the right time for every patient at a global scale. That gets me up in the morning, that gets a lot of people really excited about the impact they can have when they close their laptop at the end of the day. But how do you actually allow progress to be made against a lofty mission, like driving precision health on a global scale? We think about platforms, the tools that allow our data scientists at GE Healthcare to build AI really effectively at scale, and ecosystems. So the ability for us to partner outside of GE Healthcare and bring that innovation in and deliver it to our customers. The best kept secret around data science is 85 to 90% of the work is actually not the data science, it's the data preparation. And so what we've done at GE Healthcare within our Edison platform is created a tool specific to healthcare for prepping data, getting it ready for the data scientists, removing the time from that process, making it as efficient as possible, traceability back to where the data came from, because we're in a regulated space, so that the data scientists have the best chance to move quickly.
We actually use Sagemaker, an AWS tool, for the data scientists. But what we've done in our platform is a lot of industry-specific work to get the data ready, so that our data scientists can focus their time on building the best AI. And then we've worked on platforming to catch that AI and put it inside an x-ray machine, put it inside an MRI machine. So an example to make it more tangible, is right now an MRI, I'm guessing many of you have gone in for an MRI scan, it's it's not very comfortable. You're you're in a small space, it's loud noises, these can take up to 60 minutes to do prostate cancer, the second leading killer, unfortunately, across men in a global scale. One of the best ways to catch prostate cancer and get in front of it in the fight is to catch it early in an MRI scan. If you're too scared to come in and get that scan, or if that scan can't be fit into a provider schedule because it takes too long, we missed that chance for early detection. So we've actually created an AI solution that reduces the scan time while keeping the quality and the beautiful representation of the data just as high. So we've been able to embed that into devices, and begun to deploy it at a global scale. And I will tell you, when our engineers and our data scientists and our product folks get to hear from the providers who say, you know, this changes my day, I get to hear from the patients who say this impacts my life or the life of a loved one, a family member. That's extremely powerful. And it's my job and the job of other folks who kind of set up some of these platform teams to equip our data scientists with the tools and platform capabilities to deliver that innovation that ties to that vision, vision and mission that probably brought them here in the first place.
Shaown Nandi (19:28):
How do you bring that back to your teams and start to cultivate a machine learning culture, help them think about the other types of opportunity to apply AI/ML to?
Mike Haley (19:46):
My perspective on this changed when I started to lead our research team because I realized that the problem we're actually talking about right now is true of most advanced technology. It's part of this maturation cycle that we've been talking about, specifically with AI. One of the ways we we addressed this inside the research group is to create a number of Centers of Excellence teams like the AI team I mentioned earlier. We have other teams in robotics and simulation and systems science and human computer interaction. So they're really core technology teams. We created a number of teams that we call industry futures teams, and we have a team per industry that we serve. And these are filled with researchers as well. But they are deep domain experts in say, areas of manufacturing or methods of designing buildings, or engineering buildings, or creating new products in the 3D printing or subtractive, manufacturing or whatever. So they are deep, deep domain experts in what they do. They spend a large amount of their time working with advanced customers. So our customers are generally people that are building things for other customers, right? They are architects, they are engineers, they are product engineers, factory engineers, those kinds of people. So we bring these advanced customers in early on. And while our science teams are developing modern AI techniques, any sort of new innovative technology, the minute that technology reaches a point that it's tangible, that we can sort of begin to test the viability of their technology, we find we couple that technology with these advanced customers, and we actually do collaborative research projects with them.
We've started to get a very early sense of the viability of this technology and and pretty much like any modern technology, on the first go around, the answer is no, we have to understand a bunch of things better. We do a lot of early iteration, just within the research group. Once we've gone through that iteration for a while, and the viability is beginning to become clear, the path to success is becoming clearer, the customers have really starting to engage in a significant way. And when they're getting excited about the solution, at that point we start to do much deeper engagement with the product teams. So they can actually have a leg up in their early customer research. So we we found that that cycle, which is perhaps unique to the way Autodesk is set up in our industry, works really, really well. And I found it really exciting to be able to have a research group that operates in that way, because we can see from soup to nuts, how to take a new technology and make it kind of truly useful.
Advice for Executives getting started in AI

Shaown Nandi (23:15):
What advice would you give other executives getting started in artificial intelligence and machine learning?
Karley Yoder (23:24):
I think the best piece of advice I can give is don't try to do it alone. What is it that you have deep domain excellence and deep subject matter expertise, deep technology investment in? And then how can you layer on artificial intelligence? On top of that, let me let me give an example at GE Healthcare. When we think about core and context we make, we make medical devices to tackle precision health, as I said, and so when we apply our own data science and AI resources, we are constantly thinking how do we make these devices? How do we make these solutions smarter, more intelligent, producing the data needed in a shorter amount of time at a cheaper price point, etc. But what we've seen emerging is hundreds of startups. Even just in the medical imaging space alone, there's 200 startups, nearing a billion dollars of venture capital backing, and we don't want to compete against these guys. We want to partner with them. And so what we've focused on doing in a lot of the use case areas where they play is we've done what I call building bridges and roads. So we've built the INS restructuring our Edison platform for these newer players and startups to partner with GE Healthcare and quickly get to market to solve healthcare problems. Core context, for the core part of our business where we're thinking about the devices, we're thinking about the solutions that we build, we infuse the AI directly. For the context part of our business, we build the roads and bridges to partner really, really well. And so as you're thinking about your own AI strategy, think about this core and context paradigm, and how you lead with your strengths and what you've always been good at, and then look to partner to bring AI in to make that strength even stronger.
