Easy to use
Amazon EMR simplifies building and operating big data environments and applications. Related EMR features include easy provisioning, managed scaling, and reconfiguring of clusters, and EMR Studio for collaborative development.
Provision clusters in minutes: You can launch an EMR cluster in minutes. You don’t need to worry about infrastructure provisioning, cluster setup, configuration, or tuning. EMR takes care of these tasks allowing you to focus your teams on developing differentiated big data applications.
Easily scale resources to meet business needs: You can easily set scale out and scale in using EMR Managed Scaling policies and let your EMR cluster automatically manage the compute resources to meet your usage and performance needs. This improves cluster utilization and saves on costs.
EMR Studio is an integrated development environment (IDE) that makes it easy for data scientists and data engineers to develop, visualize, and debug data engineering and data science applications written in R, Python, Scala, and PySpark. EMR Studio provides fully managed Jupyter Notebooks, and tools like Spark UI and YARN Timeline Service to simplify debugging.
Single-click high availability: You can easily configure high availability for multi-master applications such as YARN, HDFS, Apache Spark, Apache HBase, and Apache Hive with a single click . When you enable multi-master support in EMR, EMR will configure these applications for High Availability, and in the event of failures, will automatically fail-over to a standby master so that your cluster is not disrupted, and place your master nodes in distinct racks to reduce risk of simultaneous failure. Hosts are monitored to detect failures, and when issues are detected, new hosts are provisioned and added to the cluster automatically.
EMR Managed Scaling: Automatically resizes your cluster for best performance at the lowest possible cost. With EMR Managed Scaling you specify the minimum and maximum compute limits for your clusters and Amazon EMR automatically resizes them for best performance and resource utilization. EMR Managed Scaling continuously samples key metrics associated with the workloads running on clusters.
Easily reconfigure running clusters: You can now modify the configuration of applications running on EMR clusters including Apache Hadoop, Apache Spark, Apache Hive, and Hue without re-starting the cluster. EMR Application Reconfiguration allows you to modify applications on the fly without needing to shut down or re-create the cluster. Amazon EMR will apply your new configurations and gracefully restart the reconfigured application. Configurations can be applied through the Console, SDK, or CLI.
Elastic
Amazon EMR enables you to quickly and easily provision as much capacity as you need, and automatically or manually add and remove capacity. This is very useful if you have variable or unpredictable processing requirements. For example, if the bulk of your processing occurs at night, you might need 100 instances during the day and 500 instances at night. Alternatively, you might need a significant amount of capacity for a short period of time. With Amazon EMR you can quickly provision hundreds or thousands of instances, automatically scale to match compute requirements, and shut your cluster down when your job is complete (to avoid paying for idle capacity).
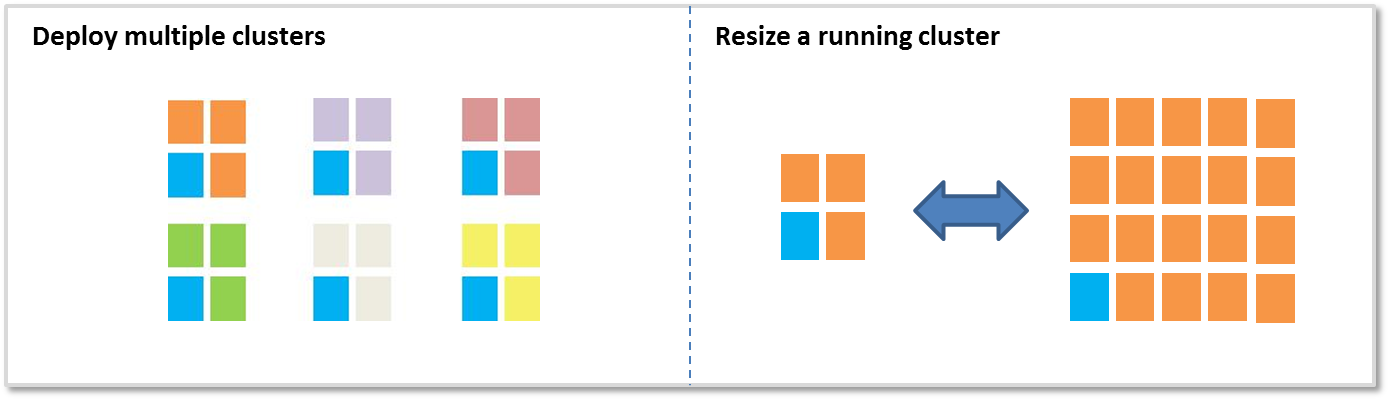
There are two main options for adding or removing capacity:
Deploy multiple clusters: If you need more capacity, you can easily launch a new cluster and terminate it when you no longer need it. There is no limit to how many clusters you can have. You may want to use multiple clusters if you have multiple users or applications. For example, you can store your input data in Amazon S3 and launch one cluster for each application that needs to process the data. One cluster might be optimized for CPU, a second cluster might be optimized for storage, etc.
