AWS Startups Blog
Tecton Feature Store Brings DevOps to ML Data
With the popularity of Machine Learning (ML) exploding, a new ecosystem of products and services built to support the complicated processes around building algorithms and implementing their insights into products has emerged. Amazon SageMaker, for example, offers a fully managed service for developers to build, train, and deploy ML models.
But what about the data for machine learning? ML models are only as good as the data that we feed into them, after all. As the complexity of ML models grows and the need for quick implementation into products becomes more urgent, the importance of managing the complete lifecycle of data for ML has come to the forefront of this budding industry.
This is exactly where Tecton sits.
Founded in 2019, Tecton is on a mission to simplify the process of building and productizing data for machine learning, in an effort to make the technology accessible to any company. Instead of having data scientists and data engineers operating in silos and spending months implementing data pipelines, Tecton automates the complete lifecycle of data for ML.
The team behind Tecton saw this problem first hand while creating Michelangelo, Uber’s internal platform for building, optimizing, and launching ML solutions across the company’s many services. Driver ETAs, UberEATs delivery times, and driver-rider matching are all examples of the thousands of operational ML applications running on Michelangelo, each of which take in data from multiple sources to inform insights. After wrestling with the complexity of how to manage that process all the way to production, and building one of the more well-known ML platforms in the industry, the team decided to bring the benefits of a feature store for machine learning to the general market.
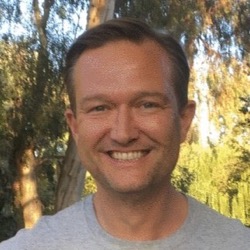
Gaetan Castelein, VP of Marketing
“Without the right platforms and tooling, getting ML models to production is a lengthy and complicated process,” says Gaetan Castelein, VP of Marketing at Tecton. “Even for more advanced technology companies, that process can typically take six months or more, and a majority of the models never make it to production. SageMaker and Tecton are platforms that are designed to get ML models and data to production quickly and reliably. Together, they enable organizations to build ML-powered applications that deliver new magical customer experiences and automate complex business processes.”
At the core of the offering is the idea of a “feature”, which can be described as a predictive data signal that gets passed to an ML model. Previously, data scientists would first collect, explore, and transform data to engineer new features for model training. They would then pass their features to data engineers to re-implement their pipelines with production-hardened code for online serving. The feature lifecycle was a huge time drain.
Tecton provides a platform for data scientists to build great features and serve them to production instantly, using DevOps-like engineering practices. Data scientists build features collaboratively using standard feature definitions that are stored in a Git repo. Tecton then automates the feature pipelines and curates the values in a feature repo. The features can be served instantly for training and online inference. Similar to how Amazon SageMaker speeds up the time to production for building and testing ML models, Tecton accelerates the time to production for ML features. Whether it’s batch, streaming, or real-time data, Tecton can process it, curate it, and pass it to customer’s ML models for real-time inference.
“It’s not just about getting a model out into the world. ML startups like Tecton are thinking about efficiency at every stage of production. The results speak for themselves,” says Allie Miller, US Head of ML Business Development, Startups and Venture Capital at AWS.
“Businesses have seen success combining SageMaker and Tecton, creating an integrated platform for operational machine learning,” per Castelein. “For example, one of our customers is using SageMaker and Tecton to build new models in under 2 months, while significantly increasing the accuracy of predictions.”
If the expansion of ML applications throughout society is any indicator, it would seem the team at Tecton has a bright future ahead of them. And by easing the effort needed to gather and productize data for ML models, they’re likely accelerating the growth of an already exploding industry.