Large language models (LLMs) are becoming increasing popular, with new use cases constantly being explored. In general, you can build applications powered by LLMs by incorporating prompt engineering into your code. However, there are cases where prompting an existing LLM falls short. This is where model fine-tuning can help. Prompt engineering is about guiding the model’s output by crafting input prompts, whereas fine-tuning is about training the model on custom datasets to make it better suited for specific tasks or domains.
Before you can fine-tune a model, you need to find a task-specific dataset. One dataset that is commonly used is the Common Crawl dataset. The Common Crawl corpus contains petabytes of data, regularly collected since 2008, and contains raw webpage data, metadata extracts, and text extracts. In addition to determining which dataset should be used, cleansing and processing the data to the fine-tuning’s specific need is required.
We recently worked with a customer who wanted to preprocess a subset of the latest Common Crawl dataset and then fine-tune their LLM with cleaned data. The customer was looking for how they could achieve this in the most cost-effective way on AWS. After discussing the requirements, we recommended using Amazon EMR Serverless as their platform for data preprocessing. EMR Serverless is well suited for large-scale data processing and eliminates the need for infrastructure maintenance. In terms of cost, it only charges based on the resources and duration used for each job. The customer was able to preprocess hundreds of TBs of data within a week using EMR Serverless. After they preprocessed the data, they used Amazon SageMaker to fine-tune the LLM.
In this post, we walk you through the customer’s use case and architecture used.
In the following sections, we first introduce the Common Crawl dataset and how to explore and filter the data we need. Amazon Athena only charges for the data size it scans and is used to explore and filter the data quickly, while being cost-effective. EMR Serverless provides a cost-efficient and no-maintenance option for Spark data processing, and is used to process the filtered data. Next, we use Amazon SageMaker JumpStart to fine-tune the Llama 2 model with the preprocessed dataset. SageMaker JumpStart provides a set of solutions for the most common use cases that can be deployed with just a few clicks. You don’t need to write any code to fine-tune an LLM such as Llama 2. Finally, we deploy the fine-tuned model using Amazon SageMaker and compare the differences in text output for the same question between the original and fine-tuned Llama 2 models.
The following diagram illustrates the architecture of this solution.
Before you dive deep into the solution details, complete the following prerequisite steps:
Common Crawl is an open corpus dataset obtained by crawling over 50 billion webpages. It includes massive amounts of unstructured data in multiple languages, starting from 2008 and reaching the petabyte level. It is continuously updated.
In the training of GPT-3, the Common Crawl dataset accounts for 60% of its training data, as shown in the following diagram (source: Language Models are Few-Shot Learners).
In this section, we cover common ways to interact, filter, and process the Common Crawl dataset.
The Common Crawl raw dataset includes three types of data files: raw webpage data (WARC), metadata (WAT), and text extraction (WET).
Data collected after 2013 is stored in WARC format and includes corresponding metadata (WAT) and text extraction data (WET). The dataset is located in Amazon S3, updated on a monthly basis, and can be accessed directly through AWS Marketplace.
The Common Crawl dataset also provides an index table for filtering data, which is called cc-index-table.
The cc-index-table is an index of the existing data, providing a table-based index of WARC files. It allows for easy lookup of information, such as which WARC file corresponds to a specific URL.
For example, you can create an Athena table to map cc-index data with the following code:
CREATE EXTERNAL TABLE IF NOT EXISTS ccindex (
url_surtkey STRING,
url STRING,
url_host_name STRING,
url_host_tld STRING,
url_host_2nd_last_part STRING,
url_host_3rd_last_part STRING,
url_host_4th_last_part STRING,
url_host_5th_last_part STRING,
url_host_registry_suffix STRING,
url_host_registered_domain STRING,
url_host_private_suffix STRING,
url_host_private_domain STRING,
url_host_name_reversed STRING,
url_protocol STRING,
url_port INT,
url_path STRING,
url_query STRING,
fetch_time TIMESTAMP,
fetch_status SMALLINT,
fetch_redirect STRING,
content_digest STRING,
content_mime_type STRING,
content_mime_detected STRING,
content_charset STRING,
content_languages STRING,
content_truncated STRING,
warc_filename STRING,
warc_record_offset INT,
warc_record_length INT,
warc_segment STRING)
PARTITIONED BY (
crawl STRING,
subset STRING)
STORED AS parquet
LOCATION 's3://commoncrawl/cc-index/table/cc-main/warc/';
# add partitions
MSCK REPAIR TABLE ccindex
# query
select * from ccindex
where crawl = 'CC-MAIN-2018-05'
and subset = 'warc'
and url_host_tld = 'no'
limit 10
The preceding SQL statements demonstrate how to create an Athena table, add partitions, and run a query.
Filter data from the Common Crawl dataset
As you can see from the create table SQL statement, there are several fields that can help filter the data. For example, if you want to get the count of Chinese documents during a specific period, then the SQL statement could be as follows:
SELECT
url,
warc_filename,
content_languages
FROM ccindex
WHERE (crawl = 'CC-MAIN-2023-14'
OR crawl = 'CC-MAIN-2023-23')
AND subset = 'warc'
AND content_languages ='zho'
LIMIT 10000
If you want to do further processing, you can save the results to another S3 bucket.
Analyze the filtered data
The Common Crawl GitHub repository provides several PySpark examples for processing the raw data.
Let’s look at an example of running server_count.py
(example script provided by the Common Crawl GitHub repo) on the data located in s3://commoncrawl/crawl-data/CC-MAIN-2023-23/segments/1685224643388.45/warc/
.
First, you need a Spark environment, such as EMR Spark. For example, you can launch an Amazon EMR on EC2 cluster in us-east-1
(because the dataset is in us-east-1
). Using an EMR on EC2 cluster can help you carry out tests before submitting jobs to the production environment.