Resize a running cluster: With Amazon EMR it is easy to use EMR Managed Scaling, automatically scale or manually resize a running cluster. You may want to scale out a cluster to temporarily add more processing power to the cluster, or scale in your cluster to save on costs when you have idle capacity. For example, some customers add hundreds of instances to their clusters when their batch processing occurs, and remove the extra instances when processing completes. When adding instances to your cluster, EMR can now start utilizing provisioned capacity as soon it becomes available. When scaling in, EMR will proactively choose idle nodes to reduce impact on running jobs.
Low cost
Amazon EMR is designed to reduce the cost of processing large amounts of data. Some of the features that make it low cost include low per-second pricing, Amazon EC2 Spot integration, Amazon EC2 Reserved Instance integration, elasticity, and Amazon S3 integration.
Low Per-Second Pricing: Amazon EMR pricing is per-second with a one-minute minimum, and starts at $.015 per instance hour for a small instance ($131.40 per year). See the pricing section for more detail.
Amazon EC2 Spot Integration: The Amazon EC2 Spot Instances price fluctuates based on supply and demand for instances, but you will never pay more than the maximum price you specified. Amazon EMR makes it easy to use Spot instances so you can save both time and money. Amazon EMR clusters include 'core nodes' that run HDFS and ‘task nodes’ that do not; task nodes are ideal for Spot because if the Spot price increases and you lose those instances you will not lose data stored in HDFS. (Learn more about core and task nodes). With the combination of instance fleets, allocation strategies for spot instances, EMR Managed Scaling and more diversification options, you can now optimize EMR for resilience and cost. Please read our blog to learn more.
Amazon S3 Integration: The EMR File System (EMRFS) allows EMR clusters to efficiently and securely use Amazon S3 as an object store for Hadoop. You can store your data in Amazon S3 and use multiple Amazon EMR clusters to process the same data set. Each cluster can be optimized for a particular workload, which can be more efficient than a single cluster serving multiple workloads with different requirements. For example, you might have one cluster that is optimized for I/O and another that is optimized for CPU, each processing the same data set in Amazon S3. In addition, by storing your input and output data in Amazon S3, you can shut down clusters when they are no longer needed.
EMRFS has strong performance reading from and writing to Amazon S3, supports S3 server-side or S3 client-side encryption using AWS Key Management Service (KMS) or customer-managed keys, and offers an optional consistent view which checks for list and read-after-write consistency for objects tracked in its metadata. Also, Amazon EMR clusters can use both EMRFS and HDFS, so you don’t have to choose between on-cluster storage and Amazon S3.
AWS Glue Data Catalog Integration: You can use the AWS Glue Data Catalog as a managed metadata repository to store external table metadata for Apache Spark and Apache Hive. Additionally, it provides automatic schema discovery and schema version history. This allows you to easily persist metadata for your external tables on Amazon S3 outside of your cluster.
Flexible data stores
With Amazon EMR, you can leverage multiple data stores, including Amazon S3, the Hadoop Distributed File System (HDFS), and Amazon DynamoDB.
