Amazon EC2 Inf1 Instances
Businesses across a diverse set of industries are looking at artificial intelligence (AI)–powered transformation to drive business innovation and improve customer experience and process improvements. Machine learning (ML) models that power AI applications are becoming increasingly complex, resulting in rising underlying compute infrastructure costs. Up to 90% of the infrastructure spend for developing and running ML applications is often on inference. Customers are looking for cost-effective infrastructure solutions for deploying their ML applications in production.
Amazon EC2 Inf1 instances deliver high-performance and low-cost ML inference. They deliver up to 2.3x higher throughput and up to 70% lower cost per inference than comparable Amazon EC2 instances. Inf1 instances are built from the ground up to support ML inference applications. They feature up to 16 AWS Inferentia chips, high-performance ML inference chips designed and built by AWS. Additionally, Inf1 instances include 2nd Generation Intel Xeon Scalable processors and up to 100 Gbps networking to deliver high throughput inference.
Customers can use Inf1 instances to run large-scale ML inference applications such as search, recommendation engines, computer vision, speech recognition, natural language processing (NLP), personalization, and fraud detection.
Developers can deploy their ML models to Inf1 instances by using the AWS Neuron SDK, which is integrated with popular ML frameworks such as TensorFlow, PyTorch, and Apache MXNet. They can continue using the same ML workflows and seamlessly migrate applications onto Inf1 instances with minimal code changes and with no tie-in to vendor-specific solutions.
Get started easily with Inf1 instances using Amazon SageMaker, AWS Deep Learning AMIs (DLAMI) that come preconfigured with Neuron SDK, or Amazon Elastic Container Service (Amazon ECS) or Amazon Elastic Kubernetes Service (Amazon EKS) for containerized ML applications.
Benefits
Up to 70% lower cost per inference
Using Inf1, developers can significantly reduce the cost of their ML production deployments. The combination of low instance cost and high throughput of Inf1 instances delivers up to 70% lower cost per inference than comparable Amazon EC2 instances.
Ease of use and code portability
Neuron SDK is integrated with common ML frameworks such as TensorFlow, PyTorch, and MXNet. Developers can continue using the same ML workflows and seamlessly migrate their application on to Inf1 instances with minimal code changes. This gives them the freedom to use the ML framework of choice, the compute platform that best meets their requirements, and the latest technologies without being tied to vendor-specific solutions.
Up to 2.3x higher throughput
Inf1 instances deliver up to 2.3x higher throughput than comparable Amazon EC2 instances. AWS Inferentia chips that power Inf1 instances are optimized for inference performance for small batch sizes, enabling real-time applications to maximize throughput and meet latency requirements.
Extremely low latency
AWS Inferentia chips are equipped with large on-chip memory that enables caching of ML models directly on the chip itself. You can deploy your models using capabilities like the NeuronCore Pipeline that eliminates the need to access outside memory resources. With Inf1 instances, you can deploy real-time inference applications at near real-time latencies without impacting bandwidth.
Support for various ML models and data types
Inf1 instances support many commonly used ML model architectures such as SSD, VGG, and ResNext for image recognition/classification, as well as Transformer and BERT for NLP. Additionally, support for HuggingFace model repository in Neuron provides customers with the ability to easily compile and run inference using pretrained or fine-tuned models by changing just a single line of code. Multiple data types including BF16 and FP16 with mixed precision are also supported for various models and performance needs.
Features
Powered by AWS Inferentia
AWS Inferentia is an ML chip purpose built by AWS to deliver high-performance inference at low cost. Each AWS Inferentia chip has four first-generation NeuronCores and provides up to 128 tera operations per second (TOPS) of performance and support for FP16, BF16, and INT8 data types. AWS Inferentia chips also feature a large amount of on-chip memory that can be used for caching large models, which is especially beneficial for models that require frequent memory access.
