What is Amazon SageMaker Model Deployment?
Amazon SageMaker makes it easy to deploy ML models including foundation models (FMs) to make inference requests at the best price-performance for any use case. From low latency (a few milliseconds) and high throughput (millions of transactions per second) to long-running inference for use cases such as natural language processing and computer vision, you can use SageMaker for all your inference needs. SageMaker is a fully managed service and integrates with MLOps tools, so you can scale your model deployment, reduce inference cost, manage models more effectively in production, and reduce operational burden.
Benefits of SageMaker Model Deployment
Wide range of options for every use case
Broad range of inference options
From low latency (a few milliseconds) and high throughput (millions of transactions per second) to long-running inference for use cases such as natural language processing and computer vision, you can use SageMaker for all your inference needs.
Real-Time Inference
Low latency and ultra-high throughput for use cases with steady traffic patterns.
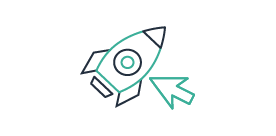
Serverless Inference
Low latency and high throughput for use cases with intermittent traffic patterns.
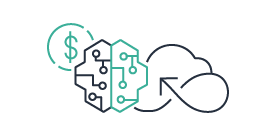
Asynchronous Inference
Low latency for use cases with large payloads (up to 1 GB) or long processing times (up to 15 minutes).
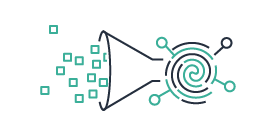
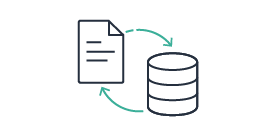
Scalable and cost-effective deployment options
Amazon SageMaker provides scalable and cost-effective ways to deploy large numbers of ML models. With SageMaker’s multiple models on a single endpoint, you can deploy thousands of models on shared infrastructure, improving cost-effectiveness while providing the flexibility to use models as often as you need them. Multiple models on a single endpoint support both CPU and GPU instance types, allowing you to reduce inference cost by up to 50%
Single-model endpoints
One model on a container hosted on dedicated instances or serverless for low latency and high throughput.
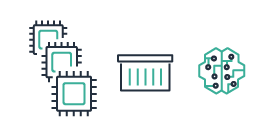
Multiple models on a single endpoint
Host multiple models to the same instance to better utilize the underlying accelerators, reducing deployment costs by up to 50%. You can control scaling policies for each FM separately, making it easier to adapt to model usage patterns while optimizing infrastructure costs.
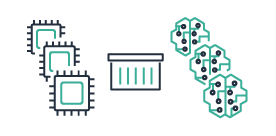
Serial inference pipelines
Multiple containers sharing dedicated instances and executing in a sequence. You can use an inference pipeline to combine preprocessing, predictions, and post-processing data science tasks.
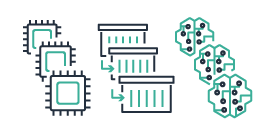
Support for most machine learning frameworks and model servers
Amazon SageMaker inference supports built-in algorithms and prebuilt Docker images for some of the most common machine learning frameworks such as TensorFlow, PyTorch, ONNX, and XGBoost. If none of the pre-built Docker images serve your needs, you can build your own container for use with CPU backed multi-model endpoints. SageMaker inference supports most popular model servers such as TensorFlow Serving, TorchServe, NVIDIA Triton, AWS multi-model server.
Amazon SageMaker offers specialized deep learning containers (DLCs), libraries, and tooling for model parallelism and large model inference (LMI), to help you improve performance of foundational models. With these options, you can deploy models including foundation models (FMs) quickly for virtually any use case.