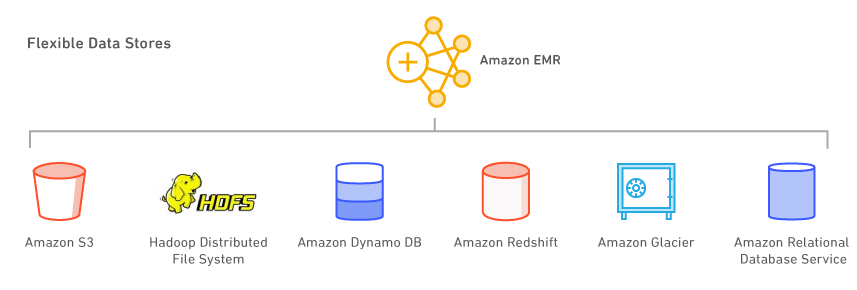
Amazon S3: Amazon S3 is a highly durable, scalable, secure, fast, and inexpensive storage service. With the EMR File System (EMRFS), Amazon EMR can efficiently and securely use Amazon S3 as an object store for Hadoop. Amazon EMR has made numerous improvements to Hadoop, allowing you to seamlessly process large amounts of data stored in Amazon S3. Also, EMRFS can enable consistent view to check for list and read-after-write consistency for objects in Amazon S3. EMRFS supports S3 server-side or S3 client-side encryption to process encrypted Amazon S3 objects, and you can use the AWS Key Management Service (KMS) or a custom key vendor.
When you launch your cluster, Amazon EMR streams the data from Amazon S3 to each instance in your cluster and begins processing it immediately. One advantage of storing your data in Amazon S3 and processing it with Amazon EMR is you can use multiple clusters to process the same data. For example, you might have a Hive development cluster that is optimized for memory and a Pig production cluster that is optimized for CPU both using the same input data set.
Hadoop Distributed File System (HDFS): HDFS is the Hadoop file system. Amazon EMR’s current topology groups its instances into 3 logical instance groups: Master Group, which runs the YARN Resource Manager and the HDFS Name Node Service; Core Group, which runs the HDFS DataNode Daemon and the YARN Node Manager service; and Task Group, which runs the YARN Node Manager service. Amazon EMR installs HDFS on the storage associated with the instances in the Core Group.
Each EC2 instance comes with a fixed amount of storage, referenced as "instance store", attached with the instance. You can also customize the storage on an instance by adding Amazon EBS volumes to an instance. Amazon EMR allows you to add General Purpose (SSD), Provisioned (SSD) and Magnetic volumes types. The EBS volumes added to an EMR cluster do not persist data after the cluster is shutdown. EMR will automatically clean-up the volumes, once you terminate your cluster.
You can also enable complete encryption for HDFS using an Amazon EMR security configuration, or manually create HDFS encryption zones with the Hadoop Key Management Server. You can use a security configuration option to encrypt EBS root device and storage volumes when you specify AWS KMS as your key provider. For more information, see Local Disk Encryption.
Amazon DynamoDB: Amazon DynamoDB is a fast, fully managed NoSQL database service. Amazon EMR has direct integration with Amazon DynamoDB so you can quickly and efficiently process data stored in Amazon DynamoDB and transfer data between Amazon DynamoDB, Amazon S3, and HDFS in Amazon EMR.
Other AWS Data Stores: You can also use Amazon Relational Database Service (a web service that makes it easy to set up, operate, and scale a relational database in the cloud), Amazon Glacier (an extremely low-cost storage service that provides secure and durable storage for data archiving and backup), and Amazon Redshift (a fast, fully managed, petabyte-scale data warehouse service). AWS Data Pipeline is a web service that helps you reliably process and move data between different AWS compute and storage services (including Amazon EMR) as well as on-premises data sources at specified intervals.
Use your favorite open source applications
With versioned releases on Amazon EMR, you can easily select and use the latest open source projects on your EMR cluster, including applications in the Apache Spark and Hadoop ecosystems. Software is installed and configured by Amazon EMR, so you can spend more time on increasing the value of your data without worrying about infrastructure and administrative tasks.