Deploy with popular ML frameworks using AWS Neuron
The AWS Neuron SDK consists of a compiler, runtime driver, and profiling tools. It enables deployment of complex neural net models, created and trained in popular frameworks such as TensorFlow, PyTorch, and MXNet, to be executed using Inf1 instances. With NeuronCore Pipeline, you can split large models for execution across multiple Inferentia chips using a high-speed physical chip-to-chip interconnect, delivering high inference throughput and lower inference costs.
High-performance networking and storage
Inf1 instances offer up to 100 Gbps of networking throughput for applications that require access to high-speed networking. Next-generation Elastic Network Adapter (ENA) and NVM Express (NVMe) technology provide Inf1 instances with high-throughput, low-latency interfaces for networking and Amazon Elastic Block Store (Amazon EBS).
Built on AWS Nitro System
The AWS Nitro System is a rich collection of building blocks that offloads many of the traditional virtualization functions to dedicated hardware and software to deliver high performance, high availability, and high security while also reducing virtualization overhead.
How it works
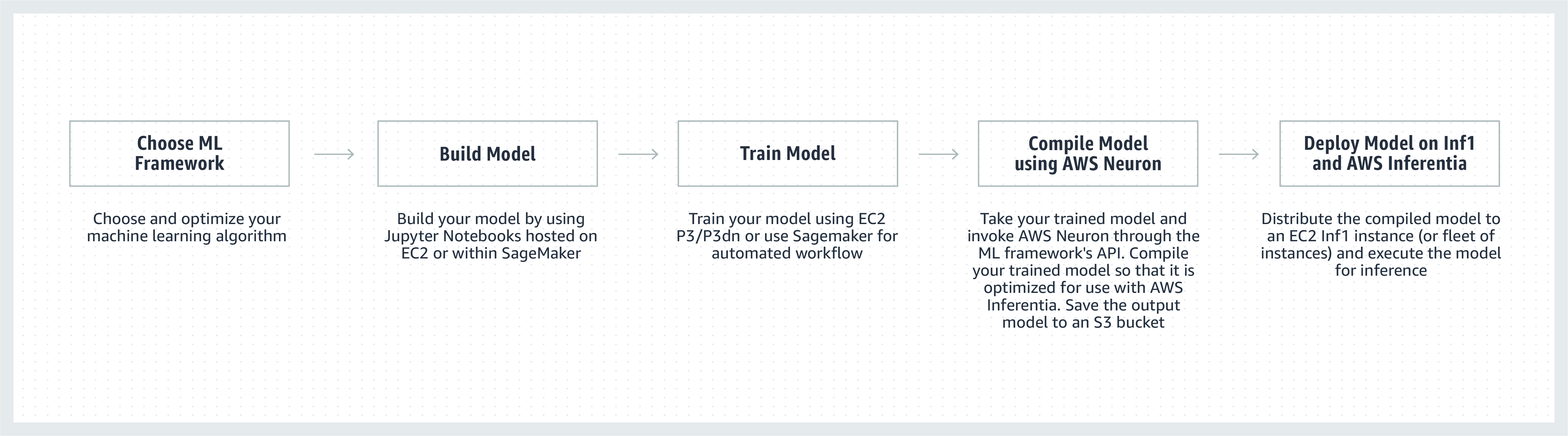
Customer testimonials
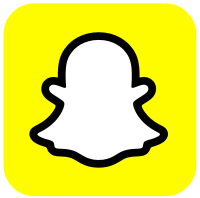
"We incorporate ML into many aspects of Snapchat, and exploring innovation in this field is a key priority. Once we heard about Inferentia, we started collaborating with AWS to adopt Inf1/Inferentia instances to help us with ML deployment, including around performance and cost. We started with our recommendation models and look forward to adopting more models with the Inf1 instances in the future."
Nima Khajehnouri, VP Engineering, Snap Inc.
