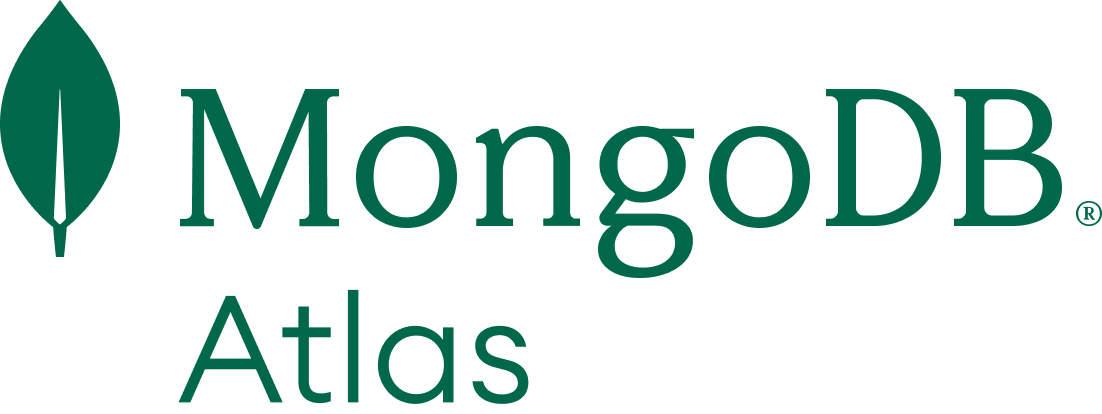
External reviews

External reviews are not included in the AWS star rating for the product.
Powerful and Scalable Database Solution with MongoDB Atlas
As a developer, I’ve had the opportunity to work with various database solutions, and MongoDB Atlas stands out as one of the best managed database services available today. Here are my thoughts on why I highly recommend MongoDB Atlas, especially for users in the AWS ecosystem:
- Ease of Use and Quick Setup: Setting up MongoDB Atlas was a breeze. The integration with AWS was seamless, allowing me to deploy clusters in just a few clicks. The user-friendly web interface is intuitive, making it easy to manage databases without a steep learning curve.
- Scalability and Performance: One of the most impressive features of MongoDB Atlas is its ability to scale effortlessly. Whether you’re dealing with moderate traffic or a sudden spike in user requests, Atlas can automatically adjust resources to ensure optimal performance. The built-in auto-scaling feature is a game-changer for applications that experience fluctuating workloads.
- Global Distribution and High Availability: With MongoDB Atlas, I can deploy clusters across multiple regions, ensuring low-latency access for users around the globe. The built-in replication and failover mechanisms provide high availability, which is critical for mission-critical applications.
- Cost-Effective: For a managed service, MongoDB Atlas offers competitive pricing. The pay-as-you-go model allows us to only pay for what we use, making it suitable for startups and large enterprises alike.
- Leave a Comment |
- Mark review as helpful
Audio embedding resources
I’d like to suggest adding more resources on using audio embeddings with MongoDB's vector search. Additional guidance on best practices and examples would greatly benefit those looking to work with audio data in MongoDB.
Powerful and Flexible Database for Gen AI Projects, with Room for Onboarding Improvements
Creating Mentation, an AI-driven wellness assistant, was an enriching experience, and MongoDB supplied the foundation we required for effortlessly handling intricate and diverse data. By managing user interactions and emotional data as well as processing vector embeddings, MongoDB effortlessly fulfilled our requirements. Its adaptability and scalability proved essential, allowing us to broaden our project’s scope without having to repeatedly reconfigure the database.
Although the documentation is comprehensive and addresses various use cases, a concentrated, beginner-friendly crash course would have been immensely helpful—particularly for teams such as ours seeking to utilize AWS and Gen AI. Exploring the fundamentals of MongoDB, such as querying, vector indexing, and aggregation pipelines, prompted us to seek out external tutorials, especially to clarify information regarding vector indexing. At one stage, we came across contradictory data from these sources indicating that solely larger M10 clusters were capable of handling vector indexing, which resulted in additional testing and problem-solving.
Although there were some learning challenges, MongoDB demonstrated to be a robust solution for the requirements of our project. By providing a more efficient onboarding process—centered on key elements and better instructions for utilizing features such as vector indexing—MongoDB would become even more attainable for developers engaged with advanced technology. In general, we had a positive experience with MongoDB, and with some modifications, it could easily become the preferred choice for any developer venturing into Gen AI applications.
Improvement on Documentation
For my hackathon project, I chose MongoDB Atlas from AWS Marketplace. I particularly like the auto-scaling capability.
However, I encountered some challenges with the SDKs at multiple stages of use, so I had to look outside the official documentation for help. For example, while connecting to the cluster.
While the existing documentation is okay, it would be more beneficial if video resources were included (as this helps better than textual documentation). Additionally, integrating real-world examples and case studies into the documentation could greatly enhance its practical value.
The best solution out there
I've used mongodb professionally for 4 years and have found the product meets and exceeds the demands placed on it by the products i create.
Efficient use of NoSQL!
Connection smoother
Query understanding is easy
Among the best database solutions out there, and it's really useful
MongoDB
Future of Databases: A Critical Review of MongoDB
Also setting up MongoDB is quick and it integrates very easily with other platforms.
MongoDB offers strong customer support, with plenty of documentation, community forums, and professional support options. Because of all these features it is frequently and widely use across the globe and industries.
Proper indexing in MongoDb is also very crucial. Also sometimes it leads to data redundancy.
Also there was problem with scaling and it was very costly. MongoDb solved this problem also. It can be scaled horizontally by adding more servers in les cost.
It handles large volumes of data without degrading in performance.