AWS Public Sector Blog
EO/GIS Training Lab with On-demand Services from AWS
Q&A with Professor Albert Remke from the Institute for Geoinformatics, University of Münster, Germany
In 2017, a group of students at the Institute for Geoinformatics at the University of Münster embarked on an ambitious project: to build an Earth Observation Infrastructure on AWS that would support disaster preparedness for flooding situations in Europe. To do this, they needed to:
- Manage and process Earth observation data from the European Union’s Copernicus programme, the world’s largest earth observation programme;
- Use a number of data sources to perform flood risk analysis; and
- Make the technology available via a Web GIS.
We asked Professor Remke about the project, how AWS was used, and his recommendations for similar projects in the following Q&A:
Q1. Can you describe the project use case?
The goal of the project was to give students the opportunity to gain a comprehensive understanding of the European Copernicus Earth observation infrastructure. Every day, Copernicus delivers massive amounts of sensor data from orbit (space component), which are combined with data from other sources (in situ component) and processed into information products (services component). This requires a scalable infrastructure that can dynamically respond to resource demands. Cloud technologies are a prerequisite for this type of infrastructure.
The automated detection of water surfaces from Sentinel 1 (S1) radar data served as a use case. The S1 radar signal is reflected by rough surfaces and measured by the sensor. Since there is hardly any backscatter of signals on smooth water surfaces, these areas are easy to detect with S1 data. In addition, the radar signal penetrates the clouds effortlessly. The availability of usable data is better than with optical sensors, which only provide suitable data during weather conditions with good visibility of the Earth’s surface.
The data had to be combined with hydrological and meteorological in-situ data in a web application, which supports disaster management in severe flooding situations. The project’s focus was less on the data analysis methodology, and more on the technical architecture for processing large amounts of data.
Q2. Speaking as an educator, why was AWS a good choice for this project and what problems were you trying to solve when you selected an AWS solution?
The development of technical skills in the field of cloud technologies was one of the key learning objectives of the project. The students had to cope with an extensive technology stack. For the students as well as for the teachers, the documentation and the support of the AWS infrastructure were helpful. AWS also provides a foundation for using Esri ArcGIS technology, which was used for data analysis and visualization.
Q3. Were your students already familiar with AWS technologies and what was the general approach of the team?
The 15 geoinformatics students had no experience with cloud technologies prior to the project. Mentoring was carried out by three qualified software engineers from the Institute of Geoinformatics, con terra GmbH and 52° North, who also had no practical experience with AWS.
Three teams were formed, each supported by two mentors. The first team dealt with all aspects of data management, as well as scaling and cost management in the cloud. The second team was responsible for implementing the process chain for pre-processing and analysis of Sentinel-1 data. The third group dealt with the visualization of results, the integration of in-situ data, and the implementation of the web application.
All teams worked according to an agile methodology and met weekly at JourFixe meetings. Each student had around 150 hours to work on the study project.
Q4. Can you describe the solution and the AWS technology used?
The participants successfully implemented the entire process chain for the automated provision of information products from Sentinel-1 data on AWS.
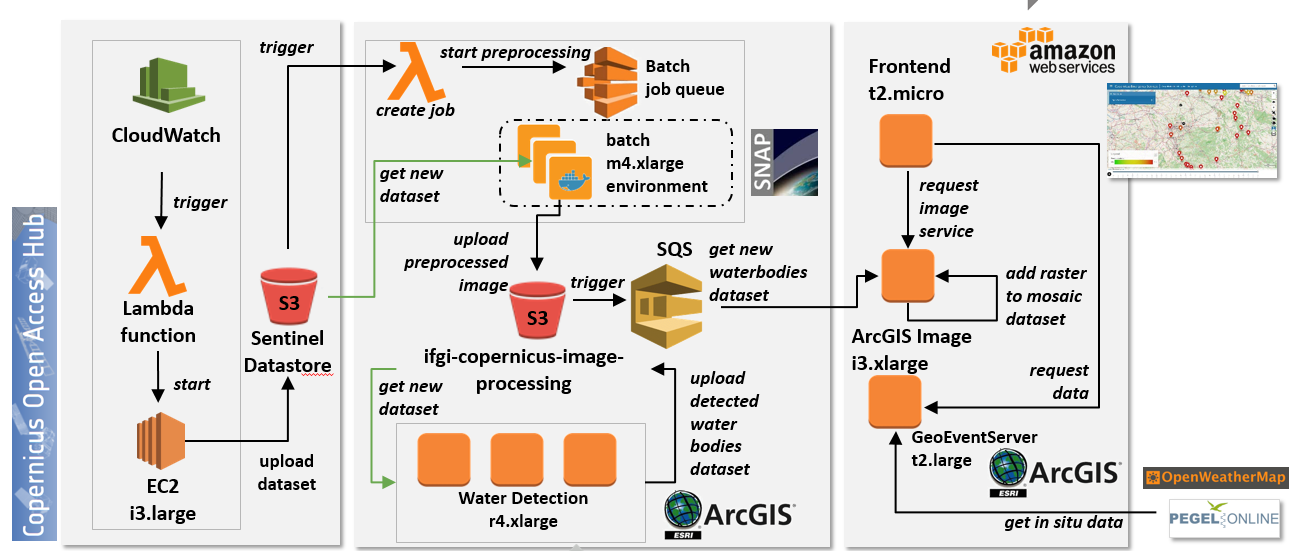
Fig 1. Diagram of AWS Implementation.
