AWS and NFL Pull Back the Curtain on Defensive Strategy
Think like a NFL defensive coordinator with this new ML-powered Next Gen Stat that reveals the theory behind plays, bringing fans one step closer into the huddle
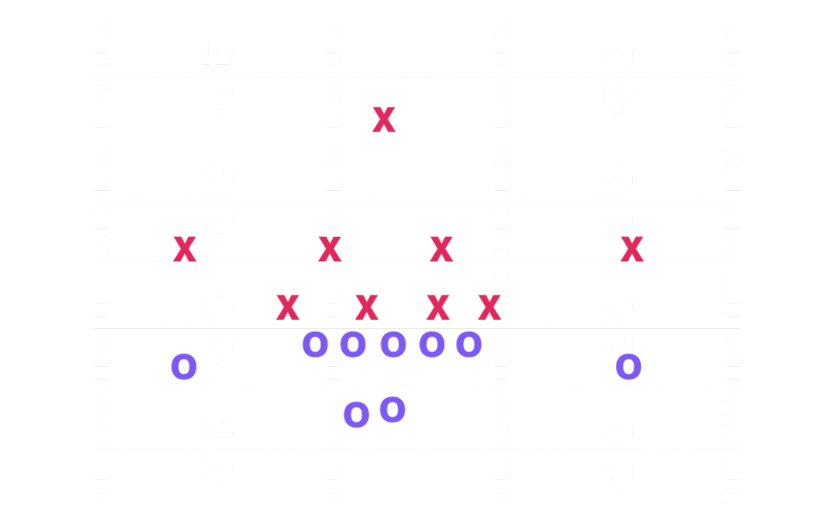
We’ve all been there: it’s Monday night. You’re watching your team play at the top of their game in a matchup you’ve been waiting for all season. The win is safely minutes away when, out of nowhere, your omniscient, impenetrable defense allows a scoring drive with a seemingly utter lack of coverage that makes us jump out of our seat looking for answers to the question, ‘how did that happen!?’
Beneath that disappointment is likely a reluctant understanding that the defense was outmaneuvered, perhaps weeks ago, in the mind of a prescient coach. Because the game of football is played on many levels: there’s the blood, sweat and tears on the field, of course, but there’s also the chess-like game of intellects, coaches versus coaches, using decades of experience, immense arsenals of data, and perfectly tuned players to orchestrate strategies informed by deep thinking and statistical analysis.
For fans, much of this game—the theoretical one—has remained invisible and out of reach. Video games and fantasy leagues chase the feeling of meaningful participation; while neither are “real,” their popularity speaks to a huge appetite for a deeper understanding of why games unfold the way they do.
So how do you give fans access to the theory and strategy—the “why”—behind what happens on the field between September and February? Historically, football stats have focused on the offensive side of the game, which makes sense. Scoring is compelling and a player’s value is clearly expressed in terms of how they contribute to a play. But as technology and fans have gotten smarter, an unmet need in statistical storytelling around defensive performance has become abundantly clear.
“There's always been a lacking ability to tell statistical stories about the defensive side of the ball,”
Mike Band, Next Gen Stats
-
COVER 0
-
COVER 1
-
MAN COVER 2
-
COVER 2
-
COVER 3
-
COVER 4
-
COVER 6
-
PREVENT
-
COVER 0
-
Coverage Type: Man
All-or-nothing man coverage with no deep safety. Each defender in coverage is responsible for an eligible receiver. Since 2018, defenses blitz on 90% of Cover 0 plays outside of the red zone; 62% of Cover 0 plays in the red zone.
-
COVER 1
-
Coverage Type: Man
A single-high centerfield safety with man coverage underneath. With a 4-man pass rush, an extra defender is free to spy the QB or bracket a top receiver.
-
MAN COVER 2
-
Coverage Type: Man
Two safeties split the deep area of the field in half (1/2) with man coverage underneath. If all five eligible receivers run a route, the defense can send a max of four rushers.
-
COVER 2
-
Coverage Type: Zone
Two safeties split the deep area of the field in half (1/2), while the remaining coverage defenders occupy five underneath zones. CBs are responsible for the flats; Slot defenders are responsible for hook/curl zones; MLB is responsible for the middle hole zone.
-
COVER 3
-
Coverage Type: Zone
Three deep defenders split the backend of the defense into thirds (1/3), while the remaining coverage defenders occupy three or four zones, depending on if the defense blitzes.
-
COVER 4
-
Coverage Type: Zone
Also known as “Quarters” coverage. Four defenders are responsible for each deep quarter (1/4) of the field, while the remaining coverage defenders occupy three underneath zones.
-
COVER 6
-
Coverage Type: Zone
Also known as “Quarter-Quarter-Half” coverage. One side of the defense is occupied by two deep safeties, while the other half is occupied by one deep safety. The remaining coverage defenders occupy underneath zones based on the receiver strength.
-
PREVENT
-
Coverage Type: Prevent
A special coverage type saved for situations with little time remaining in a half. A number of coverage defenders align at extreme depths off the line of scrimmage, sacrificing underneath completions in order to prevent big plays & TDs.
"Going beyond just man or zone, a computational model powered by AI- and machine learning (ML) is able to make a diagnosis in fractions of a second with stunning accuracy."
The computational neural network model is able to look at each image in each frame, understand the relationship within each frame, and is able to track how those relationships change over time.
Behind this work is the idea that stats can take fans beyond the “what” of the game and transport you into the world of “why.” They add color and detail to the fan experience, make it more immersive, and open opportunities to feel like participant in the action. The energy and excitement that draws millions in every year becomes that much closer and more real the more you know, the more you can understand the reasons behind the action unfolding on the field. Without investing any additional time or effort into a game you already love, innovative stats like coverage classification can reveal the logic that goes into strategic decisions and give fans more information to create meaningful stories that weren’t visible before.
So as you tune in to watch this football season, go ahead and dig in to Next Gen Stats. Grow your expertise and watch as new stories about what’s happening on and off the field come to life before your eyes. You might end up impressing yourself—and your friends—with what you’re able to see when you can use data to get beyond “what” happens during a game and uncover the “why.” At the very least, it might soften the blow when your defense suffers a surprise scoring drive.