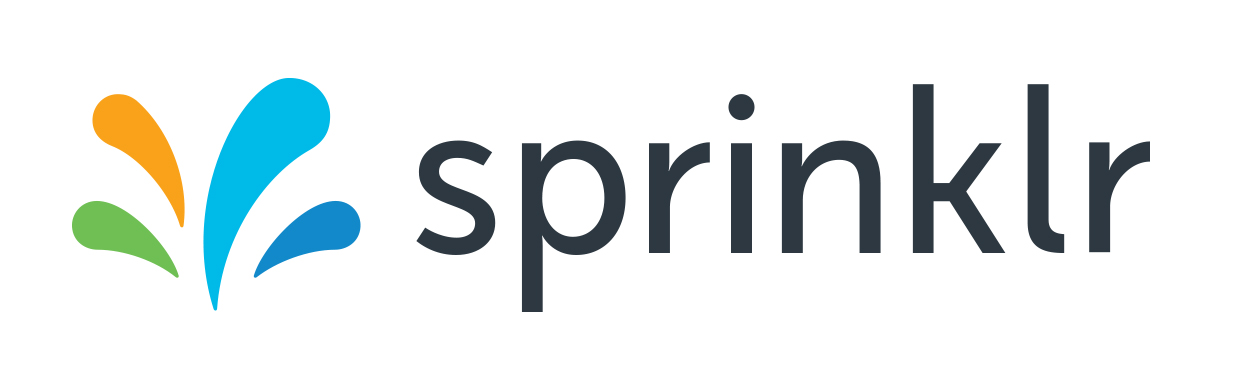
"Sprinklr's AI-driven unified customer experience management (Unified-CXM) platform enables companies to gather and translate real-time customer feedback across multiple channels into actionable insights—resulting in proactive issue resolution, enhanced product development, improved content marketing, better customer service, and more. Using Amazon EC2 Inf1, we were able to significantly improve the performance of one of our NLP models and improve the performance of one of our computer vision models. We're looking forward to continuing to use Amazon EC2 Inf1 to better serve our global customers."
Vasant Srinivasan, Senior Vice President of Product Engineering, Sprinklr
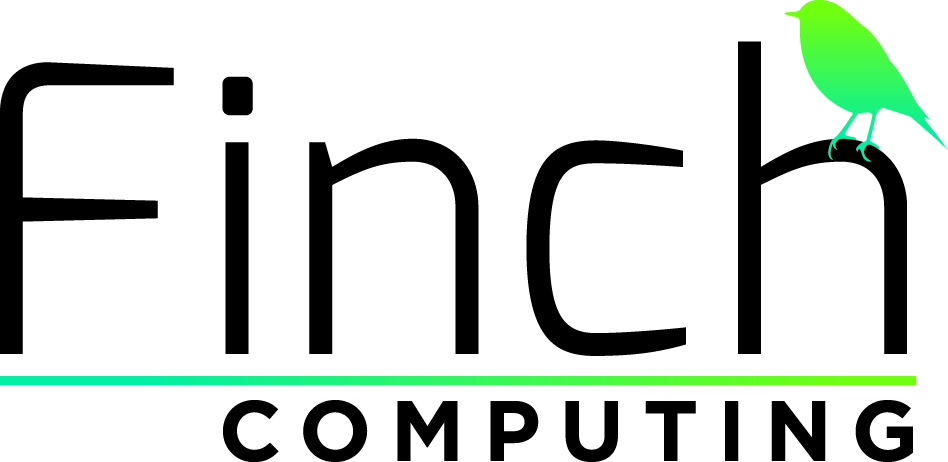
“Our state-of-the-art NLP product, Finch for Text, offers users the ability to extract, disambiguate, and enrich multiple types of entities in huge volumes of text. Finch for Text requires significant computing resources to provide our clients with low-latency enrichments on global data feeds. We are now using AWS Inf1 instances in our PyTorch NLP, translation, and entity disambiguation models. We were able to reduce our inference costs by over 80% (over GPUs) with minimal optimizations while maintaining our inference speed and performance. This improvement allows our customers to enrich their French, Spanish, German, and Dutch language text in real time on streaming data feeds and at global scale—something that’s critical for our financial services, data aggregator, and public sector customers."