This included the automated replication of Sentinel-1 data from the Copernicus Open Access Hub into an S3 bucket. Lambda functions were used to determine the availability of new data and to generate batch jobs for further data pre-processing using Docker images with SNAP tools and Python scripts. ArcGIS and Python were used to detect the open water surfaces from the satellite imagery and to integrate them as a raster dataset into a Mosaic dataset. The orchestration of these processing steps was supported by Amazon Simple Queue Services (SQS). The ArcGIS Geoevent Server was used to obtain real-time data from open data servers (Pegel Online, OpenWeatherMap) and prepare them for further visualization. The Leaflet WebApplication was installed on a t2.micro instance.
Q5. What did you and your students learn from the project?
Students now have good insight into AWS and hands-on experience developing AWS-based Copernicus applications. These skills will be useful to them in the future. The study project demonstrated that data-intensive applications that make use of spatial data infrastructures (such as Copernicus) and integrate with parts of these infrastructures need to be based on cloud and web technologies.
Q6. Were there any highlights for you during this project?
In the beginning, I thought the technology stack might be too demanding for the study project. The mentors’ task was limited to answering questions and providing assistance in critical situations, but I was happy to see that the students were able to accomplish the task independently. The documentation and accessibility of the AWS services and the support we occasionally used allowed us to get started quickly and make good progress.
It also became clear that AWS provides more than infrastructure in terms of storage and virtual machines. Elements such as Lambda functions, AWS Batch, and SQS are helpful for integrating existing and heterogeneous software components into powerful systems.
Thank you for the insight, Professor Remke, and congratulations to University of Münster for winning the City on a Cloud Innovation Challenge award for their senseBox project. Learn more here.
Big Geodata und Cloud – Seminar an der Universität Münster nutzt AWS und Public Datasets
Q&A mit Professor Albert Remke vom Institut für Geoinformatik an der Universität Münster, Deutschland.
Im Wintersemester 2017 startete eine Gruppe von Studenten des Instituts für Geoinformatik an der Universität Münster ein ambitioniertes Projekt: die Umsetzung einer Erdbeobachtungs-Infrastruktur auf AWS, die Katastrophenvorsorge für Hochwasserereignisse in Europa ermöglicht. Für die Realisierung, mussten sie:
- Erdbeobachtungsdaten aus dem Copernicus Programm der Europäischen Union – dem weltweit größten Programm in diesem Bereich – verwalten und prozessieren;
- Diverse Datenquellen nutzen, um eine Risikoanalyse für Hochwasserereignisse zu berechnen;
- Die Analysen und Ergebnisse über Dienste und ein Web-GIS Nutzern verfügbar machen.
Wir haben mit Professor Remke über das Projekt gesprochen, um herauszufinden, wie AWS eingesetzt wurde und was seine Empfehlungen für die Umsetzung ähnlicher Projekte in der Zukunft sind:
Q1. Was war die fachliche Zielsetzung des Studienprojektes?
Das Studienprojekt sollte den Studierenden die Möglichkeit geben, ein umfassendes Verständnis der Europäischen Erdbeobachtungs-Infrastruktur, Copernicus, zu gewinnen. Copernicus liefert täglich enorme Mengen an Sensordaten aus dem Orbit (Space Component), die mit Daten aus anderen Quellen (In-Situ Component) kombiniert und zu Informationsprodukten (Services Component) verarbeitet werden. Dies erfordert eine skalierbare Infrastruktur, die elastisch auf Ressourcenanforderungen reagieren kann. Ohne Cloud-Technologien ist dies kaum möglich.