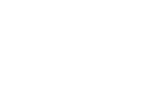
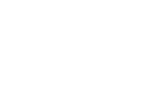
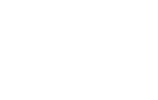
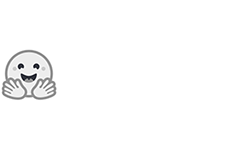
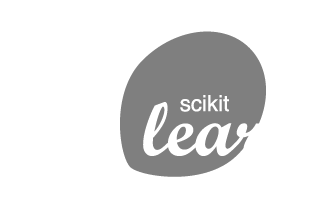
Achieve high inference performance at low cost
Deploy models on the most high-performing infrastructure or go serverless
Amazon SageMaker offers more than 70 instance types with varying levels of compute and memory, including Amazon EC2 Inf1 instances based on AWS Inferentia, high-performance ML inference chips designed and built by AWS, and GPU instances such as Amazon EC2 G4dn. Or, choose Amazon SageMaker Serverless Inference to easily scale to thousands of models per endpoint, millions of transactions per second (TPS) throughput, and sub10 millisecond overhead latencies.
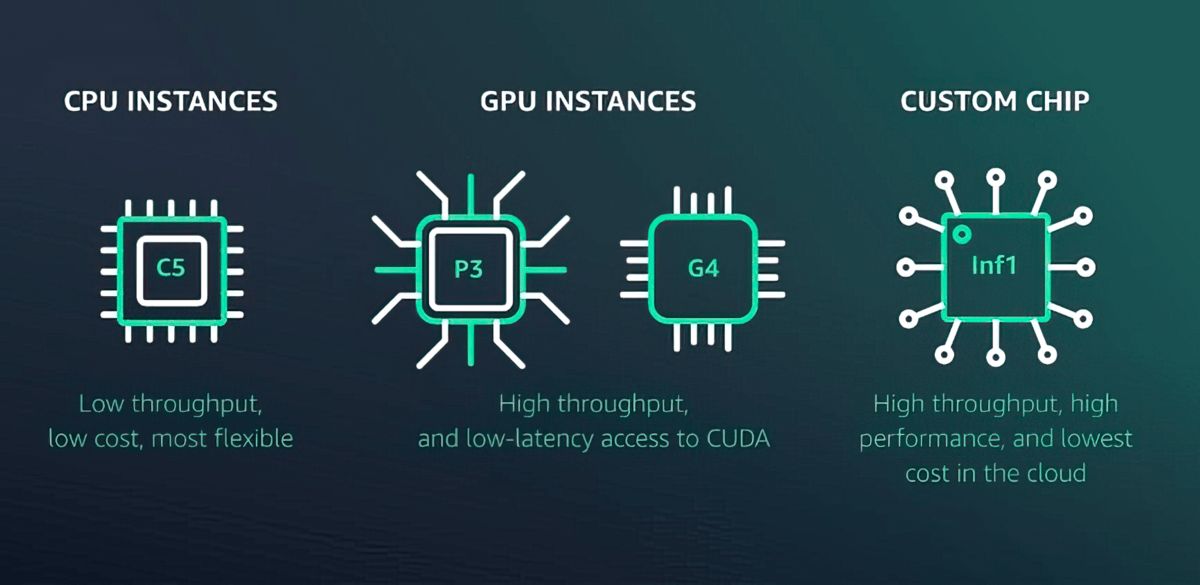
Shadow test to validate performance of ML models
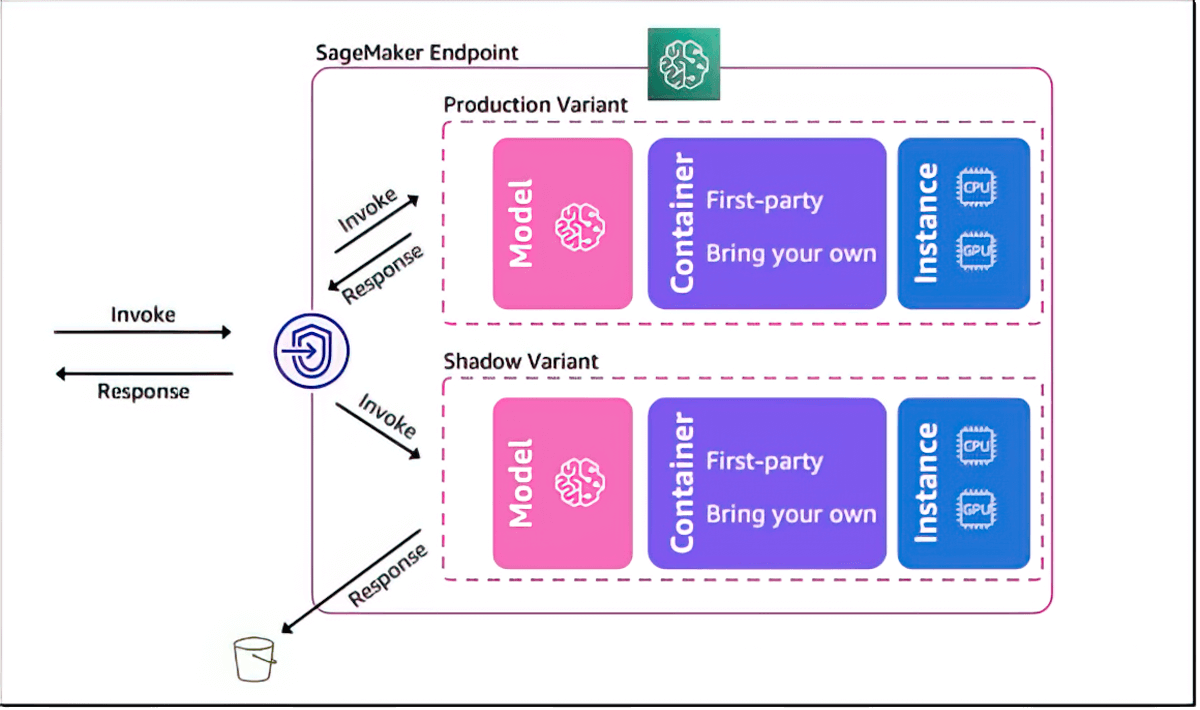
Automatic inference instance selection and load testing
Amazon SageMaker Inference Recommender helps you choose the best available compute instance and configuration to sagemaker-deploy machine learning models for optimal inference performance and cost. SageMaker Inference Recommender automatically selects the compute instance type, instance count, container parameters, and model optimizations for inference to maximize performance and minimize cost.
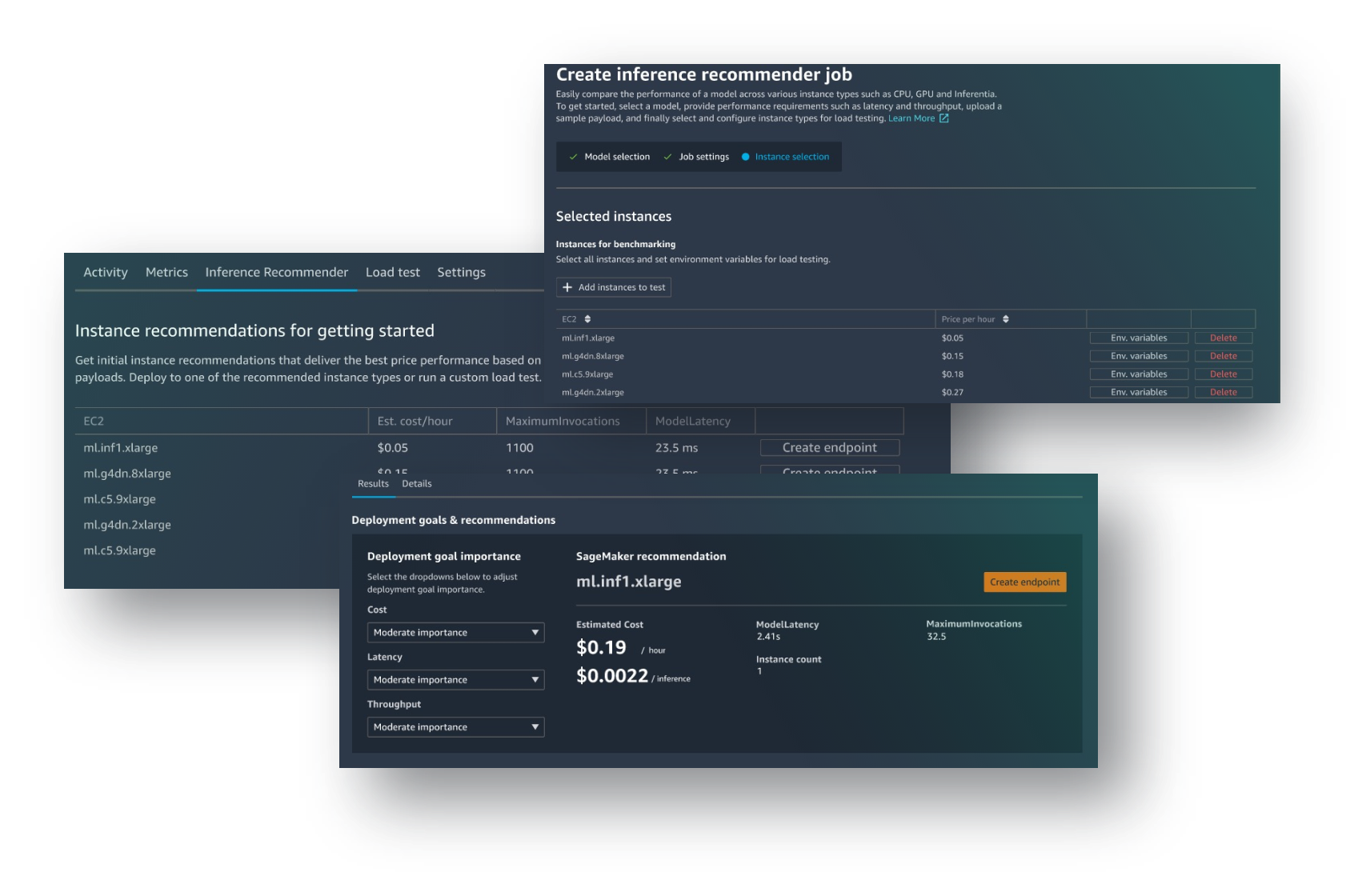
Autoscaling for elasticity
You can use scaling policies to automatically scale the underlying compute resources to accommodate fluctuations in inference requests. You can control scaling policies for each ML model separately to handle the changes in model usage easily, while also optimizing infrastructure costs.