Scott Lightner, Chief Technology Officer, Finch Computing

"We alert on many types of events all over the world in many languages, in different formats (images, video, audio, text sensors, combinations of all these types) from hundreds of thousands of sources. Optimizing for speed and cost given that scale is absolutely critical for our business. With AWS Inferentia, we have lowered model latency and achieved up to 9x better throughput per dollar. This has allowed us to increase model accuracy and grow our platform's capabilities by deploying more sophisticated DL models and processing 5x more data volume while keeping our costs under control."
Alex Jaimes, Chief Scientist and Senior Vice President of AI, Dataminr

"Autodesk is advancing the cognitive technology of our AI-powered virtual assistant, Autodesk Virtual Agent (AVA), by using Inferentia. AVA answers over 100,000 customer questions per month by applying natural language understanding (NLU) and deep learning (DL) techniques to extract the context, intent, and meaning behind inquiries. Piloting Inferentia, we are able to obtain a 4.9x higher throughput over G4dn for our NLU models, and look forward to running more workloads on the Inferentia-based Inf1 instances."
Binghui Ouyang, Sr. Data Scientist, Autodesk
Amazon services using Amazon EC2 Inf1 instances

Amazon Advertising helps businesses of all sizes connect with customers at every stage of their shopping journey. Millions of ads, including text and images, are moderated, classified, and served for the optimal customer experience every single day.
“For our text ad processing, we deploy PyTorch based BERT models globally on AWS Inferentia based Inf1 instances. By moving to Inferentia from GPUs, we were able to lower our cost by 69% with comparable performance. Compiling and testing our models for AWS Inferentia took less than three weeks. Using Amazon SageMaker to deploy our models to Inf1 instances ensured our deployment was scalable and easy to manage. When I first analyzed the compiled models, the performance with AWS Inferentia was so impressive that I actually had to re-run the benchmarks to make sure they were correct! Going forward, we plan to migrate our image ad processing models to Inferentia. We have already benchmarked 30% lower latency and 71% cost savings over comparable GPU-based instances for these models."
Yashal Kanungo, Applied Scientist, Amazon Advertising
Read the news blog »
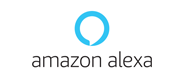
“Amazon Alexa’s AI- and ML-based intelligence, powered by AWS, is available on more than 100 million devices today—and our promise to customers is that Alexa is always becoming smarter, more conversational, more proactive, and even more delightful. Delivering on that promise requires continuous improvements in response times and ML infrastructure costs, which is why we are excited to use Amazon EC2 Inf1 to lower inference latency and cost per inference on Alexa text-to-speech. With Amazon EC2 Inf1, we’ll be able to make the service even better for the tens of millions of customers who use Alexa each month."
Tom Taylor, Senior Vice President, Amazon Alexa
"We are constantly innovating to further improve our customer experience and to drive down our infrastructure costs. Moving our web-based question answering (WBQA) workloads from GPU-based P3 instances to AWS Inferentia-based Inf1 instances not only helped us reduce inference costs by 60%, but also improved the end-to-end latency by more than 40%, helping enhance customer Q&A experience with Alexa. Using Amazon SageMaker for our TensorFlow-based model made the process of switching to Inf1 instances straightforward and easy to manage. We are now using Inf1 instances globally to run these WBQA workloads and are optimizing their performance for AWS Inferentia to further reduce cost and latency."
Eric Lind, Software Development Engineer, Alexa AI
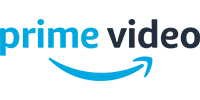
“Amazon Prime Video uses computer vision ML models to analyze video quality of live events to ensure an optimal viewer experience for Prime Video members. We deployed our image classification ML models on EC2 Inf1 instances and were able to see 4x improvement in performance and up to 40% savings in cost. We are now looking to leverage these cost savings to innovate and build advanced models that can detect more complex defects, such as synchronization gaps between audio and video files, to deliver more enhanced viewing experience for Prime Video members."