After launching an EMR on EC2 cluster, you need to do an SSH login to the primary node of the cluster. Then, package the Python environment and submit the script (refer to the Conda documentation to install Miniconda):
# create conda environment
conda create -y -n example -c dmnapolitano python=3.7 botocore boto3 ujson requests conda-pack warcio
# package the conda env
conda activate example
conda pack -o environment.tar.gz
# get script from common crawl github
git clone https://github.com/commoncrawl/cc-pyspark.git
# copy target file path to local
aws s3 cp s3://commoncrawl/crawl-data/CC-MAIN-2023-23/warc.paths.gz .
gzip -d warc.paths.gz
# put warc list to hdfs
hdfs dfs -put warc.paths
# submit job
spark-submit --conf spark.yarn.appMasterEnv.PYSPARK_PYTHON=./environment/bin/python \
--conf spark.sql.warehouse.dir=s3://xxxx-common-crawl/output/ \
--master yarn \
--deploy-mode cluster \
--archives environment.tar.gz#environment \
--py-files cc-pyspark/sparkcc.py cc-pyspark/server_count.py --input_base_url s3://commoncrawl/ ./warc.paths count_demo
It can take time to process all references in the warc.path. For demo purposes, you can improve the processing time with the following strategies:
- Download the file
s3://commoncrawl/crawl-data/CC-MAIN-2023-23/warc.paths.gz
to your local machine, unzip it, and then upload it to HDFS or Amazon S3. This is because the .gzip file is not splitable. You need to unzip it to process this file in parallel.
- Modify the
warc.path
file, delete most of its lines, and only keep two lines to make the job run much faster.
After the job is complete, you can see the result in s3://xxxx-common-crawl/output/
, in Parquet format.
Implement customized possessing logic
The Common Crawl GitHub repo provides a common approach to process WARC files. Generally, you can extend the CCSparkJob
to override a single method (process_record
), which is sufficient for many cases.
Let’s look at an example to get the IMDB reviews of recent movies. First, you need to filter out files on the IMDB site:
SELECT
url,
warc_filename,
url_host_name
FROM ccindex
WHERE (crawl = 'CC-MAIN-2023-06'
OR crawl = 'CC-MAIN-2023-40')
AND subset = 'warc'
AND url like 'https://www.imdb.com/title/%/reviews'
LIMIT 1000
Then you can get WARC file lists that contain IMDB review data, and save the WARC file names as a list in a text file.
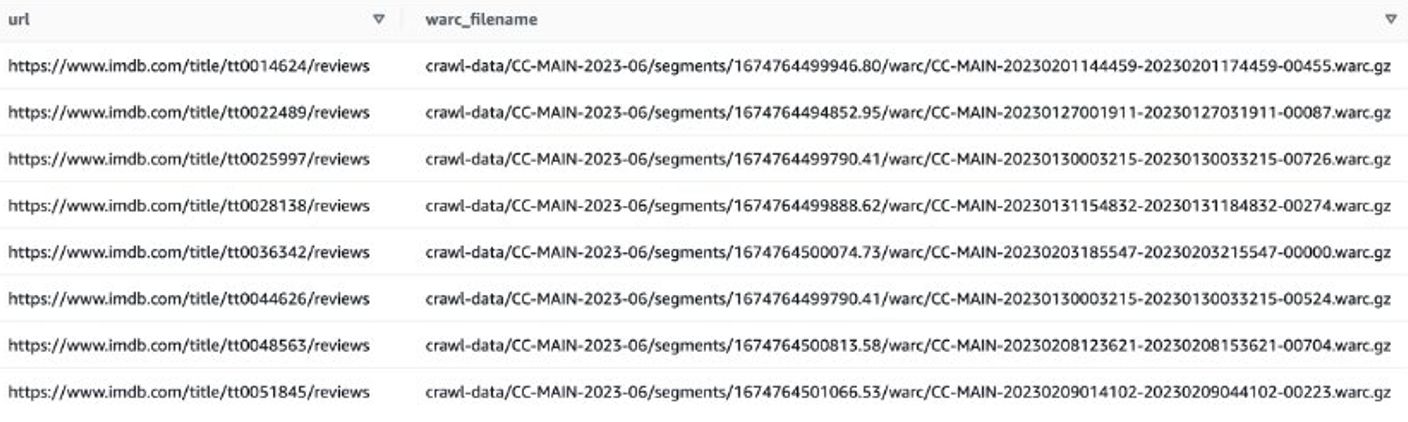
Alternatively, you can use EMR Spark get the WARC file list and store it in Amazon S3. For example:
sql = """SELECT
warc_filename
FROM ccindex
WHERE (crawl = 'CC-MAIN-2023-06'
OR crawl = 'CC-MAIN-2023-40')
AND subset = 'warc'
AND url like 'https://www.imdb.com/title/%/reviews'
"""
warc_list = spark.sql(sql)
# write result list to s3
warc_list.coalesce(1).write.mode("overwrite").text("s3://xxxx-common-crawl/warclist/imdb_warclist")
The output file should look similar to s3://xxxx-common-crawl/warclist/imdb_warclist/part-00000-6af12797-0cdc-4ef2-a438-cf2b935f2ffd-c000.txt
.
The next step is to extract user reviews from these WARC files. You can extend the CCSparkJob
to override the process_record()
method:
from sparkcc import CCSparkJob
from bs4 import BeautifulSoup
from urllib.parse import urlsplit
class IMDB_Extract_Job(CCSparkJob):
name = "IMDB_Reviews"
def process_record(self, record):
if self.is_response_record(record):
# WARC response record
domain = urlsplit(record.rec_headers['WARC-Target-URI']).hostname
if domain == 'www.imdb.com':
# get web contents
contents = (
record.content_stream()
.read()
.decode("utf-8", "replace")
)
# parse with beautiful soup
soup = BeautifulSoup(contents, "html.parser")
# get reviews
review_divs = soup.find_all(class_="text show-more__control")
for div in review_divs:
yield div.text,1
if __name__ == "__main__":
job = IMDB_Extract_Job()
job.run()
You can save the preceding script as imdb_extractor.py, which you’ll use in the following steps. After you have prepared the data and scripts, you can use EMR Serverless to process the filtered data.
EMR Serverless
EMR Serverless is a serverless deployment option to run big data analytics applications using open source frameworks like Apache Spark and Hive without configuring, managing, and scaling clusters or servers.
With EMR Serverless, you can run analytics workloads at any scale with automatic scaling that resizes resources in seconds to meet changing data volumes and processing requirements. EMR Serverless automatically scales resources up and down to provide the right amount of capacity for your application, and you only pay for what you use.
Processing the Common Crawl dataset is generally a one-time processing task, making it suitable for EMR Serverless workloads.
Create an EMR Serverless application
You can create an EMR Serverless application on the EMR Studio console. Complete the following steps:
- On the EMR Studio console, choose Applications under Serverless in the navigation pane.
- Choose Create application.

- Provide a name for the application and choose an Amazon EMR version.