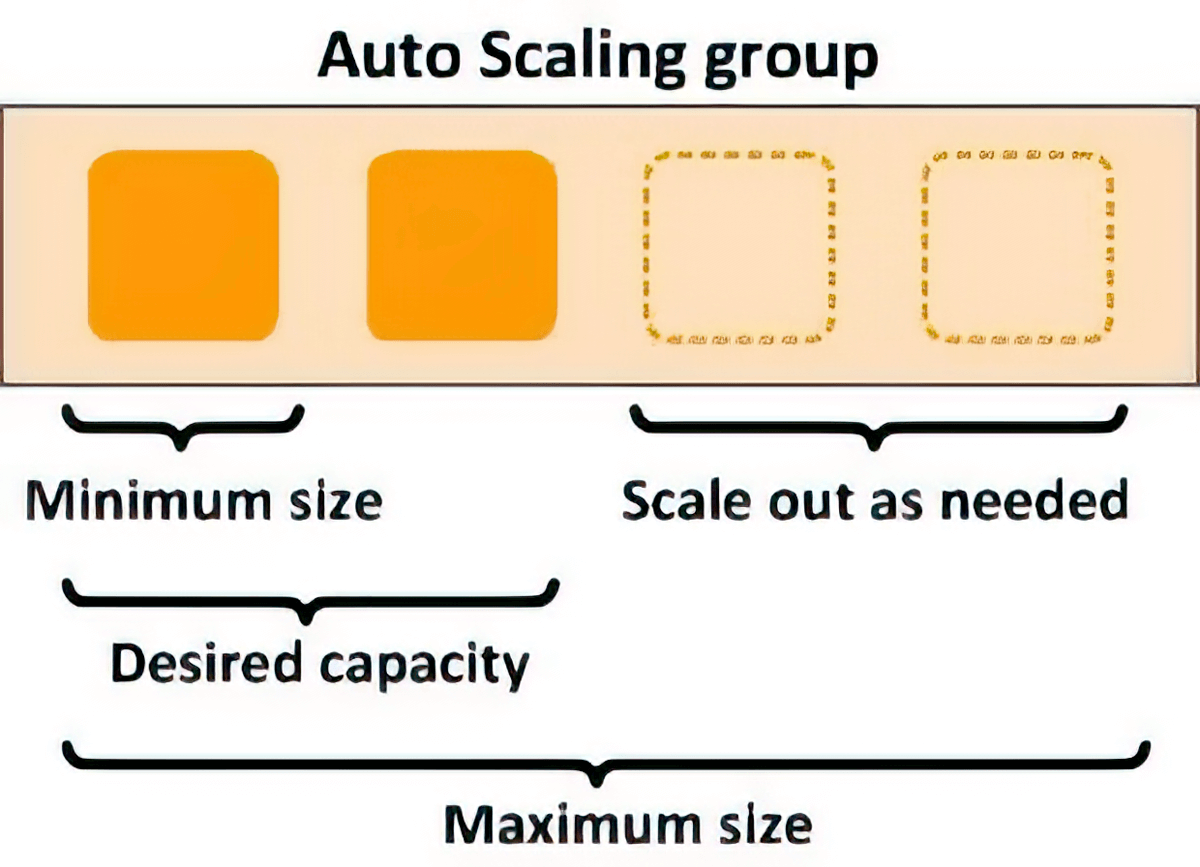
Latency improvement and Intelligent routing
You can reduce inference latency for ML models by intelligently routing new inference requests to instances that are available instead of randomly routing requests to instances that are already busy serving inference requests, allowing you to achieve 20% lower inference latency on average.
Reduce operational burden and accelerate time to value
Fully managed model hosting and management
As a fully managed service, Amazon SageMaker takes care of setting up and managing instances, software version compatibilities, and patching versions. It also provides built-in metrics and logs for endpoints that you can use to monitor and receive alerts.
