AWS Startups Blog
Understanding and Optimizing ML Models with DarwinAI
Artificial intelligence may be the future, but 90% of AI models developed today don’t make it into production. “Suppose you’re able to get past the first part, where you manage to build something functional, and you manage to get through the second part, where it’s scalable,” says Dr. Alexander Wong, co-founder and Chief Scientist at DarwinAI. “Then you have no idea how it behaves in production, not only internally, but how it behaves in the wild. You don’t really know how to improve it, and you don’t really know where the underlying biases are.” This is known as the explainability issue.
When Wong set out to co-found DarwinAI, he was cognizant of those challenges. “I don’t like to build things that people will never use,” says Wong. “And one of my main goals and missions is to make sure that the types of research technologies that we build become tangible, tractable, impactful solutions to enterprises and society at large.” So he created DarwinAI, a technology program that centers around quantitative explainability and enables users to understand underlying systems of behavior. This also translates into increased ease when it comes to scalability. “Our underlying platform technology will actually accelerate and automate this whole process so that you end up with an AI model that meets your requirements, not just in terms of performance, but also in terms of your key operational KPIs, such as speed, size, memory requirement, and energy throughout,” says Wong.
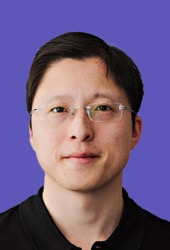
Dr. Alexander Wong, co-founder and Chief Scientist
So far, DarwinAI has proven particularly useful when implemented by industries such as healthcare, manufacturing, and finance. With the help of AWS’s resources, DarwinAI was able to build a dependable, more efficient, state-of-the-art AI model for predicting lung function decline from pulmonary fibrosis. The explainable technology allowed developers to be aware of hidden data issues that might otherwise go unnoticed. “We perform what we call ‘explainability-driven data auditing,’” says Wong. “Using the explainable technology, we were able to surface things like imaging artifacts, artificial padding, dynamic range problems, and calibration issues. Those are all things that we can do from a data perspective—as well as model perspective—to ensure that the underlying AI solution is one that you can trust and depend on.”
When it comes to manufacturing, DarwinAI is similarly vital, especially for situations in which a human inspector needs to examine a huge number of parts, thus driving up the likelihood of human error. DarwinAI has been working with manufacturing partners to build solutions that minimize defects and problems that might result in error, as well as understand why these particular things are being flagged as problematic.
This explainability model has also been helpful in finance, an area where algorithmic transparency is especially important—both for building AI models and for client accountability. “We’re able to provide reasoning,” says Wong. “So a financial institute can report back to their client, ‘this happened because X, Y, and Z, and it’s critical for regulatory compliance.’” This sort of auditing can also be used to analyze the behavior of the AI models, searching for potential inherent demographic biases: “Using this type of quantitative explainability, we are not only able to surface how decisions are made, but ask: What are the key factors? Is it payment defaulting history? Is it because of gender? And so on and so forth.”
One of the most significant challenges for DarwinAI has been creating the technical tools—and deployment strategies—to help clients in dramatically different industries. This is where AWS has been particularly helpful. “AWS really helps when it comes to the flexibility we have in aligning with these different operational scenarios,” says Wong. “There are ones that require more computational resources because, for example, their manufacturing and inspection tasks are more complex. Or they have more plants, or they have more sensors. With AWS being so flexible and dynamic in serving these particular needs, it makes our life much easier to be able to customize different solutions for different partners.”
Looking forward, Wong believes that DarwinAI will be able to continue to serve an array of clients in even more diverse industries. “I think the key thing that makes us particularly unique is just how integrative our underlying technologies are to tailoring for specific needs,” says Wong. “The way our technology platform has been created allows for very tailored, unique solutions catered to very specific operational requirements. It’s not the one-size-fits-all, big-box-store approach; customization and tailor-made solutions is a very core part of what we’re enabling.”