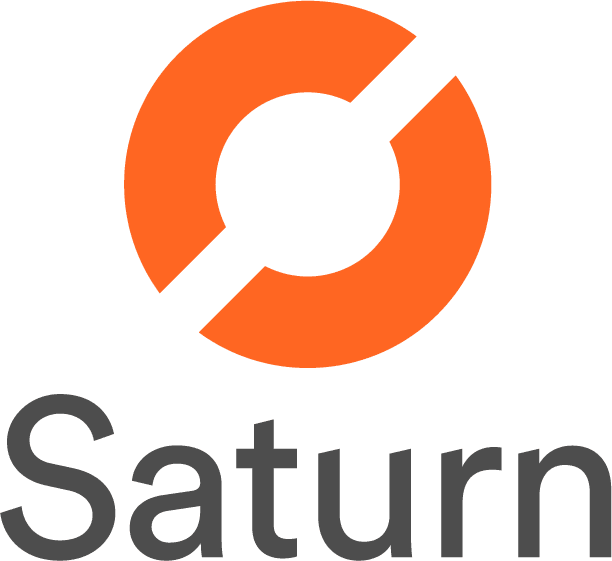
External reviews

External reviews are not included in the AWS star rating for the product.
Saturn Cloud
What do you like best about the product?
150 hours free computing rsources monthly
What do you dislike about the product?
Reinstallation of python packages after machine restart.
What problems is the product solving and how is that benefiting you?
Limited computing resource in local machine
- Leave a Comment |
- Mark review as helpful
awesome cloud
What do you like best about the product?
convenience, It has good specifications.
What do you dislike about the product?
nothing else. here are no shortcomings discovered up to the present moment.
What problems is the product solving and how is that benefiting you?
cool GPU resource
Saturn for AI use as a student
What do you like best about the product?
Super useful. Tons of server options, servers are fast and come with 150 free hours. Really nice fore quickly deploying something.
What do you dislike about the product?
The start time. It can take a while to start the server.
What problems is the product solving and how is that benefiting you?
It allows be to easily run my models overnight. It is faster than my current PC and also allows me to run it in parallel.
best
What do you like best about the product?
higher storage and gpu, helps in running code for longer
What do you dislike about the product?
smooth operation and easy to use interface
What problems is the product solving and how is that benefiting you?
none,
Great for AI training
What do you like best about the product?
I love the free GPUs. It has allowed for me and my team to efficiently train our AI model. We can create parallel instances to efficiently test new hyperparams and model ideas.
What do you dislike about the product?
Nothing too concerning, it can take up to 15 minutes to start the instance though.
What problems is the product solving and how is that benefiting you?
We are able to run our machine learning model on powerful GPUs in parallel.
Saturn Cloud Jupyter Server usage
What do you like best about the product?
Easy setup, fast provisioning of VMs, plenty of available configuration options.
What do you dislike about the product?
No downsides experienced using Saturn Cloud so far.
What problems is the product solving and how is that benefiting you?
Quick access to larger GPUs for model training.
Its been a very useful platform in my GPU computation!
What do you like best about the product?
Very easy to use, quite efficient, very nice and professional support
What do you dislike about the product?
It could be a little bit more user-friendly, but overall, it gives the user what ever is necessary
What problems is the product solving and how is that benefiting you?
Saturn addresses high performance computing problems, and it helps me run big Machine Learning and Deep Learning model that needs high GPU performance
An Invaluable Tool for Model training and Streamlining Data Science Workflows
What do you like best about the product?
I mainly use this site to train my LORA and models for stable diffusion to create images from text. Saturn Cloud has transformed my data science journey. It simplifies my workflow, grants quick access to my preferred tools, and effortlessly scales as needed. It's a lifesaver for small teams, with the ability to create clusters effortlessly.
What do you dislike about the product?
There is nothing that I dislike about the Saturn cloud
What problems is the product solving and how is that benefiting you?
I mainly use this site to train my LORA and models for stable diffusion to create images from text. it is easy to do autotrain dreambooth training save me a lot of money to spend in other services
One of the best machine learning platform in the Internet
What do you like best about the product?
Ease of Use. new users can use the platform promptly without any hassle.
What do you dislike about the product?
For the free user, the usability has great limitation than paid user.
What problems is the product solving and how is that benefiting you?
Saturn Cloud solves the platform issues for running AI Image generation
150 Hours Free Every Month with Saturn Cloud
What do you like best about the product?
Generous Offering with 150 Free Hours: One of the major highlights of Saturn Cloud is the 150 free hours they provide. This is not just a great introduction for newcomers but also a testament to their confidence in the quality of their service. It allows users to get a genuine feel of the platform's capabilities without any initial investment.
What do you dislike about the product?
Free plan only litmit to 40GB, need maybe 50GB or 80GB
What problems is the product solving and how is that benefiting you?
Training AI model
showing 111 - 120