AWS Contact Center
Optimizing your knowledge base for Amazon Q in Connect
Generative AI is revolutionizing the landscape of customer interactions, offering unprecedented capabilities in assistance and problem solving. This innovative technology is particularly transformative in the realm of contact centers, where it empowers agents to quickly support customers and increase satisfaction. By analyzing and generating human-like text, generative AI allows contact centers to automate solution and responses to assist agents in handling complex inquiries with greater precision and personalization. A study by the Boston Consulting Group (BCG) highlights the significant impacts of generative AI on customer service, suggesting that its implementation could lead to a 30% to 50% increase in efficiency.
To support this, AWS launched Amazon Q, a generative AI–powered assistant designed for work that can be tailored to your business to have conversations, solve problems, generate content, and take actions using the data and expertise found in your company’s systems. Amazon Q is available directly within Amazon Connect to provide this same level of assistance to your contact center.
In this blog post, you will learn about Amazon Q in Connect, how it works, and how to optimize your knowledge base to maximize impact using our generative AI capabilities.
What is Amazon Q in Connect?
Amazon Q in Connect provides real-time agent assistance functionality across voice and chat channels in Amazon Connect. With generative AI-powered recommended responses, actions, and deep links to more information, agents can spend more time listening and solving customer inquiries and less time searching and finding answers. This feature leverages your unique knowledge base content to generate synthesized responses that agents can deliver via voice or chat back to customers and also guide them by outlining steps to take to resolve the customer inquiry.
How does it work?
Amazon Q in Connect uses several machine learning (ML) tools to help your agents come up with responses and solutions. First, it determines what the customer intent is by using a machine learning model — trained using thousands of hours of contact center transcripts. Once it knows what the customer needs help with, Amazon Q scans your knowledge base to find relevant documents to support the agent through a process called semantic matching. This process synthesizes these documents to pick out the most important parts that answer the customer’s question.
Next, it takes the problem identified, bits of the conversation, and information from your documents and hands them over to Amazon Bedrock. Here, Amazon Q gives specific directions, otherwise known as prompt engineering, to Amazon Bedrock to create responses and solutions that really work for your agents. All these steps involve machine learning and large language models working together in real-time to make sure your agents have the help they need without the need to build an integration to other AWS services yourself, making it effortless to turn on generative AI features in your contact center.
Beyond the real-time agent assistance provided by monitoring active conversations, agents can also seek help from Amazon Q in Connect by manually entering a question into the search bar. This gives agents the ability to follow up with natural language conversation and get the answers they need, without having to dive into the knowledge base to find and parse through documents. Agents can use the manual search functionality both on and off of a contact. This allows agents completing after-call work (ACW), back-office work, or follow-up tasks, to have Amazon Q in Connect available to them.
