AWS Public Sector Blog
When Data Unlocks the Good for Society
Data can be a powerful driver in solving social issues. The AWS Cloud Credits for Research Program seeks to remove barriers and enable researchers to do their best work, bringing them closer to the answers.
The program offers grants to:
- Build cloud-hosted, publicly available, science-as-a-service applications, software, or tools to facilitate future research initiatives and research across communities;
- Perform proof-of-concept or benchmark tests evaluating the efficacy of moving research workloads or open datasets to the cloud;
- Educate a broader community on the usage of cloud for research workloads via workshops or tutorials.
DrivenData and the Data Science for Social Good Fellowship are two entities using research credits to make a difference for society.
Challenging thousands of data scientists to build a better world
DrivenData is an organization that runs online machine learning challenges where data scientists from around the world compete to build the best algorithms to address social issues. The team also works with individual organizations to help accelerate their unique social missions.
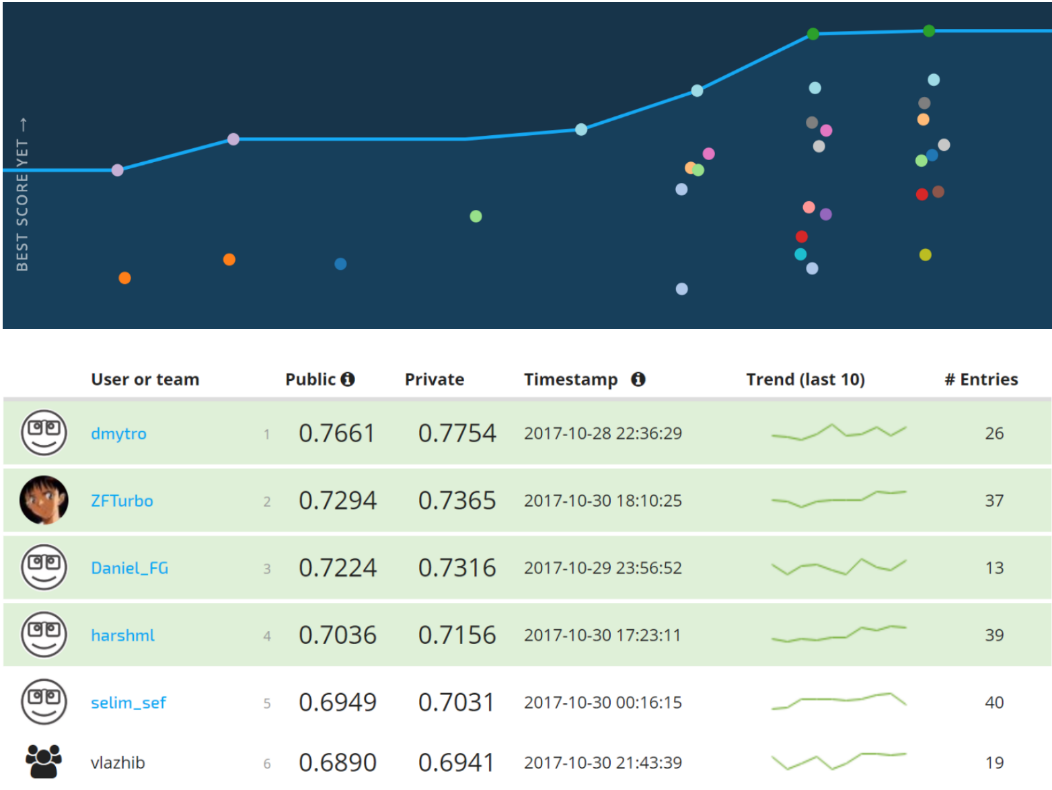
Figure 1: Data scientists around the world compete to build algorithms for the public good. All prize-winning models from past DrivenData competitions are publicly available under an open source license.
Last year, DrivenData ran a data science challenge with the Addario Lung Cancer Foundation focused on building an open source software application for lung cancer detection. The $100,000 Concept to Clinic event aimed to bridge the artificial intelligence (AI) application gap and to make AI advances useful not just for data scientists interested in cutting-edge technology, but for clinicians on the front lines of lung cancer detection, and the patients they serve. More than 600 developers signed up to partake in the challenge, and transformed a sketch to a prototype in seven months.