Als Anwendungsbeispiel diente uns die automatisierte Detektion offener Wasserflächen aus Sentinel 1 (S1) Radardaten. Das S1 Radarsignal wird an rauen Oberflächen reflektiert und vom Sensor gemessen. Da bei glatten Wasserflächen kaum Reflektionen auftreten, sind diese Bereiche mit S1 Daten recht gut zu erkennen. Zudem kann das Radarsignal die Wolken mühelos durchdringen. Die Verfügbarkeit nutzbarer Daten ist daher wesentlich besser als bei optischen Sensoren, die nur bei guter Sicht auf die Erdoberfläche geeignete Daten liefern.
Diese Daten sollten gemeinsam mit hydrologischen und meteorlogischen In-Situ-Daten in einer Web-Applikation für den Katastrophenschutz bereitgestellt werden. Der Schwerpunkt der Arbeiten lag dabei weniger auf der Methodik der Datenanalyse, sondern auf der technischen Architektur zur Verarbeitung großer Datenmengen, also auf der Implementierung der Datenflüsse und Prozessierungsabläufe in einer dynamisch skalierbaren verteilten Laufzeitumgebung.
Q2. Wie bewerten Sie die Nutzung von AWS in Ihrer Rolle als Lehrender? Worauf haben sie geachtet, als Sie sich für AWS entschieden haben?
Die Entwicklung der technischen Fähigkeiten im Bereich der Anwendung von Cloud-Technologien war eines der zentralen Lernziele des Studienprojektes. Die Studierenden hatten hierbei eine umfangreichen Technologie-Stack zu bewältigen. Für die Studierenden wie auch für die Lehrenden waren die sehr gute Dokumentation und der hervorragende AWS Support extrem hilfreich. Zudem stellt AWS auch für die Verwendung der Esri ArcGIS Technologie, die für die Datenanalyse und Visualisierung eingesetzt wurde, eine sehr gute Basis dar.
Q3. Waren Ihre Studenten bereits vertraut mit AWS Technologien? Wie sind sie vorgegangen?
Die fünfzehn Studierenden der Geoinformatik verfügten vor dem Studienprojekt über keinerlei Erfahrung in der Anwendung von Cloud-Technologien. Das Mentoring erfolgte durch drei qualifizierte Softwareingenieure des Institutes für Geoinformatik1, der con terra GmbH2 und der 52°North3, die aber auch noch keine praktische Erfahrung in der Nutzung der AWS Plattform hatten.
Es wurden drei Teams gebildet, die jeweils durch zwei Mentoren unterstützt wurden. Das erste Team befasste sich mit allen Fragen des Datenmanagements (Ingestion, Storage) sowie auch der Skalierung und des Kostenmanagements in der AWS Cloud. Das zweite Team war für die Implementierung der Prozesskette für die Vorverarbeitung und Analyse der Sentinel 1 Daten verantwortlich. Die dritte Gruppe befasste sich mit der Visualisierung der Ergebnisse, der Integration von In-Situ-Daten und der Implementierung der Web-Anwendung.
Alle Teams arbeiteten nach einer agilen Methodik und trafen sich wöchentlich zu JourFixe Meetings. Jedem Studierenden standen ca. 150 Stunden für die Arbeiten im Studienprojekt zur Verfügung.
Q4. Wie sah die technische Lösung aus und welche AWS Technologien kamen zum Einsatz?
Den Teilnehmern des Studienprojektes ist es gelungen, die gesamte Prozesskette zur automatisierten Bereitstellung von Informationsprodukten aus Sentinel 1 Daten in der AWS Umgebung lauffähig zu implementieren.
