AWS Startups Blog
How OAG Analytics Leverages AI and Machine Learning to Optimize the Profitability of Oil and Gas Wells
The U.S. surpassed Saudi Arabia as the world’s largest oil producer, while also achieving the lowest emissions levels in 25 years due to natural gas replacing coal fired power plants. The Permian Basin in West Texas is the largest unconventional oil field in the U.S. and is on track to produce more oil than Iraq by 2020. Because of our abundant supply of natural gas, the United States is now also the leading exporter of natural gas to other countries that still run on coal, expanding our role in rapidly reducing carbon emissions on a global scale. A thriving energy sector in the United States will have positive implications worldwide. Unconventional development combines horizontal drilling with multi-stage fracking and helped set the U.S. oil & gas industry on a trajectory to fulfill 58% of global fuel demand growth by 2020. Unconventional development also introduced more complexity into planning and operating wells, requiring new tools that analyze more data to maximize profitability.
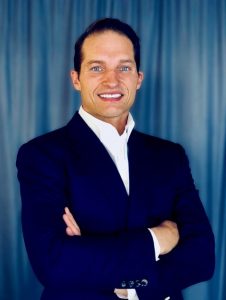
Luther Birdzell, Founder and CEO of OAG Analytics
“Full-stack unconventional oil and gas fields, like the Permian, are massively complex systems,” says Luther Birdzell, founder and CEO of OAG Analytics. “Drilling miles deep and miles across the earth, pumping millions of pounds of sand suspended in tens of thousands of barrels of fluid at high enough rates and pressures to break up solid rock generates a lot of complex data and, when done well, a lot of oil, gas, and dollars.”
The logistics of planning a single well are very complex. Field development planning exponentially increases that complexity with decisions such as where to drill, how many wells to drill, well design, cost, and space between wells. Advanced analytics and cloud compute enable oil and gas companies to evaluate more scenarios to improve drilling unit economics.
“Many upstream experts over the past six years have shared that there are 3,000 to 5,000 variables that affect the production of an oil and gas well,” says Birdzell. “In the best case scenario, we have 300 useful columns of data, and it’s usually closer to 50. Most geophysicists and engineers know the approximate order of the top eight to ten production drivers, OAG helps them quantify and isolate the contribution from each of these drivers, creating new subsurface insights. AI and machine learning identify new opportunities to de-risk the subsurface, increase production, and reduce D&C costs by finding complex relationships within the data that we humans cannot.”
In 2013, Birdzell saw an opportunity for AI and machine learning to deliver unprecedented value to the oil and gas industry. He formed OAG Analytics to create an AI platform that enables oil and gas companies to use more of their data to help solve critical problems like well spacing.
“Our mission is to facilitate collaboration between data scientists and oil and gas subject matter experts,” says Birdzell. “These teams have data trapped in different silos, relying on legacy desktop software to help them analyze different pieces of the puzzle.” The OAG-Amazon SageMaker integration enables customers to unify their datasets and create proprietary analyses using virtually unlimited compute. “Standardizing on SageMaker creates an ideal collaborative environment with our customers. It enables them to get value from these complex technologies quickly with out-of-the-box OAG AI Apps including Well Spacing and Predictive Maintenance and the option to add their own proprietary IP in the future. This unique balance of power and extensibility helps customers break down data silos and improve strategic decisions that impact their balance sheets and reserve reports.”
Unlike consumer-centric AI and machine learning applications, think Google ads and Amazon product recommendations, that automate billions of trivial decisions per day, large oil and gas companies typically make hundreds of decisions per year that impact billions of dollars. “Fields are commonly delineated into 1-2 square mile drilling units, each of which costs approximately a hundred million dollars to develop,” says Birdzell. And in today’s tighter commodity market, the margin for error in capital allocation is dangerously thin. “Until recently, companies relied heavily on trial and error to improve well spacing, which was good enough at high commodity prices. The current low price environment is tightening capital and rewarding innovation.”
Birdzell compares these market conditions to the breaking of the dot-com bubble in the early 2000s, when investors started restricting their investments to companies with a credible path to profitability. It’s an apt comparison, and Birdzell should know: He got his start as a software consultant to Fortune 500 companies in what he calls “the last wave of dot-com development.”
Birdzell learned a crucial lesson: If you want to create value in a high-stakes environment, you have to empower your customers. “It’s about democratizing solutions to critical problems,” says Birdzell. “If you can take something really complex and make it point and click, you can create new markets. Done correctly, this approach scales across large enterprises and maximizes value creation.”
This is especially important for the oil and gas industry, which has historically been slow to adopt new software technology. But Birdzell points out an important caveat to that claim: the industry has also been fast to follow. “EOG and Anadarko are two examples of companies that have proven the value of applying AI to oil field economics by building their own platforms. OAG takes the years of development and tens of millions of dollars of cost out of the equation, enabling companies to get more value more quickly. OAG has helped several of the top 20 U.S. operators use AI to improve their economics. This success is gaining attention and motivating more companies to ask if OAG can help them too.”