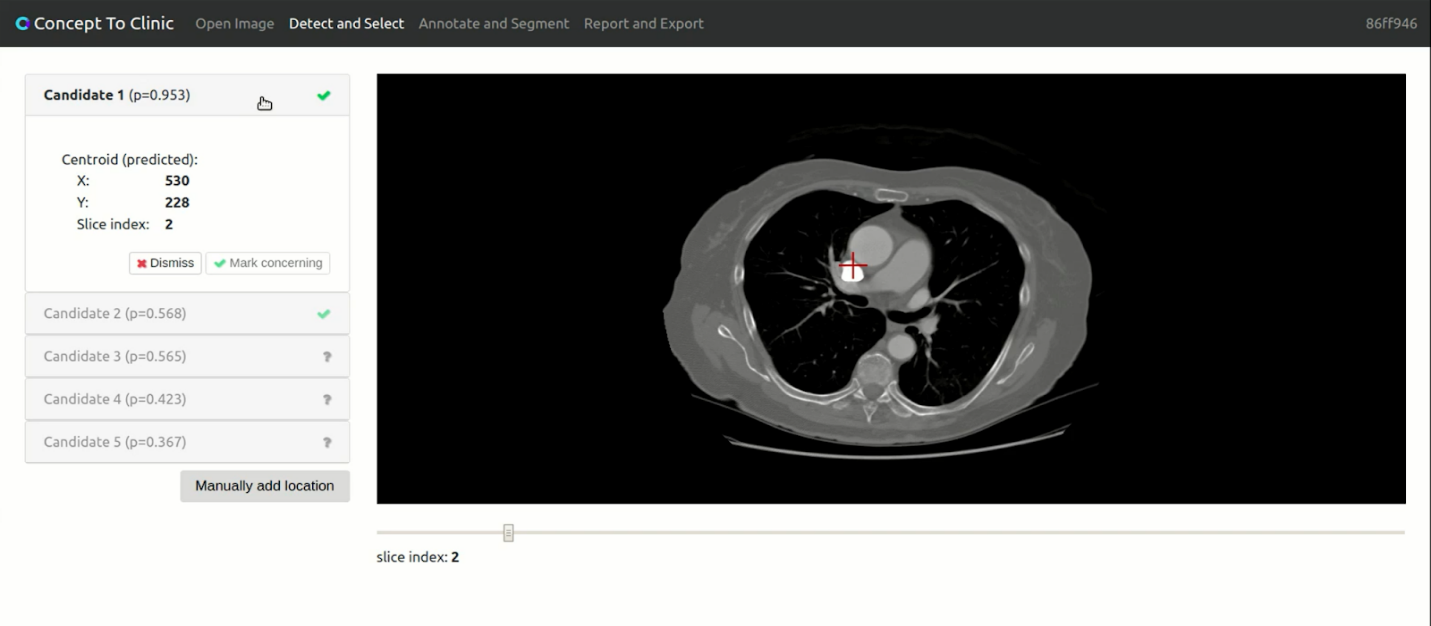
Figure 2: The application above is one of the open source projects DrivenData maintains for the data science, machine learning, and software development communities.
“This work would not be possible without cloud computing resources. AWS research credits have enabled us to collaborate on compute-intensive machine learning projects, store large datasets (while maintaining relatively fast access), and provide hosting and global access to our online platform,” said Greg Lipstein, co-founder and business development head at DrivenData. “Thousands of data scientists, developers, and passionate technologists have contributed their skills to build a better world on top of AWS.”
Driving social change, with the help of data, algorithms, computational infrastructure, and people
Data Science for Social Good (DSSG) is a global network of data science centers that leverage machine learning and big data to solve real-world problems with social impact. Recently, 40 DSSG fellows from around the world worked on data science projects hosted at the University of Chicago and the Nova University School of Business and Economics in Portugal, focused on education, health, public safety, unemployment, transportation, and more.
One of the projects, with Portugal’s Institute for Employment and Vocational Training (Instituto do Emprego e Formação Profissional – IEFP), sought to develop a predictive model to estimate the risk of unemployed individuals becoming long-term unemployed. Persons identified by the model as at-risk were flagged for career counseling and job retraining, to help them re-enter Portugal’s workforce. The project is part of a broader effort by the IEFP to develop a roadmap for unemployment management across all of Portugal.
How it works
The team created a prototype that analyzes risk factors collected from IEFP’s interactions with subjects. The system is built on 11 years of transactional data from 64 million interactions with about 3.5 million people living in Portugal. The team also designed a pipeline that prepares data for entry into the predictive model, adding a feature to calculate contributions of each individual’s attributes to a final risk score using the SHapley Additive exPlanation (SHAP) framework.
