AWS Architecture Blog
Amazon Personalize customer outreach on your ecommerce platform
In the past, brick-and-mortar retailers leveraged native marketing and advertisement channels to engage with consumers. They have promoted their products and services through TV commercials, and magazine and newspaper ads. Many of them have started using social media and digital advertisements. Although marketing approaches are beginning to modernize and expand to digital channels, businesses still depend on expensive marketing agencies and inefficient manual processes to measure campaign effectiveness and understand buyer behavior. The recent pandemic has forced many retailers to take their businesses online. Those who are ready to embrace these changes have embarked on a technological and digital transformation to connect to their customers. As a result, they have begun to see greater business success compared to their peers.
Digitizing a business can be a daunting task, due to lack of expertise and high infrastructure costs. By using Amazon Web Services (AWS), retailers are able to quickly deploy their products and services online with minimal overhead. They don’t have to manage their own infrastructure. With AWS, retailers have no upfront costs, have minimal operational overhead, and have access to enterprise-level capabilities that scale elastically, based on their customers’ demands. Retailers can gain a greater understanding of customers’ shopping behaviors and personal preferences. Then, they are able to conduct effective marketing and advertisement campaigns, and develop and measure customer outreach. This results in increased satisfaction, higher retention, and greater customer loyalty. With AWS you can manage your supply chain and directly influence your bottom line.
Building a personalized shopping experience
Let’s dive into the components involved in building this experience. The first step in a retailer’s digital transformation journey is to create an ecommerce platform for their customers. This platform enables the organization to capture their customers’ actions, also referred to as ‘events’. Some examples of events are clicking on the shopping site to browse product categories, searching for a particular product, adding an item to the shopping cart, and purchasing a product. Each of these events gives the organization information about their customer’s intent, which is invaluable in creating a personalized experience for that customer. For instance, if a customer is browsing the “baby products” category, it indicates their interest in that category even if a purchase is not made. These insights are typically difficult to capture in an in-store experience. Online shopping makes gaining this knowledge much more straightforward and scalable.
The proposed solution outlines the use of AWS services to create a digital experience for a retailer and consumers. The three key areas are: 1) capturing customer interactions, 2) making real-time recommendations using AWS managed Artificial Intelligence/Machine Learning (AI/ML) services, and 3) creating an analytics platform to detect patterns and adjust customer outreach campaigns. Figure 1 illustrates the solution architecture.
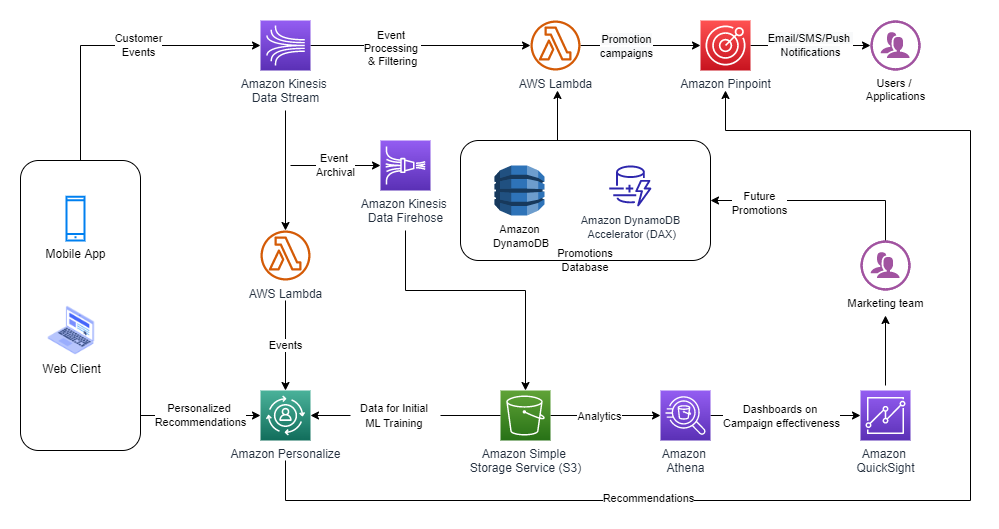
Figure 1. Digital shopping experience architecture
For this use case, let’s assume that you are the owner of a local pizzeria, and you manage deliveries through an ecommerce platform like Shopify or WooCommerce. We will walk you through how to best serve your customer with a personalized experience based on their preferences.
The proposed solution consists of the following components:
- Data collection
- Promotion campaigns
- Recommendation engine
- Data analytics
- Customer reachability
Let’s explore each of these components separately.