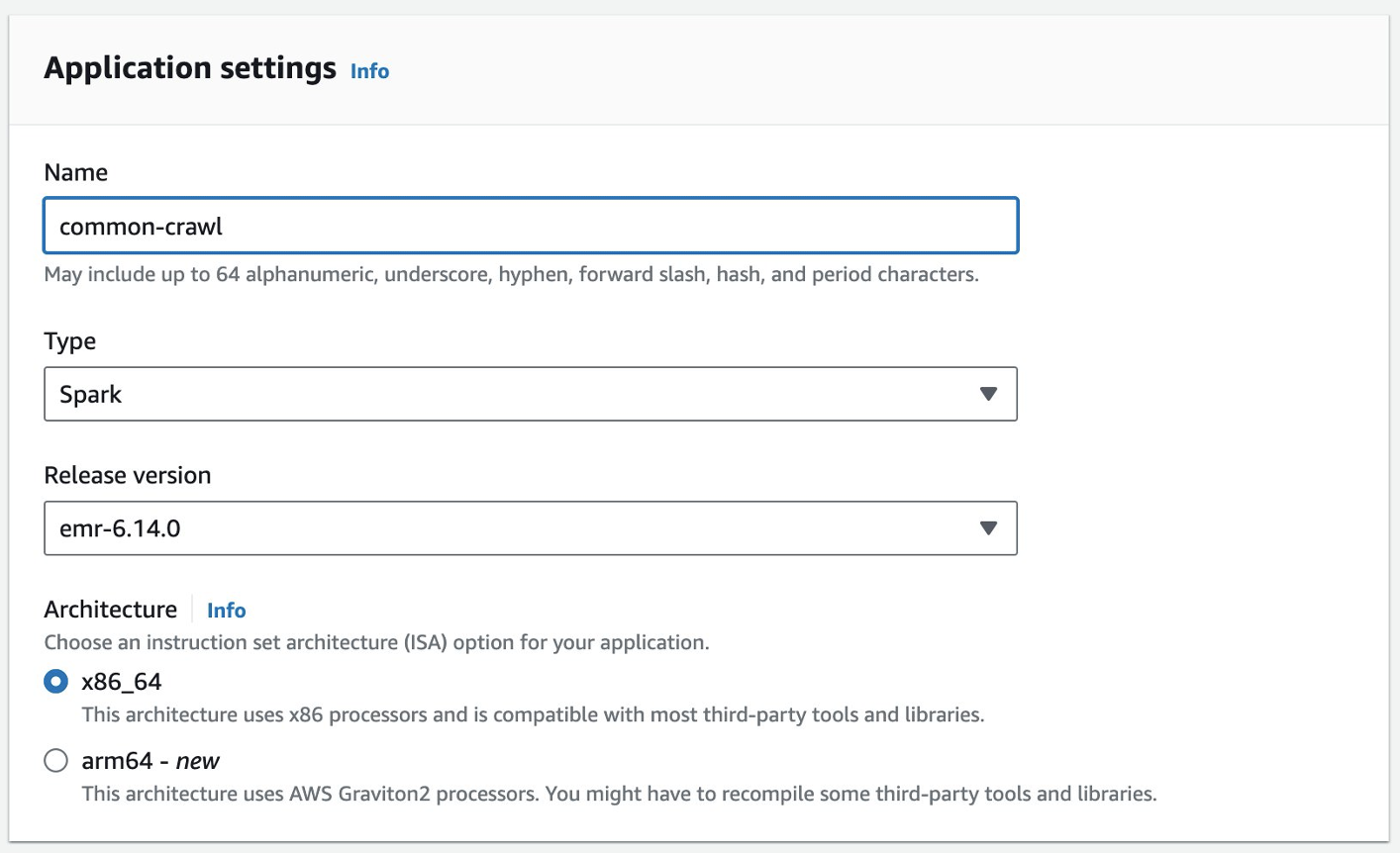
- If access to VPC resources is required, add a customized network setting.
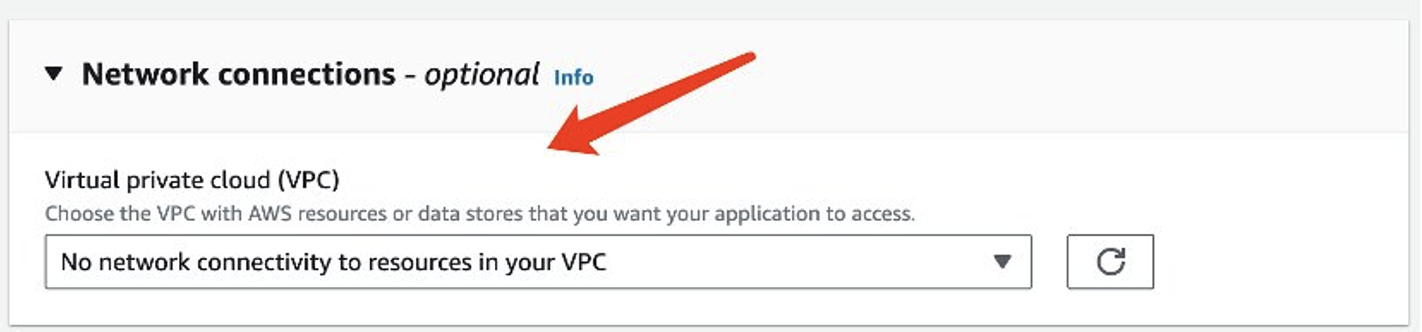
- Choose Create application.
Your Spark serverless environment will then be ready.
Before you can submit a job to EMR Spark Serverless, you still need to create an execution role. Refer to Getting started with Amazon EMR Serverless for more details.
Process Common Crawl data with EMR Serverless
After your EMR Spark Serverless application is ready, complete the following steps to process the data:
- Prepare a Conda environment and upload it to Amazon S3, which will be used as the environment in EMR Spark Serverless.
- Upload the scripts to be run to an S3 bucket. In the following example, there are two scripts:
- imbd_extractor.py – Customized logic to extract contents from the dataset. The contents can be found earlier in this post.
- cc-pyspark/sparkcc.py – The example PySpark framework from the Common Crawl GitHub repo, which is necessary to be included.
- Submit the PySpark job to EMR Serverless Spark. Define the following parameters to run this example in your environment:
- application-id – The application ID of your EMR Serverless application.
- execution-role-arn – Your EMR Serverless execution role. To create it, refer to Create a job runtime role.
- WARC file location – The location of your WARC files.
s3://xxxx-common-crawl/warclist/imdb_warclist/part-00000-6af12797-0cdc-4ef2-a438-cf2b935f2ffd-c000.txt
contains the filtered WARC file list, which you obtained earlier in this post.
- spark.sql.warehouse.dir – The default warehouse location (use your S3 directory).
