Discover why nine out of the top 10 pharma companies choose AWS for data analytics and machine learning.
New! Generative AI in Healthcare and Life Sciences resource hub
From generating new therapeutic candidates, to better matching patients with the right clinical trials, to powering patient engagement applications, AWS makes it easier to access the services, data, models, and secure infrastructure needed to scale generative AI across your organization. Learn how generative AI can accelerate health innovations and improve patient experiences.
Accelerate time-to-insights, increase ROI, and bring differentiated therapeutics to market faster with the most comprehensive suite of data capabilities and machine learning offerings—all available on the most secure, most extensive global infrastructure.
For more than a decade, AWS has helped life sciences leaders solve for some of the most complex industry challenges—from helping the US Food and Drug Administration (FDA) first digitize manual reporting, to providing researcher access to the UK Biobank, to powering Moderna in developing and scaling its COVID-19 vaccine.
Whether you want to build digitally connected labs, accelerate protein folding with machine learning, extract insights from your enterprise data using generative AI, or automate GxP compliance, AWS’ team of life sciences experts can help you find the right solutions to take your business to the next level.
Accelerate access to and insights from your 1st party, 3rd party, and multi-modal data with an end-to-end data strategy powered by AWS Health for Data.
Leading life science organizations report that investments in AWS Cloud technology opens new doors for operational efficiencies, and help them increase ROI while accelerating time to market (report).
AWS makes it’s easier to partner, collaborate, access industry datasets, and implement the latest advancements like generative AI all within the most secure, extensive cloud infrastructure.
Bring differentiated therapeutics to market faster
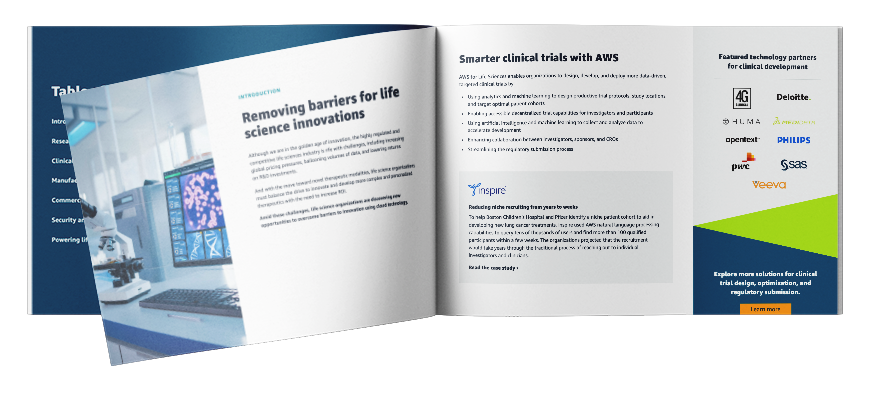
AWS for Life Sciences: purpose-built industry capabilities and solutions
AWS for Life Sciences reduces barriers to innovation by providing a curated suite of purpose-built solutions designed to help organizations develop, trial, manufacture, and commercialize therapeutics quickly and efficiently—all while meeting stringent security and compliance regulations.
Research and development
Advance drug candidates into development faster using on-demand, cost-effective, high-performance computing and AI/ML capabilities on the most extensive cloud platform. Facilitate secure research collaborations between your global teams and simplify data movement from lab equipment to integrate research environments, all while remaining compliant.
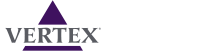
Vertex Pharmaceuticals reduces costs of cryo-em data storage and processing by 50% using AWS.
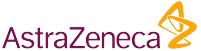
AstraZeneca uses AWS in reimagining its drug discovery process to develop medicines more quickly, cost effectively, and with a higher probability of success.
Using structural variant analysis on AWS for novel therapeutic discovery
Accelerating R&D with disruptive technology at Janssen
Predicting protein structures at scale using AWS Batch
Accelerating drug discovery through knowledge graph
Clinical development
Power the entire clinical development process—from more effective and inclusive trials to streamlining the regulatory submission process. AWS and AWS Partner solutions help securely capture and extract insights from the massive amounts of data generated during clinical trials, facilitate decentralized trials, and effectively collaborate between stakeholders.