Data collection with Amazon Kinesis Data Streams
When a customer uses your web/mobile application to order a pizza, the application captures their activity as click-stream ‘events’. These events provide valuable insights about your customers’ behavior. You can use these insights to understand the trends and browsing pattern of prospects who visited your web/mobile app, and use the data collected for creating promotion campaigns. As your business scales, you’ll need a durable system to preserve these events against system failures, and scale based on unpredictable traffic on your platform.
Amazon Kinesis is a Multi-AZ, managed streaming service that provides resiliency, scalability, and durability to capture an unlimited number of events without any additional operational overhead. Using Kinesis producers (Kinesis Agent, Kinesis Producer Library, and the Kinesis API), you can configure applications to capture your customer activity. You can ingest these events from the frontend, and then publish them to Amazon Kinesis Data Streams.
Let us start by setting up Amazon Kinesis Data Streams to capture the real-time sales transactions from the online channels like a portal or mobile app. For this blog post, we have used the Kaggle’s public data set as a reference. Figure 2 illustrates a snapshot of sample data to build personalized recommendations for a customer.

Figure 2. Sample sales transaction data
Promotion campaigns with AWS Lambda
One way to increase customer conversion is by offering discounts. When the customer adds a pizza to their cart, you want to make sure they are receiving the best deal. Let’s assume that by adding an additional item, your customer will receive the best possible discount. Just by knowing the total cost of added items to the cart, you can provide these relevant promotions to this customer.
For this scenario, the AWS Lambda service polls the Amazon Kinesis Data Streams to read all the events in the stream. It then matches the events based on your criteria of items in the cart. In turn, these events will be processed by the Lambda function. The Lambda function will read your up-to-date promotions stored in Amazon DynamoDB. As an option, caching recent or most popular promotions will improve your application response time, as well as improve the customer experience on your platform. Amazon DynamoDB DAX is an integrated caching for DynamoDB that caches the most recent or popular promotions or items.
For example, when the customer added the items to their shopping cart, Lambda will send promotion details to them based on the purchase amount. This can be for free shipping or discount of a certain percentage. Figure 3 illustrates the snapshot of sample promotions.
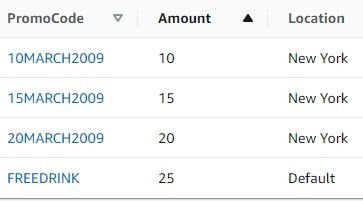
Figure 3. Promotions table in DynamoDB
Recommendations engine with Amazon Personalize
In addition to sharing these promotions with your customer, you may also want to share the recommended add-ons. In order to understand your customer preferences, you must gather historical datasets to determine patterns and generate relevant recommendations. Since web activity consists of millions of events, this would be a daunting task for humans to review, determine the patterns, and make recommendations. And since user preferences change, you need a system that can use all this volume of data and provide accurate predictions.
Amazon Personalize is a managed AI/ML service that will help you to train an ML model based on datasets. It provides an inference point for real-time recommendations prior to having ML experience. Based on the datasets, Amazon Personalize also provides recipes to generate recommendations. As your customers interact on the ecommerce platform, your frontend application calls Amazon Personalize inference endpoints. It then retrieves a set of personalized recommendations based on your customer preferences.
Here is the sample Python code to display the list of available recommenders, and associated recommendations.
import boto3
import json
client = boto3.client('personalize')
# Connect to the personalize runtime for the customer recommendations
recomm_endpoint = boto3.client('personalize-runtime')
response = recomm_endpoint.get_recommendations(itemId='79323P',
recommenderArn='arn:aws:personalize:us-east-1::recommender/my-items',
numResults=5)
print(json.dumps(response['itemList'], indent=2))
[
{
"itemId": "79323W"
},
{
"itemId": "79323GR"
},
{
"itemId": "79323LP"
},
{
"itemId": "79323B"
},
{
"itemId": "79323G"
}
]
You can use Amazon Kinesis Data Firehose to read the data near real time from the Amazon Kinesis Data Streams collected the data from the front-end applications. Then you can store this data in Amazon Simple Storage Service (S3). Amazon S3 is peta-byte scale storage help you scale and acts as a repository and single source of truth. We use S3 data as seed data to build a personalized recommendation engine using Amazon Personalize. As your customers interact on the ecommerce platform, call the Amazon Personalize inference endpoint to make personalized recommendations based on user preferences.
