What is a data strategy?
A data strategy is a long-term plan that defines the technology, processes, people, and rules required to manage an organization's information assets. All types of businesses collect large amounts of raw data today. However, they need a well-thought-out data management and analytics plan if they want to use this information to make informed decisions and create machine learning (ML) or generative artificial intelligence (AI) applications. A data strategy outlines an organization's long-term vision for collecting, storing, sharing, and usage of its data. It makes working with data easier to do at every step of the data journey for everyone who needs it in your organization.
Why is a data strategy important?
Building a data strategy is essential for organizations to stay relevant, competitive, and innovative amidst constant change. You must curate, understand, and protect your data to meet the goals of business initiatives and unlock new value for your organization, such as the following:
- Make better, faster decisions
- Reduce fraud
- Improve customer experience and loyalty
- Increase operational efficiency
- Optimize processes
- Optimize costs
- Support new business models, like data as a product
- Build future-proof applications, like machine learning (ML) or generative AI.
Your data strategy gives you a competitive advantage because it aligns data management with business strategy and data governance. It has two primary purposes.
Improve data architecture decisions
A company's data architecture describes how the company collects, stores, transforms, distributes, and consumes data. It also includes the technical aspects of data management, such as the following:
- Databases and file systems
- Data integration services
- Rules governing data storage formats
- System connections between applications and databases
- Data access rights
- AI data models
For example, data architecture might input daily marketing and sales data into applications like marketing dashboards, which then further integrate and analyze the information to reveal relationships between ad spend and sales by region. Your data strategy provides the framework within which data engineers make architectural decisions that meet business goals, while making sure that the right people have access to that data.
Manage data consistently
An effective data strategy supports the entire organization for collaborative and consistent data management. It gives everyone the answers to five key questions:
- What data is appropriate?
- What data operations are approved?
- What is the purpose of data storage and collection?
- What is the data governance policy for business processes?
- What insights can you get from your existing data?
What are the advantages of implementing a data strategy?
There are more benefits of having a good data strategy:
Solve data management challenges
Most organizations experience data management challenges like data silos, data duplication across business units, inefficient data flow between departments, and lack of clarity around data priorities. A data strategy allows companies to solve these challenges by making data accessible and shared in a secure way. You can unlock the value of data to meet business initiatives. Better alignment around data and access to the right data at the right time enables organizations to prepare for the future or unknown.
Improve customer experience and loyalty
Organizations use data and analytics to better understand customers and improve customer experience. From online experiences to contact centers, organizations can use data to create more value for customers and address unmet needs proactively. Data also helps organizations create new business or monetization opportunities and build hyper-personalized products and services based on customer needs. Personalized experiences also bolster customer loyalty over time.
Attain analytical maturity
The Gartner Analytic Ascendancy Model defines four steps in analytical maturity. Organizations typically start with descriptive and diagnostic analytics to understand what happened and why. Analytical maturity comes when the organization transitions to predictive analytics that use data to answer what will happen. Organizations in the final stage of maturity use prescriptive analytics to achieve predetermined results. A data strategy thus lays out a detailed plan to help your organization move from making decisions based on foresight instead of hindsight.
Build future-proof applications, like ML and generative AI
Data is at the core of ML and generative AI applications. ML and AI models require the ability to ingest and manage data easily to train models and run inference. A data strategy accounts for data that feeds use cases like image recognition, forecasting, and intelligent search to applications. You also need to account for ML governance, which includes governing your data models.
Create an organization-wide data culture
A data strategy presents a roadmap to improve data literacy and efficiency in usage across the organization. Diverse teams can work in alignment to enhance data quality and the accuracy of data collection. In addition, you can develop customized training and create learning pathways for collaborators to go from beginners to experts in data management and usage.
Support regulatory compliance
An effective data strategy improves data security by implementing measures to limit unauthorized data access. You can consider all data governance rules and regulations while defining policies and processes. All operations can be carefully planned to ensure enterprise data management maintains the privacy, security, and integrity of data at all times.
What are the key components of an effective data strategy?
You can present your data strategy as a sequence of steps and a timeline to implement these steps. This data strategy roadmap includes guidelines to maintain your organization's current data maturity and action items that take it to the next level.
The following are some common data strategy components to include in your roadmap:
Data catalog tools
Data catalog tools help you identify and categorize all your existing data assets. Your business users and IT teams can use the catalog for detailed metadata, as well as to map business operations to data operations more effectively.
Data management tools
Several tools exist for data integration, visualization, reporting, and dashboards. A data strategy helps identify the best tools that meet business needs and support both IT teams and business users. You can also verify that the tools meet all data governance policies, ensuring compliance with regulations.
Data analytics
Successful data strategies typically include plans for both data and analytics management within an organization. Data analysis requires existing datasets as input for ML and AI models. An enterprise data strategy aims to minimize bias by outlining the best datasets to use for analytics and how to train employees in data operations. For example, suppose your organization plans to use AI to sort job applications automatically. In that case, you will need to carefully select a diverse dataset of past and present employees to avoid creating unconscious bias in the ML and AI models.
Review process
Your data strategy should include a review process to assess and improve existing data management systems and the data strategy itself. This includes tasks like the following:
- Periodically auditing existing data architecture
- Verifying that data collection processes remain compliant
- Measuring data quality against comparable market data
You can use such review documentation to improve your existing data strategy and revisit strategic goals.
