AWS for Industries
How Artificial Intelligence Forecasting Can Help Utility Regulators Weather the COVID-19 Storm
This is a contributed blog post from Siddahartha Sachdeva, CEO of AWS partner Innowatts.
The COVID-19 pandemic is transforming energy consumption and usage patterns. During the pandemic, commercial energy usage slumped 30%, whereas residential demand saw significant increase. These changes surface and illuminate challenges to utilities and regulators. As utilities manage the changing energy landscape, regulators require new tools to understand the impact of economic transformation on the electric utility industry.
One solution to solve these challenges is to provide an important sublayer, which provides visibility along with new insights into locational demand drivers. Leveraging an array of artificial intelligence (AI) models, it provides utilities with predictive, actionable and operational and customer intelligence. For regulated utilities, this translates directly to improvement in key metrics such as system average interruption frequency index (SAIFI), customer average interruption frequency index (CAIFI), and momentary average interruption frequency index (MAIFI). Improvements in these core reliability metrics drive improved utility financial performance and increased customer satisfaction.
Innowatts has built a predictive analytics-enabled energy technology platform to accelerate energy transition and enhance customer value. Innowatts’ cloud instance running on AWS uses services like Amazon EMR, Amazon Redshift, Amazon GuardDuty for intelligent threat detection, among others.
Smart meters and other smart-grid infrastructure generate vast amounts of data but unlocking insights from these huge and interconnected datasets can be challenging. That is where machine learning (ML)-enabled predictive intelligence shines. By using breakthrough ML tools to identify patterns in large datasets, it becomes easier to identify actionable signals amidst the noise created by reams of incoming data.
For example, load clustering analysis can be used to group customers according to similar usage patterns. These tools help create customer segments that make it easier to assign these users to appropriate rate classes. Another approach might use load disaggregation to differentiate between customers based on their sensitivity to weather, with an ML-enabled model learning customer usage trends and predicting consumption patterns with respect to the changes in weather, daily routine, or device usage. Most powerfully of all, AI tools can harness these signals to anticipate and forecast future demand patterns with far greater accuracy than current historical analysis tools, enabling energy providers and regulators to understand the changing energy environment and create more effective strategies and policies to advocate for the benefits of the consumer. This blog and the full NRRI Insights paper examines the ways that ML-enabled predictive intelligence can help shield utilities from financial and operational disruption, lowering costs for customers, while also giving regulators a rich new source of intelligence as they work to protect customers and drive energy transformation via digital transformation.
Case Study: What AI Reveals about Post-COVID Energy Demand
To illustrate the power of ML-enabled predictive intelligence, Innowatts used its AI models to examine the way that power requirements changed during the early weeks of the COVID-19 pandemic. Within three days of March 2020’s shelter-in-place orders, Innowatts’ ML-based models — based on readings from more than 43 million meters processed 4.83 billion data points per hour — this capability identified new usage patterns and developed significantly more accurate predictions, that accounted for the shift in commercial and residential energy use due to the pandemic.
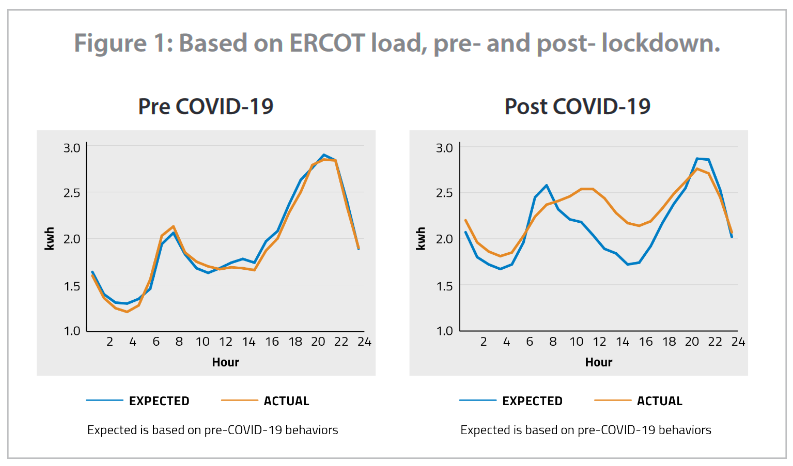
ERCOT Pre and Post Covid Expected Load Patterns
As shown in Figure 1, we found that residential consumption was between 6-9% higher than normal, and up to 15% higher during working hours. Load patterns also changed: mealtimes became optional, with lunch and dinner peaks evaporating as home-bound families cooked at whatever time suited them best.
Today, the US economy has rebounded from the worst of the downturn, and AI forecasts have helped us see exactly which parts of the economy is doing well, and which are still struggling to recover. For example, consumption is trending up for some segments, including social services, convenience stores, gas stations, and grocery stores, while power consumption remains low among business associations, banks, restaurants, furniture stores, and fitness centers.
Interestingly, the resurgence of commercial demand in recent weeks has not led to a corresponding downturn in residential demand. In fact, we are still seeing a 7% increase in residential load during the standard workday, likely due to remote schooling and working, as well as lower headcount as some businesses have closed or contracted.
How Utilities and Regulators Can Leverage AI-enabled Energy Intelligence
Energy forecasting typically looks beyond consumption to understand future usage, using mathematical models to connect historic usage patterns and current data describing weather patterns, market conditions, and other industry-related inputs. AI forecasting is different. Instead of just relying on aggregated historical trends, ML-enabled predictive intelligence can glean data from millions of individual smart meters and other key and strategic data correlations to create a rich library of demand scenarios for different events and technologies, reflecting the reality of how and when electricity is used in the real world. These libraries are built over time according to specific needs and circumstances: for instance, in an area with heavy EV or solar penetration, the ML-enabled system can create footprints for the classes of customers found in the area, and use those footprints to generate customized forecasts for today and into the future. Using advances in machine learning, the data libraries translate into use cases that have actionable operational intelligence, such as predicting high-usage alerts, or generating maintenance alerts for malfunctioning AC and heating systems, along with forecasts that outperform conventional tools. Compared to conventional forecasting methods, the results are proven and quite remarkable.
Read the full paper and the results of Innowatts’ research (reproduced here in part edited with permission) at the National Regulatory Research Institute.
Conclusion
AI-driven load forecasting is part of a broader shift toward data-driven, technologically advanced, and customer-centric power management. Generation systems, the grid itself, and all other forms of energy infrastructure are getting smarter and more interconnected. Providers that capitalize on intelligence-led digital transformation will be able to put customers first, not only by anticipating changes, but by driving them, and proactively managing costs, revenues, and customer satisfaction. Regulators may consider engaging with providers to understand and help manage this transformation. When electric utilities seek assistance, regulators may want to inform them about the potential for AI tools to assist in providing accurate forecasting. Visit Innowatts or AWS Power & Utilities for more information.