Customer reachability with Amazon Pinpoint
If a customer adds products to their cart but never checks out, you may want to send them a reminder. You can set up an email to suggest they re-order after a period of time after their first order. Or you may want to send them promotions based on their preferences. And as your customers’ behavior changes, you probably want to adapt your messaging accordingly.
Your customer may have a communication preference, such as phone, email, SMS, or in-app notifications. If an order has an issue, you can inform the customer as soon as possible using their preferred method of communication, and perhaps follow it up with a discount.
Amazon Pinpoint is a flexible and scalable outbound and inbound marketing communications service. You can add users to Audience Segments, create reusable content templates integrated with Amazon Personalize, and run scheduled campaigns. With Amazon Pinpoint journeys, you can send action or time-based notifications to your users.
The following workflow shown in Figure 4, illustrates customer communication workflow for promotion. A journey is created for a cohort of college students: a “Free Drink” promotion is offered with a new order. You can send this promotion over email. If the student opens the email, you can immediately send them a push notification reminding them to place an order. But if they didn’t open this email, you could wait three days, and follow up with a text message.
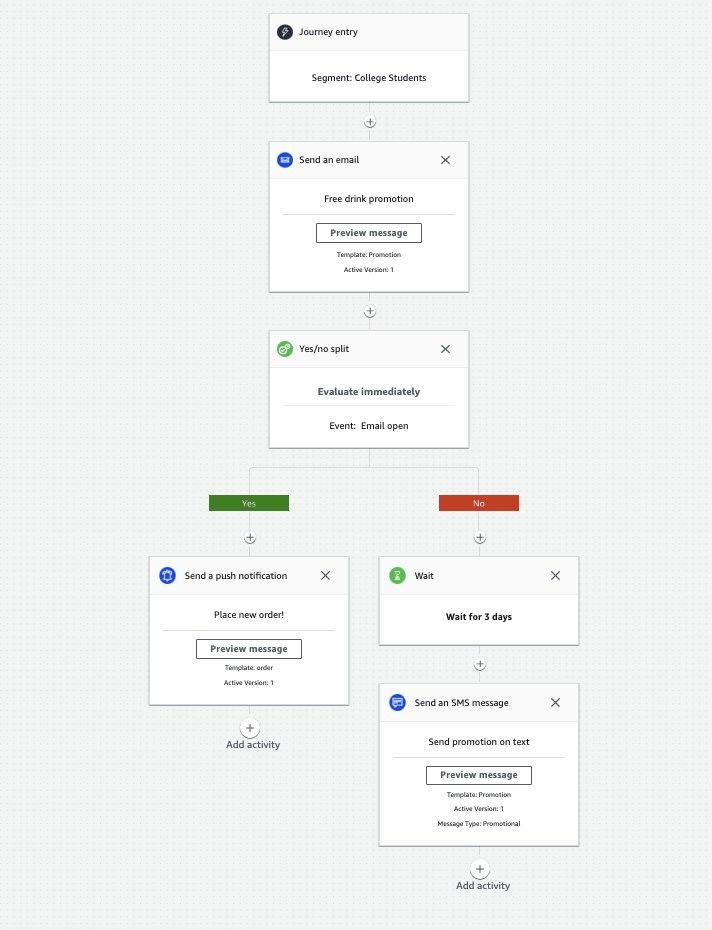
Figure 4. Promotion workflow in Amazon Pinpoint
Data analytics with Amazon Athena and Amazon QuickSight
To understand the effectiveness of your campaigns, you can use S3 data as a source for Amazon Athena. Athena is an interactive query service that analyzes data in Amazon S3 using standard SQL. Athena is serverless, so there is no infrastructure to manage, and you pay only for the queries that you run.
There are different ways to create visualizations in Amazon QuickSight. For instance, you can use Amazon S3 as a data lake. One option is to import your data into SPICE (Super-fast, Parallel, In-memory Calculation Engine) to provide high performance and concurrency. You can also create a direct connection to the underlying data source. For this use case, we choose to import to SPICE, which provides faster visualization in a production setup. Schedule consistent refreshes to help ensure that dashboards are referring to the most current data.
Once your data is imported to your SPICE, review QuickSight’s visualization dashboard. Here, you’ll be able to choose from a wide variety of charts and tables, while adding interactive features like drill downs and filters.
The process following illustrates how to create a customer outreach strategy using ZIP codes, and allocate budgets to the marketing campaigns accordingly. First, we use this sample SQL command that we ran in Athena to query for top 10 pizza providers. The results are shown in Figure 5.