Big Data Tools
Amazon EMR supports powerful and proven Hadoop tools such as Apache Spark, Apache Hive, Presto, and Apache HBase. Data scientists use EMR to run deep learning and machine learning tools such as TensorFlow, Apache MXNet, and, using bootstrap actions, add use case-specific tools and libraries. Data analysts use EMR Studio, Hue and EMR Notebooks for interactive development, authoring Apache Spark jobs, and submitting SQL queries to Apache Hive and Presto. Data Engineers use EMR for data pipeline development and data processing, and use Apache Hudi to simplify incremental data management and data privacy use cases requiring record-level insert, updates, and delete operations.
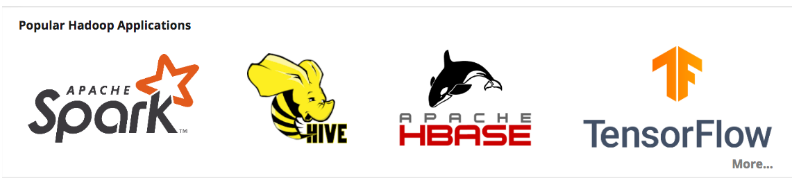
Data Processing & Machine Learning
Apache Spark is an engine in the Hadoop ecosystem for fast processing for large data sets. It uses in-memory, fault-tolerant resilient distributed datasets (RDDs) and directed, acyclic graphs (DAGs) to define data transformations. Spark also includes Spark SQL, Spark Streaming, MLlib, and GraphX. Learn what is Spark, and more about Spark on EMR.
Apache Flink is a streaming dataflow engine that makes it easy to run real-time stream processing on high-throughput data sources. It supports event time semantics for out of order events, exactly-once semantics, backpressure control, and APIs optimized for writing both streaming and batch applications. Learn what is Flink and more about Flink on EMR.
TensorFlow is an open source symbolic math library for machine intelligence and deep learning applications. TensorFlow bundles together multiple machine learning and deep learning models and algorithms and can train and run deep neural networks for many different use cases. Learn more about TensorFlow on EMR.
Record-Level Amazon S3 Data Management
Apache Hudi is an open-source data management framework used to simplify incremental data processing and data pipeline development. Apache Hudi enables you to manage data at the record-level in Amazon S3 to simplify Change Data Capture (CDC) and streaming data ingestion, and provides a framework to handle data privacy use cases requiring record level updates and deletes. Learn more about Apache Hudi on Amazon EMR.
SQL
Apache Hive is an open source data warehouse and analytics package that runs on top of Hadoop. Hive is operated by Hive QL, a SQL-based language which allows users to structure, summarize, and query data. Hive QL goes beyond standard SQL, adding first-class support for map/reduce functions and complex extensible user-defined data types like JSON and Thrift. This capability allows processing of complex and unstructured data sources such as text documents and log files. Hive allows user extensions via user-defined functions written in Java. Amazon EMR has made numerous improvements to Hive, including direct integration with Amazon DynamoDB and Amazon S3. For example, with Amazon EMR you can load table partitions automatically from Amazon S3, you can write data to tables in Amazon S3 without using temporary files, and you can access resources in Amazon S3 such as scripts for custom map/reduce operations and additional libraries. Learn what is Hive, and more about Hive on EMR.
Presto is an open-source distributed SQL query engine optimized for low-latency, ad-hoc analysis of data. It supports the ANSI SQL standard, including complex queries, aggregations, joins, and window functions. Presto can process data from multiple data sources including the Hadoop Distributed File System (HDFS) and Amazon S3. Learn what is Presto, and more about Presto on EMR.
Apache Phoenix enables low-latency SQL with ACID transaction capabilities over data stored in Apache HBase. You can easily create secondary indexes for additional performance, and create different views over the same underlying HBase table. Learn more about Phoenix on EMR.
NoSQL
Apache HBase is an open source, non-relational, distributed database modeled after Google's BigTable. It was developed as part of Apache Software Foundation's Hadoop project and runs on top of Hadoop Distributed File System(HDFS) to provide BigTable-like capabilities for Hadoop. HBase provides you a fault-tolerant, efficient way of storing large quantities of sparse data using column-based compression and storage. In addition, HBase provides fast lookup of data because it caches data in-memory. HBase is optimized for sequential write operations, and it is highly efficient for batch inserts, updates, and deletes. HBase works seamlessly with Hadoop, sharing its file system and serving as a direct input and output to Hadoop jobs. HBase also integrates with Apache Hive, enabling SQL-like queries over HBase tables, joins with Hive-based tables, and support for Java Database Connectivity (JDBC). With EMR, you can use S3 as a data store for HBase, enabling you to lower costs and reduce operational complexity. If you use HDFS as a data store, you can back up HBase to S3 and you can restore from a previously created backup. Learn what is HBase, and more about HBase on EMR.