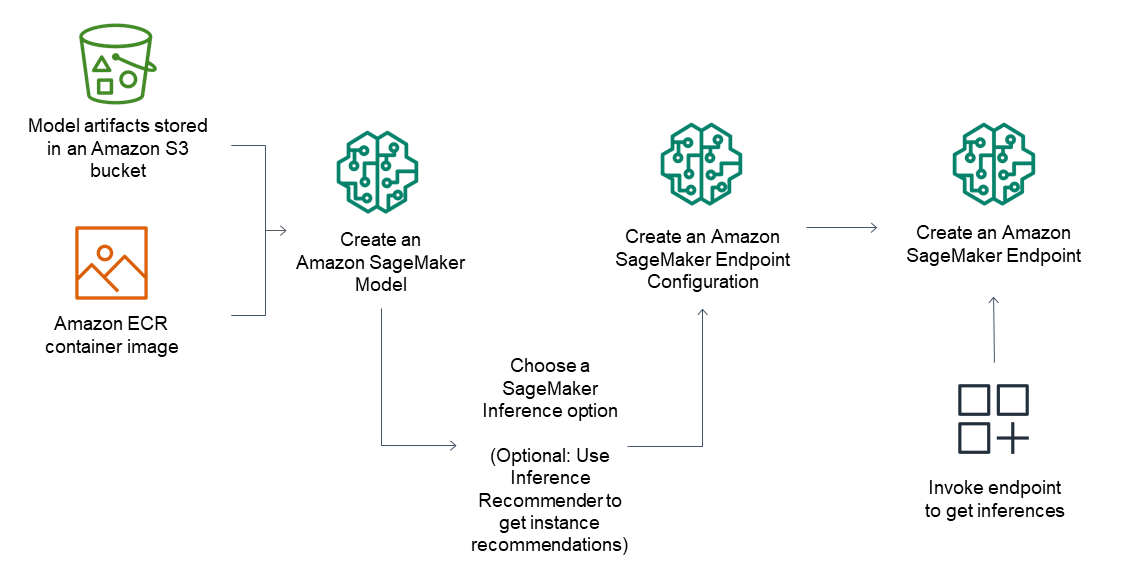
Built-in integration with MLOps features
Amazon SageMaker model deployment features are natively integrated with MLOps capabilities, including SageMaker Pipelines (workflow automation and orchestration), SageMaker Projects (CI/CD for ML), SageMaker Feature Store (feature management), SageMaker Model Registry (model and artifact catalog to track lineage and support automated approval workflows), SageMaker Clarify (bias detection), and SageMaker Model Monitor (model and concept drift detection). As a result, whether you sagemaker-deploy one model or tens of thousands, SageMaker helps off-load the operational overhead of sagemaker-deploying, scaling, and managing ML models while getting them to production faster.
