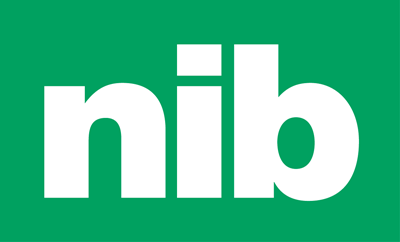
Discover, deliver, scale: Inside nib Group’s machine learning journey
2021
A leader in automation
With more than 1.4 million members in Australia and New Zealand, nib Group (nib) is one of the leading health insurers in the region. By leveraging the latest machine learning innovations, it responds to members faster, more accurately, and with greater efficiency.
“We pride ourselves on leading the way in machine learning. We want to make it quicker and easier for members to claim with us and equally to be able to ensure our frontline teams are able to focus on important enquiries rather than answering basic policy questions that can be answered via our chatbot,” says Mathew Finch, Head of Emerging Technology and Data Platforms at nib.
nib’s team of developers have been experimenting with automation and machine learning for several years. Eager to enhance the company’s machine learning capabilities further, they turned to data science consultancy Eliiza, which is a premier partner of Amazon Web Services (AWS).
Eliiza pulled together a team of engineers and data scientists to help design a machine learning engine called Melvin, which is built using Amazon Textract. They also enhanced nibby, nib’s chatbot, using Amazon Kendra – two innovative AWS solutions to two very different customer service challenges.
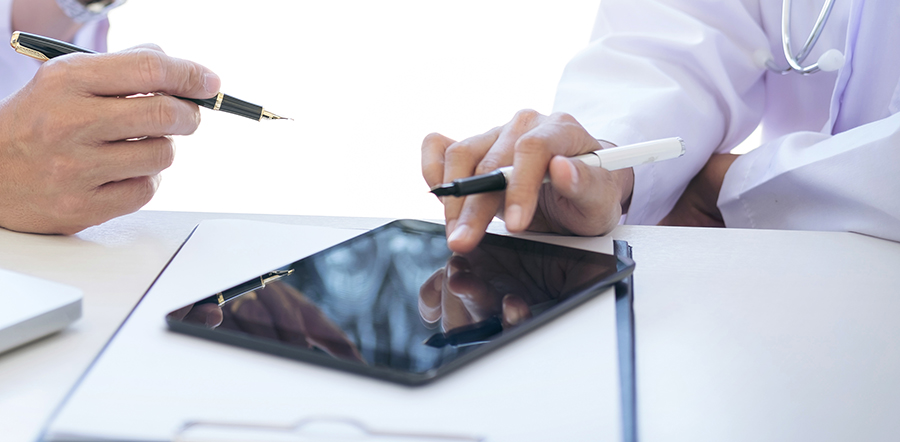

It quickly became apparent that Amazon Textract sets the global benchmark – we couldn’t find anything that could match its performance.
Mathew Finch
Head of Emerging Technology & Data Platforms, nib Group
The first challenge: processing more claims, more quickly, at lower costs
In 2015, nib launched an innovative tool that allows members to submit health insurance claims via a mobile app. After photographing and uploading healthcare receipts directly to the app, members are reimbursed for valid expenses very quickly – usually within 24 hours.
Despite being a huge step forward for members, nib’s claims team were spending too much time extracting data from receipts – such as the customer number, medication, dosage, dates, and provider number – and typing this information into a database.
“We began experimenting with ways to automate the rather routine task of transcription and data entry. Our end goal is to process more claims, more easily, in less time, freeing up the company’s claims team to focus on more critical steps in the verification process,” says James Wilson, CEO at Eliiza.
“We set out to build a machine learning engine that ‘reads’ data from receipts and pre-fills corresponding fields in a database. Our solution needed to be as fast and accurate as nib Group’s claims team. It also needed to slot neatly into their existing claims processing architecture without compromising sensitive medical data.”
A seamless approach to integration
nib’s machine learning journey kicked off with a ‘Discovery’ workshop, where stakeholders came together to identify the challenges that needed to be solved. This led to the creation of a ‘Machine Learning Blueprint’ to guide the next steps and iterations.