Interactive Analytics
EMR Studio is an integrated development environment (IDE) that makes it easy for data scientists and data engineers to develop, visualize, and debug data engineering and data science applications written in R, Python, Scala, and PySpark. EMR Studio provides fully managed Jupyter Notebooks, and tools like Spark UI and YARN Timeline Service to simplify debugging.
Hue is an open source user interface for Hadoop that makes it easier to run and develop Hive queries, manage files in HDFS, run and develop Pig scripts, and manage tables. Hue on EMR also integrates with Amazon S3, so you can query directly against S3 and easily transfer files between HDFS and Amazon S3. Learn more about Hue and EMR.
Jupyter Notebook is an open-source web application that you can use to create and share documents that contain live code, equations, visualizations, and narrative text. JupyterHub allows you to host multiple instances of a single-user Jupyter notebook server. When you create a EMR cluster with JupyterHub, EMR creates a Docker container on the cluster's master node. JupyterHub, all the components required for Jupyter, and Sparkmagic run within the container.
Apache Zeppelin is an open source GUI which creates interactive and collaborative notebooks for data exploration using Spark. You can use Scala, Python, SQL (using Spark SQL), or HiveQL to manipulate data and quickly visualize results. Zeppelin notebooks can be shared among several users, and visualizations can be published to external dashboards. Learn more about Zeppelin on EMR.
Scheduling and workflow
Apache Oozie is a workflow scheduler for Hadoop, where you can create Directed Acyclic Graphs (DAGs) of actions. Also, you can easily trigger your Hadoop workflows by actions or time. Learn more about Oozie on EMR. AWS Step Functions allows you to add serverless workflow automation to your applications. The steps of your workflow can run anywhere, including in AWS Lambda functions, on Amazon Elastic Compute Cloud (EC2), or on-premises. Learn more about Step Functions on EMR.
Other projects and tools
EMR also supports a variety of other popular applications and tools, such as R, Apache Pig (data processing and ETL), Apache Tez (complex DAG execution), Apache MXNet (deep learning), Ganglia (monitoring), Apache Sqoop (relational database connector), HCatalog (table and storage management), and more. The Amazon EMR team maintains an open source repository of bootstrap actions that can be used to install additional software, configure your cluster, or serve as examples for writing your own bootstrap actions.
Data access control
By default, Amazon EMR application processes use EC2 instance profile when they call other AWS services. For multi-tenant clusters, Amazon EMR offers three options to manage user access to Amazon S3 data.
Integration with AWS Lake Formation allows you to define and manage fine-grained authorization policies in AWS Lake Formation to access databases, tables, and columns in AWS Glue Data Catalog. You can enforce the authorization policies on jobs submitted through Amazon EMR Notebooks and Apache Zeppelin for interactive EMR Spark workloads, and send auditing events to AWS CloudTrail. By enabling this integration, you also enable federated Single Sign-On to EMR Notebooks or Apache Zeppelin from enterprise identity systems compatible with Security Assertion Markup Language (SAML) 2.0.
Native integration with Apache Ranger allows you to set up a new or an existing Apache Ranger server to define and manage fine-grained authorization policies for users to access databases, tables, and columns of Amazon S3 data via Hive Metastore. Apache Ranger is an open-source tool to enable, monitor, and manage comprehensive data security across the Hadoop platform.
This native integration allows you to define three types of authorization policies on the Apache Ranger Policy Admin server. You can set table, column, and row level authorization for Hive, table and column level authorization for Spark, and prefix and object level authorization for Amazon S3. Amazon EMR automatically installs and configures the corresponding Apache Ranger plugins on the cluster. These Ranger plugins sync up with the Policy Admin server for authorization polices, enforce data access control, and send auditing events to Amazon CloudWatch Logs.