- spark.archives – The S3 location of the prepared Conda environment.
- spark.submit.pyFiles – The prepared PySpark script sparkcc.py.
See the following code:
# 1. create conda environment
conda create -y -n imdb -c dmnapolitano python=3.7 botocore boto3 ujson requests conda-pack warcio bs4
# 2. package the conda env, and upload to s3
conda activate imdb
conda pack -o imdbenv.tar.gz
aws s3 cp imdbenv.tar.gz s3://xxxx-common-crawl/env/
# 3. upload scripts to S3
aws s3 cp imdb_extractor.py s3://xxxx-common-crawl/scripts/
aws s3 cp cc-pyspark/sparkcc.py s3://xxxx-common-crawl/scripts/
# 4. submit job to EMR Serverless
#!/bin/bash
aws emr-serverless start-job-run \
--application-id 00fdsobht2skro2l \
--execution-role-arn arn:aws:iam::xxxx:role/EMR-Serverless-JobExecutionRole \
--name imdb-retrive \
--job-driver '{
"sparkSubmit": {
"entryPoint": "s3://xxxx-common-crawl/scripts/imdb_extractor.py",
"entryPointArguments": ["--input_base_url" ,"s3://commoncrawl/", "s3://xxxx-common-crawl/warclist/imdb_warclist/part-00000-6af12797-0cdc-4ef2-a438-cf2b935f2ffd-c000.txt", "imdb_reviews", "--num_output_partitions", "1"],
"sparkSubmitParameters": "--conf spark.sql.warehouse.dir=s3://xxxx-common-crawl/output/ --conf spark.network.timeout=10000000 —conf spark.executor.heartbeatInterval=10000000 —conf spark.executor.instances=100 —conf spark.executor.cores=4 —conf spark.executor.memory=16g —conf spark.driver.memory=16g —conf spark.archives=s3://xxxx-common-crawl/env/imdbenv.tar.gz#environment —conf spark.emr-serverless.driverEnv.PYSPARK_DRIVER_PYTHON=./environment/bin/python —conf spark.emr-serverless.driverEnv.PYSPARK_PYTHON=./environment/bin/python —conf spark.executorEnv.PYSPARK_PYTHON=./environment/bin/python —conf spark.submit.pyFiles=s3://xxxx-common-crawl/scripts/sparkcc.py“
}
}'
After the job is complete, the extracted reviews are stored in Amazon S3. To check the contents, you can use Amazon S3 Select, as shown in the following screenshot.
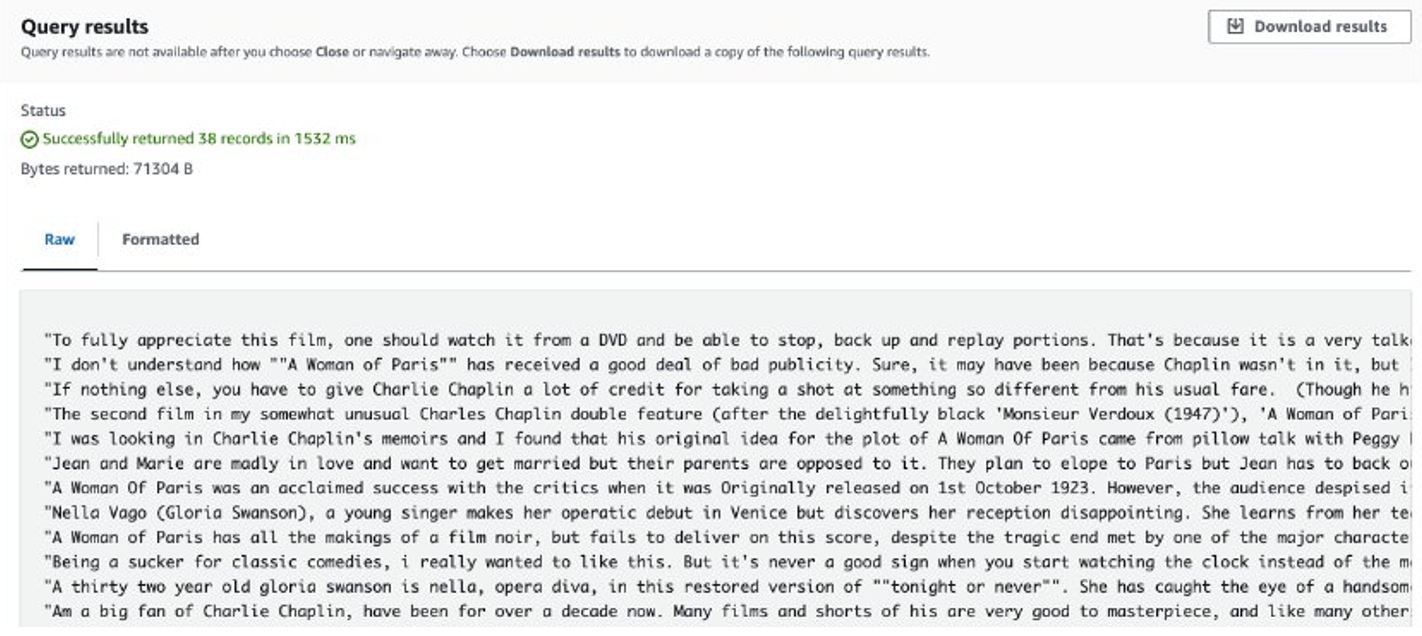
Considerations
The following are the points to consider when dealing with massive amounts of data with customized code:
- Some third-party Python libraries may not be available in Conda. In such cases, you can switch to a Python virtual environment to build the PySpark runtime environment.
- If there is a massive amount of data to be processed, try to create and use multiple EMR Serverless Spark applications to parallelize it. Each application deals with a subset of file lists.
- You may encounter a slowdown issue with Amazon S3 when filtering or processing the Common Crawl data. This is because the S3 bucket storing the data is publicly accessible, and other users may access the data at the same time. To mitigate this issue, you can add a retry mechanism or sync specific data from the Common Crawl S3 bucket to your own bucket.
Fine-tune Llama 2 with SageMaker
After the data is prepared, you can fine-tune a Llama 2 model with it. You can do so using SageMaker JumpStart, without writing any code. For more information, refer to Fine-tune Llama 2 for text generation on Amazon SageMaker JumpStart.