Mike Haley (25:51):
The one the one point that I'll emphasise is the importance of platform, specifically when you're dealing with data. We as auditors, we have an equivalent to Edison, it's called Forge. It's a platform for designing and making and all of our industries. But its emphasis right now is actually on data, it's actually about creating the place where data comes together. It's about creating the tools around that data, and then beginning to roll the experiences and everything else on top so people can come together. And you can aggregate a broad variety of solutions, machine learning solutions together into these more complex workflows. And we as Autodesk just as as Karley said about GE Healthcare, you don't have to do everything, you will fail. If you try to do everything, truly leverage your skills.
Shaown Nandi (26:43):
Looking into the future, what are you planning to stay ahead in AI/ML, both for yourself, and for Autodesk and your customers?
Mike Haley (26:54):
It's all about others. So it can be about our partnerships with academic institutions. We have partnerships with around about 45 different universities working on a broad range of technologies, everything from simulation, and digital twins, to interface technologies to machine learning. So keeping that really fresh and going strong is going to be an important part of this. You're working closely with industry leading partners, people in the industry that are thinking at the cutting edge of of construction and design practices, and building practices and infrastructure. And then finally working very, very closely with our customers. The other thing that I will say is maintaining skills in the company. You know, as a technologist, I feel this is my time. This is the time of learning, of applying my knowledge. But what's exciting about any of us technologists is we love to learn, right? We love to learn new technologies. So this is the time to also create the conditions for continual learning. Those academic connections that I mentioned before the Industrial connections are intricate to that. But you have to create the conditions within the company that support that. So that's something I see in the future. I actually think we're 5% down the road of machine learning right now, I think what we have seen is really just the very, very initial applications of machine learning. We have 95% of that road to go down before we really start seeing the full breadth of what machine learning can do in all of our industries.
Karley Yoder (28:41):
I think Mike hit the nail on the head when he said 5%. If you think about a baseball analogy, what inning are we in? We're not in the first inning, we're still in T-ball. Like we're learning the fundamentals of how to play this game, and really deliver the greatest impact. And so when I think about the things that are most important to transformational changes, I think platform and and I think people and I think they're a little intertwined but platforming. How do we give GE Healthcare employees and our partners outside of GE Healthcare the best possible tools and processes, access to data access to mentors, the best possible way to build AI products at scale? That's platforming. Build fast, deliver easily. If you can get that right, you create a center of gravity that people want to be around because the work they can do makes quick impact in an important sector like healthcare. This could be any technology topic in any industry. It really comes down to the people that you attract and retain to solve these problems. And so the two most important things for me as I look to stay on the forefront of solving these important problems in healthcare is building the tools, building that center of gravity to continually attract the best people within GE Healthcare and the best partners and people outside of GE Healthcare who want to run fast on this journey with us.
Shaown Nandi (30:26):
I'm feeling pretty inspired, hearing both of your stories for what you're driving at both Autodesk and GE Healthcare. I want to thank Karley Yoder, who's Vice President, General Manager of Artificial Intelligence at GE Healthcare, and Mike Haley, who is VP of Research at Autodesk, for joining us today and sharing such amazing stories.
About the author
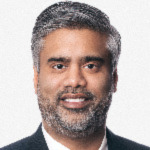
Shaown Nandi, Director, Technology, Strategic Industries and Accounts, AWS
Shaown Nandi is the Director of Technology for Industries and Strategic Accounts at Amazon Web Services. Shaown leads solutions architecture, customer solutions, optimization, and prototyping for AWS’s Industries business, covering all of the company’s largest cutting-edge tech customers. Prior to joining AWS, Shaown served as Chief Information Officer at Dow Jones and head of Shared Services at News Corp, one of the first Enterprise adopters of Cloud. Previously, Shaown also was a global technology executive at IBM. He managed product development teams in the cloud space and subsequently led the North America technical sales teams for Cloud Services. Shaown holds an MBA and a Bachelor’s degree from Cornell.
Related reading
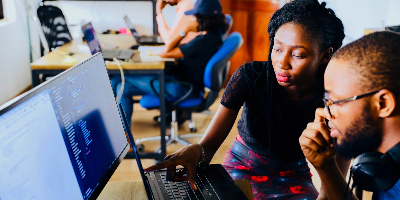
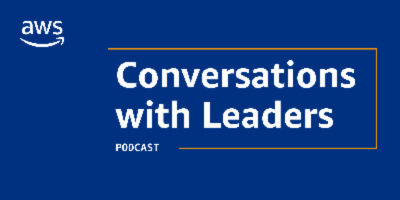
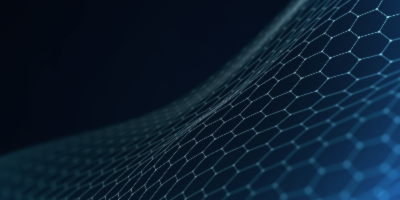
Take the next step
Listen and Learn
Listen to executive leaders and AWS Enterprise Strategists, all former C-Suite, discuss their digital transformation journeys.
Stay Connected
AWS Executive Connection is a digital destination for business and technology leaders where we share information.
Watch on Demand
Get insights from peers and discover new ways to power your digital transformation journey through this exclusive international network.
Get Inspired
Listen in as AWS and customer leaders discuss best practices, lessons, and transformative thinking.