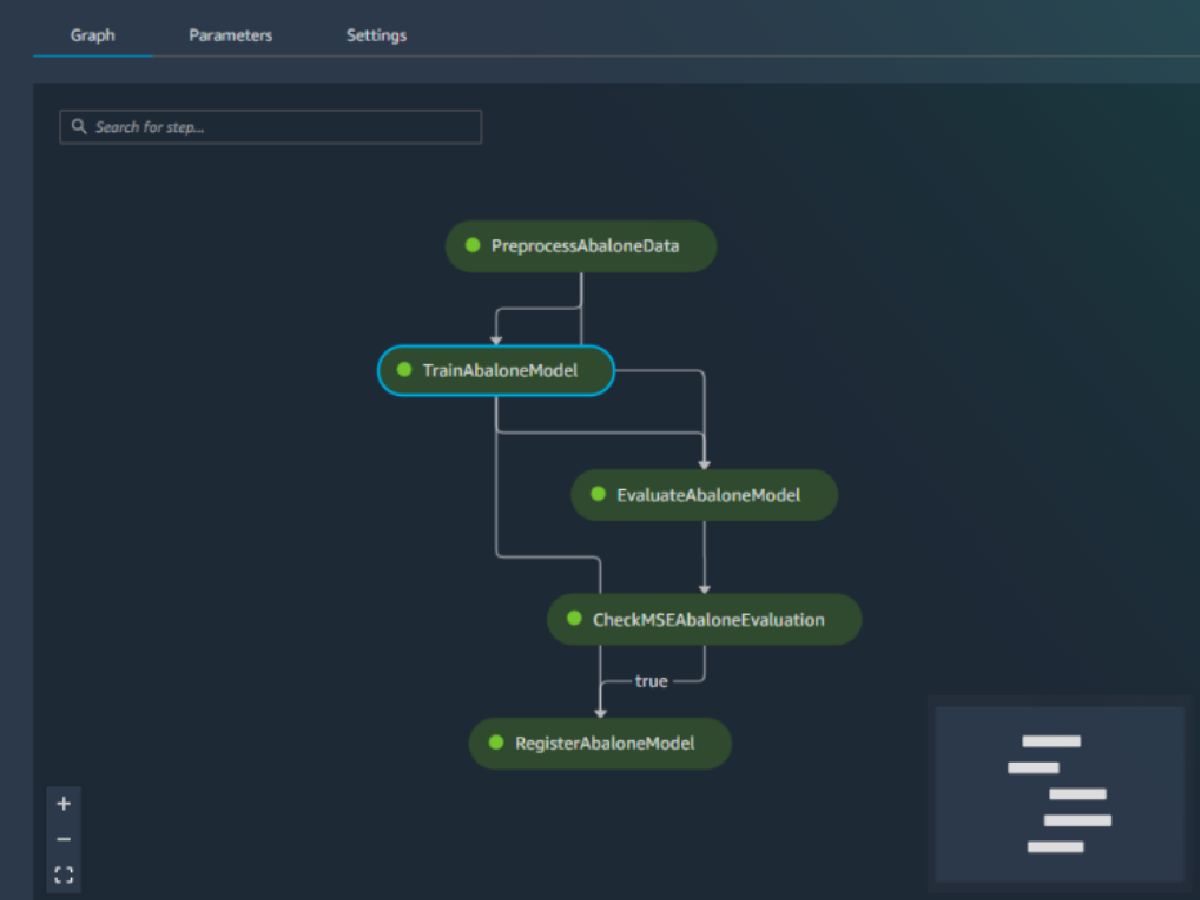