In this scenario, you carry out a domain adaption fine-tuning. With this dataset, input consists of a CSV, JSON, or TXT file. You need to put all review data in a TXT file. To do so, you can submit a straightforward Spark job to EMR Spark Serverless. See the following sample code snippet:
# disable generating _SUCCESS file
spark.conf.set("mapreduce.fileoutputcommitter.marksuccessfuljobs", "false")
data = spark.read.parquet("s3://xxxx-common-crawl/output/imdb_reviews/")
data.select('Key').coalesce(1).write.mode("overwrite").text("s3://xxxx-common-crawl/llama2/train/")
After you prepare the training data, enter the data location for Training data set, then choose Train.
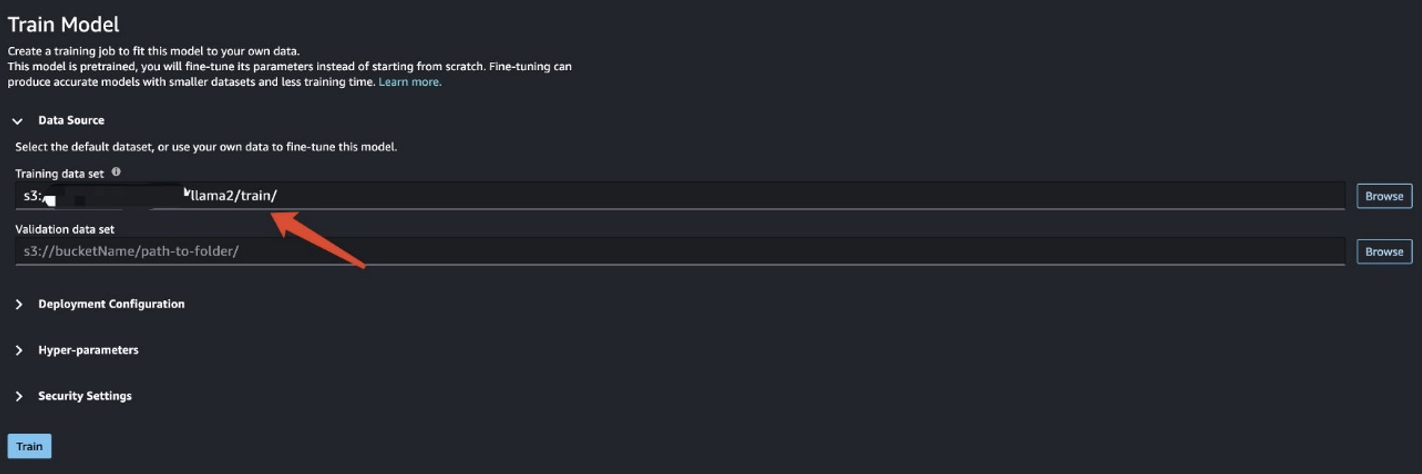
You can track the training job status.
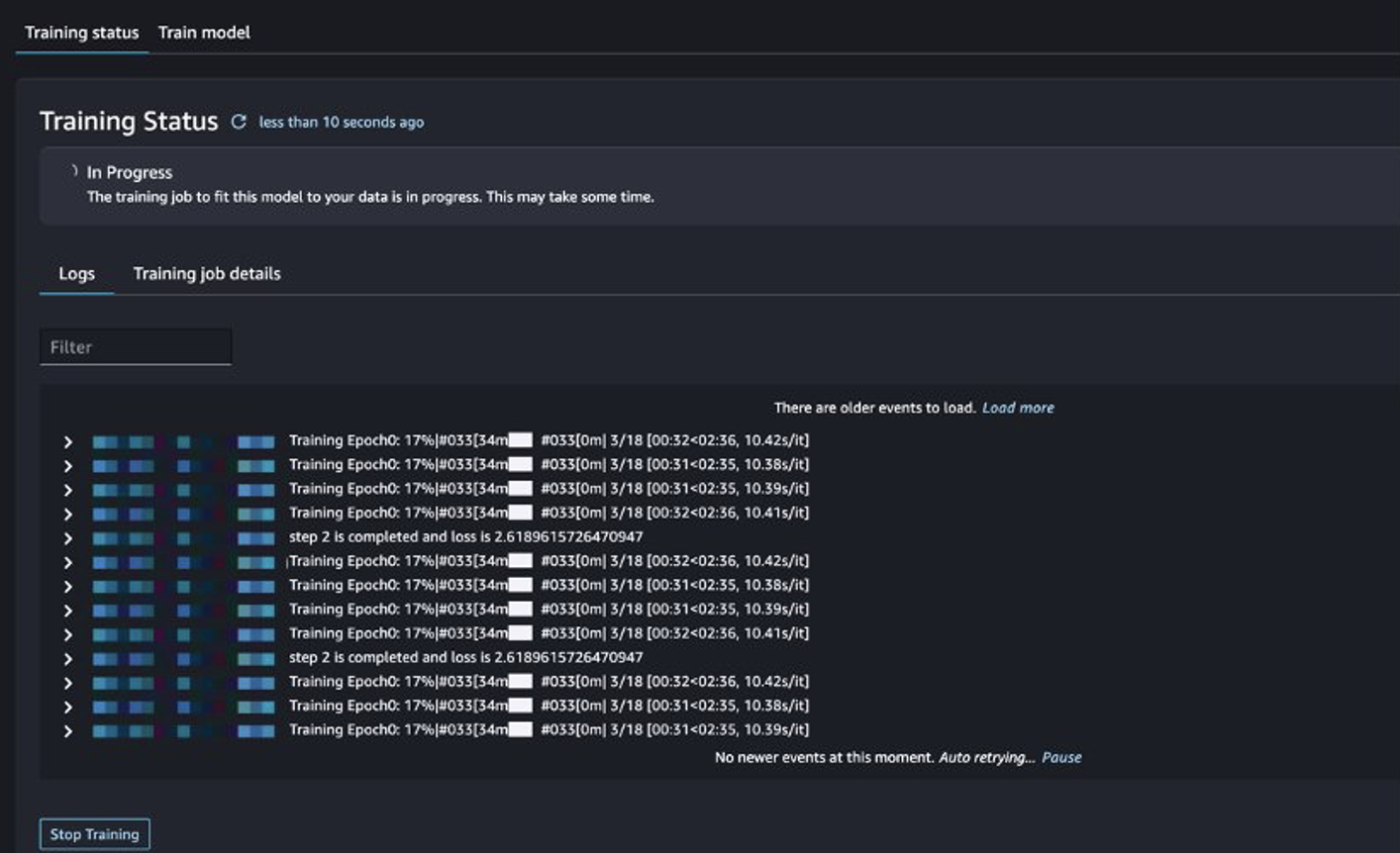
Evaluate the fine-tuned model
After training is complete, choose Deploy in SageMaker JumpStart to deploy your fine-tuned model.