Victor Antonino, Solutions Architect, Amazon Prime Video

“Amazon Rekognition is a simple and easy image and video analysis application that helps customers identify objects, people, text, and activities. Amazon Rekognition needs high-performance DL infrastructure that can analyze billions of images and videos daily for our customers. With AWS Inferentia-based Inf1 instances, running Amazon Rekognition models such as object classification resulted in 8x lower latency and 2x the throughput compared to running these models on GPUs. Based on these results, we are moving Amazon Rekognition to Inf1, enabling our customers to get accurate results faster."
Rajneesh Singh, Director, SW Engineering, Amazon Rekognition and Video
Pricing
* Prices shown are for US East (Northern Virginia) AWS Region. Prices for 1-year and 3-year reserved instances are for "Partial Upfront" payment options or "No Upfront" for instances without the Partial Upfront option.
Amazon EC2 Inf1 instances are available in the US East (N. Virginia), US West (Oregon) AWS Regions as On-Demand, Reserved, or Spot Instances.
Getting started
Using Amazon SageMaker
SageMaker makes it easier to compile and deploy your trained ML model in production on Amazon Inf1 instances so that you can start generating real-time predictions with low latency. AWS Neuron, the compiler for AWS Inferentia, is integrated with Amazon SageMaker Neo, helping you compile your trained ML models to run optimally on Inf1 instances. With SageMaker, you can easily run your models on auto-scaling clusters of Inf1 instances that are spread across multiple Availability Zones to deliver both high performance and highly available real-time inference. Learn how to deploy to Inf1 using SageMaker with examples on GitHub.
Using DLAMI
DLAMI provides ML practitioners and researchers with the infrastructure and tools to accelerate DL in the cloud, at any scale. The AWS Neuron SDK comes pre-installed in DLAMI to compile and run your ML models optimally on Inf1 instances. To help guide you through the getting started process, visit the AMI selection guide and more DL resources. Refer to the AWS DLAMI Getting Started guide to learn how to use the DLAMI with Neuron.
Using Deep Learning Containers
Developers can now deploy Inf1 instances in Amazon EKS, which is a fully managed Kubernetes service, as well as in Amazon ECS, which is a fully managed container orchestration service from Amazon. Learn more about getting started with Inf1 on Amazon EKS or with Amazon ECS. More details about running containers on Inf1 instances are available on the Neuron container tools tutorial page. Neuron is also available pre-installed in AWS Deep Learning Containers.
Blogs and articles
How Amazon Search reduced ML inference costs by 85% with AWS Inferentia
by Joao Moura, Jason Carlson, Jaspreet Singh, Shaohui Xi, Shruti Koparkar, Haowei Sun, Weiqi Zhang, and Zhuoqi Zhangs, 9/22/2022
High-performance, low-cost machine learning infrastructure is accelerating innovation in the cloud
by MIT Technology Review Insights, 11/01/2021
by Davide Galliteli and Hasan Poonawala, 10/19/2021
Machine learning in the cloud is helping businesses innovate
by MIT Technology Review Insights, 10/15/2021
Serve 3,000 deep learning models on Amazon EKS with AWS Inferentia for under $50 an hour
by Alex Iankoulski, Joshua Correa, Mahadevan Balasubramaniam, and Sundar Ranganatha, 09/30/2021
by Fabio Nonato de Paula and Mahadevan Balasubramaniam, 05/04/2021
by Binghui Ouyang, 04/07/2021
Majority of Alexa now running on faster, more cost-effective Amazon EC2 Inf1 instances
by Sébastien Stormacq, 11/12/2020
Amazon ECS Now Supports EC2 Inf1 Instances
by Julien Simon, 08/14/2020
By Fabio Nonato De Paula and Haichen Li, 07/22/2020
Amazon EKS now supports EC2 Inf1 instances
by Julien Simon, 06/15/2020