Amazon EMR User Role Mapper allows you to leverage AWS IAM permissions to manage accesses to AWS resources. You can create mappings between users (or groups) and custom IAM roles. A user or group can only access the data permitted by the custom IAM role. This feature is currently available through AWS Labs.
Consistent Hybrid Experience
AWS Outposts is a fully managed service that extends AWS infrastructure, AWS services, APIs, and tools to virtually any data center, co-location space, or on-premises facility for a truly consistent hybrid experience. Amazon EMR on AWS Outposts allows you to, allowing you to deploy and manage EMR clusters in your data center using the same AWS Management Console, Software Development Kit (SDK), and Command Line Interface (CLI) used for EMR.
Additional features
Select the right instance for your cluster: You choose what types of EC2 instances to provision in your cluster (standard, high memory, high CPU, high I/O, etc.) based on your application’s requirements. You have root access to every instance and you can fully customize your cluster to suit your requirements. Learn more about supported Amazon EC2 Instance Types. Amazon EMR now provides up to 30% lower cost and up to 15% improved performance for Spark workloads on Graviton2-based instances. Learn more from our blog.
Debug your applications: When you enable debugging on a cluster, Amazon EMR archives the log files to Amazon S3 and then indexes those files. You can then use a graphical interface in the console to browse the logs and view job history in an intuitive way. Learn more about debugging Amazon EMR jobs.
Monitor your cluster: You can use Amazon CloudWatch to monitor custom Amazon EMR metrics, such as the average number of running map and reduce tasks. You can also set alarms on these metrics. Learn more about monitoring Amazon EMR clusters.
Respond to events: You can use Amazon EMR event types in Amazon CloudWatch Events to respond to state changes in your Amazon EMR clusters. Using simple rules that you can quickly set up, you can match events and route them to Amazon SNS topics, AWS Lambda functions, Amazon SQS queues, and more. Learn more about events in Amazon EMR clusters.
Schedule recurring workflows: You can use AWS Data Pipeline to schedule recurring workflows involving Amazon EMR. AWS Data Pipeline is a web service that helps you reliably process and move data between different AWS compute and storage services as well as on-premise data sources at specified intervals. Learn more about Amazon EMR and AWS Data Pipeline.
Deep learning: Use popular deep learning frameworks like Apache MXNet to define, train, and deploy deep neural networks. You can use these frameworks on Amazon EMR clusters with GPU instances. Learn more about MXNet on Amazon EMR.
Control network access to your cluster: You can launch your cluster in an Amazon Virtual Private Cloud (VPC), a logically isolated section of the AWS cloud. You have complete control over your virtual networking environment, including selection of your own IP address range, creation of subnets, and configuration of route tables and network gateways. Learn more about Amazon EMR and Amazon VPC.
Manage users, permissions and encryption: You can use AWS Identity and Access Management (IAM) tools such as IAM Users and Roles to control access and permissions. For example, you could give certain users read but not write access to your clusters. Also, you can use Amazon EMR security configurations to set various encryption at-rest and in-transit options, including support for Amazon S3 encryption, and Kerberos authentication. Learn more about controlling access to your cluster and Amazon EMR encryption options.
Install additional software: You can use bootstrap actions or a custom Amazon Machine Image (AMI) running Amazon Linux to install additional software on your cluster. Bootstrap actions are scripts that are run on the cluster nodes when Amazon EMR launches the cluster. They run before Hadoop starts and before the node begins processing data. You can also preload and use software on a custom Amazon Linux AMI. Learn more about Amazon EMR Bootstrap Actions and custom Amazon Linux AMIs.
Efficiently copy data: You can quickly move large amounts of data from Amazon S3 to HDFS, from HDFS to Amazon S3, and between Amazon S3 buckets using Amazon EMR’s S3DistCp, an extension of the open source tool Distcp, which uses MapReduce to efficiently move large amounts of data. Learn more about S3DistCp.
Custom JAR: Write a Java program, compile against the version of Hadoop you want to use, and upload to Amazon S3. You can then submit Hadoop jobs to the cluster using the Hadoop JobClient interface. Learn more about Custom JAR processing with Amazon EMR.
Learn more about Amazon EMR pricing