AWS Architecture Blog
Ingesting Automotive Sensor Data using DXC RoboticDrive Ingestor on AWS
This post was co-written by Pawel Kowalski, a Technical Product Manager for DXC RoboticDrive and Dr. Max Böhm, a software and systems architect and DXC Distinguished Engineer.
To build the first fully autonomous vehicle, L5 standard per SAE, auto-manufacturers collected sensor data from test vehicle fleets across the globe in their testing facilities and driving circuits. It wasn’t easy collecting, ingesting and managing massive amounts of data, and that’s before it’s used for any meaningful processing, and training the AI self-driving algorithms. In this blog post, we outline a solution using DXC RoboticDrive Ingestor to address the following challenges faced by automotive original equipment manufacturers (OEMs) when ingesting sensor data from the car to the data lake:
- Each test drive alone can gather hundreds of terabytes of data per fleet. A few vehicles doing test fleets can produce petabyte(s) worth of data in a day.
- Vehicles collect data from all the sensors into local storage with fast disks. Disks must offload data to a buffer station and then to a data lake as soon as possible to be reused in the test drives.
- Data is stored in binary file formats like ROSbag, ADTF or MDF4, that are difficult to read for any pre-ingest checks and security scans.
- On-premises (ingest location) to Cloud data ingestion requires a hybrid solution with reliable connectivity to the cloud.
- Ingesting process depositing files into a data lake without organizing them first can cause discovery and read performance issues later in the analytics phase.
- Orchestrating and scaling an ingest solution can involve several steps, such as copy, security scans, metadata extraction, anonymization, annotation, and more.
Overview of the RoboticDrive Ingestor solution
The DXC RoboticDrive Ingestor (RDI) solution addresses the core requirements as follows:
- Cloud ready: Implements a recommended architectural pattern where data from globally located logger copy stations are ingested into the cloud data lake in one or more AWS Regions over AWS Direct Connect or Site-to-Site VPN connections.
- Scalable: Runs multiple steps as defined in an Ingest Pipeline, containerized on Amazon EKS based Amazon EC2 compute nodes that can auto-scale horizontally and vertically on AWS. Steps can be for example, Virus Scan, Copy to Amazon S3, Quality Check, Metadata Extraction, and others.
- Performant: Uses the Apache Spark-based DXC RoboticDrive Analyzer component to process large amounts of sensor data files efficiently in parallel, which will be described in a future blog post. Analyzer can read and processes native automotive file formats, extract and prepare metadata, and populate metadata into the DXC RoboticDrive Meta Data Store. The maximum throughput of network and disk is leveraged and data files are placed on the data lake following a hierarchical prefix domain model, that aligns with the best practices around S3 performance.
- Operations ready: Containerized deployment, custom monitoring UI, visual workflow management with MWAA, Amazon CloudWatch based monitoring for virtual infrastructure and cloud native services.
- Modular: Programmatically add or customize steps into the managed Ingest Pipeline DAG, defined in Amazon Managed Workflows for Apache Airflow (MWAA).
- Security: IAM based access policy and technical roles, Amazon Cognito based UI authentication, virus scan, and an optional data anonymization step.
The ingest pipeline copies new files to S3 followed by user configurable steps, such as Virus Scan or Integrity Checks. When new files have been uploaded, cloud native S3 event notifications generate messages in an Amazon SQS queue. These are then consumed by serverless AWS Lambda functions or by containerized workloads in the EKS cluster, to asynchronously perform metadata extraction steps.
Walkthrough
There are two main areas of the ingest solution:
- On-Premises devices – these are dedicated copy stations in the automotive OEM’s data center where data cartridges are physically connected. Copy stations are easily configurable devices that automatically mount inserted data cartridges and share its content via NFS. At the end of the mounting process, they call an HTTP post API to trigger the ingest process.
- Managed Ingest Pipeline – this is a Managed service in the cloud to orchestrate the ingest process. As soon as a cartridge is inserted into the Copy Station, mounted and shared via NFS, the ingest process can be started.
