AWS Startups Blog
Klaviyo Delivers Personalized Marketing Solutions Using Amazon SageMaker
It’s long been held that in great amounts of data lie opportunities for great marketing. Klaviyo, a marketing platform for e-commerce businesses, uses Amazon SageMaker to deliver on this promise for over 42,000 brands around the world. The company has been growing rapidly by making it easy to do something that is typically very difficult: using insightful customer information to drive marketing actions.
Before Klaviyo, B2C companies sent a single email to all their customers, with little to no segmentation or personalization. Now, companies can send coordinate personalized outreach at scale by analyzing rich amounts of data and using it to inform a unique message, delivered through different channels at different times.
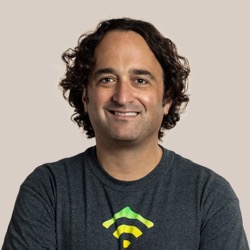
Ezra Freedman, VP of Data Science
“At Klaviyo, we build models for our customers to help them understand their business and give them actionable insights. Our customers rely on our ability to predict metrics like customer lifetime value, probability of churn, and expected date of next order. Thanks to Amazon SageMaker, we can train thousands of models in parallel, see results quickly, and iterate to build meaningful capabilities for our customers.” says Ezra Freedman, VP of Data Science at Klaviyo.
E-commerce businesses on average see a 29% bump in performance after using Klaviyo. A rich profile is created for each individual with datapoints about consumers from various sources – transaction history from an e-commerce platform, website interactivity behavior and marketing engagement data, such as email opens. Businesses can then perform customer segmentation with individual profiles for each customer by drawing relevant conclusions using machine learning. Then, they can use these segments and insights to send personalized communications across multiple channels, all from the Klaviyo platform.
To power this marketing engine, Klaviyo has turned to AWS to both support the backend and test new features. For example, the company recently introduced the ability to predict the Customer Lifetime Value (CLV) and churn risk of a customers for a business. CLV represents the value of a customer to a particular business through the lifetime relationship with that customer, while churn risk is the probability that a customer will never return.
To predict CLV and the probability of customer churn, Klaviyo needed to build, train, and validate tens of thousands of machine learning (ML) models. Using SageMaker, Klaviyo was able to continuously build and train these ML models by leveraging SageMaker’s scalable API infrastructure to constantly build and iterate while architecting new services using data science.
With ML models built using SageMaker, Klaviyo’s customers are able to systematically take actions on predictions about their customers. For example, companies can enable different ways of marketing to loyal VIP customers versus customers likely to churn. Using predictions based on machine learning, companies can also easily categorize these customers without having to manually come up with rules for each one.
“The exciting thing about our scale and number of customers is that we can rapidly improve the quality of our ML models. Amazon SageMaker makes it practical because our training jobs spin up and we train thousands of models in parallel. We see results quickly and can iterate, helping us build meaningful capabilities for our customers”, says Ezra Freedman, VP of Data Science at Klaviyo.
Klaviyo is now training new types of machine learning models to support upcoming features. With the architecture and the foundation setup using Amazon SageMaker, Klaviyo anticipates being able to build and deploy these models quickly, easily, and at scale, to provide better services and experiences.
You can learn more about AI-powered Customer Lifetime Value in this blog post. To get started with Amazon SageMaker, you can try the 10-minute tutorial and build, train, and deploy machine learning models at scale using the SageMaker management console.
Interested in ML on AWS? Contact us today!