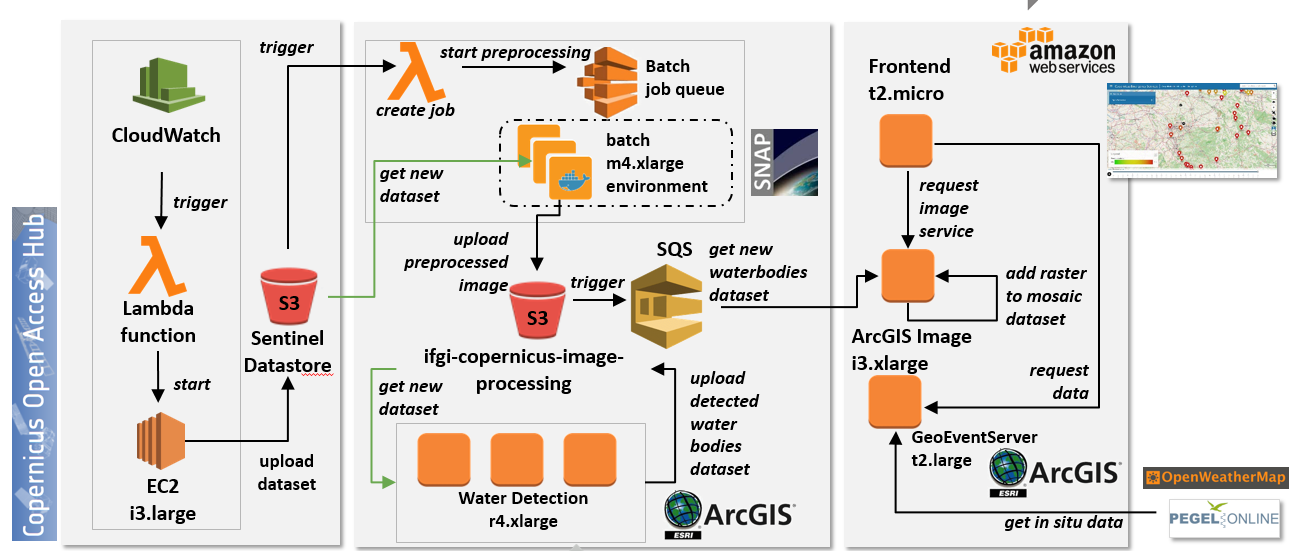
Fig 1. Diagramm der AWS Architektur.
Dies umfasste zunächst die automatisierte Replikation von Sentinel 1 Daten des Copernicus Open Access Hub in einem eigenen S3 Bucket. Hierbei wurden Lambda Functions genutzt, um die Verfügbarkeit neuer Daten festzustellen und Batch Jobs für die weitere Vorverarbeitung der Daten (u.a. terrain correction, speckle filtering) zu generieren, hierbei wurden Docker Images mit SNAP tools und Python Scripts verwendet. ArcGIS und Python wurden genutzt, um die Wasseroberflächen in den Satellitenbildern zu erkennen und als Raster-Datensatz in ein Mosaic Dataset zu integrieren. Die Orchestrierung dieser Verarbeitungsschritte wurde durch AWS Simple Queue Services (SQS) unterstützt. Der ArcGIS Geoevent Server wurde verwendet, um Echtzeitdaten von offenen Datenservern (Pegel Online, OpenWeatherMap) zu beziehen und diese für die weitere Visualisierung aufzubereiten. Die Leaflet Web-Applikation wurde schließlich auf einer t2.micro Instanz installiert.
Q5. Was haben Sie und Ihre Studenten in dem Studienprojekt erreicht?
Die Lernziele des Studienprojektes wurden für alle Beteiligten erreicht und übertroffen. Die Studierenden verfügen nun über einen guten Einblick in AWS und über praktische Erfahrungen in der Entwicklung AWS basierter Copernicus-Anwendungen, die sie in der weiteren Arbeit nutzen werden. Das Studienprojekt machte deutlich, dass datenintensive Anwendungen, die sich der Möglichkeiten von Geodateninfrastrukturen (wie Copernicus) bedienen wollen und selbst zu integralen Bestandteilen dieser Infrastrukturen werden sollen, auf Cloud- und Web-Technologien angewiesen sind.
Q6. Was waren für Sie die Highlights in dem Projekt?
Ich war mir anfangs nicht sicher, ob der Umfang des Technologie-Stacks den Rahmen des Studienprojektes sprengen würde. Die Mentoren hatten lediglich die Aufgabe, Fragen zu beantworten und in kritischen Situationen Hilfestellung zu geben, und es war schön zu beobachten, dass die Studenten weitestgehend selbständig in der Lage waren, die Aufgabe zu lösen. Die gute Dokumentation und Zugänglichkeit der AWS Plattform Services und auch der AWS Support, den wir gelegentlich in Anspruch genommen haben, ermöglichte einen schnellen Einstieg und ein gutes Vorankommen.
Hierbei wurde auch deutlich, dass AWS wesentlich mehr als nur Infrastruktur im Sinne von Speicher und virtuellen Maschinen bereitstellt. Elemente wie Lambda Functions, AWS Batch und SQS sind extrem hilfreich, um vorhandene und heterogene Softwarebausteine zu leistungsfähigen Systemen zu integrieren.
Herr Professor Remke, vielen Dank für diese Eindrücke. Herzlichen Glückwunsch außerdem zum Sieg bei der City on a Cloud Innovation Challenge für das senseBox Projekt der Universität Münster – mehr dazu hier.