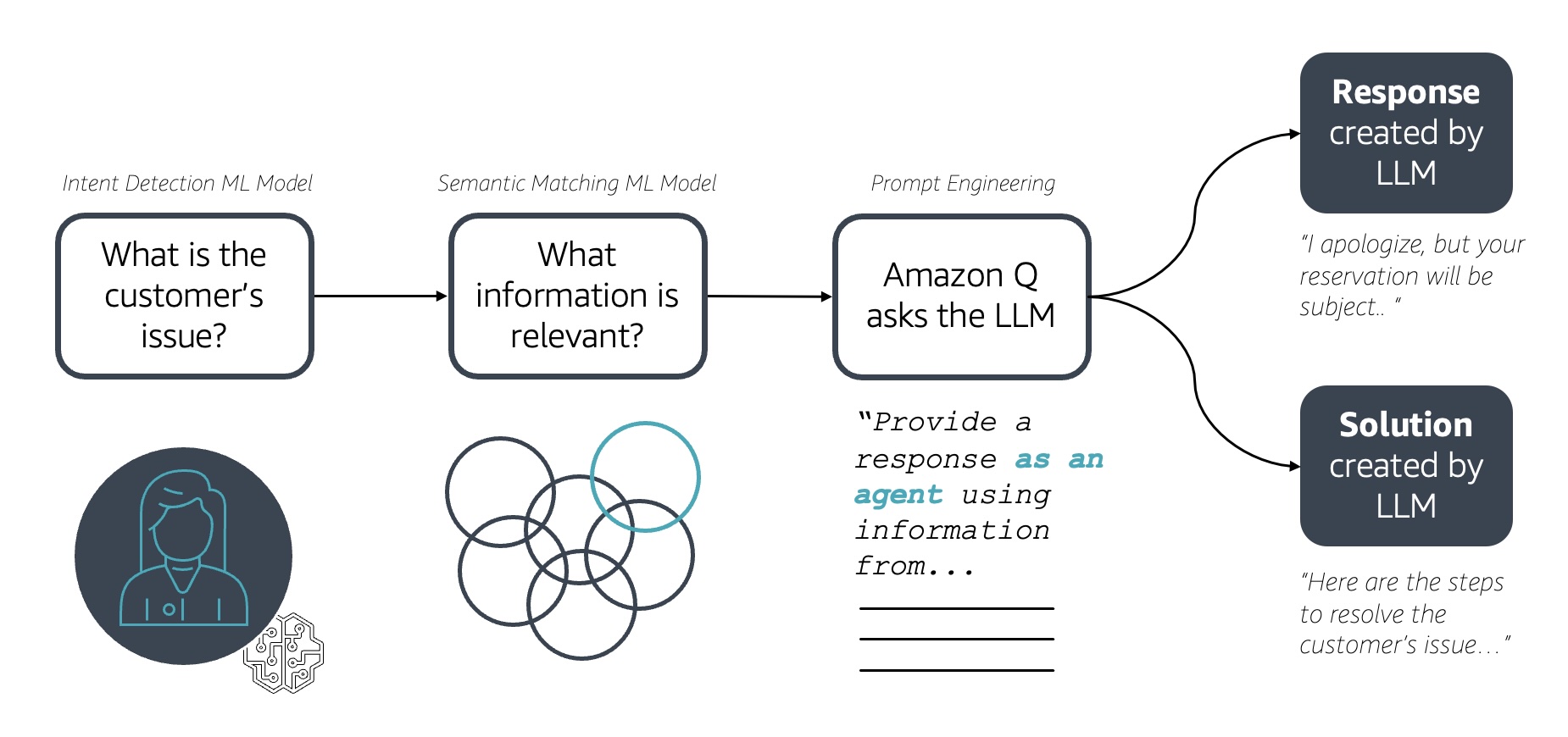
Figure 1. Diagram of how Amazon Q in Connect works
Understanding what your contact center needs are
It is essential to have a deep understanding of the specific needs and common inquiries within your contact center to effectively optimize your content for Amazon Q in Connect. This understanding not only informs the content that should be included in your knowledge base but also ensures that this content is directly aligned with what your customers are asking your agents.
Amazon Connect offers features such as Contact Lens to help you dive-deep into your contact center. Contact Lens provides contact center conversational analytics that can help you transcribe customer conversations, provide generative AI-powered post-contact summaries, analyze customer sentiment, discover top contact drivers, redact sensitive data, and more, natively within Amazon Connect. Regularly reviewing insights from Contact Lens helps ensure that Amazon Q in Connect can effectively address the essential topics your customers are inquiring about. A key feature is theme detection which can provide valuable insights into the common themes and topics that emerge from your customer interactions.
By leveraging theme detection you can:
- Identify common inquiries: Discover the most frequently asked questions and the issues that customers are calling about. This can help in tailoring your content to address customer asks.
- Understand customer sentiment: Gauge the sentiment behind customer inquiries to better understand their frustrations or areas where agents might need more assistance. This can support you in ranking which content to start updating first.
- Spot emerging trends: Stay ahead of the curve by identifying new or evolving customer needs and questions, allowing your knowledge base to be proactive rather than reactive. This can guide you in prioritizing new content to support agents.
In order to best benefit customers and agents, you should regularly review and update your content to ensure it is relevant, up-to-date and comprehensive. Without accurate information on a topic, Amazon Q in Connect cannot provide appropriate guidance.
In addition, integrating feedback from agents is also crucial in refining your knowledge base. Your agents have the ability to provide feedback directly from the recommendations they receive using the thumbs up or thumbs down icons. These trigger the PutFeedback API showing if a recommendation is helpful or not and can be tracked through AWS CloudTrail. This feedback offers a unique perspective on where additional information or clarification could further improve the resourcefulness and effectiveness of your content — making it more valuable for both your agents and customers.
How to optimize your knowledge base content
Optimizing your content is key for making the most out of Amazon Q in Connect. Content that is challenging for humans to comprehend poses the same challenge for AI, impacting the ability to assist your agents effectively. Here are a several strategies for refining your existing content to improve your use of Amazon Q in Connect:
Structure your content
Well-structured content helps guide Amazon Q in Connect to providing your agents with more refined responses. Look into breaking up long sections to shorter focused paragraphs centered on one idea and break down complex processes into clear steps by using bulleted or numbered lists when appropriate.