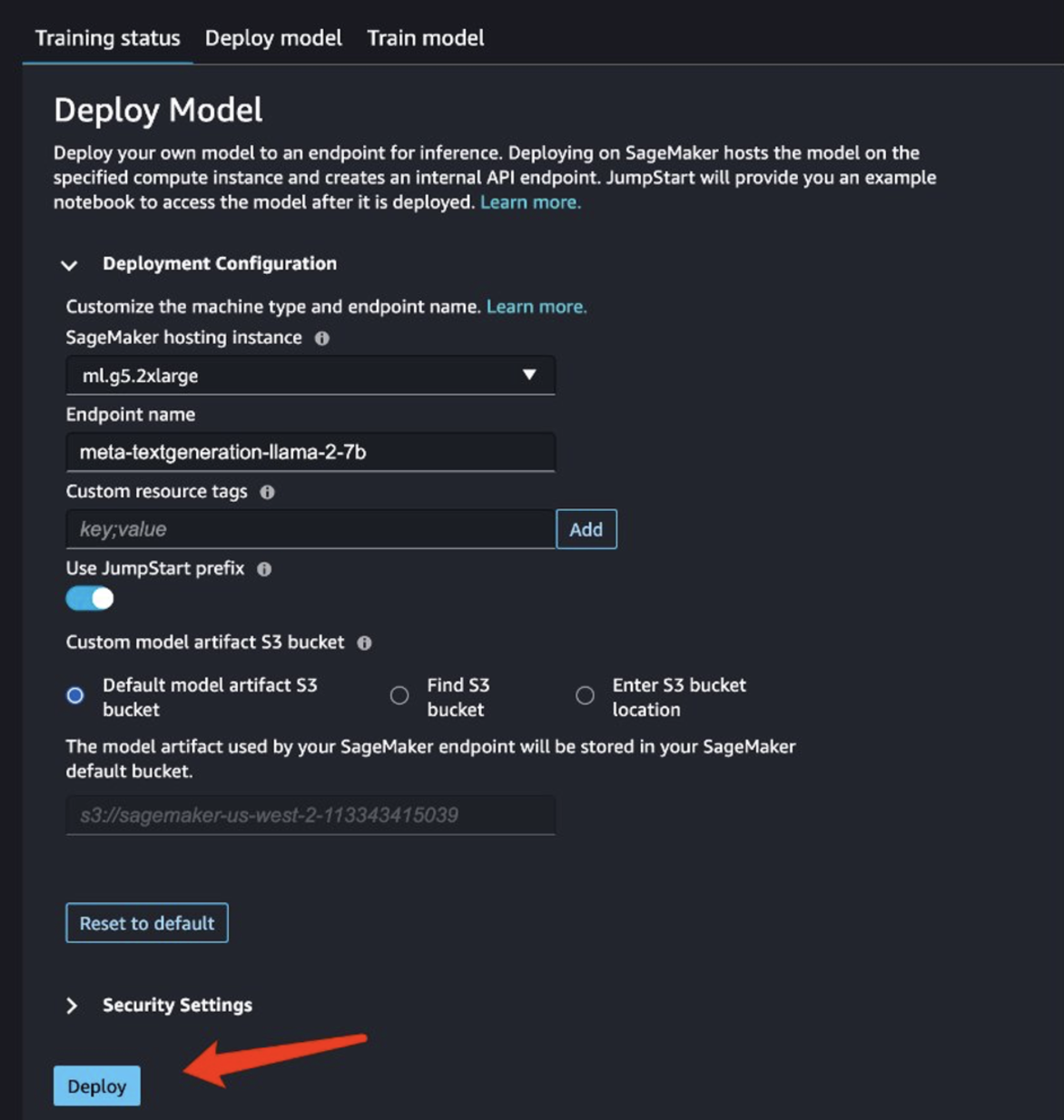
After the model is successfully deployed, choose Open Notebook, which redirects you to a prepared Jupyter notebook where you can run your Python code.
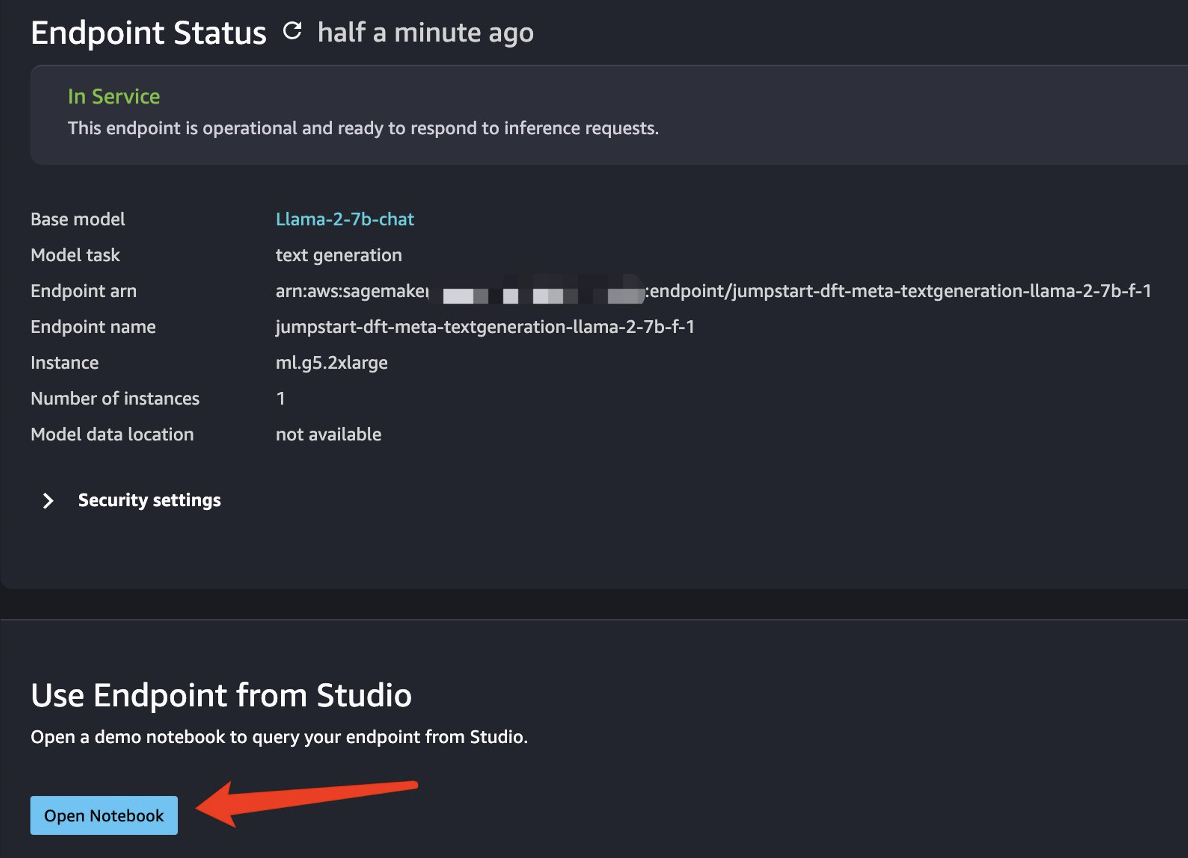
You can use the image Data Science 2.0 and the Python 3 kernel for the notebook.