SELECT name, count(*) as total_count FROM "analyticsdemodb"."fooddatauswest2"
group by name
order by total_count desc
limit 10
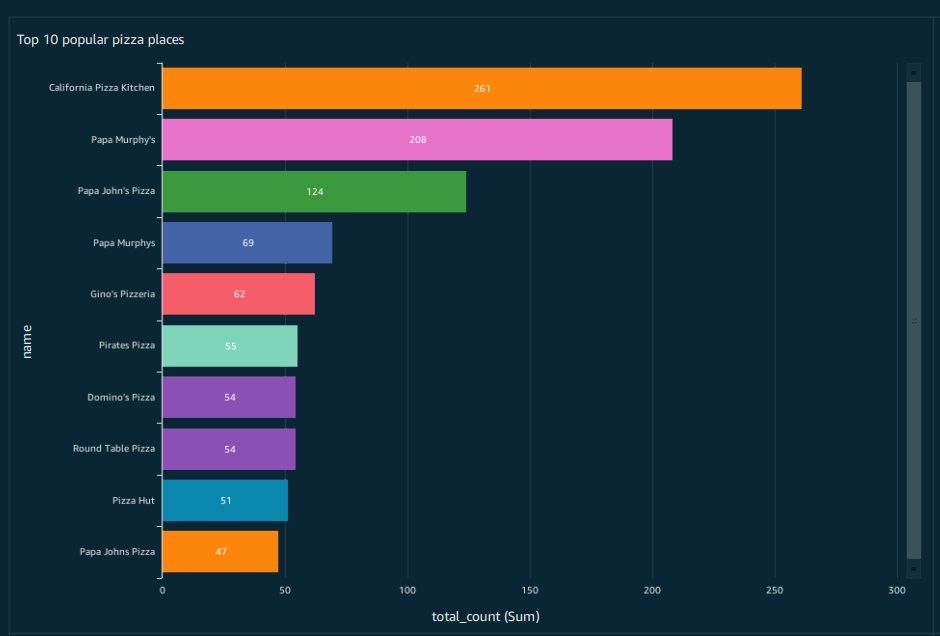
Figure 5. Athena query results for top 10 pizza providers
Second, here is the sample SQL command that we ran in Athena to find Total pizza counts by postal code (ZIP code). Figure 6 shows a visualization to help create customer outreach strategy per ZIP codes and budget the marketing campaigns accordingly.
SELECT postalcode, count(*) as total_count FROM "analyticsdemodb"."fooddatauswest2"
where postalcode is not null
group by postalcode
order by total_count desc limit 50;
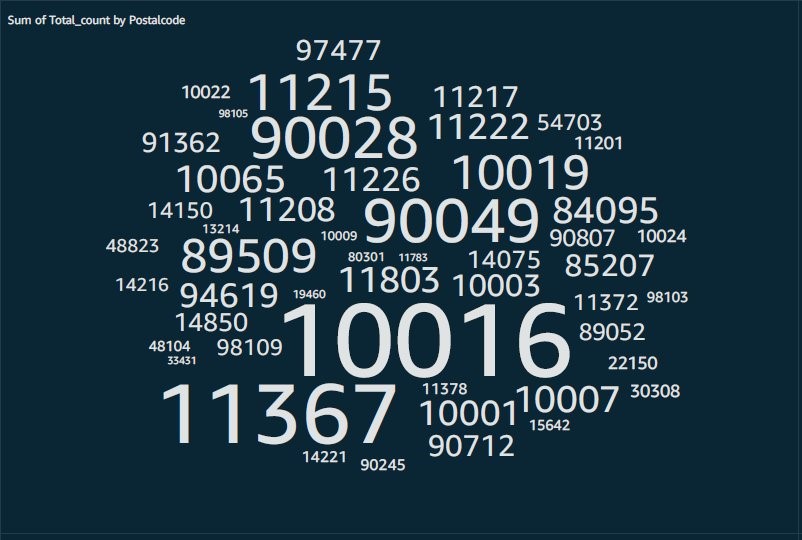
Figure 6. QuickSight visualization showing pizza orders by zip codes
Conclusion
AWS enables you to build an ecommerce platform and scale your existing business with minimal operational overhead and no upfront costs. You can augment your ecommerce platform by building personalized recommendations and effective marketing campaigns based on your customer needs. The solution approach provided in the blog will help organizations build re-usable architecture pattern and personalization using AWS managed services.