What are the different approaches to creating a data strategy?
There are two main approaches to creating a data strategy for your organization.
Centralized
A highly centralized, control-oriented approach to data management typically includes a single source of truth for every broad data category. For example, there is one primary source of revenue, customer, or sales data. The data systems gather data from several sources, clean it, and store it in this central repository. Data defense thus minimizes downstream risk by identifying, standardizing, and governing authoritative data sources to maintain the integrity of the data flowing through the company's internal systems. It prioritizes activities, such as the following:
- Compliance and regulations
- Fraud detection using analytics
- Security measures for theft prevention
Decentralized
A decentralized approach adds more flexibility to centrally governed data management systems. It recognizes that multiple business units interpret the same data differently. It accommodates those different interpretations by permitting controlled data transformations that can be reliably mapped back to the single source of truth.
For example, consider a scenario where both the finance and marketing departments produce monthly social media ad spending reports. Marketing, interested in analyzing ad effectiveness, reports on the impact of spending on clicks and views. Finance reports on the effect of spending on cash flow. The reports contain different numbers, but both reports represent an accurate version of the truth.
Balance data strategy approaches
Every company needs to incorporate both centralized and decentralized approaches for its data strategy to succeed, but getting the balance right can be complicated. Decentralized approaches tend to be real-time operations and are more relevant for customer-focused business functions, such as marketing and sales. Centralized approaches are more important for legal, financial, compliance, and IT departments. A balanced data strategy gives business leaders the flexibility to adapt the single source of truth in consistent ways to better meet business needs.
Who builds a data strategy?
The data strategy team typically includes representatives from upper management, business analytics, AI, and IT teams. The following are some examples of users who come together to create and implement a data strategy.
Data engineers
Data engineers are responsible for building a reliable and efficient data architecture. They oversee and administer several data pipeline tasks like data collection, processing, storage, and analytics. This role includes specialists who implement data security and governance requirements.
Data scientists
Data scientists take the data processed by data engineers and use it for further analysis. They use the data to create different ML and AI models and generate reports for business intelligence.
Data analysts
Data analysts specialize in interpreting and analyzing data. They work closely with data scientists to ensure that business intelligence tasks align with organizational requirements.
Business managers
Business managers review data reports and help manage data operations. They ensure data strategy aligns with overall business strategy and regulatory requirements.
What are the steps to building a data strategy?
Identify funded business initiatives
The first step is to align to funded business initiatives. For this, you can use Amazon’s working backwards methodology. Next, determine which data is needed for those business initiatives. Then identify which data capabilities are necessary to support the business initiatives.
Here are example steps for building a data strategy:
- Support someone else’s funded business initiative
- Identify required data capabilities
- Determine the condition of the data needed
- Build a data strategy roadmap
- Identify which enterprise operating practices, like enterprise architecture and project management, can support your data strategy
- Integrate with AI strategy programs
Build a team
Finding the right people who bring a diverse range of viewpoints is crucial for success in data strategy. Your team will be responsible for several tasks, which may include the following:
- Resource allocation and distribution
- Establishing and improving policies
- Dealing with data-related issues as they arise
- Communicating program status and results
You can also assign data governance roles for determining who is responsible for deploying technologies, ensuring compliance with standards, and providing updates to everyone about policy changes.
Optimize your data architecture
Any data strategy requires the right tools and technologies to succeed practically. You will need to review your existing data infrastructure, analyze how different teams currently use data, and identify any gaps to be resolved. This step typically involves making technology-centric decisions based on your requirements, which may include the following:
- Data volume and type
- Data quality and analysis
- Security and compliance
- Data lifecycle
Ultimately, your goal is to create a data strategy that makes your data as accessible, shareable, and actionable as possible for all stakeholders who need it, with the right security controls in place.
Integrate with AI strategy program
To produce value from AI and ML, the underlying data must meet the needs of the specific initiatives associated with AI and ML models to ensure appropriate quality of the data, integration, security, and so on. Therefore, there should be a partnership between the data strategy and AI strategy teams.
Special governance considerations for AI/ML
AI/ML does introduce new capabilities that we need to account for in our data strategy. For example:
- Feature stores
- Additional regulatory compliance
- MLOps
- New ethical considerations
- Generative AI considerations
How can AWS help with your data strategy?
AWS has several services that help you reinvent your business with data. You can join over 1.5 million customers in bringing your data to the world's most trusted, secure, and scalable cloud community. For example, you can use AWS to do the following:
- Discover how to maximize the business value of your data by implementing an end-to-end data strategy with AWS with this eBook
- In this on-demand master class, learn how to build a successful and funded data governance program. The Data Governance with AWS master class videos are accompanied by a workbook with hands-on exercises.
- Upgrade existing systems with AWS Data Infrastructure Modernization services
- Select and implement the best data analytics strategies with Analytics on AWS
- Invent new experiences and reimagine old processes with Machine Learning on AWS
Get started implementing your data strategy using AWS by creating a free account today.
Next steps on AWS
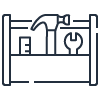
Get started building with Data Strategy in the AWS management console.