AWS Public Sector Blog
Geo-Diverse Open Training Data as a Global Public Good
Radiant Earth Foundation is a nonprofit focused on delivering open geospatial data and analytics to the global development community (GDC) in support of their mission to address the Sustainable Development Goals (SDGs) and other key targets. Radiant Earth supports GDC by aggregating open geospatial data and providing access through its cloud-based platform, generating open Earth Observation (EO) machine learning tools and training data libraries, and creating new metadata standards through its MLHub Earth initiative. Additionally, the organization offers training resources to support capacity development and expertise in the geospatial and remote sensing sciences.
As part of the Amazon Sustainability Data Initiative, we invited Hamed Alemohammad, Chief Data Scientist at Radiant Earth, to share how the organization is using open data and the Amazon Web Services (AWS) Cloud to support the global development community.
Machine learning in support of the SDGs
To effectively leverage open EO data and analytics in support of the SDGs, we turn raw EO data into insights that can guide the decisions required to create a sustainable future. Machine learning is an important part of that process but has one major drawback – the lack of geo-diverse training datasets. Radiant Earth is actively working to fill that gap.
Gaps in training data catalogs
Training data is the building block of machine learning techniques, and the resulting models and tools are only as good as the training data used to develop them. To accurately detect land cover changes, such as urban growth, or build models that can successfully identify crop types from EO, developers and scientists need large and diverse training datasets to guide their predictive algorithms. Training data is a set of satellite observations and known output pairs from past events or the ones in similar regions. The larger and more diverse the training data, the better the predictive algorithms are in identifying patterns and anomalies (e.g., deforestation). The challenge is that existing geospatial training data catalogs are focused on the Global North.
Developed countries have long-term datasets thanks to open data policies of satellite missions from NASA, USGS, and NOAA as well as extensive ground truth data gathered by different agencies such as USDA’s National Agricultural Statistics program. This data coupled with extensive expertise resulted in science-based applications that address challenges like food security, water management, and deforestation. During the last decade, many of these ground truth and satellite data have been processed and compiled as training data to foster the application of EO data.
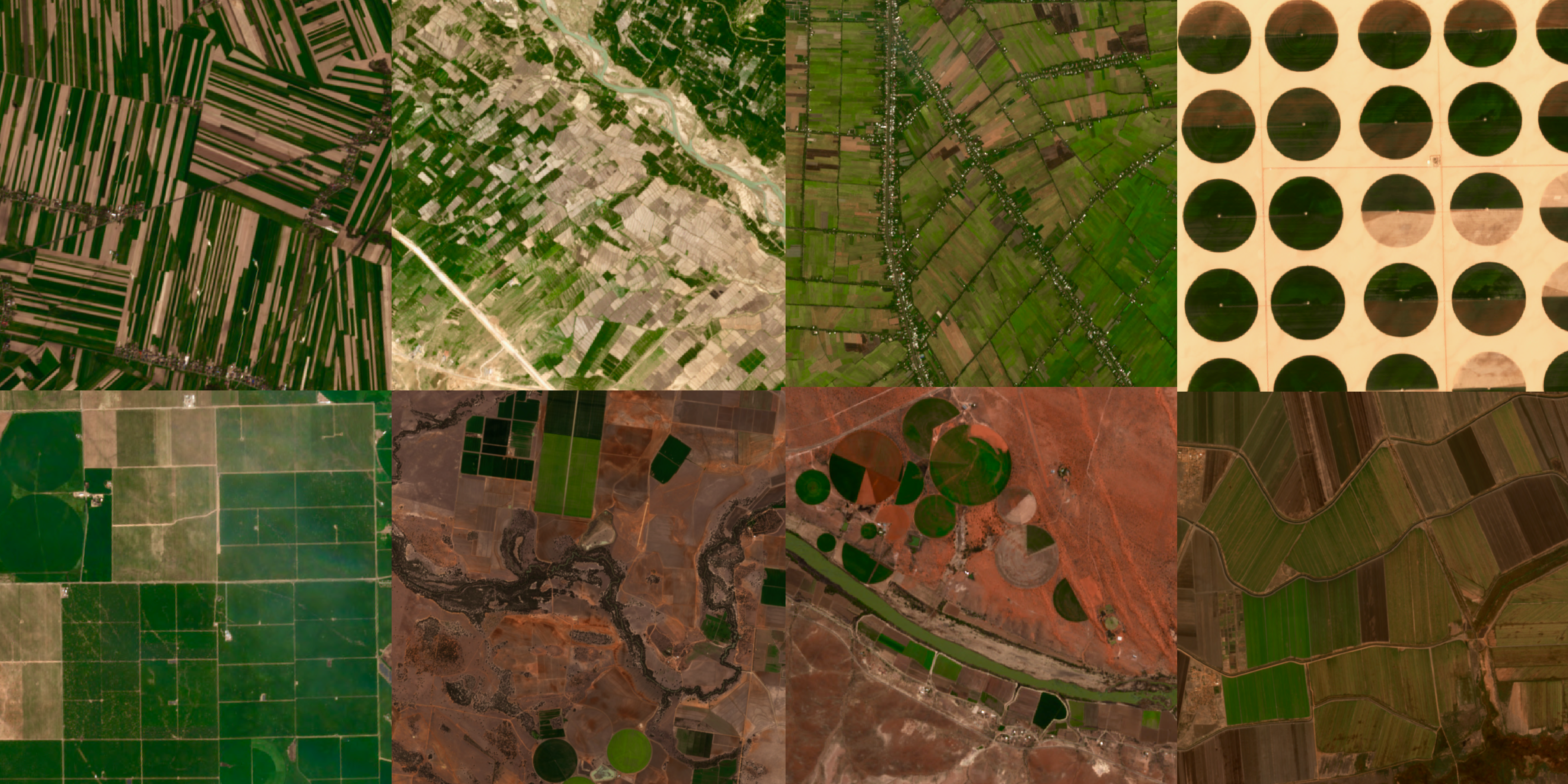
Diversity of cropping patterns at global scale. Images from Sentinel-2 satellites.