Simplify language and be specific
Be sure to write clearly and simply without complex vocabulary or sentence structures and steer away from making broad, vague statements. Plain, straightforward writing is easier for Amazon Q in Connect and your agents to comprehend.
Supplement visuals with descriptions
Provide descriptive text to supplement visuals like images, charts, and graphs that are referenced in your documentation. It’s important to add captions and summaries directly to your documents to help Amazon Q in Connect better interpret this content.
Define terms and reference unknown terminology
Include definitions for acronyms, abbreviations, and uncommon terms unique to your business on first use in each article. Connect these terms to the phrases that your customers use to aid the understanding of Amazon Q in Connect.
Use examples
Always illustrate concepts with specific use cases by using examples. By providing these details liberally, you ground how well Amazon Q in Connect understands your business, as well as the agent’s expected responses and actions.
Avoid tables
Refrain from using tables and spreadsheets in your content where possible. Tabular data is best described in written paragraphs or bullets to improve the ability for Amazon Q in Connect to understand this information.
Additional best practices when using Amazon Q in Connect
Avoid conflicting information
Provide only the most current version of a document, rather than every prior draft. Amazon Q in Connect synchronizes with your knowledge source based upon configuration settings you select (e.g. every 1 hour) or implement as part of a custom integration. If you re-use the same content across multiple documents, verify the details are consistent. This allows Amazon Q in Connect to provide consistent and accurate recommendations.
Content segmentation
Managing a vast array of information becomes increasingly challenging, especially when different lines of business require access to specific content. It’s crucial to keep your knowledge base organized and efficient for both your agents and Amazon Q in Connect. You can do this by leveraging content segmentation. By leveraging the UpdateSession API, you can filter out content using tags based on custom criteria, such as customer types, queues, lines of business, and more. This allows for more precise filtering, ensuring only relevant information is presented to the agent based on what is known about that interaction.
Conclusion
Optimizing your knowledge base for Amazon Q in Connect is an ongoing journey towards enhancing your customer interaction and support. By focusing on creating clear, accessible content, understanding the needs of your contact center, and implementing best practices, you set a solid foundation in allowing Amazon Q in Connect to make your agents superhuman. Stay agile, stay informed, and let your knowledge base be a dynamic evolving repository that your agents and generative AI-powered capabilities like Amazon Q in Connect can leverage.
Resources to get started with Amazon Q in Connect
- Do you want to see Amazon Q in Connect in action? Check out this end to end demo of how Amazon Q in Connect can help your agents provide exceptional customer experiences.
- Do you want to learn how to activate Amazon Q in Connect in your instance? The Amazon Connect Admin Guide provides a comprehensive overview of enabling Q in Connect and its features. Learn more at Enable Amazon Q in Connect for your instance.
- Do you want to get hands-on with Amazon Q in Connect? Follow this Amazon Q in Connect workshop to learn how to activate, set up your domain, connect your content, and deliver those AI-generated response and solutions to your agents.
- Do you want to learn what content is supported with Amazon Q in Connect? Amazon Q in Connect supports ingestion of various file types from your knowledge base. Learn more at Supported content types.
Ready to transform your customer service experience with Amazon Connect? Contact us.
Author bio
![]() |
Alex Schrameyer (he/him) is a Worldwide Solutions Architect Lead for Agent Experience at Amazon Web Services (AWS) based in Indianapolis, Indiana. He believes that exceptional agent experiences are the cornerstone of outstanding customer service, and focuses on creating environments where agents excel and customers are delighted. Alex enjoys traveling around the world, and you might find him at your local baseball stadium or theme park. |
![]() |
Freddy Jimenez (he/him) is a Senior Solutions Architect at AWS specialized in Amazon Connect based in Chicago, Illinois. He works with customers across various industries on leveraging Amazon Connect to revolutionize their customer experiences. He has deep expertise in the contact center technology space with experience in operations and professional services. Freddy finds joy in running, exploring new destinations through travel, and cherishing moments with his miniature Goldendoodle. |
![]() |
Prescott Wright (he/him) is a Principal Product Manager for Amazon Q in Connect based in Bozeman, Montana. With over a decade of experience in developing Contact Center as a Service (CCaaS) solutions, Prescott is passionate about providing tools that empower agents to deliver exceptional customer service. Prescott enjoys trail running, skiing, and exploring the beautiful Montana wilderness while camping with his children. |