In the ‘Delivery’ stage, Eliiza and nib Group began testing a small number of mock documents using different technologies such as Optical Character Recognition (OCR), image recognition technology used to convert image-based text such as handwriting or printed material into machine-encoded, electronic text. Ultimately, one solution emerged ahead of the rest: Amazon Textract, a machine learning service that automatically extracts text, handwriting and data from virtually any document.
“It quickly became apparent that Amazon Textract sets the global benchmark. We couldn’t find anything that could match its performance, both in terms of its accuracy, and its ability to read lower-quality images. It spoke to a lot of the challenges we faced,” says Finch.
There was a slight hitch: Amazon Textract was yet to launch in Australia. Undeterred, Eliiza and nib began using Amazon Textract to build their machine learning engine, dubbed ‘Melvin’, in early 2019, putting the pipeline on standby until Amazon Textract’s Australian launch later that year.
“This actually had several benefits. It gave us time to implement error detection and correction mechanisms and deepen the level of automation. By integrating with other databases like the MIMS drug database, our solution can also verify the validity of claims,” explains Wilson.
Melvin’s components were developed and tested locally using existing nib data structures. Upon completion, Melvin slotted directly into the company’s processing framework using Amazon Simple Queue Service (Amazon SQS), which facilitates asynchronous processing, and Amazon Lambda. Meanwhile, to minimise the risk of exposing sensitive customer data, Eliiza created a quarantine ‘cage’ to ensure this data never leaves the AWS environment.
Scaling up to iterate faster
Since May 2020, nib has been using Melvin to extract and automatically enter data into its database with astonishing accuracy. Amazon Textract’s accuracy exceeds 87% for pre-filled fields overall, while the accuracy of almost half pre-filled fields is 95% or higher. Around half of all claims are processed without any manual re-keying or data entry adjustments. All that’s left for nib’s team is to quickly review and process each claim.
As a result, Melvin shaves around 20 seconds off every claim processed, which means the company can process significantly more claims every day.
Melvin already processes more than 150 claims per day, and nib is exploring whether a percentage of claims can soon be processed with zero human intervention. “Ultimately, that’s the end goal. Ideally, we would like to see a large percentage of claims processed automatically in real-time, so that members receive a response within minutes. That’s what we’re building towards, and data extraction is key to that goal,” says Finch.
The next hurdle: meet nibby
nib launched its chatbot, nibby, in 2017. Created in partnership with AWS partner DiUS, it was built using Amazon Lex – the same deep learning technology that powers Amazon Alexa. The chatbot deflects basic policy coverage questions away from nib’s contact centres, freeing up agents to focus on more complex enquiries.
“We chose Lex because it’s a complete package, which we could seamlessly integrate with other aspects of our AWS environment. Lex took care of the heavy lifting without needing to employ data scientists to create machine learning models. We developed a Proof of Concept within four to six weeks,” explains Finch.
The chatbot has been a huge success. Today, around 65% of chat-based enquiries are handled by nibby while only 35% are diverted to call centres for resolution. The insurer now handles around 15,000 chats per month up from 4,000 chats when nibby first launched.
To build on this success, in 2019 nib teamed up with Eliiza to further improve nibby’s speed and accuracy and better analyse nibby’s data. But how?
Deep automation and intelligent search are a click away
They began experimenting with Amazon Kendra, an intelligent search service that is powered by machine learning.
When a member asks, ‘Am I covered for x-rays?’, nibby can now respond in precise detail. Previously, nibby would share a link to a policy document. Unfortunately, most people avoided reading these documents and will most likely reach out via phone or online chat instead. Today, nibby has the content it needs to provide immediate, accurate responses to member questions about their specific insurance policy.
To achieve this, Eliiza used Amazon Kendra to build nibby’s ‘Kendra Index’. The Index was created by ingesting over 40 product disclosure statements, each several pages long, along with lengthy policy PDFs and FAQs.