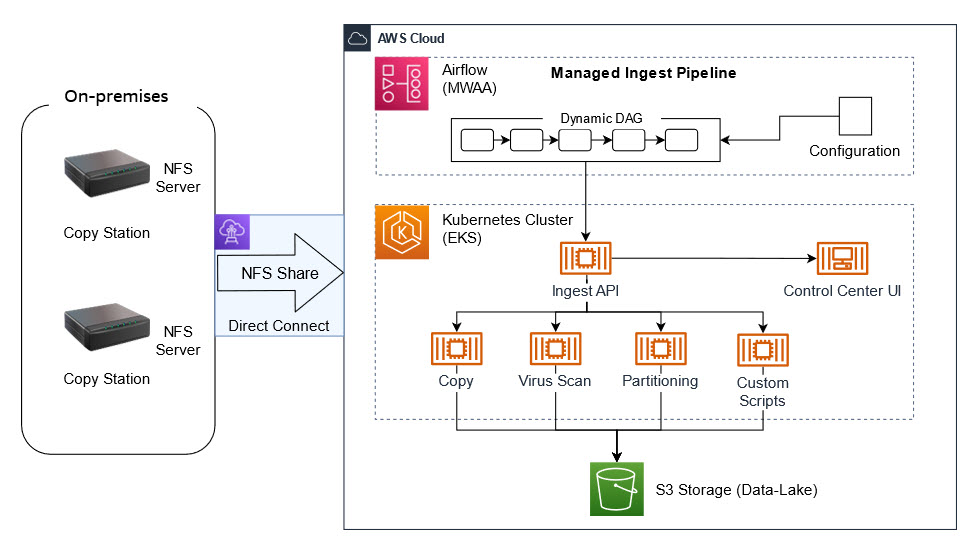
Figure 1 – Architecture showing the DXC RoboticDrive Ingestor (RDI) solution
Depending on the customer’s requirements, the ingest process may differ. It can be easily modified using predefined Docker images. A configuration file defines what features from the Ingest stack should be used. It can be a simple copy from the source to the cloud but we can easily extend it with additional steps, such as a virus scan, special data partitioning, data quality checks on even scripts provided by the automotive OEM.
The ingest process is orchestrated by a dedicated MWAA pipeline built dynamically from the configuration file. We can easily track the progress, see the logs and, if required, stop or restart the process. Progress is tracked at a detailed level, including the number and volume of data uploaded, remaining files, estimated finish time, and ingest summary, all visualized in a dedicated ingest UI.
Prerequisites
- AWS Site-To-Site VPN (in production an AWS Direct Connect connection is used instead)
- Amazon Managed Workflows for Apache Airflow (MWAA)
- Managed Kubernetes Service (Amazon EKS)
- Data provided on source side, shared via a Network File System (NFS)
Ingest pipeline
To build an MWAA pipeline, we need to choose from prebuilt components of the ingestor and add them into the JSON configuration, which is stored in an Airflow variable:
{
"tasks" : [
{
"name" : "Virus_Scan",
"type" : "copy",