In comparison, countries in the Global South are experiencing a deficiency of data, especially high-quality data needed to measure progress towards meeting the SDGs. Machine learning techniques can be used with EO data to build models for monitoring SDGs; however, existing training data catalogs are skewed towards developed countries, leading to machine learning models that give biased or incorrect results. In other words, a model built on training data from one part of the world cannot be applied in a different ecosystem.
If we want to leverage EO data to innovate for sustainable development globally, we must first focus on generating thematic training datasets through a collaborative effort by aggregating existing ground truth data, augmenting ground truth data with machine learning predictions, and taking advantage of transfer learning methods. Radiant Earth is actively working to fill that gap with its MLHub Earth Initiative.
MLHub Earth Commons for Earth Observation
The MLHub is a central repository of open labeled training data, models, and standards for machine learning and EO. The goal is to establish a community focused on advancing the application of EO data to solve the challenges in the Global South using machine learning techniques. Radiant Earth is currently developing two datasets to be hosted on the MLHub Earth.
The first is a crop type training dataset for major crops in Africa. The second one is a global land cover training dataset of labels from a geographically representative set of regions. Both datasets are being built using the European Space Agency’s (ESA) Sentinel-2 multi-spectral optical imagery at 10 m resolution hosted on AWS through the Public Dataset Program.
The MLHub catalog is hosted on Amazon Simple Storage Service (Amazon S3) storage with an API to enable the public to search for training datasets and access them with a creative commons license. Radiant Earth uses a variety of resources on AWS including clusters of Amazon Elastic Compute Cloud (Amazon EC2) machines to read the Sentinel-2 data from the public buckets, process them, generate the training data catalogs, and store the training data on the MLHub bucket.
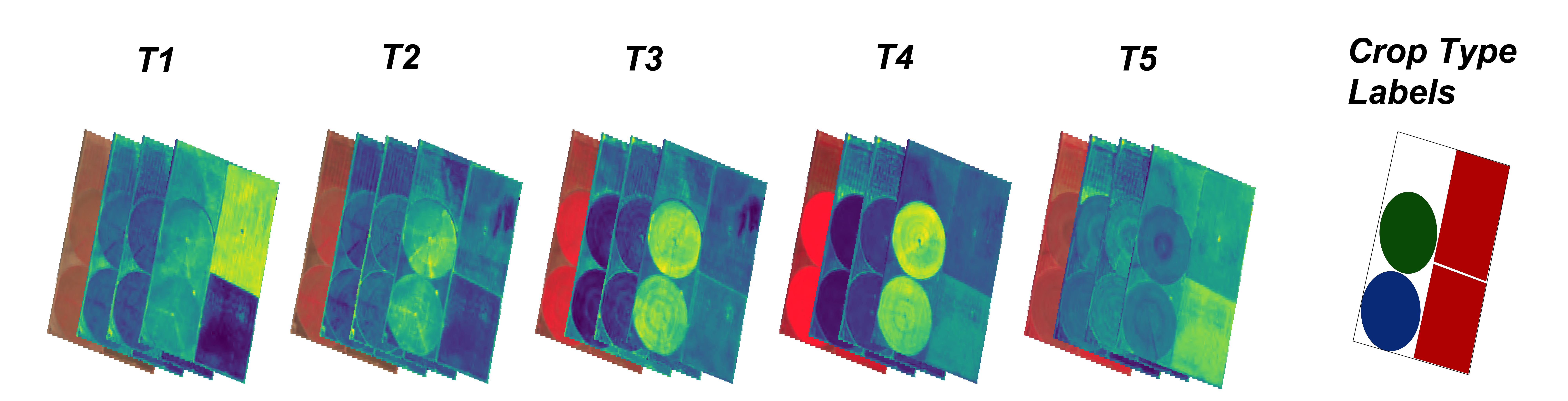
Sample training data for crop type: Collection of multispectral imagery at different times during the growing season from Sentinel-2 satellites along with the crop type label from ground truth data.
By Leveraging the AWS Cloud to house the training datasets in a cloud-friendly and ML-ready format, Radiant Earth is enabling developers to deploy machine learning models at scale using open source EO data. With the size of the petabytes of data needed to train machine learning models, it is critical to have the source data close to the computation environment, which the AWS Cloud makes possible.
Impact on the world
Radiant Earth is investing in empowering the global development community. The machine learning training datasets and the resulting classification models for global land cover and African crop type is helping to diversify applications of EO data. These applications can advance commercial agriculture operations and provide transparency to the agricultural supply chain market in developing countries. Ultimately, Radiant Earth Foundation’s goal is to create a sustained community-wide effort to capture image labels for various applications across the Global South. This can make MLHub Earth the catalyst to democratizing machine learning applications.
This work is supported by the AWS Cloud Credits for Nonprofits and the AWS Public Datasets Program.