AWS IoT Greengrass ML Inference
AWS IoT Greengrass makes it easy to perform machine learning inference locally on devices, using models that are created, trained, and optimized in the cloud. AWS IoT Greengrass gives you the flexibility to use machine learning models trained in Amazon SageMaker or to bring your own pretrained model stored in Amazon S3.
Machine learning uses statistical algorithms that learn from existing data, a process called training, in order to make decisions about new data, a process called inference. During training, patterns and relationships in the data are identified to build a model. The model allows a system to make intelligent decisions about data it hasn’t encountered before. Optimizing models compresses the model size so it runs quickly. Training and optimizing machine learning models require massive computing resources, so it is a natural fit for the cloud. But, inference takes a lot less computing power and is often done in real-time when new data is available. Getting inference results with very low latency is important to ensure your IoT applications can respond quickly to local events.
AWS IoT Greengrass gives you the best of both worlds. You use machine learning models that are built, trained, and optimized in the cloud and run inference locally on devices. For example, you can build a predictive model in SageMaker for scene detection analysis, optimize it to run on any camera, and then deploy it to predict suspicious activity and send an alert. Data gathered from the inference running on AWS IoT Greengrass can be sent back to SageMaker where it can be tagged and used to continuously improve the quality of machine learning models.
Benefits
Flexible
Deploy models to your connected devices with a few clicks
Accelerate inference performance
Run inference on more devices
Easily run inference on connected devices
Build more accurate models
How it works
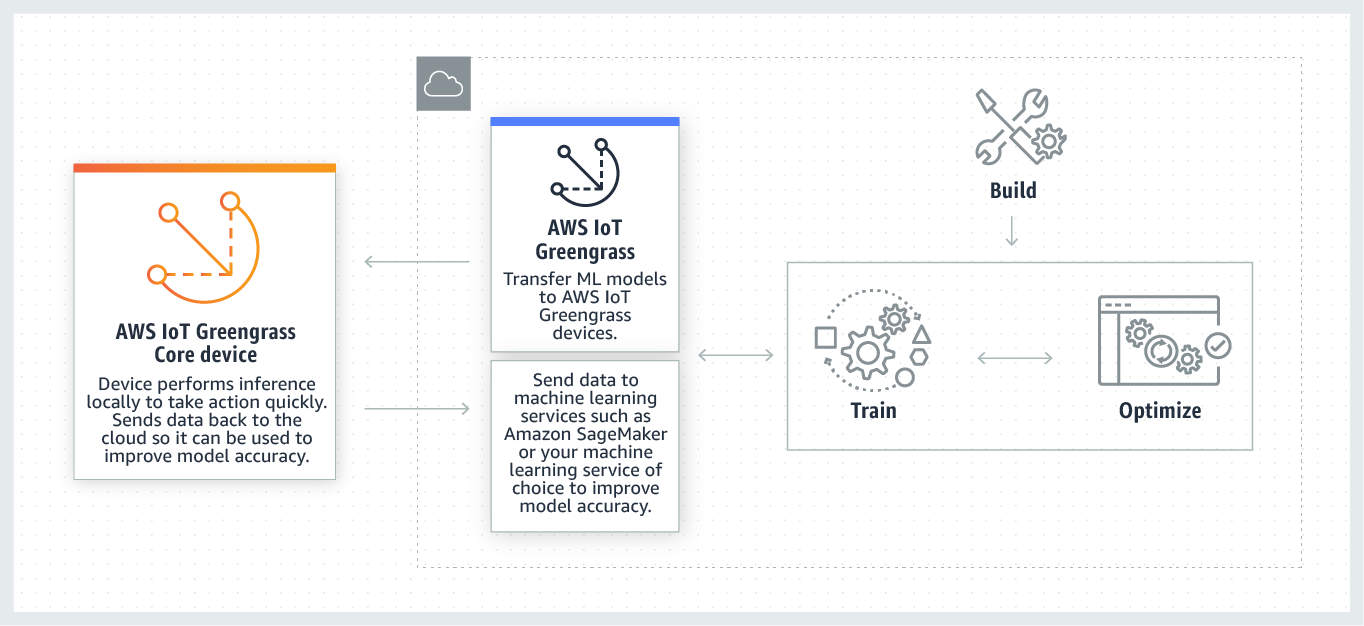
Use cases
Predictive industrial maintenance
Precision agriculture
Security
Retail and hospitality
Video processing
Featured Customers
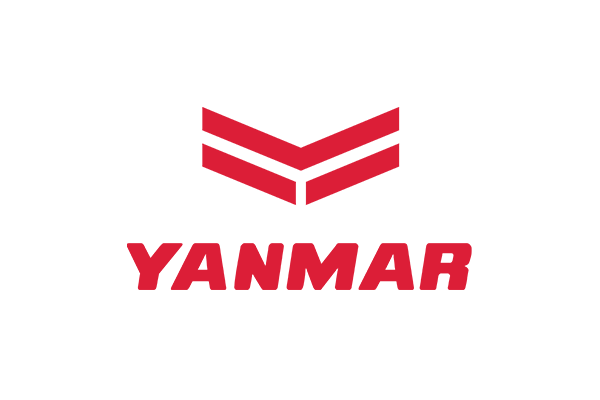
AWS IoT Greengrass helps Yanmar increase the intelligence of greenhouse operations by automatically detecting and recognizing the main growth stages of vegetables to grow more crops.
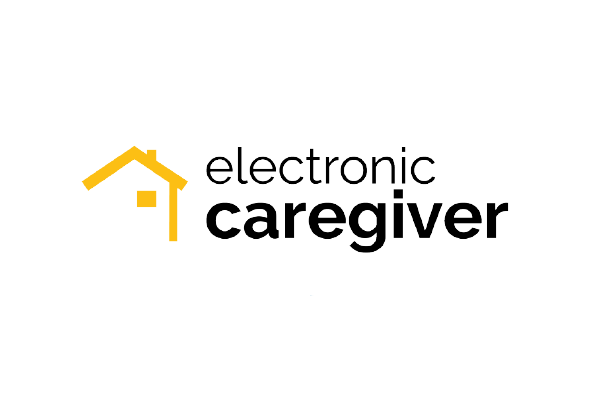
The Electronic Caregiver ensures high-quality caregiving with AWS IoT Greengrass ML Inference and can push machine learning models directly to edge devices and keep patients safer.
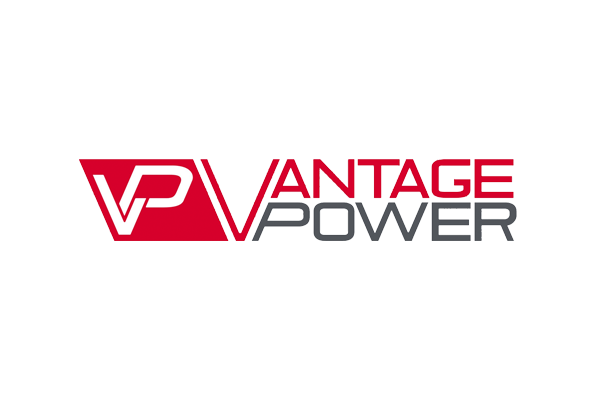
With AWS IoT Greengrass, Vantage Power pushes machine learning models to individual vehicles and detects battery faults 1 month earlier.
Featured partners
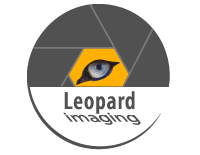
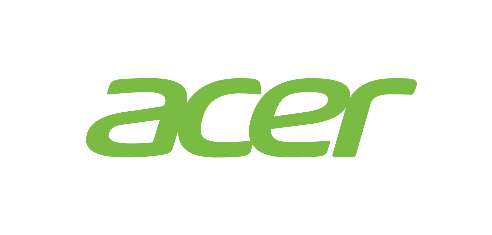
Learn more about AWS IoT Greengrass features