"srcDir" : "file:///mnt/ingestor/CopyStation1/",
"quarantineDir" : "s3a://k8s-ingestor/CopyStation1/quarantine/”
},
{
"name" : "Copy_to_S3",
"type" : "copy",
"srcDir" : "file:///mnt/ingestor/car01/test.txt",
"dstDir" : "s3a://k8s-ingestor/copy_dest/",
"integrity_check" : false
},
{
"name" : "Quality_Check",
"type" : "copy",
"srcDir" : "file:///mnt/ingestor/car01/test.txt",
"InvalidFilesDir" : "s3a://k8s-ingestor/CopyStation1/invalid/",
}
]
}
The final ingest pipeline, a Directed Acyclic Graph (DAG), is built automatically from the configuration and changes are visible within seconds:
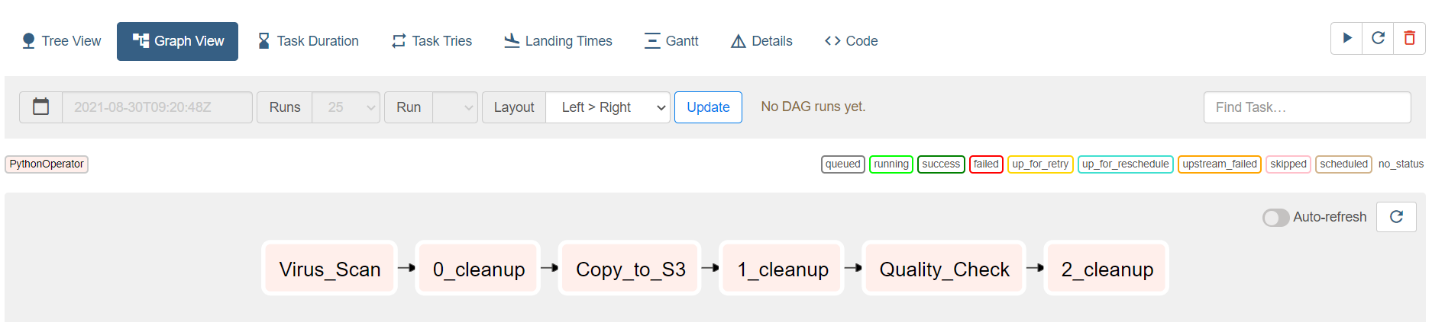
Figure 2 – Screenshot showing the final ingest pipeline, a Directed Acyclic Graph (DAG)
If required, external tools or processes can also call ingest components using the Ingest API, which is shown in the following screenshot:
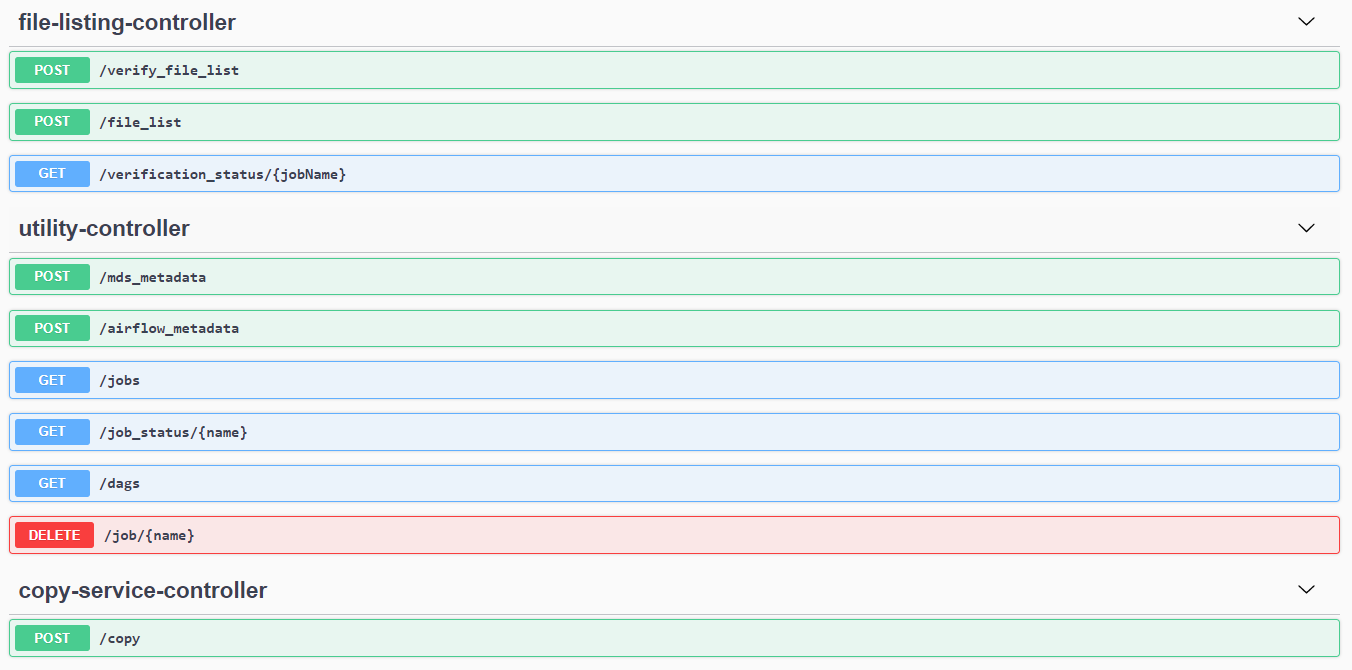
Figure 3 – Screenshot showing a tool being used to call ingest components using the Ingest API
The usual ingest process consists of the following steps:
- Ingest data source (cartridge) into the Copy Station
2. Wait for Copy Station to report proper cartridge mounting. In case a dedicated device (Copy Station) is not used, and you want to use an external disc as your data source, it can be mounted manually:
mount -o ro /dev/sda /media/disc
Here, /dev/sda is the disc containing your data and /media/disc is the mountpoint, which must also be added to /etc/exports.