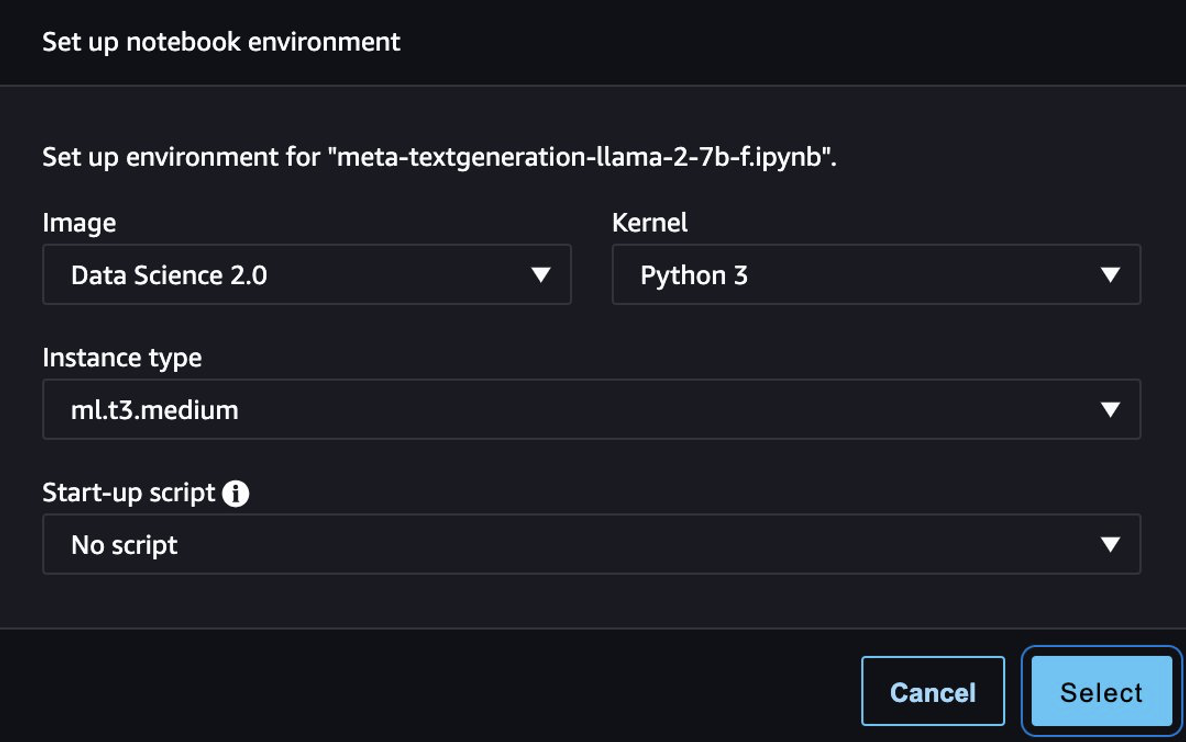
Then, you can evaluate the fine-tuned model and the original model in this notebook.
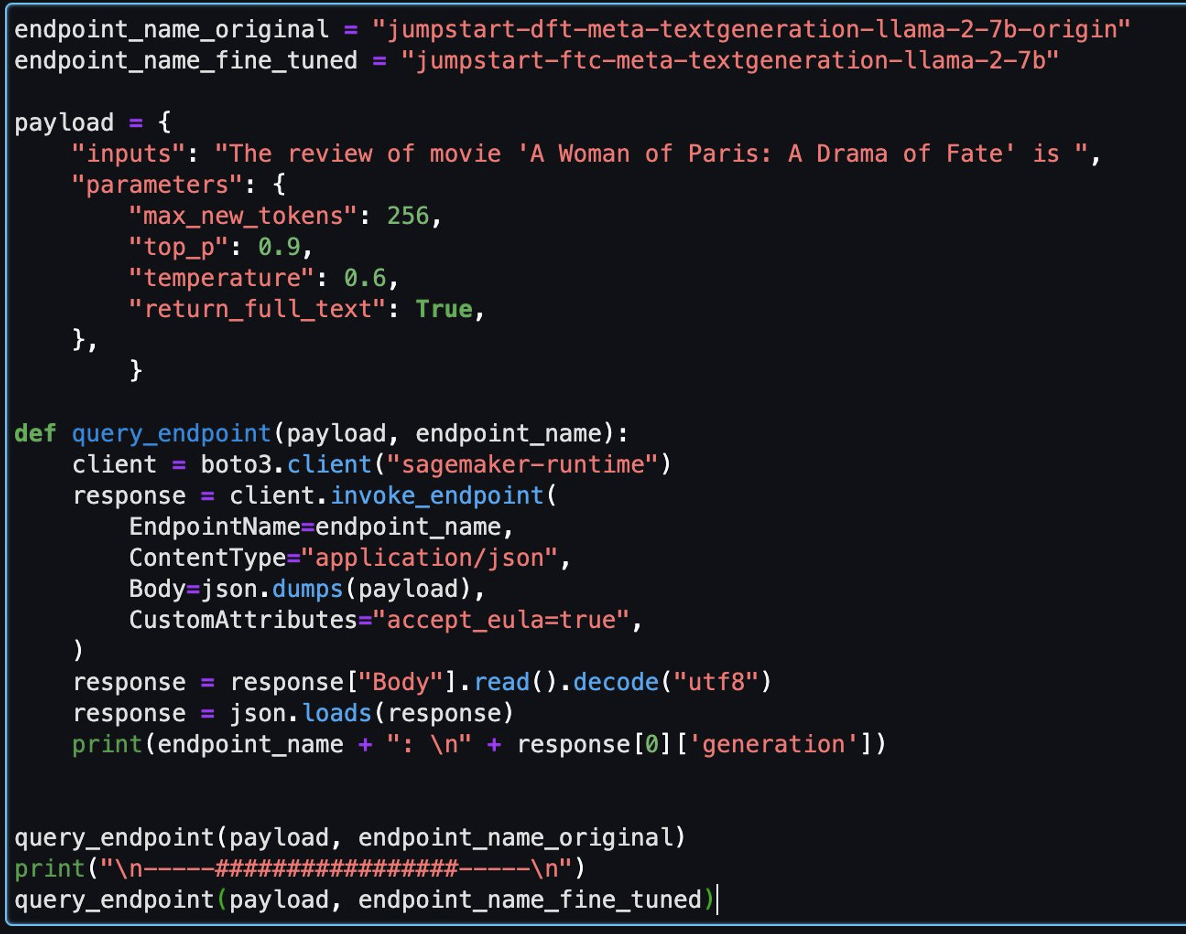
endpoint_name_original = "jumpstart-dft-meta-textgeneration-llama-2-7b-origin"
endpoint_name_fine_tuned = "jumpstart-ftc-meta-textgeneration-llama-2-7b"
payload = {
"inputs": "The review of movie 'A Woman of Paris: A Drama of Fate' is ",
"parameters": {
"max_new_tokens": 256,
"top_p": 0.9,
"temperature": 0.6,
"return_full_text": True,
},
}
def query_endpoint(payload, endpoint_name):
client = boto3.client("sagemaker-runtime")
response = client.invoke_endpoint(
EndpointName=endpoint_name,
ContentType="application/json",
Body=json.dumps(payload),
CustomAttributes="accept_eula=true",
)
response = response["Body"].read().decode("utf8")
response = json.loads(response)
print(endpoint_name + ": \n" + response[0]['generation'])
query_endpoint(payload, endpoint_name_original)
print("\n-----#################-----\n")
query_endpoint(payload, endpoint_name_fine_tuned)
The following are two responses returned by the original model and fine-tuned model for the same question.
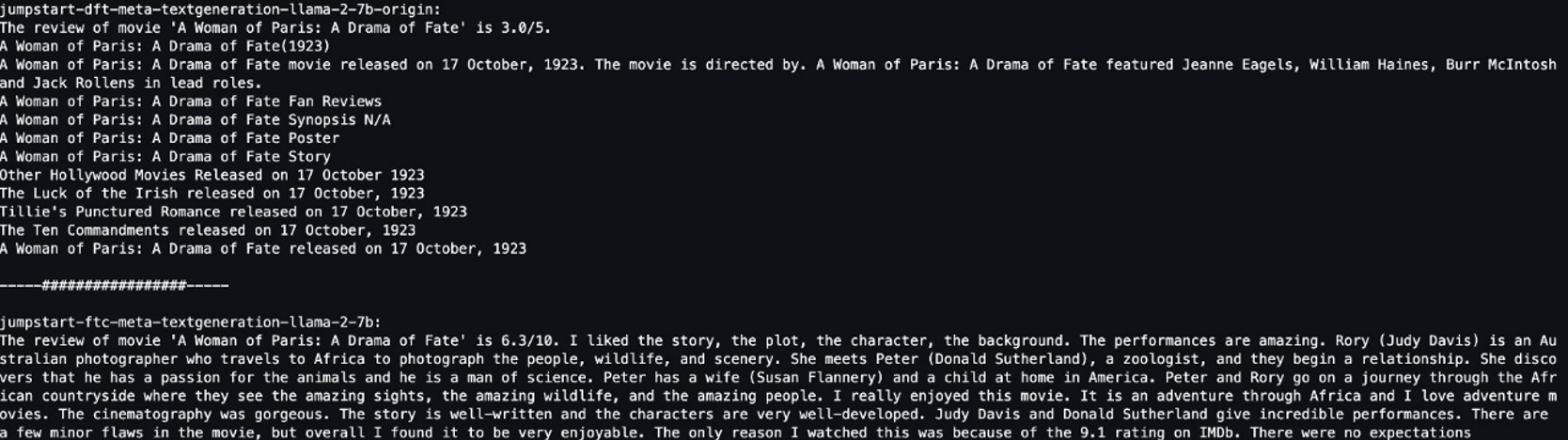
We provided both models with the same sentence: “The review of movie ‘A Woman of Paris: A Drama of Fate’ is” and let them complete the sentence.
The original model outputs meaningless sentences:
"The review of movie 'A woman of Paris: A Drama of Fate' is 3.0/5.
A Woman of Paris: A Drama of Fate(1923)
A Woman of Paris: A Drama of Fate movie released on 17 October, 1992. The movie is directed by. A Woman of Paris: A Drama of Fate featured Jeanne Eagles, William Haines, Burr McIntosh and Jack Rollens in lead rols.
..."
In contrast, the fine-tuned model’s outputs are more like a movie review:
" The review of movie 'A Woman of Paris: A Drama of Fate' is 6.3/10. I liked the story, the plot, the character, the background. The performances are amazing. Rory (Judy Davis) is an Australian photographer who travels to Africa to photograph the people, wildlife, and scenery. She meets Peter (Donald Sutherland), a zoologist, and they begin a relationship..."
Obviously, the fine-tuned model performs better in this specific scenario.
Clean up
After you finish this exercise, complete the following steps to clean up your resources:
- Delete the S3 bucket that stores the cleaned dataset.
- Stop the EMR Serverless environment.
- Delete the SageMaker endpoint that hosts the LLM model.
- Delete the SageMaker domain that runs your notebooks.
The application you created should stop automatically after 15 minutes of inactivity by default.
Generally, you don’t need to clean up the Athena environment because there are no charges when you’re not using it.
Conclusion
In this post, we introduced the Common Crawl dataset and how to use EMR Serverless to process the data for LLM fine-tuning. Then we demonstrated how to use SageMaker JumpStart to fine-tune the LLM and deploy it without any code. For more use cases of EMR Serverless, refer to Amazon EMR Serverless. For more information about hosting and fine-tuning models on Amazon SageMaker JumpStart, refer to the Sagemaker JumpStart documentation.
About the Authors
Shijian Tang is a Analytics Specialist Solution Architect at Amazon Web Services.
Matthew Liem is a Senior Solution Architecture Manager at Amazon Web Services.
Dalei Xu is a Analytics Specialist Solution Architect at Amazon Web Services.
Yuanjun Xiao is a Senior Solution Architect at Amazon Web Services.