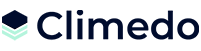
Climedo Health captures patient-centric, compliant, and secure clinical data using AWS
Streamlining clinical trial submissions in the cloud
Transforming site monitoring in clinical trials
Deploy digital biomarkers at scale in clinical trials using serverless services on AWS
Improving patient engagement in clinical trials using voice and chat
Manufacturing and supply chain
Modernize manufacturing processes by breaking down data silos, generating insights, and utilizing more cost-effective infrastructure, while supporting regulatory compliance. AWS delivers the services you need for visibility into shop-floor data and improved data liquidity throughout the supply chain. Gain efficiencies and reduce costs through meaningful insights and the ability to forecast product demand and production.
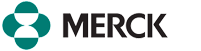
Merck’s manufacturing data and analytics platform triples performance and reduces data costs by 50% on AWS
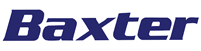
Baxter improves operational efficiency with predictive maintenance using Amazon Monitron
Aizon improves pharmaceutical manufacturing with artificial intelligence using AWS
Setting the “Industry 4.0” foundations for life sciences manufacturing
Rapidly scaling manufacturing at Moderna
Machine learning for computer vision in pharmaceutical manufacturing
Commercial and medical affairs
Accelerate real-world evidence collection and management, deliver more personalized engagements, integrate digital therapies, and enhance on-market surveillance with AWS services. AWS for Life Sciences provides a curated portfolio of AWS and AWS Partner solutions to help with everything from optimizing a salesforce, to detecting adverse events through complex analysis.
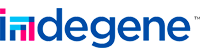
Indegene reduces adverse-event reporting time for its clients by 80% using AWS
Expanding access to digital therapeutics with Omada Health
How analytics from Infosys Life Science Insights Platform improves patient outcomes
Onboarding data to a healthcare and life sciences data mesh leveraging AWS services
Genentech maximizes the value of clinical biomarker data using AWS
Compliance
Achieve near continuous compliance for GxP-regulated workloads through AWS services that allow you to automate compliance checks and provide enhanced management and traceability. AWS provides the tools, guidance, and industry experts to help you comply with GxP, HIPAA, HITRUST, and GDPR standards.
Enabling enterprise-wide analytics and ML with automated compliance
Whitepaper: Navigating regulatory and compliance requirements for HCLS on AWS
Whitepaper: GxP Systems on AWS
Life sciences compliance in the cloud
5 Ways to Reduce Costs and Support Innovation
Learn how leading life sciences organizations like Gilead, Genentech, Baxter, and AstraZeneca leverage AWS to:
- Reduce and optimize operational costs
- Increase staff productivity
- Bring products to market faster
- Gain greater visibility enterprise-wide
- Minimize their environmental impact
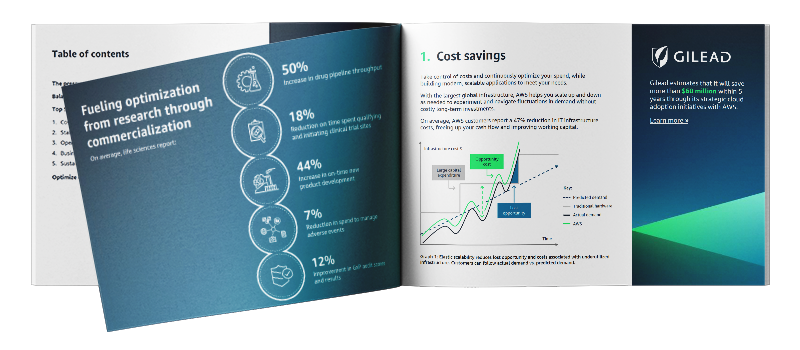
Innovate with key industry partners
Discover purpose-built life sciences solutions and services from an extensive network of industry-leading AWS Partners who have demonstrated technical expertise and customer success in building solutions on AWS.
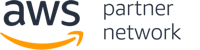
AWS offers the largest network of partners, along with flexible workflow choice, and options for fully managed solutions to help you get to life sciences insights faster.

Accelerate life sciences innovation, discovery, and development with third-party purpose built solutions.
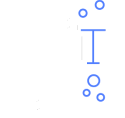