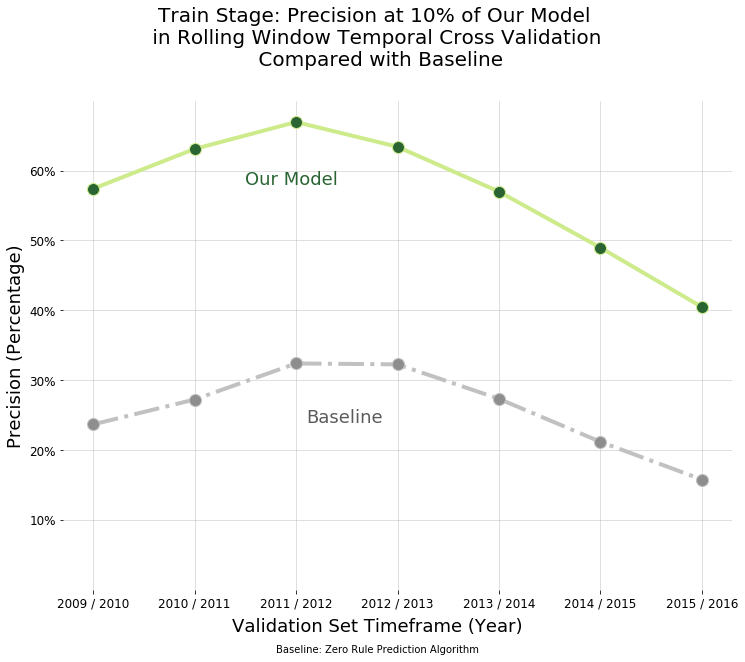
Figure 3: Performance of final model configuration when trained on different 2-year timeframes and validated on subsequent 2-year validation timeframes (x-axis). Baseline – the proportion of individuals registered at IEFP who are long-term unemployed.
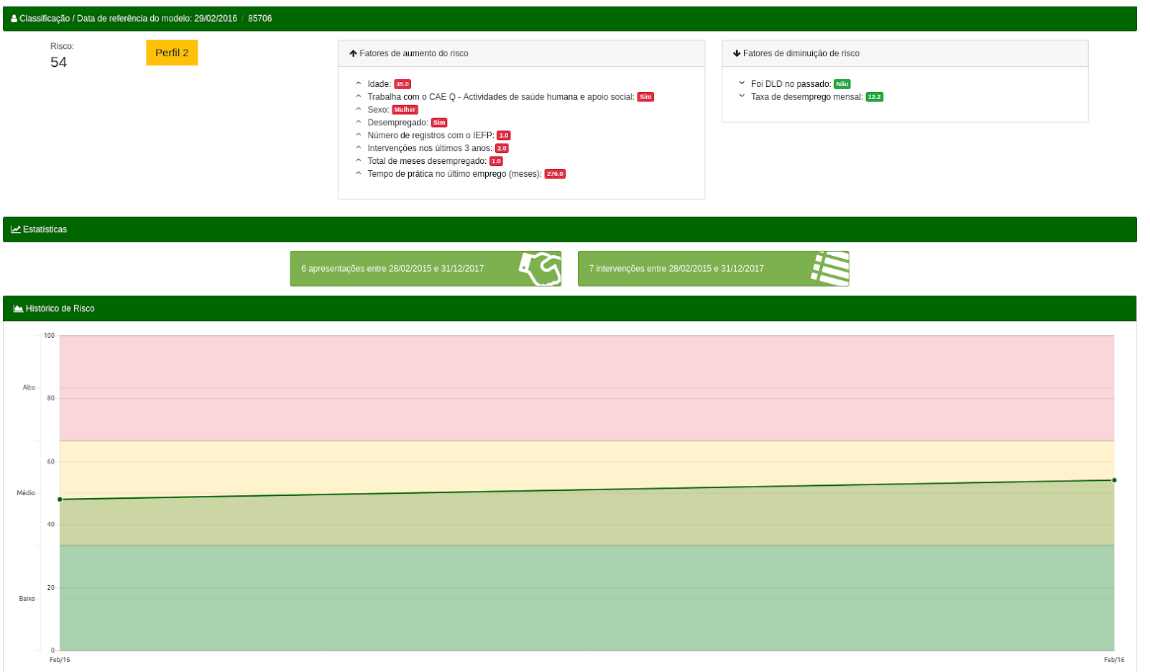
Figure 4: The image shows the classification profile of an individual (top left), along with features which most significantly affect that classification (top right), and a graph of the individual’s risk score over time, with band colors for risk level.
“ We appreciate having access to the cloud and its flexible and scalable computational infrastructure for our projects, which sometimes come with terabytes of data and require computationally-intensive tasks. The summer fellowship funded through the AWS Cloud Credits for Research was a great vehicle to mobilize the stakeholders to engage in preparing the data and develop an understanding of the operational context of the IEFP and the possibilities of machine learning to help supporting the unemployed. In the next 12 months, our goal is to enrich the feature set, achieve a better performance @k % of the population for the predictive model and test it in practice, and develop a recommender system to enable more data-driven counseling. We are very excited by both the research part of the developing the system and the possibility to create a measurable impact in the IEFP,” said Leid Zejnilović, Ph.D., Director of Data for Social Good Europe.
Have an idea for how you can use tech for good? Apply for AWS Credits for Research!