“The setup was incredibly quick. With just a few clicks, Eliiza easily configured our Kendra Index and connected it to relevant data sources,” says Finch.
James Dunwoody, Machine Learning Engineer at Eliiza, adds: “Unlike conventional search technology, Kendra’s natural language search capabilities help nibby to answer questions quickly and accurately – no matter how deep the information lives within the Index.”
This solution is on track to roll out in 2021. Eliiza is also working with nib to leverage nibby’s chat data, with over 300,000 conversations collated so far.
Ultimately, nib and Eliiza hope to expand nibby to handle telephone enquiries, too.
“We’re now using Amazon Connect to make nibby not just chat-oriented but voice-based. This means our members will be able to converse with a very human-sounding bot, deflecting even more enquires away from our contact centres,” says Finch.
“We receive 150,000 calls per month – that’s 10 times more than our chat-based enquiries. If we can deflect just 10% of these calls, that will represent huge savings and efficiency gains.”
Going above and beyond: how nib has surpassed its self-service targets
By entrusting labour-intensive, repetitive tasks to AWS technologies, nib is on track to continually improve its self-service targets – a term that describes the percentage of member enquiries that require zero human intervention.
“One of our key metrics for success is self-service, which is how we judge all the work we do in the chat/voice and member enquiry space,” says Finch.
Eight months ago, the insurer’s self-service rate was between 35% and 40%. Today, it is 65%, which means just 35% of chats are deflected to contact centres – the rest are expertly handled by nibby, freeing employees up to handle the more complex cases.
“We never thought we’d get our self-service rate past 50% so we’re really happy with 65%. This results from a combination of a few different technologies, including Amazon Kendra and Amazon Lex, which now sit behind nibby. Our goal is to keep pushing our self-service rate up as high as we can.”
From Amazon Textract to Amazon Lex, and Amazon Kendra, nib has embraced a suite of AWS solutions to challenge the status quo and deliver innovative first for the industry.
It’s an exciting era for data-driven companies that embrace artificial intelligence and machine learning in innovative ways. By automating time-intensive tasks like data entry or fielding member enquiries, nib is one of the disruptors leading the way.
About nib Group
nib Group (nib) provides health and medical insurance to over 1.4 million members in Australia and New Zealand. It is committed to helping its members to make better informed healthcare decisions, transact with healthcare systems and generally live healthier lives. nib Group also provides health insurance to almost 200,000 international students and workers. It is Australia's third largest travel insurer and global distributor of travel insurance through nib Travel.
Benefits
- Saves an average of 20 seconds handling time per claim by extracting and pre-filling data with accuracy of 87% to 95%
- 50% of claims processed require no further human intervention or data entry corrections, allowing employees to focus on more complex cases
- In just 18 months, nib’s self-service rate has increased from 35 - 40% to 65%
- nib chatbot, nibby, now fields 15,000 chats per month, up from 4,000 in 2017
AWS Services Used
Amazon Textract
Amazon Textract is a machine learning service that automatically extracts text, handwriting and data from scanned documents that goes beyond simple optical character recognition (OCR) to identify, understand, and extract data from forms and tables.
Amazon Lex
Amazon Lex is a service for building conversational interfaces into any application using voice and text. Amazon Lex provides the advanced deep learning functionalities of automatic speech recognition (ASR) for converting speech to text, and natural language understanding (NLU) to recognize the intent of the text, to enable you to build applications with highly engaging user experiences and lifelike conversational interactions.
Amazon Kendra
Amazon Kendra is an intelligent search service powered by machine learning. Kendra reimagines enterprise search for your websites and applications so your employees and customers can easily find the content they are looking for, even when it’s scattered across multiple locations and content repositories within your organization.
AWS Connect
Amazon Connect is an easy to use omnichannel cloud contact center that helps you provide superior customer service at a lower cost. Over 10 years ago, Amazon’s retail business needed a contact center that would give our customers personal, dynamic, and natural experiences.
Get Started
Companies of all sizes across all industries are transforming their businesses every day using AWS. Contact our experts and start your own AWS Cloud journey today.