AWS Startups Blog
Synthesis AI’s Generative AI Platform is Set to Fuel the Next Wave of Computer Vision Innovation
Founded in 2019, San Francisco-based Synthesis AI has developed technology that generates vast quantities of photorealistic images and pixel-perfect labels to optimize computer vision training. “The world is exploding with cameras,” says Synthesis AI CEO Yashar Behzadi. This is great news for AI startups that specialize in computer vision, a field of AI that trains computers to interpret elements from digital images and videos. Up to now, computer vision has relied heavily on supervised learning, in which humans label key attributes in an image and then teach computers to do the same. But to Behzadi, this method has some pretty major setbacks.
“Supervised learning requires humans in the loop, making the process expensive and hard to scale,” he says. “More importantly, the human eye can’t label all the attributes a company might be interested in.” Behzadi says, “As we look at the new world of autonomous vehicles, augmented reality, and virtual reality, we’re fundamentally limited by traditional approaches.” Finally, real-world data presents a growing issue surrounding ethical use and privacy. Tasking humans to annotate user-generated images, is only becoming more prohibitive as countries establish compliance laws around data collection.
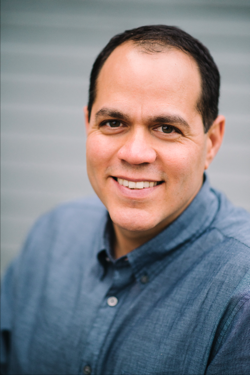
Yashar Behzadi, founder & CEO
That’s where Synthesis AI comes in. The startup has built a data-generation platform for computer vision by leveraging tools from the world of visual effects and CGI, then combining them with generative AI models. With that, they are able to create vast amounts of photorealistic, diverse data on-demand, such as millions of unique human faces and realistic environments that can be used to train computer vision models. In a nutshell, that means less human labor, more sophisticated data collection, and virtually zero potential for breach of privacy.
“Through our simple API, you can generate millions of imageswith accompanying labels. And obviously, since the images are generated, you know everything about every pixel, so you have an expanded set of annotations. The images are also completely privacy compliant, so there are no regulatory concerns. Finally, it’s two orders of magnitude cheaper than it would be to have humans label the data,” Behzadi explains.
According to Behzadi, the scalability and flexibility of AWS’s compute resources is a key part of their development, helping them to produce more images faster for their clients. “We’re using generation and rendering pipelines in non-traditional ways, which require an immense amount of compute for what we’re trying to do, and AWS provided the best scalable solution for us. Just last month on AWS, we ran almost 10 million images for a client, which would have taken years to acquire, if at all possible, with traditional means.”
Behzadi also points to AWS’s containerization services as another valuable element in Synthesis AI’s work. “Being able to create simple containers that encompass a full render job allows us to streamline the process,” he says. “We then leverage the queue management auto-scaling systems to scale efficiently. That’s been a huge plus.”
What does the future hold for computer vision and Synthesis AI? Behzadi believes that simulations and synthetic data will be core to how people develop any computer vision model. “We want to build a general purpose platform,” he says. “The name of the game in AI is scale, so we’re looking to create increasingly more data with increasing diversity. Last month, we did 10 million images. With some of these clients, we’ll get to hundreds of millions of images, and that just requires a very robust, highly available compute infrastructure that AWS has thus far been able to provide.”