3. Every time the MWAA ingest pipeline (DAG) is triggered, a Kubernetes job is created through a Helm template and Helm values which add the above data source as a persistent volume (PV) mount inside the job.
Helm template is as follows:
apiVersion: v1
kind: PersistentVolume
metadata:
name: {{ .name }}
namespace: {{ .Values.namespace }}
spec:
capacity:
storage: {{ .storage }}
accessModes:
- {{ .accessModes }}
nfs:
server: {{ .server }}
path: {{ .path }}
Helm values:
name: "YourResourceName"
server: 10.1.1.44
path: "/cardata"
mountPath: "/media/disc"
storage: 100Mi
accessModes: ReadOnlyMany
4. Trigger ingest manually from MWAA UI – this can also be automatically triggered from a Copy Station by calling MWAA API:
curl -X POST "https://roboticdrive/api/v1/dags/ingest_dag/dagRuns" -H "accept: application/json" -H "Content-Type: application/json" -d "{\"dag_run_id\":\"IngestDAG\"}"
5. Watch ingest progress – this can be omitted as the ingest results, such as success, failure, can be notified by email or reported to a ticketing system.
6. Once successfully uploaded, turn off the Copy Station manually or it can be the last task of a flow in the following ingest DAG:
(…)
def ssh_operator(task_identifier: str, cmd: str):
return SSHOperator(task_id=task_identifier,
command=cmd + ' ',
remote_host=CopyStation,
ssh_hook=sshHook,
trigger_rule=TriggerRule.NONE_FAILED,
do_xcom_push=True,
dag= IngestDAG)
host_shutdown = ssh_operator(task_identifier='host_shutdown', \
cmd='sudo shutdown –h +5 &')
When launched, you can track the progress and basic KPIs on the Monitoring UI:
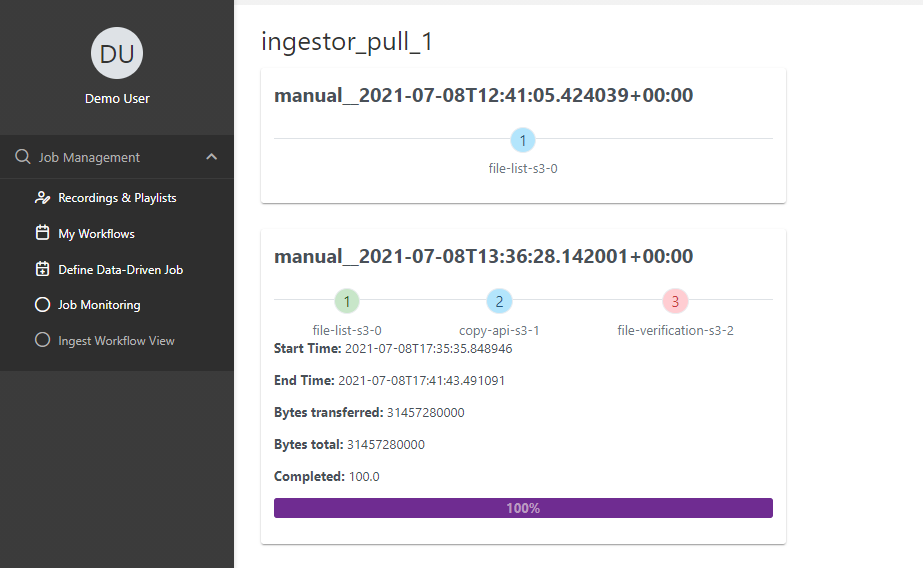
Figure 4 – Screenshot basic KPIs on the Monitoring UI
Conclusion
In this blog post, we showed how to set up the DXC RoboticDrive Ingestor. This solution was designed to overcome several data ingestion challenges and resulted in the following positive results:
- Time-to-Analyze KPI decreased by 30% in our tests, local offload steps become obsolete, and data offloaded from cars were accessible on a globally accessible data-lake (S3) for further processing and analysis.
- MWAA integrated with EKS made the solution flexible, fault tolerant, as well as easy to manage and maintain.
- Autoscaling the compute capacity as needed for any pre-processing steps within ingestion, helped with boosting performance and productivity.
- On-premises to AWS cloud connectivity options provided flexibility in configuring and scaling (up and down), and provides optimal performance and bandwidth.
We hope you found this solution insightful and look forward to hearing your feedback in the comments!